ABSTRACT
This paper considers the fault-tolerant optimised tracking control (FTOTC) problem for unknown discrete-time linear system. A research scheme is proposed on the basis of data-based parity space identification, reinforcement learning and residual compensation techniques. The main characteristic of this research scheme lies in the parity-space-identification-based simultaneous tracking control and residual compensation. The specific technical line consists of four main contents: apply subspace aided method to design observer-based residual generator; use reinforcement Q-learning approach to solve optimised tracking control policy; rely on robust H∞ theory to achieve noise attenuation; adopt fault estimation triggered by residual generator to perform fault compensation. To clarify the design and implementation procedures, an integrated algorithm is further constructed to link up these four functional units. The detailed analysis and proof are subsequently given to explain the guaranteed FTOTC performance of the proposed conclusions. Finally, a case simulation is provided to verify its effectiveness.
Acknowledgments
Authors would like to thank the associate editor and the anonymous reviewers for their valuable comments and suggestions.
Disclosure statement
No potential conflict of interest was reported by the authors.
Additional information
Funding
Notes on contributors
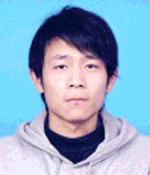
Ke-Zhen Han
Ke-Zhen Han was born in Zibo, China, in 1988. He received his B.S. degree in Automation from Dezhou University, Dezhou, China, in 2010, and the M.S. degree in Control Theory and Control Engineering from Bohai University, Jinzhou, China, in 2013. Now, he has been pursuing the Ph.D. degree in Control Theory and Control Engineering with Northeastern University, Shenyang, China, since 2013. His main research interests include robust filtering, optimal control, fault diagnosis, digital twin and system identification.
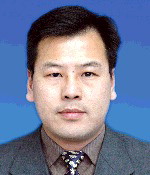
Jian Feng
Jian Feng received his B.S. degree, the M.S. degree and the Ph.D. degree in control theory and control engineering from Northeastern University of China, Shenyang City, China, in 1993, 1996 and 2005, respectively. He is a professor, doctoral supervisor and vice head of the Electric Automation Institute, Northeastern University. His main research interests are fault diagnosis, signal processing, neural networks and their industrial application.
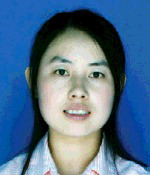
Xiaohong Cui
Xiaohong Cui received her B.S. degree in Mathematics and Applied Mathematics, M.S. degree in Operational Research and Cybernetics from Liaoning University and Northeastern University, Shenyang, China, in 2006 and 2008 respectively. She has been pursuing the Ph.D. degree in Control Theory and Control Engineering with Northeastern University, Shenyang, China, since 2013. She is currently working in Mudanjiang Normal University as a lecturer. Her research interests include approximate dynamic programming, neural networks adaptive control, and nonzero-sum games.