Abstract
The COVID-19 pandemic brings many unexpected disruptions, such as frequently shifting markets and limited human workforce, to manufacturers. To stay competitive, flexible and real-time manufacturing decision-making strategies are needed to deal with such highly dynamic manufacturing environments. One essential problem is dynamic resource allocation to complete production tasks, especially when a resource disruption (e.g. machine breakdown) occurs. Though multi-agent methods have been proposed to solve the problem in a flexible and agile manner, the agent internal decision-making process and resource uncertainties have rarely been studied. This work introduces a model-based resource agent (RA) architecture that enables effective agent coordination and dynamic agent decision-making. Based on the RA architecture, a rescheduling strategy that incorporates risk assessment via a clustering agent coordination strategy is also proposed. A simulation-based case study is implemented to demonstrate dynamic rescheduling using the proposed multi-agent framework. The results show that the proposed method reduces the computational efforts while losing some throughput optimality compared to the centralised method. Furthermore, the case study illustrates that incorporating risk assessment into rescheduling decision-making improves the throughput.
Disclosure statement
The authors report there are no competing interests to declare.
Data availability statement
The authors confirm that the data supporting the findings of this study are available within the article and its supplementary materials.
Notes
1 For simplicity, the symbol is used to represent the sub-sequence relationship in this work.
Additional information
Funding
Notes on contributors
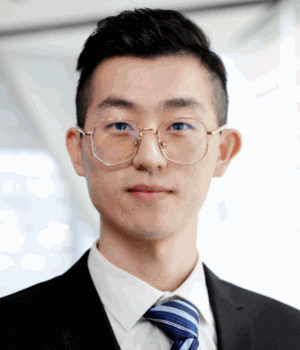
Mingjie Bi
Mingjie Bi received the B.S. degree in Marine Engineering from Huazhong University of Science and Technology, China, in 2018, and the M.S. degree in Mechanical Engineering from the University of Michigan, Ann Arbor, in 2020, where he is currently pursuing the Ph.D. degree in Robotics Department. His research interests include system-level control, multi-agent systems, smart manufacturing, agile supply chain, and robotics.
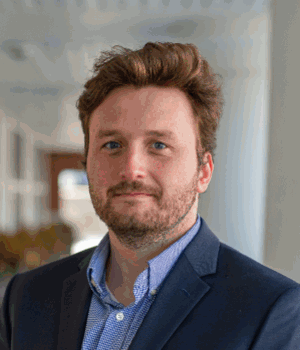
Ilya Kovalenko
Ilya Kovalenko received the B.S. degree in mechanical engineering from the Georgia Institute of Technology, Atlanta, GA, USA, in 2015, and the M.S. and Ph.D. degrees in mechanical engineering from the University of Michigan, Ann Arbor, MI, USA, in 2018 and 2020, respectively. He held a postdoctoral research position at the University of Michigan, from Fall 2020 until Fall 2021. In January 2022, he joined the Department of Mechanical Engineering and the Department of Industrial & Manufacturing Engineering at the Pennsylvania State University, where he currently holds the position of Assistant Professor. His research interests include control theory and application, with a focus on multi-agent systems, advanced manufacturing, cyber-physical systems, and robotics.
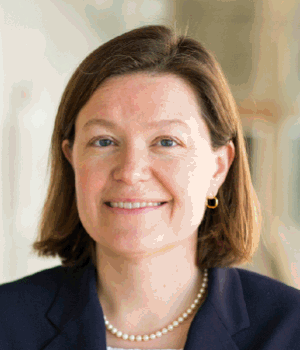
Dawn M. Tilbury
Dawn M. Tilbury received the B.S. degree (summa cum laude) in electrical engineering from the University of Minnesota, in 1989, and the M.S. and Ph.D. degrees in electrical engineering and computer sciences from the University of California, Berkeley, in 1992 and 1994, respectively. In 1995, she joined the Mechanical Engineering Department, University of Michigan, Ann Arbor, where she is currently a Professor, with a joint appointment as a Professor of EECS. In June of 2017, she became the Assistant Director for Engineering at the National Science Foundation, while maintaining her position at the University of Michigan. She has published more than 150 articles in refereed journals and conference proceedings. Her research interests include control systems, including applications to robotics and manufacturing systems.

Kira Barton
Kira Barton received the B.Sc. degree in mechanical engineering from the University of Colorado at Boulder, in 2001, and the M.Sc. and Ph.D. degrees in mechanical engineering from the University of Illinois at Urbana-Champaign, in 2006 and 2010, respectively. She held a postdoctoral research position at the University of Illinois, from Fall 2010 until Fall 2011, at which point she joined the Mechanical Engineering Department at the University of Michigan, Ann Arbor. She is currently an Associate Professor with the Department of Mechanical Engineering and a Core Robotics Faculty Member at the University of Michigan. She conducts research in modelling, sensing, and control for applications in advanced manufacturing and robotics, with a specialisation in cooperative learning and smart manufacturing. She was selected as a Miller Faculty Scholar from 2018-2021, and was a recipient of an NSF CAREER Award, in 2014, the 2015 SME Outstanding Young Manufacturing Engineer Award, the 2015 University of Illinois, Department of Mechanical Science and Engineering Outstanding Young Alumni Award, the 2016 University of Michigan, Department of Mechanical Engineering Department Achievement Award, and the 2017 ASME Dynamic Systems and Control Young Investigator Award.