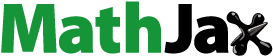
Abstract
Relative to other developing regions, the role of land consolidation in increasing crop yields is poorly understood in sub-Saharan Africa. This paper examines the role of land use consolidation on agricultural productivity among smallholder farmers in Rwanda. Household-level data are used to estimate a fixed-effects model with matched control groups to mitigate selection bias. The study finds a positive association between land use consolidation and crop yields, but only among farm households with landholdings greater than one hectare, which is well above the average farm size in Rwanda. Findings also point to the importance of non-organic fertilisers and irrigation as there appear to be significant benefits associated with further increases in their use among the consolidated farms.
1. Introduction
Increasing the productivity of the agricultural sector is important to improve rural welfare and achieve economic growth in developing countries (Johnston & Mellor, Citation1961), given that most poor households live in rural areas and depend on agriculture for their survival as they combine small-scale food cropping and livestock rearing with a diverse set of agricultural related activities. This is particularly evident in Rwanda where 80 per cent of the population live in rural areas, have agriculture as their primary source of income, and cultivate landholdings smaller than one hectare. The important role of agriculture for rural development and food security has motivated policies with the aim to create the conditions for improved agricultural productivity. In Rwanda, owing to increasing land fragmentation and population pressure, land use consolidation was implemented in 2008 in the framework of the Crop Intensification Programme (CIP). The basic idea is that farmers consolidate their land parcels and cultivate one selected crop while keeping ownership of their lands intact (MINAGRI, Citation2009). This should result in an expansion of the land area under cultivation of priority crops, increased crop yields, and improved food security among farm households. Participation in land use consolidation is voluntary but it is a prerequisite to join the CIP programme which distributes agricultural inputs in the form of non-organic fertilisers and seeds for priority food crops.Footnote1
From the start of Asia’s Green Revolution there is ample evidence that investments to improve agricultural productivity, through crop intensification and land consolidation, have been important for rural poverty reduction and aggregate incomes in many developing regions (Dethier & Effenberger, Citation2012; Timmer, Citation2002). Different types of consolidation policies have contributed to improved land management and agricultural productivity in Asia, Europe, and South America (Crecente, Alvarez, & Fra, Citation2002; Niroula & Thapa, Citation2005; Pašakarnis & Maliene, Citation2010). However, this evidence applies to regions that have favourable agroecological conditions and high technological adoption. Considerable concern remains about the importance of comparable agricultural policies for poverty reduction in the context of sub-Saharan Africa (SSA) (Dorward, Kydd, Morrison, & Urey, Citation2004). Farmers in sub-Sahara are dependent on rainfed agriculture, have limited access to harvest and postharvest technologies and face high production and market risks (Ali, Deininger, & Duponchel, Citation2014; Tilman, Cassman, Matson, Naylor, & Polasky, Citation2002). Under such conditions, little is known about the extent to which land use consolidation may lead to improved land management and agricultural productivity; whether these potential benefits are realised depends on the ability of farmers to adapt to the policy (Du, Zhang, & Jin, Citation2018), as well as the implementation and specifics of the policy, which calls for country specific studies. Previous studies that focus on Rwanda tend to be limited as they focus on the conditions that apply in certain geographic areas, provinces, or districts, with the limitation that their results are not generalisable. Bizoza and Havugimana (Citation2013) find that gender, family size, and trust are among the most important determinants of a farmer’s decision to adopt land use consolidation but for only one district in Rwanda, and the study does not address the effects on crop yields. Considering the increased focus on land use consolidation as a key policy to address food insecurity and the amount of resources allocated to its implementation, there is a need for further studies on a nationwide scale.
This study addresses the effects of land use consolidation on crop production using a random sample of 1538 households across Rwanda of which 25 per cent participated in land use consolidation between 2010 and 2014. These data are obtained from two of the latest rounds of the Integrated Household Living Conditions Survey (EICV), 2010 and 2014, conducted by the National Institute of Statistics in Rwanda (NISR). Assessing the impact of policies to spur agricultural productivity is challenging from a methodological viewpoint. Better skilled and motivated farmers are more likely to participate in welfare enhancing governmental programmes. It is also difficult to interpret the effect of land use consolidation, and the associated use of improved inputs, as participation is not random but concentrated to larger farms and the districts in Rwanda that have better agroecological conditions (Cioffo, Ansoms, & Murison, Citation2016). As an attempt to mitigate selection bias, this study applies a model with household fixed effects and the coarsened exact matching method to estimate a control group to the farm households that participate in land use consolidation (Iacus, King, & Porro, Citation2009). This study estimates the average treatment effect on treated, permitting a comparison of the outcome between farm households that participate in land use consolidation (between 2010 and 2014) and similar farm households that did not participate. Unlike previous studies, this study models the selection bias associated with land use consolidation and estimates the effects on the value of per hectare crop yields.
2. Background
Agricultural productivity growth in SSA lags behind the rest of the world, which has led to debates about the importance of agriculture in economic development (Dorward et al., Citation2004; Diao, Hazell, & Thurlow, Citation2010; Dethier & Effenberger, Citation2012; Jayne, Mather, & Mghenyi, Citation2010). The debate is about the best ways to promote poverty alleviation among small-scale farmers that depend on rainfed agriculture and operate under conditions of land scarcity, population pressure, low technological development, and high production and market risks (Collier & Dercon, Citation2014). Small-scale farming is widespread in Rwanda and farmers typically cultivate several small plots at different locations to minimise risk and take advantage of varying soil fertility. The average size of a landholding is 0.76 hectares and is typically divided over four to five plots at different locations (NISR, Citation2016). Several benefits can be linked to land fragmentation and diversified cropping systems as they allow farmers to hedge for seasonality and improve their risk management (Blarel, Hazell, Place, & Quiggin, Citation1992). Being able to grow different crops at multiple locations is particularly important among farmers with limited landholdings as they tend to use crop diversification as a risk minimising strategy (Cioffo et al., Citation2016). However, studies do also argue that land fragmentation can be an obstacle to overall agricultural growth as it gives rise to transaction costs. Transaction costs arise because of distance to travel between multiple plots, lack of supervision, and inefficiencies associated with input use and farm management (Tan, Heerink, & Qu, Citation2006; van Dijk, Citation2003). Niroula and Thapa (Citation2005) show that fragmented land tends to produce a farm infrastructure that prevents the efficient use of agricultural inputs as it is costly to apply inputs evenly across plots, thereby undermining the efficient use of land. Land fragmentation may also be detrimental to agricultural modernisation as it discourages farmers from adopting new productivity enhancing technology (Du et al., Citation2018).
2.1. Land use consolidation in Rwanda
The view that land fragmentation gives rise to high costs of production and unproductive farms has been the motivation behind several land reforms and agricultural intensification programmes. In Rwanda, land use consolidation was implemented in 2008 with the overall goal to overcome the effects of land fragmentation by increasing the scale of agricultural yields and stimulating a transformation to a competitive and market oriented agricultural sector (MINAGRI, Citation2009). The policy also aims to promote a more productive use of land resources by facilitating the implementation of soil and water conservation practices and by improving the opportunities for and benefits derived from investments in productivity enhancing inputs (Bizoza & Havugimana, Citation2013; USAID, Citation2007). There are some aspects that distinguish consolidation in Rwanda from other countries in which comparable policies have been implemented. The policy was initiated by the government of Rwanda through the Ministry of Agriculture and Animal Resources as a part of the CIP programme. The CIP operates across the country and has the overall goal to improve food security and farmers’ incomes. The programme focuses on increasing the production of priority food crops and distributing agricultural inputs to the consolidated farmers, including seeds for the priority crops and non-organic fertilisers. Participation in consolidation is voluntary, and farmers are incentivised to consolidate as it provides them access to productivity enhancing inputs through the CIP. Another aspect that distinguishes consolidation in Rwanda is that it is defined by consolidation in the use of land rather than in the ownership, so farmers can choose to engage in a process of putting together their land parcels under the cultivation of one selected crop, while keeping ownership of their lands intact (Kathiresan, Citation2012).
2.2. Land use consolidation and agricultural productivity
Given effective implementation, land use consolidation can improve the productivity of household crop production through several mechanisms. A reduction in transaction costs, including the costs of transporting inputs and outputs across plots, and other operational difficulties, should increase labour productivity and enable more efficient use of the land (van Dijk, Citation2003). Improvements in land quality through improved access to agricultural inputs, fertilisers, and improved seeds should also lead to increases in land productivity. Specifically, the initiative to distribute agricultural inputs to the consolidated farms, through the CIP, is expected to be one of the major factors in raising the productivity of land among the consolidated farms in Rwanda (Kathiresan, Citation2012; MINAGRI, Citation2009). Studies from South Asia and Europe lend support to this view, showing that similar types of land consolidation programmes have contributed to improved agricultural productivity and land management mainly because of improved access to non-organic fertilisers and new seed varieties (Crecente et al., Citation2002; Niroula & Thapa, Citation2005). Land use consolidation in Rwanda is also designed to facilitate the adjustment to priority food crops selected based on the available land and the agroecological conditions that apply in each district (Kathiresan, Citation2012). This may enable households to reap benefits associated with the districts comparative advantages (Wu, Liu, & Davis, Citation2005). Moreover, and given that land use consolidation result in increased crop yields, it can allow farmers to produce both for subsistence and for the markets which increases their market orientation and possibility to take part in non-farm activities (Bizoza & Havugimana, Citation2013). Finally, land use consolidation may also lead to improved land productivity because of efforts to improve the sustainable use of land through the adoption of soil and water conservation practices. The CIP contains measures to facilitate the implementation of techniques for irrigation, terracing, and protection against erosion among the consolidated farms.
Although there is ample evidence that comparable policy programmes have been important in increasing crop yields, studies have noted that efforts to spur economies of scale in agriculture may be challenging in the context of SSA (Diao et al., Citation2010). The rationale can be linked to theories on farming systems in tropical areas developed by Biswanger & Rosenzweig (Citation1986), Boserup (Citation1965), and Ruthenberg (Citation1980). These studies emphasise the problems that arise when population pressure determines the labour intensity of farming under conditions of low technological development. Given population pressure, the labour requirements per unit of output will rise if the supply of productivity enhancing techniques remains unchanged (Boserup, Citation1965). Small-scale farmers have limited landholdings and they often lack the resources and collateral needed to invest in new technologies. Thus, the small scale of farms in Rwanda may prevent the adoption of productivity enhancing techniques and incentives to reduce fragmentation may be limited to farms with access to enough land and capital (Wu et al., Citation2005). Some of the benefits associated with reduced land fragmentation can be linked to saving labour input, but in Rwanda, with surplus labour and small-scale farming, crop production is dependent on labour rather than capital inputs. Moreover, the high prevalence of subsistence farming means that farm households tend to engage in diversified rather than specialised crop production (Blarel et al., Citation1992).
Another relevant issue is the observed inverse relationship between farm size and productivity in many countries in Africa. Studies have found that smaller farms in countries such as Rwanda, Kenya, Uganda, and Tanzania are more productive than larger ones (Carletto, Savastano, & Zezza, Citation2013; Holden & Otsuka, Citation2014; Larson, Otsuka, Matsumoto, & Kilic, Citation2014). Ali, et al. (Citation2014) and Ansoms, Verdoodt, and Van Ranst (Citation2008) show evidence of a strong inverse relationship between farm size and productivity in Rwanda; Ansoms et al. (Citation2008) also found a slight positive impact of plot fragmentation on farm productivity. These findings do not support the alleged scale hypothesis, which is essential in the debate on land use consolidation.Footnote2 Ali et al. (Citation2014) explain the inverse size productivity relationship found among Rwandan farmers with failures in markets for agricultural land, labour, and capital, which prevents small farmers from expanding. This follows the view of Sen (Citation1966), who noted that when markets for land and labour are absent or inefficient, productive farms will not be able to expand as they are unable to acquire more land and rely on family labour. Inefficient labour markets can also prevent farmers from measuring the alternative cost of their labour with the result that they may continue in unproductive activities (Byiringiro & Reardon, Citation1996). Such arguments are supported by the finding that the inverse size productivity relationship generally weakens with technical progress and modernisation (Foster & Rosenzweig, Citation2010). Sheng, Zhao, Nossal, and Zhang (Citation2015) highlight the difference between ‘returns to scale’ and ‘returns to size’ in agriculture. They show that large farms in Australia achieved higher productivity by adopting production technology rather than increasing their scale. They also argue that productivity improvements among smaller farms can be made through increasing their ability to access new technologies, rather than simply expanding their scale. Whether land use consolidation can improve crop productivity when combined with efforts to spur technological adoption and crop intensification has not yet been addressed in the context of Rwanda.
3. Data and estimated model
The data used for this study are household-level survey data obtained from two of the latest rounds of the EICV. These are nationwide surveys conducted by NISR with the aim to collect welfare indicators from a random sample of households across the country. These data are publicly available and considerable efforts are made to ensure representativeness. The households are chosen to be nationally representative and they are stratified at the district and urban/rural levels and those that relocated, or split, were tracked to obtain current information for the corresponding household members (NISR, Citation2016, p 6). This study uses the most recent EICV survey, conducted in 2013–2014, which is combined with the earlier survey, conducted in 2010–2011, and only those 1920 households that were surveyed in both rounds are included to enable repeated measurement over time. The sample used in the estimations is restricted to include only farm households implying that 382 households located in the capital region Kigali and other urban areas, that depend entirely on non-agricultural wage work, are removed. This result in a balanced panel with 1538 households and 3076 observations. The geographic coverage of the sample households used in the estimations and the consolidated farms are illustrated in Figure S1 (in Supplementary Materials). Having access to a sample of households observed twice, the baseline equation can be expressed as:
where denote the dependent variable of household
at time
corresponding to years 2010 and 2014, respectively,
denote fixed characteristics of the household, and
is a treatment dummy that takes the value one if the household participated in land use consolidation during 2010–2014, but not prior to 2010, and zero if the household did not participate before or during the studied period. Moreover,
denote a time dummy,
denote a vector of household and locational controls and
is the composite error term. Following the first-difference approach, Equation (1) can be expressed as:
Apart from the household fixed effect which is differenced away, Equation (2) is identical to the one above, with the time dummy incorporated in
. Should there be any selection bias resulting from household specific factors that are time-invariant, Equation (2) should address this (Hsiao, Pesaran, & Tahmiscioglu, Citation2002). However, there are other confounding factors that could violate the assumption of exogeneity. One issue is that participation in land use consolidation in Rwanda is not random but concentrated in larger farms, in particular among medium and large farms able to invest in capital intensive agricultural techniques (Cioffo et al., Citation2016). Based on what has emerged from the previous literature, it also seems like subsidised inputs, through the CIP, are provided in greater supply to those districts that have favourable agro-ecological conditions (Bizoza & Havugimana, Citation2013).
3.1. Coarsened exact matching (CEM)
The empirical approach to account for selection bias is to use the CEM technique and estimate a control group that is as similar as possible to the consolidated farm households (Iacus et al., Citation2009; Iacus, King, & Porro, Citation2012). The main motivation for CEM is that it allows the balance between the treatment and the control group to be chosen ex ante rather than being revealed through an iterative process of ex post balance checking. The CEM guarantees that adjusting the imbalance on one variable has no effect on the balance of other variables, and since the matching is done ex ante, it reduces the degree of model dependence (Ho, Imai, King, & Stuart, Citation2007). To improve the balance between the treated and the control group the CEM approach is to temporarily and ex ante coarsen all pre-treatment covariates used in the matching and then match on the coarsened data, using the uncoarsened values of the matched units in the subsequent regression analysis. Given the available data and the limitation that households can only be observed twice, households that participated in land use consolidation between 2010–2014 (but not prior to 2010) are compared to households that reported they did not take part in land use consolidation prior to 2010 nor between 2010–2014. This implies that the matched control group is based on a set of relevant pre-treatment characteristics observed in 2010. Due to limitations in the EICV data it is not possible to cover a longer time period which may lead to an underestimation of the effects associated with land use consolidation. Having this limitation in mind, the significance levels are treated as lower bounds in the interpretation of the results.
The variables used in the matching are selected using the Wald test and a logistic regression to determine which of the predictor variables are significant in explaining the probability to adopt land use consolidation. Results show that location, by district, the size of landholdings, and expenditures on agricultural inputs are positive and significant at the 1 per cent level.Footnote3 Hence, the size of landholdings is included to account for the selection linked to farm size and district is included to account for differences in agroecological conditions. Household expenditures on agricultural inputs including fertilisers, irrigation, and improved seeds are included to account for similarities linked to input use and the fact that inputs are not restricted to the consolidated farm households. Since the CEM produces a balanced match on all pre-treatment covariates, it guarantees that the consolidated farms are compared to farm households of similar size, in the same district, and with equivalent expenditures on agricultural inputs.
Results of the matching are displayed in Tables S1 and S2 in Supplementary Materials showing that the CEM reduces the imbalance between the treated and the control group. The overall heterogeneity between the groups is indicated by the multivariate L1 distance and the outcome can be evaluated by comparing the value of the L1 statistic in the pre-match test with the value in the post-match test and by comparing the difference in means, which are indicated in the two first columns in Table S1. Including all three variables (algorithm 2) shows that the LI statistic reduces from 0.42 to 0.36, indicating that the matching improves the balance between the groups. Comparing the outcome of the matching algorithms shows that the overall heterogeneity increases as more variables are added to the CEM. The weights generated through the match are included in the estimation of Equation (2) and robustness test are conducted to assess if the choice of the variables included in the match influences the outcome. Iacus et al. (Citation2012) show that including the weights in the subsequent regression analysis is equivalent to a difference-in-difference and yields an unbiased estimate of the average treatment effect on treated. They also show that the inclusion of control variables (including those used in the matching) further reduces the imbalance between the treated and non-treated. The rationale is that there will naturally remain imbalance even after the matching, as it is impossible to fully account for heterogeneity between the groups.Footnote4 Thus, the inclusion of household and locational controls in the subsequent regression, as indicated in Equation (2), accounts for the remaining heterogeneity.
3.2. Dependent and independent variables
To assess the effects of land use consolidation on household crop production this study uses the value of crop yields divided by the number of hectares of land as the dependent variable. This indicates the ratio of crop output to the input factor land, which is a common approach to measure agricultural productivity (Ali et al., Citation2014). presents variable definitions and summary statistics for the dependent and independent variables and Table S3 (in Supplementary Materials) displays summary statistics for the two survey waves. Summary statistics show that average per hectare crop yields amount to an average of 793 thousand Rwandan francs and that crop yields have increased over the studied period.
Table 1. Definitions and summary statistics
The independent variable in focus is the treatment dummy , but the model is also estimated with a continuous treatment variable using the share of land in consolidation calculated as the number of hectares in consolidation divided by total landholdings. This follows the approach in Zhu, Demeter, and Lansink (Citation2012) who uses the share rather than the actual level in the estimation of the DiD. The rationale is that the size of the consolidation project increases with the size of the farm, which gives rise to multicollinearity. shows that 25 per cent of the households in the sample participated in land use consolidation between 2010 and 2014 and that the average share of land in consolidation amounts to 0.46 hectares. Table S4 (Supplementary Materials) presents summary statistics split by the consolidated and the non-consolidated with a focus on crop yields, input use, and location by province. Mean value comparisons show that the consolidated have larger landholdings compared to the non-consolidated, 0.87 hectares compared to 0.58. They also have higher crop yields, both in terms of value and weight. The value of per hectare crop yields is indicated to be almost 66 per cent higher among the consolidated. Location by province shows that 23 per cent of the consolidated farm households are in the Eastern Province, 25 per cent in the Western, 23 in the Southern Province, and 27 and 2 per cent in the Northern and Kigali Province, respectively.Footnote5 The surveys are also designed to collect information on the use of agricultural inputs and the production of priority crops. As discussed, land use consolidation is a prerequisite to take part in the CIP programme which identifies the priority crops, including Bananas, Beans, Cassava, Irish potatoes, Maize, Rice, Soy, and Wheat. Table S4 shows that the consolidated have higher overall expenditures on inputs, particularly chemical fertiliser and improved seeds. Average real expenditures on chemical fertilisers is almost 13 thousand Rwanda francs among the consolidated and 6 thousand among the non-consolidated. Household investment in irrigation is generally very low, although it is indicated to be almost three times higher among the consolidated. The cultivation of priority food crops as the main crop is also indicated to be more pronounced among the consolidated, especially the cultivation of bananas, beans, Irish potatoes, and rice. Specifically, the percentage of households that grow beans as their main crop is almost 12 times higher among the consolidated. Differences in socio-economic characteristics are not displayed in the table as they are less pronounced, female headed households are slightly underrepresented among the consolidated (24% versus 30%) and they have more primary and secondary education.
Household and locational variables are included to control for other influences on crop production. The approach is to include a broad set of variables to account for inputs, non-farm incomes, and wealth. The selection of variables and their definitions follows the approach of prior studies and they are hypothesised to improve crop yields as they reduce transaction costs and provide access to capital (Abdulai & CroleRees, Citation2001; Bigsten & Tengstam, Citation2011; Nilsson, Citation2018). The amount of land is included to proxy for farm size and based on previous studies on Rwanda, the prediction is an inverse relationship between the size of land and agricultural productivity (Ali & Deininger, Citation2014; Amsoms et al., Citation2008). Including an interaction term defined as the share of land in consolidation multiplied with land is also used to assess size effects. Labour inputs (number of hours used in cropping activities) and other agricultural inputs, for example, organic and non-organic fertilisers, are included and they are defined and summarised in . Livestock is also a key variable as it can be used as collateral, which raises the ability to invest (Abdulai & CroleRees, Citation2001). Animals and their by-products may also provide a source of additional income for the family (Dercon & Krishnan, Citation2000). A variable that indicates the value of the livestock is therefore included.
A variable is included to proxy for land fragmentation calculated using the Simpson index in the following:
where denote the size of plot i. The S index ranges from zero to one and the larger the index value, the more fragmented is the land. In cases where the household only has one plot, S will be zero. Thus, the index value will increase with the number of plots and in cases when the size of the plots tends to be similar. The index will decrease when plot size increases (Anderson, Reynolds, Merfeld, & Biscaye, Citation2017). Distance to all-weather roads is included to proxy for market access. Market access can lower the cost of marketing farm produce and may lead to new employment opportunities (Ellis, Citation1998). It can also increase agricultural production by connecting plots and improving the links between agricultural and non-agricultural activities in rural areas (Jayne et al., Citation2010). The share of total crop yields that is sold on markets and non-farm incomes are included to proxy for market orientation and the extent that farm households engage in non-farm activities. The share of crop yields sold on the market is also used to test if land-use consolidation improves farm household’s ability produce for the markets through an interaction term. To further account for farming practices, variables are introduced to control for the number of plots that are protected against erosion and the type of priority food crop cultivated by the household.
Variables are also included to control for socio-economic structure including the gender of the household head, education, access to credit, and remittances. Education is a key variable that enhances the ability of farmers to adopt more advanced technologies and crop-management techniques, which increases the rate of return on land (Rosegrant & Cline, Citation2003).
The ownership of land is often seen as an important indicator of household wealth as it can be used as collateral for loans (Deininger & Feder, Citation2009; Pritchard, Citation2013). In Rwanda, several reforms have been undertaken to improve land rights (including the land titling programme [LTR] and the ongoing reform for land tenure regularisation). Studies have found that the LTR programme resulted in significant improvements in the welfare and productivity of farmers and in strengthening women’s rights to land (Ali, Deininger, & Goldsten, Citation2011). The fact that reforms are implemented during the study period may influence farm household’s possibility to realise investments. However, due to limitations in the EICV data (namely the lack of a variable that indicate if households land rights improved), it is not possible to address this perspective. Due to data limitations, a variable is included to control for documented land rights in the form of having a lease certificate defined as the share of cultivated plots that have documented rights. Although this may not be a reliable indicator of household wealth it is included as a crude proxy for land rights. Lastly, the model is estimated using cluster robust standard errors at the district level to account for agro-ecological conditions and spatial autocorrelation.Footnote6
4. Regression results
Results from estimating Equation (2) including the pre-estimated CEM weights are presented in . Results in the first two specifications show the results of substituting between the dichotomous and the continuous treatment variables and the results in the third specification display the results from including an interaction term defined as the share of land in consolidation multiplied with landholdings, to assess size effects. The treatment variables indicate the unique effect of land use consolidation on crop yields and given the CEM weights, they indicate the average treatment effect on treated (Iacus et al., Citation2009). This means a comparison with non-consolidated farm households of similar size, in the same district, and with equivalent expenditures on improved inputs. The coefficient of the treatment dummy is insignificant in the first specification indicating that land use consolidation does not give rise to any significant differences in crop yields between the consolidated and the non-consolidated. This result holds when using the share of land in consolidation as the dependent variable. However, including the interaction term indicates a positive association between the combined effect of consolidation and farms size on crop yields. The interaction term is statistically significant at the 1 per cent level and since the term is the product of two continuous variables it is possible to compute the slope for crop yields on consolidation while holding the value of the moderator variable, land, constant. Results show that the slopes for consolidation are significant for all values of land above 1.10 hectares. This implies a positive association between land use consolidation and crop yields for farmers with landholdings greater than 1 hectare, which is well above the average farm size (0.66 in the sample). This finding is consistent with many other studies arguing that the productivity effects associated with land use consolidation should adhere to larger farms that have enough access to land and capital (Bizoza & Havugimana, Citation2013; Cioffo et al., Citation2016).
Table 2. Regression results: the effects of land use consolidation on per hectare crop yields
The results from the estimations also indicate a positive and significant association between the use of chemical fertilisers, irrigation, and crop yields. As a log form is used, the coefficients can be read as elasticities suggesting that a doubling of expenditures on chemical fertilisers is associated with a 4 per cent increase in the value of per hectare crop yields, and that a doubling of expenditures on irrigation is associated with a 3 per cent increase. There is no significant association between improved seeds and yields. One possible reason could be the inability to control for the quality of the seed varieties, which could give rise to measurement errors. In the absence of data that could offer means to control for this, it is difficult to know if the results are affected by quality or lack of supply in the local markets.
Results show a negative and significant association between land and the value of crop yields per unit of hectare indicating that a doubling of land result in a 63 per cent decrease in the value of output per hectare. Summing the elasticities for land, labour and agricultural inputs indicates that the studied households are more or less operating with decreasing returns to scale. Comparable results are found in studies that focus on counties with abundant rural labour (Wu et al., Citation2005). One issue is that the model does not include variables that directly controls for soil quality at the level of the farm. Given what is found in Ali et al. (Citation2014), the inability to control more precisely for soil quality characteristics should not bias the result. They use plot level data from Rwanda collected in 2010/2011 and show that the inverse size productivity relationship remains unchanged when soil quality characteristics are omitted, suggesting that measurement errors linked to land quality are not the reason for the regularity.
Results also suggest that an increase in land fragmentation is associated with a negative effect on yields. This finding is consistent with studies showing that fragmented land causes different types of transaction costs that tend to result in high production costs and unproductive farms (Du et al., Citation2018; Niroula & Thapa, Citation2005). Many of the control variables show the anticipated signs and are consistent with the view that factors such as market share, tenure, protection against erosion, and education are important in determining the productivity of farm households (Ali et al., Citation2014; Byiringiro & Reardon, Citation1996). Dummy variables for the priority food crops are included in all the estimations. The coefficient for Bananas is positive and significant at the 1 per cent level, whereas the coefficients for Irish potatoes, Maize, and Soy are all negative and significant across the estimations. To further disentangle the effects associated with land use consolidation the model is estimated including a set of interaction terms between land use consolidation and agricultural inputs. The rationale is to test if consolidation can improve crop yields when combined with efforts to spur the use of productivity enhancing inputs. The model is also estimated including an interaction term between consolidation and the share of crop yields sold on the market. The results are displayed in and the interaction terms are introduced individually to avoid multicollinearity. The interaction terms indicating the combined effect of land use consolidation and the use of chemical fertilisers and irrigation are positive and significant indicating that the use of such inputs is important in improving crop yields among the consolidated farms. Thus, there appear to be benefits associated with further increases in the scope of their use, which applies to the average consolidated farm and not only to the larger farms. These results are consistent with many other studies, from Asia and Europe, showing that land consolidation have contributed to improved crop production mainly because of improved access to productivity enhancing inputs (Crecente et al., Citation2002; Niroula & Thapa, Citation2005). The interaction term between consolidation and the share of crop yields sold on the market is also positive and significant suggesting that consolidation improves the ability to produce for the markets.
Table 3. Regression results: the effects of land use consolidation on per hectare crop yields including interaction terms
Additional robustness tests are performed to validate the results. The sampling weights have been included to control for the probability to be surveyed and the models have also been estimated using a 1 per cent trim of the dependent and the independent variables with no major changes to the results. Other interaction terms were also tested (between consolidation and fragmentation, labour input, and other inputs), but the coefficients were all insignificant. These results are available on request.
5. Conclusions
The effects associated with land use consolidation have received considerable attention in the literature, but much of the focus has been on countries or regions that have favourable agroecological conditions and high technological adoption. This study addresses the effects associated with land use consolidation in the context of Rwanda, which has received less attention. Recent debates have raised the issue that efforts to spur economies of scale, through land consolidation, may not be sufficient to raise agricultural productivity in the context of sub-Sahara (Diao et al., Citation2010; Collier & Dercon, 2013). The debate is linked to the finding that there is an inverse relationship between farm size and productivity in many countries in sub-Saharan Africa and that there are failures in input markets, which may prevent small farms from expanding (Ali et al., Citation2014; Ansoms et al., Citation2008).
Assessing the impact of land use consolidation is challenging since participation tends not to be random. This is found among a sample of farm households that participated in land use consolidation in Rwanda during 2010–2014. These data are from the two latest rounds of the EICV surveys (EICV3 and EICV4), showing that households that participated in land use consolidation have larger landholdings and higher yields compared to the non-consolidated. To account for selection bias, this study estimates a weighted fixed-effects model and the coarsened exact matching method (Blackwell, Iacus, King, & Porro, Citation2009).
Results indicate that land use consolidation give rise to a significant effect on crop yields, but only for the larger farms (1.10 hectares). This suggest that the positive effect on crop yields adhere to larger farms that have sufficient access to land. Results also indicate a positive and significant association between the use of chemical fertilisers, irrigation, and crop yields among the consolidated farms. This suggests that the association between land use consolidation and agricultural productivity depends importantly on accompanied investments in agricultural inputs in the form of chemical fertilisers and infrastructures for irrigation. The importance of supporting inputs emerging from the analysis calls for further analyses in this direction.
Due to limitations in the EICV data and the inability to cover longer time periods calls for additional studies to strengthen the findings. Specifically, the relatively short period covered by this study could limit the assessment and lead to an underestimation of the effects. The policy is also interlinked with other ongoing land reforms, which calls for further research. Despite these limitations, this study can be the starting point for more comprehensive evaluations of crop yields and agricultural productivity caused by land use consolidation in Rwanda. Further analyses on the role of land use consolidation using parcel level or plot level data and in contexts with more widespread mechanisation and technological progress could also help to shed additional light on important relationships. Exploring other aspects of the CIP programme, such as extension services and measures to spur market orientation and farmers’ motivation to participate, would also add to the understanding of land use consolidation in Rwanda.
Supplemental Material
Download PDF (350.5 KB)Acknowledgements
The author wishes to gratefully thank the editor and the anonymous referees for their most useful comments and suggestions. Any errors are on the authors own account. The data supporting the results together with codes are available on request ([email protected]).
Supplementary Materials
Supplementary Materials are available for this article which can be accessed via the online version of this journal available at https://doi.org/10.1080/00220388.2018.1520217
Disclosure statement
No potential conflict of interest was reported by the author.
Notes
1. The concepts of land consolidation and land use consolidation are often used interchangeably in Rwandan governmental reports, but they are essentially different from each other. Land consolidation is often referred to as a process that aims to reallocate fragmented land parcels used for agriculture to form large scale parcels for more rational land holding (Niroula & Thapa, Citation2005). Land use consolidation, implemented in Rwanda, refers to a process of putting together small land parcels under one selected crop to manage the land and use it in an efficient and uniform manner (Kathiresan, Citation2012). Hence, land use consolidation in the Rwandan context refers to a specific type of consolidation policy which applies in the use of land and under the cultivation of one of the priority food crops identified in the CIP.
2. Studies that show an inverse relationship between size and productivity are often questioned on the basis that they tend to rely on cross-sectional data and suffer from limitations in the measurement of land quality and landholdings, which could bias the results (Lamb, Citation2003; Collier & Dercon, 2013).
3. These results are not presented in the paper, but they are available upon request.
4. See Blackwell et al. (Citation2009) for a formal derivation and details on its implementation in Stata.
5. The limited number of consolidated farm households in the Kigali province reflects that this is the most urbanised region with relatively few households involved in agriculture. The lack of observations from this province implies that the empirical analysis does not apply to farm households in this province.
6. The data does not contain information about the geographic location at a more disaggregated level than the district, which prevents the model from accounting for spatial dependencies at the local (cell or village) level.
References
- Abdulai, A., & CroleRees, A. (2001). Determinants of income diversification amongst rural households in Southern Mali. Food Policy, 26(4), 437–452.
- Ali, D. A., & Deininger, K. (2014). Is there a farm-size productivity relationship in African agriculture? Evidence from Rwanda (Policy Research Working Paper 6770). The World Bank Research Group.
- Ali, D. A., Deininger, K., & Duponchel, M. (2014). Credit constraints and agricultural productivity: Evidence from rural Rwanda. Journal of Development Studies, 50(5), 649–665.
- Ali, D. A., Deininger, K., & Goldstein, M. (2011). Environmental and gender impacts of land tenure regularization in Africa pilot evidence from Rwanda (Policy Research Paper 5765). The World Bank Research Group.
- Anderson, C. L., Reynolds, T., Merfeld, J. D., & Biscaye, P. (2017). Relating seasonal hunger and prevention and coping strategies: A panel analysis of Malawian farm households. The Journal of Development Studies, 54(10), 1–19.
- Ansoms, A., Verdoodt, A., & Van Ranst, E. (2008). The inverse relationship between farm size and productivity in rural Rwanda (Discussion Paper 2008.9). Antwerp: Institute of Development Policy and Management.
- Bigsten, A., & Tengstam, S. (2011). Smallholder diversification and income growth in Zambia. Journal of African Economies, 20(5), 781–822.
- Binswanger, H. P., & Rosenzweig, M. R. (1986). Behavioural and material determinants of production relations in agriculture. The Journal of Development Studies, 22(3), 503–539.
- Bizoza, A. R., & Havugimana, J. M. (2013). Land use consolidation in Rwanda: A case study of Nyanza district, Southern province. International Journal of Sustainable Land Use and Urban Planning, 1(1), 64–75.
- Blackwell, M., Iacus, S. M., King, G., & Porro, G. (2009). cem: Coarsened exact matching in Stata. The Stata Journal, 9, 524–546.
- Blarel, B., Hazell, P., Place, F., & Quiggin, J. (1992). The economics of farm fragmentation: Evidence from Ghana and Rwanda. The World Bank Economic Review, 6(2), 233–254.
- Boserup, E. (1965). The condition of agricultural growth. The economics of Agrarian change under population pressure. London: Allan and Urwin.
- Byiringiro, F., & Reardon, T. (1996). Farm productivity in Rwanda: Effects of farm size, erosion, and soil conservation investments. Agricultural Economics, 15(2), 127–136.
- Carletto, C., Savastano, S., & Zezza, A. (2013). Fact or artifact: The impact of measurement errors on the farm size–Productivity relationship. Journal of Development Economics, 103, 254–261.
- Cioffo, G. D., Ansoms, A., & Murison, J. (2016). Modernising agriculture through a ‘new’ Green Revolution: The limits of the crop intensification programme in Rwanda. Review of African Political Economy, 43(148), 277–293.
- Collier, P., & Dercon, S. (2014). African agriculture in 50 years: Smallholders in a rapidly changing world? World Development, 63, 92–101.
- Crecente, R., Alvarez, C., & Fra, U. (2002). Economic, social and environmental impact of land consolidation in Galicia. Land Use Policy, 19(2), 135–147.
- Deininger, K., & Feder, G. (2009). Land registration, governance, and development: Evidence and implications for policy. The World Bank Research Observer, 24, 233–266.
- Dercon, S., & Krishnan, P. (2000). Vulnerability, seasonality and poverty in Ethiopia. The Journal of Development Studies, 36(6), 25–53.
- Dethier, J. J., & Effenberger, A. (2012). Agriculture and development: A brief review of the literature. Economic Systems, 36(2), 175–205.
- Diao, X., Hazell, P., & Thurlow, J. (2010). The role of agriculture in African development. World Development, 38(10), 1375–1383.
- Dorward, A., Kydd, J., Morrison, J., & Urey, I. (2004). A policy agenda for pro-poor agricultural growth. World Development, 32(1), 73–89.
- Du, X., Zhang, X., & Jin, X. (2018). Assessing the effectiveness of land consolidation for improving agricultural productivity in China. Land Use Policy, 70, 360–367.
- Ellis, F. (1998). Household strategies and rural livelihood diversification. The Journal of Development Studies, 35(1), 1–38.
- Foster, A., & Rosenzweig, M. R. 2010. Barriers to farm profitability in India: Mechanization, scale, and credit markets (Working Paper). University of Pennsylvania, PA.
- Ho, D. E., Imai, K., King, G., & Stuart, E. A. (2007). Matching as nonparametric pre-processing for reducing model dependence in parametric causal inference. Political Analysis, 15, 199–236.
- Holden, S. T., & Otsuka, K. (2014). The roles of land tenure reforms and land markets in the context of population growth and land use intensification in Africa. Food Policy, 48, 88–97.
- Hsiao, C., Pesaran, M. H., & Tahmiscioglu, A. K. (2002). Maximum likelihood estimation of fixed effects dynamic panel data models covering short time periods. Journal of Econometrics, 109(1), 107–150.
- Iacus, S., King, G., & Porro, G. (2009). CEM: Software for coarsened exact matching. Journal of Statistical Software, 30(13), 1–27.
- Iacus, S. M., King, G., & Porro, G. (2012). Causal inference without balance checking: Coarsened exact matching. Political Analysis, 20(1), 1–24.
- Jayne, T. S., Mather, D., & Mghenyi, E. (2010). Principal challenges confronting smallholder agriculture in sub-Saharan Africa. World Development, 38(10), 1384–1398.
- Johnston, B. F., & Mellor, J. W. (1961). The role of agriculture in economic development. The American Economic Review, 51(4), 566–593.
- Kathiresan, A. (2012). Farm land use consolidation in Rwanda. Kigali, Rwanda: Republic of Rwanda, Ministry of Agriculture and Animal Resources.
- Lamb, R. L. (2003). Inverse productivity: Land quality, labor markets, and measurement error. Journal of Development Economics, 71(1), 71–95.
- Larson, D. F., Otsuka, K., Matsumoto, T., & Kilic, T. (2014). Should African rural development strategies depend on smallholder farms? An exploration of the inverse‐productivity hypothesis. Agricultural Economics, 45(3), 355–367.
- MINAGRI. (2009, February). Strategic plan for the transformation of agriculture in Rwanda (Phase II [PSTA II] final report). Kigali, Rwanda: Ministry of Agriculture and Animal Resources.
- Nilsson, P. (2018). Spatial spillovers and households’ involvement in the non-farm sector: Evidence from rural Rwanda. Regional Studies. Advance online publication. doi:10.1080/00343404.2018.1482415
- Niroula, G. S., & Thapa, G. B. (2005). Impacts and causes of land fragmentation, and lessons learned from land consolidation in South Asia. Land Use Policy, 22(4), 358–372.
- NISR. (2016). Poverty trend analysis report 2010/11-2013/14. Kigali: National Institute of Statistics of Rwanda.
- Pašakarnis, G., & Maliene, V. (2010). Towards sustainable rural development in Central and Eastern Europe: Applying land consolidation. Land Use Policy, 27(2), 545–549.
- Pritchard, M. F. (2013). Land, power and peace: Tenure formalization, agricultural reform, and livelihood insecurity in rural Rwanda. Land Use Policy, 30(1), 186–196.
- Rosegrant, M. W., & Cline, S. A. (2003). Global food security: Challenges and policies. Science, 302(5652), 1917–1919.
- Ruthenberg, H. (1980). Farming systems in the tropics (No. Ed. 3). Oxford: Oxford University Press.
- Sen, A. K. (1966). Labour allocation in a cooperative enterprise. The Review of Economic Studies, 33(4), 361–371.
- Sheng, Y., Zhao, S., Nossal, K., & Zhang, D. (2015). Productivity and farm size in Australian agriculture: Reinvestigating the returns to scale. Australian Journal of Agricultural and Resource Economics, 59, 16–38.
- Tan, S., Heerink, N., & Qu, F. (2006). Land fragmentation and its driving forces in China. Land Use Policy, 23(3), 272–285.
- Tilman, D., Cassman, K. G., Matson, P. A., Naylor, R., & Polasky, S. (2002). Agricultural sustainability and intensive production practices. Nature, 418(6898), 671–677.
- Timmer, C. P. (2002). Agriculture and economic development. Handbook of Agricultural Economics, 2, 1487–1546.
- USAID. 2007. Agricultural land use consolidation and alternative farming models: Programs and projects implementation strategy. Kigali Rwanda. Retrieved from https://www.land-links.org/wp-content/uploads/2016/09/USAID_Land_Tenure_Rwanda_Land_Use_Implementation_Strategy.pdf
- van Dijk, T. (2003). Scenarios of Central European land fragmentation. Land Use Policy, 20(2), 149–158.
- Wu, Z., Liu, M., & Davis, J. (2005). Land consolidation and productivity in Chinese household crop production. China Economic Review, 16(1), 28–49.
- Zhu, X., Demeter, R. M., & Lansink, A. O. (2012). Technical efficiency and productivity differentials of dairy farms in three EU countries: The role of CAP subsidies. Agricultural Economics Review, 13(1), 66–92.