Abstract
The inventory management of medical items in humanitarian operations is a challenging task due to the intermittent nature of their demand and long replenishment lead-times. An effective response to humanitarian emergencies is often associated with excess inventories, which leads to high costs. Henceforth, using accurate demand forecasts to control the inventories of these medical items is shown to be a significant lever to reduce inventory costs while keeping high service levels. This paper investigates the effectiveness of parametric and non-parametric demand forecasting methods that are commonly considered to deal with items characterised with intermittent demand patterns. Moreover, we propose a new hybrid deep learning method that combines the long short-term memory neural network and the support vector regression. Such combination enables to deal with complex and non-linear data. We conduct an empirical investigation by means of data related to 523 medical items managed in two warehouses of a major humanitarian organisation. The forecast accuracy and the inventory efficiency of the methods are analysed. The empirical results show the high performance of the deep learning methods. These insights are valuable not only in the context of medical items in humanitarian operations, but also for any items with intermittent demand patterns.
Disclosure statement
No potential conflict of interest was reported by the author(s).
Data availability statement
The data that support the findings of this study are available from the corresponding author upon request.
Additional information
Notes on contributors
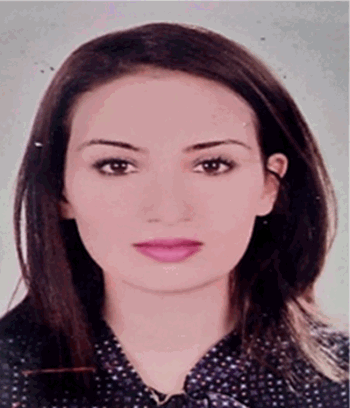
Marwa Hasni
Marwa Hasni is Assistant professor in Industrial Engineering at ENIB – University of Carthage. She holds a PhD in Industrial Engineering from the National Engineering School of Tunis- University of Tunis El Manar and member of the Laboratory of OASIS lab at the National Engineering Scholl of Tunis since 2016. She also holds a diploma of Engineer in Industrial Engineering and Logistics of the National Engineering School of Carthage-University of Carthage. Her research focuses on the development of machine learning based models for improving operational performances within industrial and healthcare systems. Marwa Hasni has published and reviewed a number of research studies on the side of several international journals namely: International Journal of Production Economics, International Journal of Production Research, International Journal of Decision Sciences, Risk and Management, Managerial and Decision Economics, International, Journal of Economics, and Strategic Management of Business Processes.
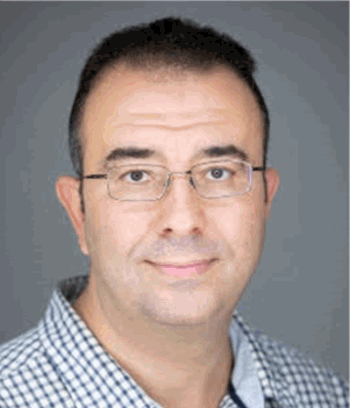
M. Zied Babai
M. Zied Babai is Senior Professor in Operations Management at Kedge Business School. He holds a PhD in Industrial Engineering from Ecole Centrale Paris (France) where he also worked as a Teaching and Research Assistant for four years. From October 2006 to September 2008, he joined the Centre for Operational Research and Applied Statistics at the University of Salford (UK), working on a project funded by the Engineering and Physical Sciences Research Council (EPSRC, UK). His research interests relate primarily to operations and supply chain management including demand forecasting and inventory management with a special emphasis on the development of quantitative models. He is the Editor-In-Chief of Supply Chain Forum: An International Journal (Francis & Taylor), Area Editor of IMA Journal of Management Mathematics (Oxford Press) and Associate Editor of International Journal of Production Research (Francis & Taylor).
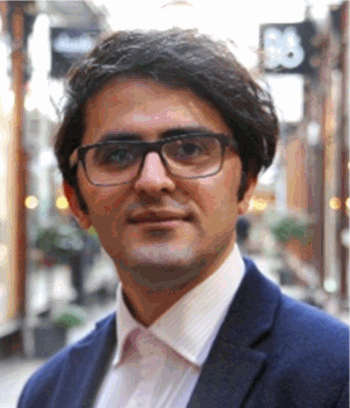
Bahman Rostami-Tabar
Bahman Rostami-Tabar, Professor of Data-Driven Decision Science at Cardiff University, the United Kingdom, is the director of Data Lab for Social Good at Cardiff Business School. His research focus lies in leveraging analytical methodologies to provide informed insights from data and modelling for policy & decision-making processes in sectors contributing to social good.