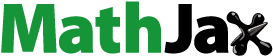
Abstract
There is a lack of a design method for the manufacturing system reconfiguration to cope with the changing demand and evolving production technologies while minimising energy consumption. The key drivers for the new industrial paradigm are flexibility and sustainable manufacturing, which have been studied independently in the prior research. The aim of this research is to study two drivers simultaneously by designing robust models and analysing manufacturing system configurations to achieve feasible solutions in any scenario that may arise due to evolving, incomplete, and unforeseen production requirements, while minimising energy usage during product manufacture. To achieve this goal, this research develops a robustly validated pre-emptive decision engineering framework (DEF) for the manufacturing system reconfiguration process to manage future uncertainty of future conditions and identifies current production vulnerabilities and alternative production portfolios. In this research, a robust RMS reconfiguration strategy is designed using a compromise decision support problem (cDSP), and decentralised decision-making designs are explored through the use of game theory. The findings provide a new production system for adaptable, responsive, and sustainable manufacturing processes in the dynamic global economy. These results can empower stakeholders to make timely design decisions that lead to significant cost savings and sustainable manufacturing.
1. Introduction
In recent years, the globalisation of markets and the dynamic global economy has heightened the demand for manufacturing processes that are adaptable, responsive, and sustainable, allowing them to effectively address the constantly evolving customer requirements (Napoleone, Pozzetti, and Macchi Citation2018). However, traditional manufacturing systems, such as lean manufacturing, agile manufacturing, Just-In-Time, dedicated manufacturing systems (DMS), flexible manufacturing systems (FMS), or cellular manufacturing systems, fall short in meeting individual customer requirements in a prompt and cost-effective manner. Moreover, external factors like climate change, pandemics, and inadequate regulations in the free market system, coupled with internal factors like random equipment failures, increase the vulnerability of global supply chains. Consequently, manufacturers may not be adequately prepared for the instability of the free-market system and the vulnerability of global supply chains, leading to significant financial losses for the national economy (Foresight, Citation2013).
To address the challenge of developing adaptable, responsive, and sustainable manufacturing processes, it is crucial to reconsider production strategies and develop a reconfigurable manufacturing system (RMS) that enhances production system stability and reduces energy consumption. The digitisation of the manufacturing industry, driven by the implementation of Industry 4.0 and the emerging Industry 5.0, offers a promising solution (Xu et al. Citation2021).
The Industry 4.0 initiative focuses on intelligent manufacturing, supply and logistics processes, encompassing vertical, horizontal, and end-to-end operations (Pereira and Romero Citation2017). On the other hand, Industry 5.0 emphasises human-centric and sustainable manufacturing (Leng et al. Citation2022). By integrating the existing manufacturing system infrastructure with the technological advancements of Industry 4.0 and Industry 5.0, disruptive changes in manufacturing paradigms are realised, enabling the achievement of agile, flexible, highly responsive, and sustainable manufacturing processes.
Responsive manufacturing systems, driven by disruptive technologies, have the potential to foster reconfigurable, adaptive, and evolving factories capable of mass customisation production, innovative business models, flexible and digital market models, enhanced levels of servitisation, and increased stakeholder engagement and collaboration (Pereira and Romero Citation2017). Moreover, sustainable manufacturing systems will seamlessly integrate physical products and cyber services across their entire lifecycle. This integration will lead to improved productivity, flexibility, and resource efficiency while simultaneously reducing waste, energy consumption, and overproduction. Furthermore, sustainable manufacturing systems will foster the creation of new forms of product value through practices such as reusing, remanufacturing, and recycling (Machado, Peter Winroth, and Ribeiro da Silva Citation2020).
The new industrial paradigm is driven by two key factors: flexibility (Driver 1) and sustainable manufacturing (Driver 2), which have been examined independently in previous research (Shrouf, Ordieres, and Miragliotta Citation2014). Flexibility plays a vital role in enabling manufacturing systems to adapt to changing demands in the global market. Three decades ago, the development of RMS was initiated through the integration of high flexibility, reconfigurability, and artificial intelligence (Koren et al. Citation1999). Reconfiguration enables manufacturing systems to seamlessly transition between products, providing the necessary flexibility to support customisation and personalisation while maintaining dynamic capacity and functionality (Battaïa, Dolgui, and Guschinsky Citation2017). However, traditional manufacturing systems are inadequate in responding to the rapid changes in demand for customised and personalised products within a free-market system. Additionally, sustainability has become increasingly important in manufacturing and design practices. Sustainable manufacturing aims to minimise environmental impacts, conserve energy and natural resources, and ensure the safety of employees, communities, and customers throughout the product life cycle. Many companies have adopted approaches such as design for environment and life cycle assessment to inform decision-making related to products, services, and sectors. Enterprises must develop dynamically stable capabilities to adapt, integrate, and reconfigure manufacturing systems in response to evolving business environments and promptly meet customer requirements while minimising energy consumption. This also influences design decisions to achieve greater energy savings (Seow and Rahimifard Citation2011).
It is evident that the challenge of adapting traditional manufacturing systems to enable both customised and personalised production amidst dynamic changes remains unresolved, presenting a formidable obstacle to the advancement of responsive manufacturing (Jiao et al. Citation2021). Furthermore, although significant efforts have been devoted to developing cost-effective reconfiguration strategies (Wang et al. Citation2018), minimising energy consumption during the manufacturing process remains a challenge for the continued progress of energy-saving technologies in manufacturing.
In this paper, our focus is on simultaneously study two drivers, namely flexibility and sustainable manufacturing, by designing robust models and analysing manufacturing system configurations. The aim is to achieve feasible solutions for various scenarios that may arise due to evolving, incomplete, and unforeseen production requirements, while minimising energy usage during product manufacture. To accomplish this, we propose the development of a responsive and sustainable RMS and responsibly innovate it. We will assess the potential of the RMS in meeting the aforementioned drivers by addressing four research objectives: (1) design a robustly validated pre-emptive decision engineering framework (DEF) for the reconfiguration process in the manufacturing system. This framework will enable the system to effectively cope with the uncertainty of future conditions, (2) analyse the generation of future scenarios and identify current production vulnerabilities, as well as alternative production portfolios. This analysis will provide insights into potential risks and opportunities for the manufacturing system; (3) design a robust RMS reconfiguration strategy using a compromise decision support problem (cDSP) approach. This strategy will facilitate the adaptation of the manufacturing system to changing requirements while considering multiple conflicting objectives, (4) utilise game theory to explore decentralised decision-making designs for manufacturing systems. This exploration will identify systematic reconfigurations that optimise decision-making processes within the manufacturing system. By addressing these research objectives, we aim to contribute to the development of a responsive and sustainable manufacturing system that can effectively adapt to changing conditions and minimise energy consumption.
The findings of this research will empower stakeholders to make informed and timely design decisions. By gaining a fundamental understanding of systematic reconfiguration strategies for manufacturing systems and processes, stakeholders will be able to achieve significant cost savings. This research will also contribute to advancing sustainable manufacturing practices, taking a step towards a more environmentally conscious approach.
The remaining sections of this paper are organised as follows. Section 2 presents a systematic literature review on reconfigurable manufacturing and sustainable manufacturing. In Section 3, the research framework is introduced, highlighting the structural and logical validity of the study as well as its overall consistency. Section 4 examines the example problem(s) utilised to validate the design method. The analysis of experimental results is discussed in Section 5. Section 6 addresses the limitations of the study and outlines potential directions for future research.
2. Literature review
To clarify the research problems in this study, a literature review is presented in this section. First, different types of manufacturing systems are introduced. Secondly, the issue of sustainability in manufacturing is discussed. Next, publications related to managing uncertainty in the manufacturing process are reviewed and analysed. Lastly, current research related to reconfigurable manufacturing is reviewed and analysed.
2.1 Manufacturing systems
Manufacturing systems are used to produce finished product from raw materials. Normally a manufacturing system contains machines, materials, material handling machines, labour and information. Different types of manufacturing systems which are applied in practices are introduced in this section including DMS, FMS, and RMS.
2.1.1 Dedicated manufacturing system (DMS)
DMS is extensively used in mass production, employing dedicated or specialised machines to produce parts or products (Renzi et al. Citation2014). It operates with a dedicated manufacturing line designed for high production rates of a specific part or product, optimising the sequence of operations for efficient performance. The fixed structures and configurations of machines in a DMS make changes time-consuming and costly (Renzi et al. Citation2014). With high production volumes, the cost per part is relatively low, resulting in cost efficiency. DMS was favoured in the past century for its cost-saving advantages. However, the advent of Industry 4.0 has transformed the manufacturing industry. Global sourcing of parts and increased demand for customised designs have diminished the capacity utilisation of DMS and its ability to meet customer demands.
2.1.2 Flexible manufacturing system (FMS)
FMS is a highly flexible manufacturing system composed of a group of processing stations, typically computer numerical control (CNC) machines, arranged in an automated machine cell. These CNC machines are interconnected through automated material storage and handling systems, controlled by an integrated computer system (Frolov et al. Citation2017). FMS is capable of producing a variety of part types. Despite its potential, FMS has not been widely adopted in practice, and many manufacturers who have implemented FMS are dissatisfied with its performance. This reluctance can be attributed to the high initial cost, lower production rates compared to DMS, and the requirement for highly skilled workers to operate the system, which incurs additional expenses and training time.
2.1.3 Reconfigurable manufacturing system (RMS)
RMS is a manufacturing approach that addresses the limitations of DMS and FMS. It enables easy and rapid changes to its capabilities and functionalities based on fluctuating part volume and variety demands. In the era of Industry 4.0, the ability to quickly adapt to new market requirements has become increasingly crucial, leading to heightened attention towards RMS. Introduced by Koren et al. (Citation1999), RMS is considered the most suitable solution for coping with changing market conditions. It typically consists of reconfigurable machines that can be easily added, removed, or adjusted, either by hardware changes or software reprogramming. According to Koren et al. (Citation1999), a RMS should possess the following characteristics: modularity, integrability, customisation, convertibility, diagnosability, and scalability.
Machado, Peter Winroth, and Ribeiro da Silva (Citation2020) provided a summary of key functionality indicators for RMS. The first indicator is cost, which encompasses capital cost, operation cost, and reconfiguration cost. Reconfiguration cost refers to the expenses involved in adjusting the system to meet new requirements, such as adding or removing reconfigurable machines or tools. The second indicator is reconfiguration time, which includes both the time required for changing the production line layout and the time needed to modify the structure or reconfigurable machine tool (RMT) of the machines. Energy consumption is another significant indicator, as it poses a major challenge in the manufacturing sector. Consequently, research on RMS energy consumption has garnered significant attention. Additionally, other indicators like system flexibility, configuration convertibility, and system reliability have been considered. Yelles-Chaouche et al. (Citation2021) conducted a comprehensive survey of research publications focused on optimising RMS (Reliability, Maintainability, and Supportability) and the methods used to address associated problems. The survey presents relevant objective functions and performance indicators used to evaluate the effectiveness of RMS.
2.2 Sustainable manufacturing
Sustainable manufacturing is a vital aspect of achieving sustainable development (SD), as highlighted in the Brundtland Report (Brundtland Citation1987). It aims to improve the quality of life for current and future generations. Manufacturing, with its significant impact on the economy (GDP), environment (material and energy consumption, carbon emissions), and society (job opportunities), plays a crucial role in this pursuit. Hence, sustainable manufacturing is a critical element in the overall objective of sustainable development.
In this paper, the definition of sustainable manufacturing used is from Garetti and Taisch (Citation2012): ‘Sustainable manufacturing can be defined as the ability to smartly use natural resources for manufacturing, by creating products and solutions that, thanks to new technology, regulatory measures, and coherent social behaviors, are able to satisfy economic, environmental, and social objectives, thus preserving the environment while continuing to improve the quality of human life.’ This definition is chosen because it encompasses the three perspectives and impacts of the manufacturing industry.
The importance of sustainable manufacturing has been widely recognised and accepted by governments and organisations worldwide. Here are some examples of sustainable manufacturing projects initiated by governments and organisations:
A more sustainable manufacturing in UK – UK (Machado, Peter Winroth, and Ribeiro da Silva Citation2020).
Minimised material inputs; waste management; energy efficiency; reduced water usage; efficiency in land usage; leadership in low-carbon technology.
New forms of value associated with products including sustainability; products reused, remanufactured, recycled and redesigned with recovery in mind; more durable products designed for shared ownership; spare capacity built into supply chains to ensure resilience.
Products use smaller amounts of materials and energy; material is not land-filled but kept in a ‘productive loop’; cleaner and quieter factories close to consumers, suppliers, and academic institutions; supply chains with spare capacity at all stages.
Made in China 2025 – China.
A management system for safeguarding sustainable manufacturing should be established to provide technical and policy support to meet guidelines.
Technical support for theory and methodology of life-cycle and end-of-life assessment of products.
Implementation of ‘5R’ strategy for promoting a ‘technology and information platform’ for green design of Chinese products.
Efficient and Sustainable Manufacturing – EU.
Increasing throughput, quality, environmental and social sustainability of manufacturing activities while reducing cost.
Reducing emissions, energy, resources, and materials consumption.
Increasing the inclusion of humans in factories.
2.2.1 Links between sustainable manufacturing and Industry 4.0
Existing literature has highlighted a strong correlation between Industry 4.0 and sustainable manufacturing. The literature exploring the connection between Industry 4.0 and sustainable manufacturing can be categorised into five areas.
Area 1: Business Model. Gerlitz (Citation2016) developed a business modelling and value creation method for smart and sustainable manufacturing in small and medium-sized enterprises (SMEs). Stock and Seliger (Citation2016) presented a use case for retrofitting machine tools as an opportunity for new services and sustainable manufacturing. Kiel et al. (Citation2017) assessed the benefits and challenges of the industrial internet of things (IIoT) in achieving sustainable industrial value creation. Their research highlighted the importance of considering economic, social, ecological/environmental, technical integration, data and information, and public context dimensions. Bakkari and Khatory (Citation2017) focused on leveraging Industry 4.0 technology to enhance working conditions.
Area 2: Production. Larreina et al. (Citation2013) studied sustainability indicators for smart manufacturing extrusion systems and production processes. Shrouf, Ordieres, and Miragliotta (Citation2014) explored how IIoT systems support energy efficiency management in smart factories. Lee et al. (Citation2015) developed a sustainable manufacturing testbed system to assess the sustainability performance of production systems. Trentesaux, Borangiu, and Thomas (Citation2016) emphasised the importance of considering machine lifecycle and maintenance in sustainable manufacturing within the context of Industry 4.0. Kumar, Shankar, and Thakur (Citation2018) focused on optimising maintenance strategies using a big data-driven sustainable manufacturing framework for condition-based maintenance optimisation. Ferrera et al. (Citation2017) proposed a total-efficiency framework to enhance the environmental and economic performance of industrial companies. Angioletti et al. (Citation2016) discussed the benefits and challenges of additive manufacturing in improving material and energy efficiency for sustainable manufacturing.
Area 3: Supply Chain. Prause (Citation2015) evaluated the creation of complex manufacturing networks using cyber-physical systems (CPS). Severengiz et al. (Citation2015) proposed a Sustainable Manufacturing Community, a web-based platform that utilises CPS to facilitate networking and knowledge management in production networks, including material flow and component recycling. Prause and Atari (Citation2017) conducted a case study on cross-company production processes in an internationally operating high-tech manufacturing enterprise. They examined the relationship between networking, organisational development, structural conditions, and sustainability in the context of Industry 4.0.
Area 4: Products. Stark et al. (Citation2014) developed a new process for printed circuit board repair and overhaul, aiming to reduce errors, automate processes, and enable non-destructive testing. Gerlitz (Citation2016) focused on design integration for extensive value creation in manufacturing, products, and services. Stock and Seliger (Citation2016) addressed sustainable product design with the aim of achieving closed-loop life cycles. Miranda et al. (Citation2019) proposed a reference framework for the systematic development of sensing, smart, and sustainable products, incorporating an integrated product, process, and manufacturing system development reference model.
Area 5: Policy Development. Morrar, Arman, and Mousa (Citation2017) emphasised the need for policymakers to consider the social dimensions and global impact of new technologies. Bogle (Citation2017) highlighted the growing importance of cybersecurity as a national priority for countries. Frolov et al. (Citation2017) stated that improved collaboration between universities and research organisations positively affects the implementation of Industry 4.0.
2.3 Managing uncertainties in manufacturing systems
Uncertainty is a key aspect of decision-making in complex engineered systems. In the context of Industry 4.0, these uncertainties arise throughout the product value chain (Wang et al. Citation2021). They can be categorised into three areas: problem modelling, decision process uncertainty, and design exploration uncertainty.
2.3.1 Uncertainty in problem modelling
Internal and external uncertainties are inherent in the model-based realisation of complex engineering systems, particularly in simulation-based design (Burggräf, Wagner, and Weißer Citation2020). Internal uncertainties arise from variabilities in the system model, which can be attributed to assumptions, simplifications, limited data, and computational considerations (Frazzon et al. Citation2020). External uncertainties stem from decision preferences and design expectations, including factors like customer satisfaction, evolving quality demands, and disruptions in manufacturing or the supply chain (Pessôa and Jauregui Becker Citation2020).
2.3.2 Uncertainty in decision process
Traditional design models such as IDEF0, BPMN, and EPC are inadequate for capturing uncertain information and its transitivity in a process chain (Wang et al. Citation2021) and for addressing missing data (Souifi et al. Citation2022). In the design of multi-stage manufacturing systems, uncertainty propagates due to unpredictable parameters and structural unpredictability in the model chains. The key challenge lies in developing a hierarchical process model that graphically represents interconnected subsystem parameters and the propagation characteristics of uncertainty in the model and process chain (Wang et al. Citation2021).
2.3.3 Uncertainty in design exploration
The goal of uncertainty management in the design of complex engineered systems is to create a resilient and adaptive system, particularly in the Industry 4.0 era (Hsieh Citation2022). In the context of managing a multi-stage manufacturing system, designers must account for uncertainties in design exploration, such as incomplete models and decision preferences, to ensure adaptability and diagnose potential risks. This requires systematically adjusting the design space to mitigate error accumulation and propagation throughout the process chain, utilising data from sensors and exploring the dynamic propagation characteristics of uncertainty in decision-making. The challenge lies in assessing the impact of uncertainty on the system’s design results and its effects on the decision-making process.
2.4 Sustainable reconfigurable manufacturing
As discussed in Section 1, both sustainable manufacturing and reconfigurable manufacturing are crucial components of Industry 4.0. The integration of sustainable manufacturing, with its potential for cost savings, resource conservation, and improved efficiency, along with reconfigurable manufacturing, which offers enhanced flexibility, adaptability, and customisability, enables companies to gain a competitive advantage in Industry 4.0. Researchers have recognised the importance of considering sustainable manufacturing and reconfigurable manufacturing together. Battaïa, Dolgui, and Guschinsky (Citation2020) emphasised the inclusion of environmental indicators such as energy consumption and waste disposal when implementing RMS. The following sections will review relevant publications in this field, focusing on system performance optimisation and energy consumption.
2.4.1 System performance optimisation
In this class, the authors focused on optimising the performance of sustainable RMS by considering various indicators such as cost, lead time, waste, emissions, and energy consumption. Huang, Badurdeen, and Jawahir (Citation2018) explored the characteristics of RMS and developed a method to assess the changes in sustainable manufacturing performance when these characteristics vary. The indicators they assessed included emissions, waste, water use and efficiency, energy use and efficiency, manufacturing cost, and operational performance. However, their study was limited to considering only the system convertibility as a variable.
Khezri, Benderbal, and Benyoucef (Citation2019) investigated the sustainability of RMS from the perspective of waste liquid and gas emissions. They aimed to optimise the operation progress of RMS by considering objectives such as hazardous liquid waste, greenhouse gas emissions, production cost, and production time. In their subsequent study, Khezri, Benderbal, and Benyoucef (Citation2021) further developed a multi-objective mathematical model for sustainable RMS based on their previous work. Khettabi, Benyoucef, and Boutiche (Citation2022) proposed a multi-objective optimisation approach using dynamic NSGA-II and new NSGA-III to optimise objectives such as hazardous liquid waste, greenhouse gas emissions, production cost, and production time.
2.4.2 Energy consumption
In this class, energy consumption is considered a prime indicator. Battaïa et al. (Citation2020) analysed the state of the art on the topic of sustainable and energy-efficient RMS and suggested five new avenues of research. Choi and Xirouchakis (Citation2015) calculated the energy consumed per part for each configuration. Energy consumption depends on production planning and the corresponding configurations of the system. Idle times, setup, transfer of parts or tools (with conveyors, gantries, or automated guided vehicles), as well as waste and chip management, are considered when calculating. Zhang et al. (Citation2015) introduced reconfigurable and energy-efficient conceptual manufacturing systems and presented a discrete event simulation model to verify their energy efficiency. It allows for local reconfiguration aimed at reducing energy consumption in a given configuration. The following In Table the list of recent publications on the topic of sustainable reconfigurable manufacturing is presented.
Table 1. Resent publications on sustainable reconfigurable manufacturing.
To summarise, humans are now in the era of Industry 4.0, and the concept of Industry 4.0 is evolving over time. Sustainable manufacturing, as a critical element of Industry 4.0, has garnered significant attention from both researchers and policymakers. Similarly, reconfigurable manufacturing remains a popular topic that shows no signs of slowing down since its establishment in the 1990s, primarily due to its ability to quickly adapt to market changes. Researchers have begun to explore the integration of sustainable manufacturing and reconfigurable manufacturing to enhance companies’ competitiveness in Industry 4.0. However, some have overlooked the uncertainty inherent in real-world manufacturing processes. Therefore, to effectively merge the concepts of sustainable manufacturing and reconfigurable manufacturing, a realistic new design method is required. In response to this issue, we have developed a framework for realisation of responsive and sustainable RMS. The details of the framework are presented in the following Section 3.
3. Proposed method
To ensure cost-effective processes and high product quality while manufacturing products in an energy-efficient manner, it is crucial to design systems that are adaptable and sustainable to meet dynamic and ambitious market demands. Therefore, a robustly validated pre-emptive DEF is proposed to support adaptable, operable, sustainable, and reconfigurable manufacturing processes.
In the context of traditional manufacturing systems, several requirements must be fulfilled to achieve responsive and sustainable RMS. These requirements include flexible production, analysis of historical data and past experience, connectivity among system elements, and minimising energy usage. When designing a robustly validated pre-emptive DEF that is adaptable and sustainable in response to dynamic and ambitious market demands, foundational requirements are classified into four groups: adaptability, operability, reconfigurability, and sustainability, as illustrated in Figure .
Figure 1. Design for responsive and sustainable reconfigurable RMS.
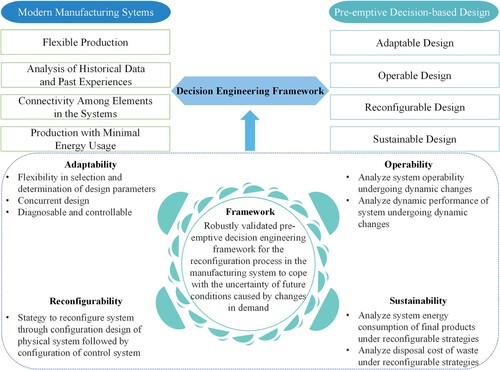
Adaptability. Adaptability is reflected in the following aspects (1) extensive solution space exploration to accommodate flexibility in selecting and determining the values of design parameters, (2) concurrent design of mechanical and sub-systems, (3) diagnosable design for error and controllable design for sub-systems under different types of uncertainty (Milisavljevic-Syed et al. Citation2020b).
Operability. Operability is reflected in the need to analyse the overall system functionality at a dynamic state to determine system functionality and performance (Milisavljevic-Syed et al. Citation2020b).
Reconfigurability. Reconfigurability manifests through the necessity of three key strategies (1) RMT, (2) reconfiguration of inspection tools, (3) RMS (Milisavljevic-Syed et al. Citation2020b).
Sustainability. Sustainability is reflected in the need to analyse the overall system energy consumption, disposal costs of hazardous liquid waste, and the emissions of greenhouse gases (GHG) within a reconfigurable strategy (Milisavljevic-Syed et al. Citation2020b).
3.1 Problem statement
The problem is to design a robustly validated pre-emptive DEF for the reconfiguration process in the manufacturing system. This framework aims to address the uncertainty of future conditions caused by changes in demand due to instability in the free-market system or changes in production technologies due to the industrial decarbonisation strategy.
We propose the design of an n-stage engineering system with different stages and operations, as illustrated in Figure . Each operation in the process is carried out within specified tolerances. The ultimate decision regarding the product's quality, whether it is deemed excellent or unacceptable, lies with the customer. The final product, manufactured with minimal energy consumption under a reconfigurable strategy, will be deemed acceptable.
Figure 2. Problem description – Design and analyse of responsive and sustainable RMS.
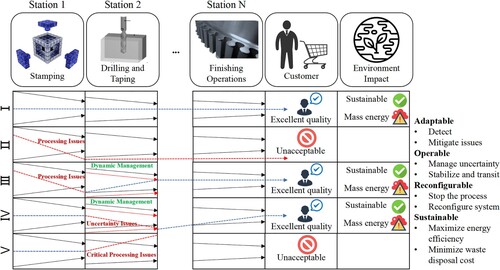
Scenario 1, Row 1 in Figure . In an ideal situation, there are no errors, the model is complete and accurate, and all data are known. We will have an ideal manufacturing process that will result in a product of excellent quality.
Scenario 2, Row 2 in Figure . Process issues may arise at any stage due to imprecise fixturing of parts, such as misalignments, which can lead to dimensional variations and impact product quality. These errors propagate throughout the rest of the process and ultimately affect the final product quality, owing to the characteristics of networked engineering systems.
Scenario 3, Row 3 in Figure . Processing issues at Station 1 may not be easily resolved. However, to make adjustments and enhance the processes in subsequent stations, it is essential to develop a system that is adaptable to these changes. The system is designed to observe and locate errors, detect their causes, and effectively mitigate them. By implementing this approach, the process can rebound after Station 1 and bring it back on track within specified tolerances, ultimately resulting in a product of excellent quality.
Scenario 4, Row 4 in Figure . If the mathematical models used for simulating a process are incomplete or incorrect, or if there is a lack of data, uncertainties may arise due to the inherent randomness or unpredictability of the system, uncertainty in model parameters, uncertainty in model structure, and propagated uncertainties. To address this, we can design a system that is capable of operating effectively, identifying and managing uncertainty, adapting to change, and stabilising the process within specified tolerances. By implementing such a system, we can ensure that the process rebounds and gets back on track, ultimately leading to the production of a product of excellent quality.
Scenario 5, Row 5 in Figure . If critical issues arise that are beyond the point of repair, we can design a system to observe and detect these issues, but not to mitigate them or manage uncertainty. In such cases, the best course of action is to reconfigure the system and refrain from proceeding with the current process.
Based on the aforementioned scenarios, the products of excellent quality will undergo a final analysis based on energy consumption and waste disposal cost. Consequently, the products that exhibit excellent quality while minimising energy consumption and waste disposal cost will be accepted.
3.2 Proposed method overview
A model is proposed to address the stated problem, as depicted in Figure . The model comprises three steps. In step one, the manufacturing company receives dynamically changing requirements from customers. The second step involves the process of designing the manufacturing system. In this step, customised or personalised products are designed based on the requirements gathered in the previous step. To ensure both customisability and cost-effectiveness of the designed manufacturing system, the model utilises the RMS design method introduced by Milisavljevic-Syed et al. (Citation2020b). This method facilitates the generation of a decision space for the RMS reconfiguration strategy through robust design analysis. In the final step, the energy consumption of the decision space for the RMS reconfiguration strategy is calculated. This ensures that the designed manufacturing system not only meets customer requirements and system cost-efficiency but also minimises energy consumption.
Figure 3. Proposed method – Implication for DEF development.
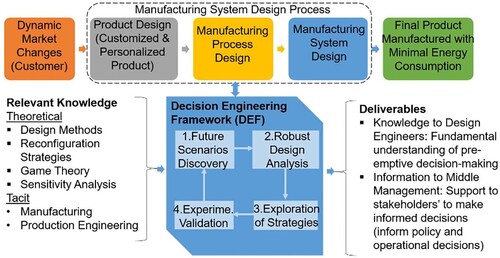
The workflow of the proposed method is depicted in Figure , highlighting four steps: Scenarios Discovery, Decision Model, Decision Interaction, and Decision Network. Step 1 involves analysing the required level of reconfiguration, resulting in the identification of future condition scenarios. This step also includes data mining to collect historical data and past experiences, as outlined in Section 3.1. Step 2 focuses on modelling the decision-making process for RMT configuration design and sustainability configuration design, as explained in Section 3.3. Step 3 revolves around defining the interaction between RMT and sustainable design, encompassing the modelling of interaction in non-cooperation design case, cooperation design case, and leader-follower design case, as detailed in Section 3.4. Finally, Step 4 encompasses forming the decision network to explore the sustainable RMS reconfiguration strategy, integrating decision models and interaction, as presented in Section 3.5.
3.3 The compromise decision support problem (cDSP) -Based model for decision-making in sustainable RMS configuration design
The cDSP is a mathematical construct utilised to facilitate multi-objective decision-making, as described by Hernandez, Allen, and Mistree (Citation2001). It is commonly employed to handle uncertainties in the design of complex engineering systems. In this paper, the cDSP is employed to design a robust RMS reconfiguration strategy. The following section provides a comprehensive overview of the cDSP-based model for decision-making in sustainable RMS configuration design, offering detailed information on its application and implementation.
3.3.1 Modelling sustainable RMS configuration
A processing route is a crucial element in the design of any sustainable RMS configuration, and it should be considered during the design phase. In order to provide a comprehensive understanding of the sustainable RMS design process, a precise description based on a set of mathematical equations is presented in Equation (1),
(1)
(1) where Cp represents the processing route and cpi represents the ith operation. N is the number of operations in processing route Cp. Based on the model of the RMS configuration and the processing route, constraints and goals are modelled as follows.
3.3.2 Designing the sustainable RMS model
The construction of the constraint model is essential for designing sustainable RMS configurations that satisfy the requirements from the operation set and environmental protection. The operation set encompasses the quantity of required operations and their specific characteristics. In this paper, the environmental requirement primarily focuses on energy consumption. To address the operation set and environmental requirement, the sustainable RMS needs to possess suitable capabilities and functionality. This entails establishing mathematical expressions for capability and functionality, which are then combined with the processing parameters of the operational and environmental requirements to form the design model.
Construction of the Capability Model
The construction of capability model is shown in Equation (2) (Wang et al. Citation2018),
(2)
(2) where olr represents the motion requirement for the rth direction of the lth operation and olr is defined as the degrees of freedom of motion on the X-, Y-, Z-, A-, B-, and C-axes (see Figure ). If the motion in this direction is needed for the operation, olr is 0; otherwise, it is equal to 1. tlr represents the motion in the rth direction of the lth tool-related configuration. h1r indicates the motion in the rth direction of the workpiece-related configuration. ⊕ represents binary addition; that is, if tlr = 0 and h1r = 0, tlr⊕h1r = 0, else tlr⊕h1r = 1.
Construction of the functionality model
Figure 5. The six axes of degree of freedom.
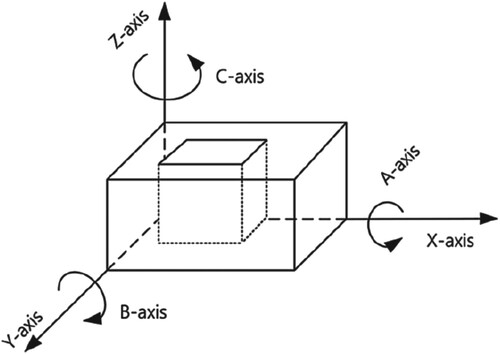
The construction of functionality models is shown in Equations (3)–(5),
(3)
(3)
(4)
(4)
(5)
(5) where TEC is total energy consumption. IEC is the energy consumption of RIS configuration, and REC is the energy consumption of RMS configuration. M is the RIMs in RIS, O is the RMT in RMS, and N is the number of RMT operations. ECx is the energy consumption of each sensor in RIMs. ECt and ECh are the energy consumption of tool-related configuration and workpiece-related configuration. xi represents the number of sensors in ith RIM.
Modelling the sustainable RMS configuration design goal
The decision model for sustainable RMS configuration design can be developed and solved by analysing sustainability aspects. Sustainability plays a critical role in ensuring minimal energy consumption during RMS configuration changes. This includes considering both the initial energy consumption and the energy consumption associated with the changeover process. The quantitative models for assessing sustainability in RMS configuration are presented in Equations (6),
(6)
(6) where SCR is the sustainability of the RMS configuration design. fef represents emission factor for electricity consumption.
3.4 Game theory-based model for interactions in RMS configuration design and sustainability design
Game theory is a mathematical framework utilised for evaluating decision-making in interactive scenarios where the actions of one player are influenced by the decisions of other players (Osborne Citation2004). In game theory, a game is defined by a group of players, a range of possible strategies for each player, and a reward function that assigns payouts to each player for every possible combination of strategies.
In this paper, we employ game theory to describe and model the interactions between RMS and RMT. Based on the information exchange between RMT configuration design and RMT configuration design process, the exploration process is divided into the following three situations: weak-weak interaction, strong-strong interaction, and strong-weak interaction, as illustrated in Figure .
Figure 6. Solution space exploration in weak-weak interaction (a), strong-strong interaction (b), and strong-weak interaction (c).
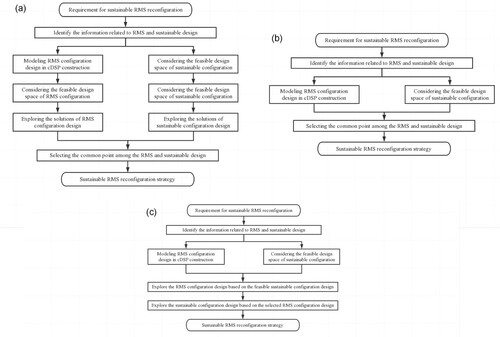
3.4.1 Solution space exploration in weak-weak interaction
In the design and construction process described in this scenario, an enterprise has independently separated the production system and sustainability design into two distinct projects, each awarded to different design companies. These companies have designed their respective missions without knowledge of the other's design. Consequently, there is no information exchange between the RMT configuration design and the sustainability design. Each design is based on assumptions about what the other party will design, and they are developed independently to optimise reconfigurability and sustainability.
Step 1: Identify the design information, including variables, constraints, and targets for both RMT and sustainable configuration design.
Step 2: Establish the cDSP-based model for RMT and sustainable configuration design based on the identified design information.
Step 3: Utilise each other's feasible design space as a parameter in the decision model. Define specific parameter values to solve the decision model and obtain the corresponding solution space.
Step 4: Select a point that lies within the intersection of the three solution spaces obtained in Step 3. This point represents a satisfactory solution for the sustainable RMS systemic reconfiguration strategy.
3.4.2 Solution space exploration in strong-strong interaction
In this situation, during the design and construction of RMS, enterprises come together to form an alliance and collaborate on completing the production system and sustainability design projects. Within this enterprise alliance, the member enterprises cooperate with each other based on the alliance agreement to achieve a consensus on the allocation of design and configuration resources, with the aim of maximising reconfigurability and sustainability.
Step 1: Identify the design information, including variables, constraints, and targets, for both RMT and sustainable configuration design.
Step 2: Establish the cDSP-based model for RMT and sustainable configuration design based on the identified design information.
Step 3: Combine the cDSP-based models of RMS and sustainable configuration design into a unified cDSP-based model for sustainable RMS reconfiguration design. Explore the solutions generated by this model as the reconfiguration strategy for achieving sustainability in the RMS.
3.4.3 Solution space exploration in strong-weak interaction
In this situation, the enterprise takes the lead in designing the RMS process and carries out the design and construction tasks of the RMS production process internally. However, it outsources the testing system project and requires the design of the testing system to align with the production process design proposed by the enterprise. In other words, the RMT configuration design serves as the foundation for the sustainability design.
Step 1: Identify the design information, including variables, constraints, and targets, for both RMT and sustainable configuration design.
Step 2: Establish the cDSP-based model for RMT and sustainable configuration design based on the identified design information.
Step 3: Use the feasible domain of sustainable design as a hypothesis parameter in the decision model for RMT configuration design. Select specific parameter values to solve the decision model and obtain the corresponding solution space for RMT configuration design.
Step 4: Utilise the solution space obtained in Step 3 for RMT configuration design and input it into the decision model for sustainable configuration design. This will yield the corresponding solution space for the sustainable configuration design.
3.5 Decision network for exploring the sustainable RMS reconfiguration strategy
The decision network for exploring the sustainable RMS reconfiguration strategy is illustrated in Figure . This decision network is constructed using cDSPs and interaction models (game theory models) to facilitate the exploration of sustainable RMS reconfiguration methods and serve as the foundation for dynamic management of such reconfigurations.
Figure 7. Decision network for exploring the sustainable RMS reconfiguration strategy.
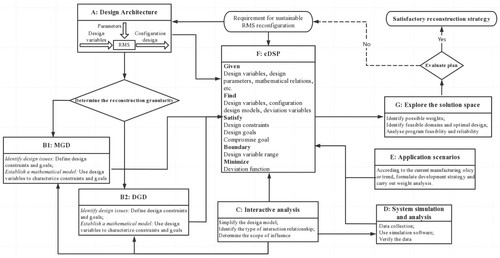
The input to the decision network consists of the requirements for sustainable RMS reconfiguration, which encompass design parameters, design variables, and other relevant factors. The output of the decision network is a satisfactory sustainable RMS reconfiguration strategy, enabling decision makers to make informed decisions and improve the efficiency and sustainability of the manufacturing process.
Step A: Preprocessing of information to effectively collect and analyse the diverse and complex input information. A common design architecture is utilised to gather and analyse the relevant information of different granularity configurations, dividing the design information into design variables, parameters, and goals.
Step B and F: Analysis and exploration of the single granularity configuration design as the initial solution. The relationship between design parameters and variables is defined based on the key features of different RMS granularity configurations and their corresponding performance indicators. Simultaneously, a single-grain configuration design scheme is explored. If design goals change, re-exploration of the solution space is required. The initial solution obtained from the single-granularity configuration design can be used to explore systematic reconfiguration methods.
Step C and F: Consideration of interactions between different granularity configuration designs and the established exploration of RMS reconfiguration methods. The cDSP model is employed to generate the most satisfactory solution scheme.
Step D: Utilisation of weights derived from system simulation or analysis of the application scenario. These weights help assess the sensitivity of the design plan to dynamic demand changes and enhance the designer's understanding of RMS reconfiguration behaviour. Finally, the designers evaluate the satisfactory design schemes to promote the dynamic management of RMS reconfiguration.
4. Case study
In this section, we validate the empirical performance of the proposed framework through an engine cylinder block case study and showcase the application of sustainable RMS configuration design. We demonstrate the most satisfactory sustainable RMS configuration design by generating a feasible RMT design. The RMT design of the RMS is constructed using a configuration tree. To illustrate the example, we present a simplified module library (Wang et al. Citation2018) in Table , along with its corresponding energy consumption (Sullivan, Burnham, and Wang Citation2010), based on the functions of modules, motion, and support.
Table 2. RMT basic modules and the corresponding energy consumption (Sullivan, Burnham, and Wang Citation2010).
The demand for automotive product customisation is rapidly increasing, leading to a wide variety of automobiles in the market. As automotive technology evolves, the core component of automotive power, the engine, is also undergoing changes. Currently, there are three common engine structures: in-line four-cylinder engine (L4), V-type six-cylinder engine (V6), and V-type eight-cylinder engine (V8). Different automotive manufacturers choose engine types based on factors such as displacement and noise reduction. Figure (a) illustrates the cylinder blocks of the L4 and V8 engines.
Figure 8. The example of the L4 (right) and V8 (left) engine block (a) and three types of the cylinder block (b) (Abbas and ElMaraghy Citation2016).
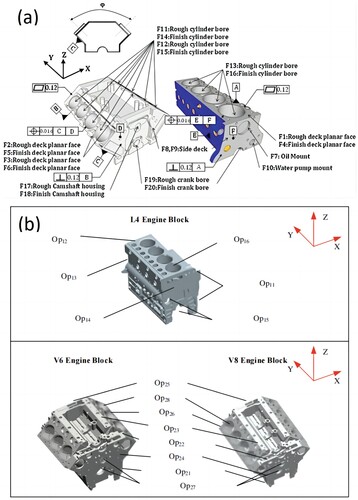
In response to dynamic market demands, enterprises strive to improve their manufacturing systems while maintaining low energy consumption and high quality. They aim to produce a wider range of parts to meet the requirements of various automotive assemblies and enhance their competitiveness. In the automotive industry, RMS hold immense development and application potential, making them an essential driving force for emerging enterprises in the future. Therefore, we demonstrate the proposed method for exploring the RMS reconfiguration strategy by manufacturing the engine cylinder block, showcasing its relevance and effectiveness in the industry.
The engine cylinder block is a complex and rigid component, with different engine types having unique structures. Machining the cylinder block involves multiple surfaces and holes, each requiring specific machining accuracy. Therefore, the proper arrangement and division of the process route are crucial for parts processing. In this example, we explore the sustainable RMS reconfiguration strategy in two scenarios: transitioning between different part families and transitioning between different parts within a part family.
Based on the cylinder block structure, we identify two part families for machining: the L-type engine block family and the V-type engine block family. The L-type engine block family consists of a single component, the L4 engine block, while the V-type engine block family consists of two components, the V6 engine block and the V8 engine block. Therefore, the first design requirement is to reconfigure the RMS to accommodate the transition from the L4 engine block to the V6 engine block. The second task involves transitioning from the V6 engine block to the V8 engine block.
Before delving into the detailed design of the sustainable reconfiguration configuration, we need to analyse the processing routes for the three engine blocks mentioned above. Considering the large number of machining features, we focus on the key operations in this example. The L4, V6, and V8 engine blocks used in this case were sourced from a company, as depicted in Figure (b), and their key operating information is presented in Table .
Table 3. Key operation for L4, V6 and V8 engine cylinder production (Milisavljevic-Syed et al. Citation2020a).
During the reconfiguration process, the process route for the L4 engine block is Op11 – Op12 – Op13 – Op14 – Op15 – Op16. The process route for the V6 engine block is Op21 – Op22 – Op23 – Op24 – Op25 – Op26 – Op27 – Op28. To minimise fixture errors caused by fixture changes, the process path change for the V8 engine block is Op21 – Op25 – Op22 – Op23 – Op24 – Op27 – Op26 – Op28.
The initial configuration of the RMS is designed to fulfil the manufacturing requirements of the L4 engine block. The system or subsystem configurations are depicted using a block diagram, while the configuration of each device is presented through a configuration tree. The initial RMS configuration for this text example is illustrated in Figure . It comprises a total of 9 workstations, with six being RMTs and three being RIMs. The configuration tree for each RMT is shown alongside the corresponding workstation.
Figure 9. The RMS initial configuration in text example.
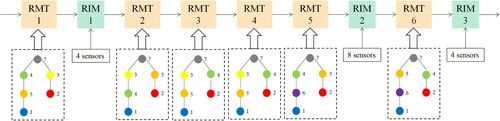
The first production partition includes RMT 1, and RIM 1 is responsible for monitoring the state of RMT 1. RIM 1 consists of four sensors to facilitate the inspection process. The second production partition encompasses four machine tools: RMT 2, RMT 3, RMT 4, and RMT 5. RIM 2 is employed to monitor the states of these four machine tools and is equipped with eight sensors for this purpose. The final production partition involves RMT 6, and RIM 3 is responsible for monitoring the state of RMT 6. RIM 3 is equipped with four sensors to carry out the necessary inspections.
Furthermore, to incorporate the company's priorities and preferences, an investigation is conducted to understand the company's manufacturing needs. Relevant parameter data are extracted, which include module fixing and assembly costs (Wang et al. Citation2018), sensor reliability, sensor cost, detector installation cost (Shang et al. Citation2021), and energy consumption (refer to Table ), among others. To assign weights to the different factors, the tool-related and workpiece-related energy consumption are given weights of 0.7 and 0.3, respectively. This decision is based on the understanding that spindle energy primarily originates from the motor across machine tools (Zhao et al. Citation2017).
The reconfiguration process in this case consists of two stages: transitioning from the L4 engine cylinder block to the V6 engine block, and then from the V6 engine block to the V8 engine block. To simplify the description, these two phases are referred to as Phase A and Phase B, respectively, considering and not considering energy consumption.
In Phase A, the requirement is to reconfigure the system due to the transition between different part families. Designers are tasked with re-designing and reconfiguring the production and detection processes. To address the challenge of multi-granularity interactions at this stage, the cooperative model presented in Figure is utilised to explore potential solutions. Figure (a) and Figure (b) illustrate the satisfactory solutions for Phase A, with and without considering energy consumption, respectively.
Figure 10. The solution of Phase A with (a) and without (b) considering energy consumption.
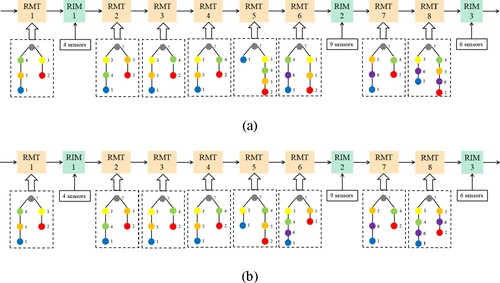
Phase B involves the conversion of different parts within the same part family, resulting in the reconfiguration of the system. To address this stage, the leader-follower model is employed, where the leader represents the RMT configuration design. Figure (a) and Figure (b) depict the satisfactory solutions for Phase B, considering and not considering energy consumption, respectively.
Figure 11. The solution of Phase B with (a) without (b) considering energy consumption.
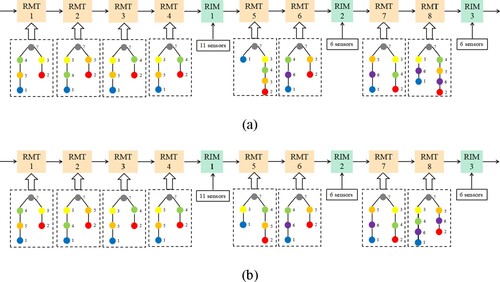
In this case study, we assume that an engine block weighs approximately 300 kg, while an inspection sensor weighs around 1 kg. Additionally, we assign an emission factor (fef) of 0.543 T CO2/MWH for electricity consumption based on previous research (Koffi et al. Citation2017b). Figure illustrates the total energy consumption and sustainability of the RMS reconfiguration design for each phase. The results indicate that Phase A(b) and Phase B(b) exhibit lower energy consumption compared to Phase A(a) and Phase B(a), respectively. Furthermore, the RMS reconfiguration designs that take sustainability into account, namely Phase A(b) and Phase B(b), outperform the designs that do not consider sustainability, namely Phase A(a) and Phase B(a).
5. Result and discussion
The proposed method, introduced in Section 3, is validated using the test case described in Section 4, aiming to identify the most satisfactory sustainable RMT configuration for dynamic requirements. As the number of workstations increases, the configuration design of an RMS becomes more complex. However, the cDSP-based construction and game theory approach can effectively address the space exploration process in configuration design. The results obtained from the proposed method demonstrate efficient utilisation of original resources. For example, the processing characteristics of the L4 engine's cylinder block align with the first four steps of the V6 engine's cylinder process line, allowing for a consistent configuration design and avoiding unnecessary reconfiguration. Additionally, different interaction relationships can be utilised to describe priorities and preferences among designers. For instance, the reconfiguration in Phase A involves different part families, necessitating the reconfiguration of both the production process and the detection process. This calls for the application of a cooperative model, which maximises the balance between reconfigurability and sustainability. On the other hand, Phase B involves different parts with many similar processes, making the reconfiguration process more influenced by sustainable production. As a result, the leader-follower model is employed.
Furthermore, the sustainability results of each phase, with and without considering energy consumption, are compared. In Phase A(a) and Phase B(a), the focus is on reconfiguring an RMT to meet functionality and diagnosability requirements. To achieve sustainable RMS configuration design, feasible configuration designs, namely Phase A(b) and Phase B(b), are generated. In Phase A(b) and Phase B(b), the objective is to minimise energy consumption during reconfiguration, based on satisfactory RMS configuration designs. The resulting design solutions enhance the sustainability of the specific production process throughout the part family processing.
Compared to existing methods, the proposed method not only supports sustainable RMT in achieving repeated reconfigurations but also enables the sustainable RMT to adapt to changing market needs. In this research, the selection of the most satisfactory design primarily focuses on sustainability, specifically reducing energy consumption based on tool-related and workpiece-related configuration tree considerations.
6. Conclusion and future work
Industry 4.0 and the associated enabling technologies present both opportunities and challenges for companies of all sizes. In this context, sustainable manufacturing and reconfigurable manufacturing play crucial roles. By incorporating the capabilities of cost savings, resource conservation, and efficiency improvement from sustainable manufacturing, along with the flexibility, adaptability, and customisability enhancement of reconfigurable manufacturing, companies can gain a competitive edge in the Industry 4.0 era.
The contributions of this research are as follows: Firstly, we discuss the demand and necessity of developing a sustainable reconfigurable manufacturing system. Secondly, we present a method for designing a sustainable RMS reconfiguration strategy under uncertain conditions, taking into account not only the characteristics of RMS but also sustainable development features. Finally, we provide a case study to validate the proposed method, demonstrating how it supports sustainable RMT by enabling repeated reconfigurations and meeting evolving market needs. However, it is important to acknowledge the limitations of this research. Firstly, the energy consumption data used for calculations are synthetic and not based on real-world measurements. Secondly, this study only considers one sustainable development indicator, namely energy consumption, while other indicators such as waste and carbon emissions are not included. In future research, we intend to gather real-world data to validate the proposed method. Additionally, we plan to incorporate waste disposal costs into the analysis since different sustainable RMS configurations result in varying amounts of waste, including hazardous liquid waste and greenhouse gas emissions, which can impact disposal costs. Achieving a satisfactory sustainable RMS configuration design will require meeting operational requirements while striking a balance between waste disposal costs and sustainability considerations.
Disclosure statement
No potential conflict of interest was reported by the author(s).
Data availability statement
The data that support the findings of this study are available from the corresponding author, Milisavljevic-Syed, J., upon reasonable request.
Additional information
Notes on contributors
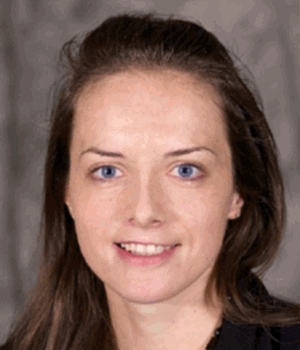
Jelena Milisavljevic-Syed
Dr. Milisavljevic Syed’s design experience spans mechanical, control, and industrial engineering. Her research focus is on integrating design thinking, strategy and innovation management in the realisation of cyber-physical product-service systems adaptable to dynamic market changes as a support further digitalisation (smart manufacturing). Prior to joining Cranfield University, she was a Lecturer in Industrial Design and Director of the Systems Realisation Laboratory at the University of Liverpool in the UK. Dr. Milisavljevic Syed earned a PhD in Mechanical Engineering with a focus on Dynamic Management of Networked Engineered (Manufacturing) Systems from the University of Oklahoma in the USA, a second MS in Mechanical Engineering with a focus on Uncertainty Management in Realisation of Complex Manufacturing Systems from the University of Oklahoma in the USA, a first MS in Mechanical Engineering with a focus on Elastohydrodinamics Lubrication in Machines from the University of Nis in Serbia, and a diploma of Mechanical Technician for Computer-Aided Design (CAD) from the College of Mechanical Engineering. Jelena has co-authored one monograph, seventeen journal publications, two magazine articles and twenty-seven technical publications dealing with dynamic management in the realisation of manufacturing systems, design, and analysis of complex systems, and product development. She is a member of ASME, ASEE, BFWG, MIET, and Design Society.
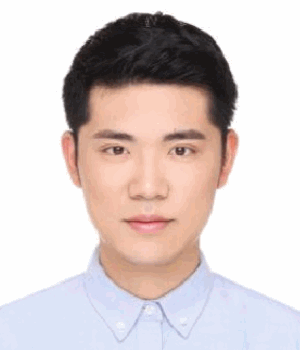
Jiahong Li
Jiahong Li obtained his MSc in Mechanical Engineering with a major in Advanced Manufacturing Systems and Technology from the University of Liverpool, UK. After that, he worked as a senior manager of business and supply chain in a Fortune 500 company. He then joined the Systems Realisation Laboratory at the University of Liverpool as a PhD research student. He has now moved to Cranfield University to continue his research at the School of Aerospace, Transport and Manufacturing. His research focuses on investigating the return on investment of predictive maintenance implementation.
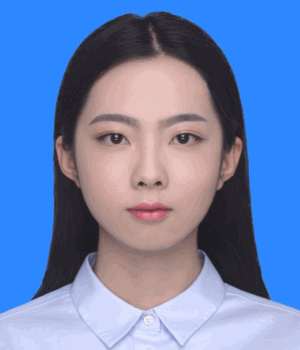
Hanbing Xia
Hanbing Xia earned her MSc in 2019 and BSc in 2017 in Logistics Management from the Jilin University, China respectively. Hanbing Xia is a PhD candidate specialising in Optimisation of Automotive Reverse Supply Chain based on AI Techniques. She moved to University of Liverpool as a PhD research student, where she undertook her research within the Systems Realisation Laboratory. She has now moved to Cranfield University to continue her research at the School of Aerospace, Transport and Manufacturing
References
- Foresight, U. K. 2013. The Future of Manufacturing: A New Era of Opportunity and Challenge for the UK. Summary Report, The Government Office for Science, London 20.
- Abbas, Mohamed, and Hoda ElMaraghy. 2016. “Design Synthesis of Machining Systems Using co-Platforming.” Journal of Manufacturing Systems 41: 299–313. https://doi.org/10.1016/j.jmsy.2016.10.001.
- Angioletti, C. E. C. I. L. I. A., Francesco Giovanni Sisca, F. G. Fg, Rossella Luglietti, Marco Taisch, and Roberto Rocca. 2016. “Additive Manufacturing as an Opportunity for Supporting Sustainability Through the Implementation of Circular Economies.” In Proceedings of the Summer School Francesco Turco, 25-. AIDI-Italian Association of Industrial Operations Professors.
- Bakkari, Mohammed, and Abdellah Khatory. 2017. “Industry 4.0: Strategy for More Sustainable Industrial Development in SMEs.” Paper Presented at the Proceedings of the IEOM 7th International Conference on Industrial Engineering and Operations Management, Rabat, Morocco.
- Battaïa, Olga, Lyes Benyoucef, Xavier Delorme, Alexandre Dolgui, and Simon Thevenin. 2020. Reconfigurable Manufacturing Systems: From Design to Implementation, 179–191
- Battaïa, Olga, Alexandre Dolgui, and Nikolai Guschinsky. 2017. “Decision Support for Design of Reconfigurable Rotary Machining Systems for Family Part Production.” International Journal of Production Research 55 (5): 1368–1385. https://doi.org/10.1080/00207543.2016.1213451.
- Battaïa, Olga, Alexandre Dolgui, and Nikolai Guschinsky. 2020. “Optimal Cost Design of Flow Lines with Reconfigurable Machines for Batch Production.” International Journal of Production Research 58 (10): 2937–2952. https://doi.org/10.1080/00207543.2020.1716092.
- Bogle, Ian David Lockhart. 2017. “A Perspective on Smart Process Manufacturing Research Challenges for Process Systems Engineers.” Engineering 3 (2): 161–165. https://doi.org/10.1016/J.ENG.2017.02.003.
- Brundtland, Gro Harlem. 1987. "Our Common Future World Commission On Environment And Developement."
- Burggräf, Peter, Johannes Wagner, and Tim Weißer. 2020. “Knowledge-based Problem Solving in Physical Product Development––A Methodological Review.” Expert Systems with Applications: X 5: 100025. https://doi.org/10.1016/j.eswax.2020.100025.
- Cerqueus, Audrey, Paolo Gianessi, Damien Lamy, and Xavier Delorme. 2020. “Balancing and Configuration Planning of RMS to Minimize Energy Cost.” Paper Presented at the Advances in Production Management Systems. Towards Smart and Digital Manufacturing: IFIP WG 5.7 International Conference, APMS 2020, Novi Sad, Serbia, August 30–September 3, 2020, Proceedings, Part II.
- Choi, Yong-Chan, and Paul Xirouchakis. 2015. “A Holistic Production Planning Approach in a Reconfigurable Manufacturing System with Energy Consumption and Environmental Effects.” International Journal of Computer Integrated Manufacturing 28 (4): 379–394. https://doi.org/10.1080/0951192X.2014.902106.
- Ferrera, Enrico, Rosaria Rossini, A. J. Baptista, Steve Evans, Gunnar Große Hovest, Maria Holgado, Emil Lezak, E. J. Lourenço, Zofia Masluszczak, and Alexander Schneider. 2017. “Toward Industry 4.0: Efficient and Sustainable Manufacturing Leveraging MAESTRI Total Efficiency Framework.” Paper Presented at the Sustainable Design and Manufacturing 2017: Selected Papers on Sustainable Design and Manufacturing 4.
- Frazzon, Enzo Morosini, Ícaro Romolo Sousa Agostino, Eike Broda, and Michael Freitag. 2020. “Manufacturing Networks in the era of Digital Production and Operations: A Socio-Cyber-Physical Perspective.” Annual Reviews in Control 49: 288–294. https://doi.org/10.1016/j.arcontrol.2020.04.008.
- Frolov, Vladislav Genrihovich, Dmitry Igorevich Kaminchenko, Dmitry Yuryevich Kovylkin, Julia Alexandrovna Popova, and Angelina Alexandrovna Pavlova. 2017. “The Main Economic Factors of Sustainable Manufacturing Within the Industrial Policy Concept of Industry 4.0.” Academy of Strategic Management Journal 16 (2): 1–11.
- Garetti, Marco, and Marco Taisch. 2012. “Sustainable Manufacturing: Trends and Research Challenges.” Production Planning & Control 23 (2-3): 83–104. https://doi.org/10.1080/09537287.2011.591619.
- Gerlitz, Laima. 2016. “Design Management as a Domain of Smart and Sustainable Enterprise: Business Modelling for Innovation and Smart Growth in Industry 4.0.” Entrepreneurship and Sustainability Issues 3 (3): 244. https://doi.org/10.9770/jesi.2016.3.3(3).
- Hernandez, Gabriel, Janet K Allen, and Farrokh Mistree. 2001. “The Compromise Decision Support Problem: Modeling the Deviation Function as in Physical Programming.” Engineering Optimization 33 (4): 445–471. https://doi.org/10.1080/03052150108940928.
- Hsieh, Fu-Shiung. 2022. “An Efficient Method to Assess Resilience and Robustness Properties of a Class of Cyber-Physical Production Systems.” Symmetry 14 (11): 2327. https://doi.org/10.3390/sym14112327.
- Huang, Aihua, Fazleena Badurdeen, and Ibrahim S Jawahir. 2018. “Towards Developing Sustainable Reconfigurable Manufacturing Systems.” Procedia Manufacturing 17: 1136–1143. https://doi.org/10.1016/j.promfg.2018.10.024.
- Jiao, Roger, Sesh Commuri, Jitesh Panchal, Jelena Milisavljevic-Syed, Janet K Allen, Farrokh Mistree, and Dirk Schaefer. 2021. “Design Engineering in the age of Industry 4.0.” Journal of Mechanical Design 143 (7): 070801 (25 pages). https://doi.org/10.1115/1.4051041.
- Khettabi, Imen, Lyes Benyoucef, and Mohamed Amine Boutiche. 2021. “Sustainable Reconfigurable Manufacturing System Design Using Adapted Multi-Objective Evolutionary-Based Approaches.” The International Journal of Advanced Manufacturing Technology 115 (11-12): 3741–3759. https://doi.org/10.1007/s00170-021-07337-3.
- Khettabi, Imen, Lyes Benyoucef, and Mohamed Amine Boutiche. 2022. “Sustainable Multi-Objective Process Planning in Reconfigurable Manufacturing Environment: Adapted new Dynamic NSGA-II vs New NSGA-III.” International Journal of Production Research 60 (20): 6329–6349. https://doi.org/10.1080/00207543.2022.2044537.
- Khezri, Amirhossein, Hichem Haddou Benderbal, and Lyes Benyoucef. 2019. “A Sustainable Reconfigurable Manufacturing System Designing with Focus on Environmental Hazardous Wastes.” Paper Presented at the 2019 24th IEEE International Conference on Emerging Technologies and Factory Automation (ETFA).
- Khezri, Amirhossein, Hichem Haddou Benderbal, and Lyes Benyoucef. 2021. “Towards a Sustainable Reconfigurable Manufacturing System (SRMS): Multi-Objective Based Approaches for Process Plan Generation Problem.” International Journal of Production Research 59 (15): 4533–4558. https://doi.org/10.1080/00207543.2020.1766719.
- Kiel, Daniel, Julian M Müller, Christian Arnold, and Kai-Ingo Voigt. 2017. “Sustainable Industrial Value Creation: Benefits and Challenges of Industry 4.0.” International Journal of Innovation Management 21 (08): 1740015. https://doi.org/10.1142/S1363919617400151.
- Koffi, Brigitte, Alessandro K Cerutti, Marlene Duerr, Andreea Iancu, Albana Kona, and Greet Janssens-Maenhout. 2017. “Covenant of Mayors for Climate and Energy: Default Emission Factors for Local Emission Inventories.” Joint Research Centre (JRC).
- Koren, Yoram, Uwe Heisel, Francesco Jovane, Toshimichi Moriwaki, Gumter Pritschow, Galip Ulsoy, and Hendrik Van Brussel. 1999. “Reconfigurable Manufacturing Systems.” CIRP Annals 48 (2): 527–540. https://doi.org/10.1016/S0007-8506(07)63232-6.
- Kumar, Ajay, Ravi Shankar, and Lakshman S Thakur. 2018. “A big Data Driven Sustainable Manufacturing Framework for Condition-Based Maintenance Prediction.” Journal of Computational Science 27: 428–439. https://doi.org/10.1016/j.jocs.2017.06.006.
- Larreina, J., A. Gontarz, C. Giannoulis, V. K. Nguyen, P. Stavropoulos, and B. Sinceri. 2013. “Smart Manufacturing Execution System (SMES): The Possibilities of Evaluating the Sustainability of a Production Process.” Paper presented at the Proceedings of the 11th Global Conference on Sustainable Manufacturing, GCSM 2013: Innovative Solutions.
- Lee, Ju Yeon, Seung-Jun Shin, Y Tina Lee, Donald Libes. 2015. “Toward Development of a Testbed for Sustainable Manufacturing.” Concurrent Engineering 23 (1): 64–73. https://doi.org/10.1177/1063293X14559527.
- Leng, Jiewu, Weinan Sha, Baicun Wang, Pai Zheng, Cunbo Zhuang, Qiang Liu, Thorsten Wuest, Dimitris Mourtzis, and Lihui Wang. 2022. “Industry 5.0: Prospect and Retrospect.” Journal of Manufacturing Systems 65: 279–295. https://doi.org/10.1016/j.jmsy.2022.09.017.
- Liu, Ming, Rongfan Liu, Zhanguo Zhu, Chengbin Chu, and Xiaoyi Man. 2018. “A bi-Objective Green Closed Loop Supply Chain Design Problem with Uncertain Demand.” Sustainability 10 (4): 967. https://doi.org/10.3390/su10040967.
- Machado, Carla Gonçalves, Mats Peter Winroth, and Elias Hans Dener Ribeiro da Silva. 2020. “Sustainable Manufacturing in Industry 4.0: An Emerging Research Agenda.” International Journal of Production Research 58 (5): 1462–1484. https://doi.org/10.1080/00207543.2019.1652777.
- Massimi, Elisa, Amirhossein Khezri, Hichem Haddou Benderbal, and Lyes Benyoucef. 2020. “A Heuristic-Based non-Linear Mixed Integer Approach for Optimizing Modularity and Integrability in a Sustainable Reconfigurable Manufacturing Environment.” The International Journal of Advanced Manufacturing Technology 108: 1997–2020. https://doi.org/10.1007/s00170-020-05366-y.
- Milisavljevic-Syed, Jelena, Janet K Allen, Sesh Commuri, and Farrokh Mistree. 2020a. Architecting Networked Engineered Systems. Springer.
- Milisavljevic-Syed, Jelena, Janet K Allen, Sesh Commuri, Farrokh Mistree, Jelena Milisavljevic-Syed, Janet K Allen, Sesh Commuri, and Farrokh Mistree. 2020b. “Architecting Networked Engineered Systems.” Architecting Networked Engineered Systems: Manufacturing Systems Design for Industry 4.0, 185–257. https://doi.org/10.1007/978-3-030-38610-8_6.
- Miranda, Jhonattan, Roberto Pérez-Rodríguez, Vicente Borja, Paul K Wright, and Arturo Molina. 2019. “Sensing, Smart and Sustainable Product Development (S3Product) Reference Framework.” International Journal of Production Research 57 (14): 4391–4412. https://doi.org/10.1080/00207543.2017.1401237.
- Morrar, Rabeh, Husam Arman, and Saeed Mousa. 2017. “The Fourth Industrial Revolution (Industry 4.0): A Social Innovation Perspective.” Technology Innovation Management Review 7 (11): 12–20. https://doi.org/10.22215/timreview/1117.
- Napoleone, Alessia, Alessandro Pozzetti, and Marco Macchi. 2018. “A Framework to Manage Reconfigurability in Manufacturing.” International Journal of Production Research 56 (11): 3815–3837. https://doi.org/10.1080/00207543.2018.1437286.
- Osborne, Martin J. 2004. An Introduction to Game Theory. 3 vols. New York: Oxford university press.
- Pereira, Ana C, and Fernando Romero. 2017. “A Review of the Meanings and the Implications of the Industry 4.0 Concept.” Procedia Manufacturing 13: 1206–1214. https://doi.org/10.1016/j.promfg.2017.09.032.
- Pessôa, MV Pereira, and J. M. Jauregui Becker. 2020. “Smart Design Engineering: A Literature Review of the Impact of the 4th Industrial Revolution on Product Design and Development.” Research in Engineering Design 31 (2): 175–195. https://doi.org/10.1007/s00163-020-00330-z.
- Prause, Gunnar. 2015. “Sustainable Business Models and Structures for Industry 4.0.” Journal of Security & Sustainability Issues 5 (2): 159–169. https://doi.org/10.9770/jssi.2015.5.2(3).
- Prause, Gunnar, and Sina Atari. 2017. “On Sustainable Production Networks for Industry 4.0.” Entrepreneurship and Sustainability Issues 4 (4): 421–431. https://doi.org/10.9770/jesi.2017.4.4(2).
- Renzi, Cristina, Francesco Leali, Marco Cavazzuti, and Angelo Oreste Andrisano. 2014. “A Review on Artificial Intelligence Applications to the Optimal Design of Dedicated and Reconfigurable Manufacturing Systems.” The International Journal of Advanced Manufacturing Technology 72: 403–418. https://doi.org/10.1007/s00170-014-5674-1.
- Seow, Yingying, and Shahin Rahimifard. 2011. “A Framework for Modelling Energy Consumption Within Manufacturing Systems.” CIRP Journal of Manufacturing Science and Technology 4 (3): 258–264. https://doi.org/10.1016/j.cirpj.2011.03.007.
- Severengiz, M., J. Seidel, J. G. Steingrímsson, and G. Seliger. 2015. “Enhancing Technological Innovation with the Implementation of a Sustainable Manufacturing Community.” Procedia CIRP 26: 52–57. https://doi.org/10.1016/j.procir.2014.07.177.
- Shang, Xinwen, Jelena Milisavljevic-Syed, Sihan Huang, Guoxin Wang, Janet K Allen, and Farrokh Mistree. 2021. “A key Feature-Based Method for the Configuration Design of a Reconfigurable Inspection System.” International Journal of Production Research 59 (9): 2611–2623. https://doi.org/10.1080/00207543.2020.1735664.
- Shrouf, Fadi, Joaquin Ordieres, and Giovanni Miragliotta. 2014. “Smart Factories in Industry 4.0: A Review of the Concept and of Energy Management Approached in Production Based on the Internet of Things Paradigm.” Paper Presented at the 2014 IEEE International Conference on Industrial Engineering and Engineering Management.
- Souifi, Amel, Zohra Cherfi Boulanger, Marc Zolghadri, Maher Barkallah, and Mohamed Haddar. 2022. “Uncertainty of key Performance Indicators for Industry 4.0: A Methodology Based on the Theory of Belief Functions.” Computers in Industry 140: 103666. https://doi.org/10.1016/j.compind.2022.103666.
- Stark, Rainer, Hendrik Grosser, Boris Beckmann-Dobrev, Simon Kind, and INPIKO Collaboration. 2014. “Advanced Technologies in Life Cycle Engineering.” Procedia CIRP 22: 3–14. https://doi.org/10.1016/j.procir.2014.07.118.
- Stock, Tim, and Günther Seliger. 2016. “Opportunities of Sustainable Manufacturing in Industry 4.0.” Procedia CIRP 40: 536–541. https://doi.org/10.1016/j.procir.2016.01.129.
- Sullivan, John Lorenzo, Andrew Burnham, and Michael Wang. 2010. Energy-consumption and Carbon-Emission Analysis of Vehicle and Component Manufacturing. Argonne, IL, USA: Argonne National Lab.(ANL).
- Trentesaux, Damien, Theodor Borangiu, and André Thomas. 2016. “Emerging ICT Concepts for Smart, Safe and Sustainable Industrial Systems.” In, 1-10. Elsevier.
- Wang, Ru, Jelena Milisavljevic-Syed, Lin Guo, Yu Huang, and Guoxin Wang. 2021. “Knowledge-based Design Guidance System for Cloud-Based Decision Support in the Design of Complex Engineered Systems.” Journal of Mechanical Design 143 (7): 072001. https://doi.org/10.1115/1.4050247.
- Wang, Guoxin, Xiwen Shang, Yan Yan, Janet K Allen, and Farrokh Mistree. 2018. “A Tree-Based Decision Method for the Configuration Design of Reconfigurable Machine Tools.” Journal of Manufacturing Systems 49: 143–162. https://doi.org/10.1016/j.jmsy.2018.07.003.
- Xu, Xun, Yuqian Lu, Birgit Vogel-Heuser, and Lihui Wang. 2021. “Industry 4.0 and Industry 5.0—Inception, Conception and Perception.” Journal of Manufacturing Systems 61: 530–535. https://doi.org/10.1016/j.jmsy.2021.10.006.
- Yelles-Chaouche, Abdelkrim R., Evgeny Gurevsky, Nadjib Brahimi, and Alexandre Dolgui. 2021. “Reconfigurable Manufacturing Systems from an Optimisation Perspective: A Focused Review of Literature.” International Journal of Production Research 59 (21): 6400–6418. https://doi.org/10.1080/00207543.2020.1813913.
- Zhang, Jiafeng, Mohamed Khalgui, Mohamed Boussahel Wassim, Georg Frey, ChiTin Hon, Naiqi Wu, and Zhiwu Li. 2015. “Modeling and Verification of Reconfigurable and Energy-Efficient Manufacturing Systems.” Discrete Dynamics in Nature and Society 2015. https://doi.org/10.1155/2015/813476.
- Zhao, G. Y., Z. Y. Liu, Yan He, H. J. Cao, and Y. B. Guo. 2017. “Energy Consumption in Machining: Classification, Prediction, and Reduction Strategy.” Energy 133: 142–157. https://doi.org/10.1016/j.energy.2017.05.110.