ABSTRACT
The robust linear parameters varying systems identification method with multiple-model approach is addressed in this paper. Various noise and outliers commonly exist in practical industrial processes and have a serious impact on data-driven system identification methods. A statistic approach is proposed in the paper where the centralised asymmetric Laplace (CAL) distribution is employed to model the noise and therefore the parameters estimation algorithm based on CAL distribution is robust to the symmetric/asymmetric noise and outliers. CAL distribution is represented as the product of exponential distribution and Gaussian distribution, and therefore the parameters estimation formulas are deduced in the expectation maximisation algorithm framework. The efficacy and the robustness of the proposed algorithm are verified by a numerical example and the continuous stirred tank reactor system experiment.
Disclosure statement
No potential conflict of interest was reported by the authors.
Additional information
Funding
Notes on contributors
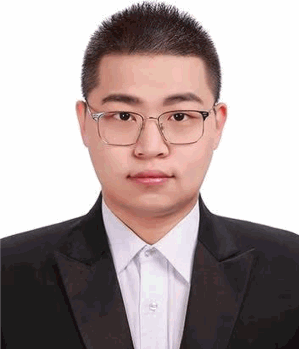
Miao Yu
Miao Yu received the B.E. degree from College of Power and Energy Engineering, Harbin Engineering University, and the M.E. degree in the Institute of Intelligent Control and System, Harbin Institute of Technology, Harbin, China, in 2018 and 2020, respectively. He is currently pursuing the Ph.D. degree with the Information Technology PhD program, Politecnico di Milano, Milano, Italy. His research interests include system identification and machine learning.

Xianqiang Yang
Xianqiang Yang (Member, IEEE) received the B.E. degree in automation and the M.E. degree in control theory and control engineering from the Shandong University of Science and Technology, Qingdao, China, in 2008 and 2011, respectively, and the Ph.D. degree in control science and engineering from the Harbin Institute of Technology, Harbin, China, in 2015. He is currently an Associate Professor with the School of Astronautics, Harbin Institute of Technology. His research interests include identification, soft sensor development, and image processing.
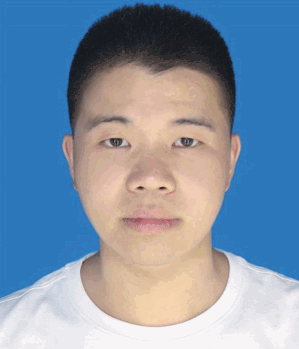
Xinpeng Liu
Xinpeng Liu received the B.E. degree from School of Astronautics, Northwestern Polytechnical University, Xian, China, in 2017. He is currently pursuing the Ph.D. degree in the Institute of Intelligent Control and Systems, Harbin Institute of Technology, Harbin, China. His research interests include system identification, machine learning and computer vision.