ABSTRACT
In this paper, we present an innovative decentralised control framework, designed to address stochastic dynamic complex systems that are influenced by multiple multiplicative noise factors. Our advanced approach builds upon the foundation of conventional Decentralised Fully Probabilistic Design (DFPD) by refining the Riccati equation to accommodate multiple noise sources effectively. By embracing the inherent stochastic nature of complex systems, our methodology fully characterises their dynamic behaviours using probabilistic state–space models, delivering a comprehensive representation of subsystem components. Importantly, the DFPD approach also incorporates system and input constraints by characterising their corresponding ideal distributions, ensuring optimal functionality and performance while adhering to permissible boundaries. To further enhance system performance, we introduce a probabilistic message passing architecture that enables seamless communication between neighbouring subsystems and promotes harmonised decision-making among local nodes. To demonstrate the efficacy of our proposed framework, we employ a three-inverted pendulum system as a numerical example and compare its performance to that of the conventional DFPD. Through this comparison, we showcase the advantages of our novel decentralised control approach in handling complex systems with multiple noise factors.
Disclosure statement
No potential conflict of interest was reported by the author(s).
Data availability statement
The authors confirm that the data supporting the findings of this study are available within the article [and/or] its supplementary materials.
Correction Statement
This article has been corrected with minor changes. These changes do not impact the academic content of the article.
Additional information
Notes on contributors

Yuyang Zhou
Yuyang Zhou received her B.Sc. in Electrical & Electricity Engineering School in The University of Manchester, United Kingdom, 2014 and Electrical & Engineering School in Beijing JiaoTong University, China, 2014, and later her Ph.D. in control theory from Electrical & Electricity Engineering School in The University of Manchester, United Kingdom, 2018. After that, she became a Research Associate for three years in the Faculty of Engineering and Applied Science at Aston University, Birmingham, United Kingdom. She currently is a lecturer in group of Engineering and Mathematics, School of Computing, Engineering and Built Environment, Edinburgh Napier University, United Kingdom. Her current research focuses on probabilistic and stochastic control, minimum entropy control, pdf control and decentralized control, time-delay systems, networked and complex systems control with applications to power grids and Kalman filtering.
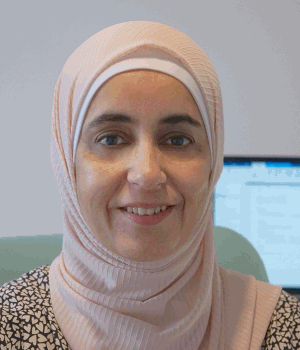
Randa Herzallah
Randa Herzallah received her B.Sc. degree in Industrial Engineering, her M.Sc. degree in Industrial Engineering/Manufacturing and Design from Jordan University, Jordan, and her PhD degree in Control Theory from Aston University, Birmingham, UK. She is currently an Associate Professor at the Warwick Mathematics Institute at the University of Warwick. She previously held the positions of Reader in the College of Engineering and Physical Sciences at Aston University, UK, Associate Professor at Al-AHliyya Amman University, and Professor at Al-Balqa Applied University, Jordan. Her general research focus is on the general topic of systems modelling, optimization, design, and control. Her current research interests include nonlinear control theory, probabilistic, and stochastic control; intelligent control; pinning control; adaptive control; distributed and decentralized control; optimal control; signal processing; time-delay systems; networked and complex systems control with applications to power grids; and manufacturing systems and biomedical signals.

Qichun Zhang
Qichun Zhang received the B.Eng. degree in automation and the M.Sc. degree in control theory and control engineering from Northeastern University, China, in 2008 and 2010, respectively, and the Ph.D. degree in electrical and electronic engineering from The University of Manchester, U.K., in 2016. He is currently an Assistant Professor in Computer Science with the University of Bradford, U.K. Before joining the University of Bradford, he was a Senior Lecturer in Dynamics and Control with De Montfort University, a Senior Research Officer in Neural Engineering with the University of Essex, and an Academic Visitor with the Control Systems Centre, the University of Manchester. His research interests include stochastic systems optimisation, stochastic distribution control, non-Gaussian filtering, and biological computational modelling. He serves as an active reviewer and an Associate Editor of Journal of Intelligent Manufacturing.