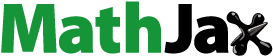
ABSTRACT
With the growing popularity of intensive longitudinal research, the modeling techniques and software options for such data are also expanding rapidly. Here we use dynamic multilevel modeling, as it is incorporated in the new dynamic structural equation modeling (DSEM) toolbox in Mplus, to analyze the affective data from the COGITO study. These data consist of two samples of over 100 individuals each who were measured for about 100 days. We use composite scores of positive and negative affect and apply a multilevel vector autoregressive model to allow for individual differences in means, autoregressions, and cross-lagged effects. Then we extend the model to include random residual variances and covariance, and finally we investigate whether prior depression affects later depression scores through the random effects of the daily diary measures. We end with discussing several urgent—but mostly unresolved—issues in the area of dynamic multilevel modeling.
The COGITO study, which took place a decade ago, is an impressive project that reflects an admirable determination on part of its researchers as well as the participants. It consists of a younger and an older sample of over 100 individuals each, who visited the laboratory on about 100 days to perform a battery of cognitive tests and fill out self-report questionnaires. This endeavor is all the more impressive if one realizes that at the time, gathering intensive longitudinal data was not only cumbersome, but it was also considered unnecessary by many, because short-term, within-person fluctuations were assumed to reflect mere noise. Since then, however, the tide has turned: first, technological developments like smart phones and wearable devices have led to new forms of data collection—such as ambulatory assessments, experience sampling, ecological momentary assessments, and electronic daily diaries (Conner, Tennen, Fleeson, & Feldman Barrett, Citation2009; Trull & Ebner-Priemer, Citation2013)—that have placed intensive longitudinal data within reach of mainstream psychology; and second, the growing body of research made possible by these innovations now forms a convincing testimony of the meaningfulness of short-term, within-person fluctuations.
While research like the COGITO study has many favorable features, one of the most valuable properties of intensive longitudinal data is that they provide a unique opportunity to study processes within-person as they unfold over time (Bolger & Laurenceau, Citation2013; Hamaker & Wichers, Citation2017; Walls & Schafer, Citation2006). To investigate the underlying dynamics of intensive longitudinal data, researchers have been borrowing techniques from other disciplines—like econometrics, physics, and engineering—were they have a long history of studying processes over time using time series analysis and dynamic systems theory. A common characteristic of these techniques is their focus on the way a preceding state of the system (e.g., person or dyad) gives rise to the subsequent state. This allows for a unique perspective on processes (see Bisconti, Bergeman, & Boker, Citation2004; Chow, Nesselroade, Shifren, & McArdle, Citation2004; Chow, Ram, Boker, Fujita, & Clore, Citation2005; Hamaker, Dolan, & Molenaar, Citation2002, Citation2003; Molenaar, Citation1985; Molenaar, Citation1987; Ram et al., Citation2005; Rovine & Walls, Citation2006; Sadler, Ethier, Gunn, Duong, & Woody, Citation2009), and extends our more conventional approaches to intensive longitudinal data, which tend to focus on concurrent relationships between variables, rather than their dynamic interplay over time.
However, in contrast to the practice in many other disciplines where longitudinal data typically come from a single case, a fundamental challenge in psychology and other social sciences is how to combine and compare the intensive longitudinal data of multiple individuals. We can distinguish between a bottom-up and a top-down approach in this context (see Liu, Citation2017, for a first comparison between these two).
In the bottom-up approach, sometimes referred to as replicated time series analyses, the data are first analyzed per person, and subsequently similarities between the dynamics of different individuals are sought. This can be done by the researchers themselves through constraining parameters across individuals (see Hamaker et al., Citation2003), for instance when using software like the R-packages OpenMx (Boker et al., Citation2011) and dynR (Ou, Hunter, & Chow, Citation2018). Alternatively, the R-package Group Iterative Multiple Model Estimation (GIMME; Beltz, Wright, Sprague, & Molenaar, Citation2016; Gates & Molenaar, Citation2012) can be used to automatically search for such similarities. This bottom-up approach can be considered a purely idiographic research form that allows for a maximum degree of idiosyncracies in the results.
In contrast, the top-down approach, also referred to as dynamic multilevel modeling, is based on choosing a particular time series model or differential equation to describe the variability within an individual at level 1, while allowing for quantitative differences at level 2 in the parameters that govern these dynamics. This approach can be considered as somewhere in between idiographic and nomothetic research (see Conner et al., Citation2009): While it is assumed that the functional form of the level 1 model is the same for individuals, there is ample room for quantitative differences between individuals in the parameters of this function. Researchers have been applying this approach using Bayesian software like JAGS (Plummer, Citation2003) and WinBUGS (Spiegelhalter, Thomas, Best, & Lunn, Citation2003; e.g., Wang, Hamaker, & Bergman, Citation2012), but there are also specialized programs that were developed for this purpose. These include the Bayesian Ornstein-Uhlenbeck Model (BOUM) toolbox package (Oravecz, Tuerlinckx, & Vandekerckhove, Citation2016), the R-package mlVAR (Epskamp, Deserno, & Bringmann, Citation2017), and the R-package ctsem (Driver, Oud, & Voelkle, Citation2017), which in turn uses either OpenMx (Boker et al., Citation2011) or Stan (Stan Development Team, Citation2017).
In the current study we take a dynamic multilevel modeling approach to analyze the affective ratings obtained as part of the COGITO study. Specifically, we will show how various modeling innovations that have been proposed and developed in recent years—including standardization of cross-lagged parameters in dynamic multilevel models, random residual variances, and indirect effects through random effects—can now be easily combined and extended when using the new dynamic structural equation modeling (DSEM) module that is implemented in Mplus (see Asparouhov, Hamaker, & Muthén, Citation2017, Citation2018). In doing so, we aim to illustrate the unique opportunities offered by dynamic multilevel modeling for studying the dynamics of processes, while also outlining the many unresolved issues in this emerging field.
This paper is organized as follows. First, we explain how intensive longitudinal data can be decomposed into different sources of variance, which is fundamental to dynamic multilevel modeling, and we illustrate this with the COGITO data. Second, we discuss some of the basics of DSEM in Mplus, which are essential for the dynamic multilevel models we use in the current study. This is followed by three analyses on the COGITO affective data, consisting of increasingly more advanced dynamic multilevel models, that is: (a) a bivariate multilevel cross-lagged model that allows for individual differences in means, autoregressive relationships, and cross-lagged relationships; (b) an extension of this model with random innovation variances and covariance, to account for individual differences in these model features; and (c) an extension that includes a predictor at level 2, which has indirect effects through the random effects of the affect dynamics on an outcome variable at level 2. In these analyses, we make the following assumptions: (a) the data are normally distributed, both within persons and between persons; (b) there are no trends or cycles over time in these data; and (c) missing data are missing at random. Clearly, these are strong assumptions that should be further investigated in practice; however, practical solutions in case these assumptions are violated are not always straightforward and/or readily available. We discuss these and other urgent—yet unresolved—issues that come up while analyzing the COGITO data at the end of this paper.
1. Total, cross-sectional, within-person, and between-person variability
There is quite a large body of literature regarding the actual meaningfulness of cross-sectional research for gaining knowledge about processes that operate within individuals (e.g., Borsboom, Mellenbergh, & Van Heerden, Citation2003; Cattell, Citation1952; Grice, Citation2004; Hamaker, Citation2012; Hamaker, Schuurman, & Zijlmans, Citation2017; Lamiell, Citation1998; Molenaar, Citation2004; Molenaar, Huizenga, & Nesselroade, Citation2003; Nesselroade, Citation2002; Schmitz & Skinner, Citation1993; Voelkle, Brose, Schmiedek, & Lindenberger, Citation2014). This topic is already complex in itself, but the discussion is further complicated by the fact that researchers sometimes use the same terms to refer to different phenomena. Therefore, we begin by briefly explaining our terminology and how this relates to the conventions in different strands of literature. Once this is in place, we decomposition the affective measurements from the COGITO study.
1.1. Terminology
While the terms within-person and intraindividual variability unambiguously refer to the variability within a person over time and/or situations, their linguistic counterparts between-person and interindividual variability have been used to refer to two distinct forms of variation: At times, they are used to refer to stable, trait-like variation between persons (Hamaker, Nesselroade, & Molenaar, Citation2007; Wang & Maxwell, Citation2015), and at other times they are used to refer to cross-sectional variation, that is, the variability across persons at a single occasion (Borsboom et al., Citation2003; Hamaker, Dolan, & Molenaar, Citation2005; Molenaar, Citation2004; Voelkle et al., Citation2014). This ambiguous usage of the terms between-person and interindividual may have led some researchers to erroneously conclude that there is no difference between trait-like variation and cross-sectional variation, and as a result, cross-sectional variation may easily be mistaken as the counterpart of intraindividual or within-person variation.
In the current paper, we will use these terms in accordance to the multilevel literature, such that between-person and interindividual are the complement of within-person and intraindividual. This implies that cross-sectional variability is the result of both within-person and between-person variability (see Hamaker et al., Citation2017; Hamaker et al., Citation2007; Schmitz, Citation2000). Finally, we will use the term total variability to refer to the variability both across individuals and across time points. The latter is thus also the result of within-person and between-person variability, but in contrast to cross-sectional variability, which is restricted to a single time point, the total variability can also contain variability over time.
1.2. Decomposing the COGITO affective data
We consider within, between, and total variability using the composite scores obtained from the positive affect negative affect schedule (PANAS; Watson, Clark, & Tellegen, Citation1988), which the COGITO participants filled out at each measurement occasion (Brose, Voelkle, et al., Citation2015). The PANAS consists of 10 items that measure positive affect (PA) and 10 items that measure negative affect (NA). We analyze the data of the younger sample (aged 20–31), consisting of 101 individuals, and the older sample (aged 65–80), which consists of 103 individuals, separately.Footnote1
First, we estimate the total variances for PA and NA and the corresponding total correlation based on all the data in the sample, both across persons and time. Second, the within-person and between-person results are obtained using a basic multivariate multilevel model (sometimes referred to as the empty model, as it does not include any predictors): It decomposes the total variance into two parts, that is, the between part, which is formed by the within-person means (sometimes referred to as cluster or group means), and the within-part, which are formed by the within-person fluctuations over time around the within-person means. Let PAit and NAit be the PA and NA scores of individual i at time t. The decomposition into the within-person and between-person parts is
or in matrix notation
(1)
(1) where the μ’s are the within-person means that form the between-person part of the model, and PA(W)it and NA(W)it represent the temporal deviations of individual i at occasion t from these within-person means.
In , the variance estimates are plotted along with bars for the standard errors. The intraclass correlation (ICC) for each variable in each group is also reported: It is computed as the ratio of between-person variance to total variance, where the latter is the sum of within-person and between-person variance. Hence, the ICC indicates the proportion of the total variance that is accounted for by stable, trait-like between-person differences. shows that: (a) the between-person variance is larger than the within-person variance for both PA and NA in both groups (ICCs lie between 0.62 and 0.89); (b) the variance of PA is larger than that of NA in both groups and this is true for the total, the between, and the within variances; and (c) the older sample is characterized by larger ICCs, and also by larger differences between the variability in PA and NA than the younger sample.
Figure 1. Variance estimates for the younger and older samples, with the corresponding standard errors, including: (1) total variance (across time and persons); (2) between-person variance (i.e., variance in within-person means across people); and (3) within-person variance (i.e., variance within people across time). For each variable in each group, we also included the intraclass correlation (ICC), which represents the proportion of total variance that is account for by the stable between-person differences.
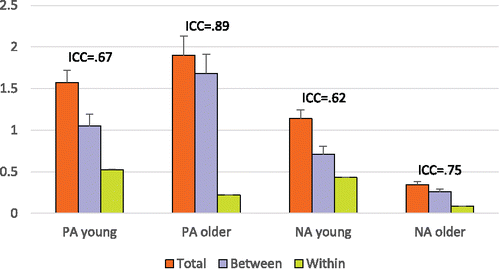
The correlations between PA and NA also differed across the between-person and within-person levels. At the between-person level the correlation between PA and NA did not differ significantly from zero in either sample, whereas at the within-person level there was a negative correlation in both samples (, SE = 0.01, p < 0.001 in the younger sample, and
, SE = 0.01, p < 0.001 in the older sample). These results can be understood in the following way (see also Brose, Voelkle, Lövdén, Lindenberger, & Schmiedek, Citation2015). The traits PA and NA, which are captured by the between-person part of the model, are not correlated in these populations, which implies that sampling an individual who is high on one of these traits and low on the other is about equally likely as sampling an individual who is high on both traits, or low on both traits. In contrast, it is not that common to experience both elevated levels of PA and NA at the same time, because repeated measures of PA and NA are typically negatively correlated within a person. In the current data set, the within-person variability refers to day-to-day fluctuations, rather than moment-to-moment fluctuations; therefore, the correlation of −0.19 in both samples suggests that individuals tend to experience good days with elevated PA and decreased NA, and bad days with elevated NA and decreased PA instead, although this pattern is not as strong as in momentary measurements (when individuals are asked how they are feeling right now).
In sum, these results show that there are marked differences between the within-person and the between-person relationships, and that neither is represented well by the results for the total. This also confirms the concern that has been expressed by many researchers before: Cross-sectional results are a mix of within-person and between-person relationships, and may not represent either very well (see Brose, Schmiedek, & Lindenberger, Citation2013; Schmitz, Citation2000).Footnote2 This decomposition of the total variance into a within-person and a between-person part is fundamental to dynamic multilevel modeling.
2. DSEM in a nutshell
To model the dynamics of these data, we make use of DSEM in Mplus. DSEM can be thought of as a combination of two time series techniques, that is: (a) state-space modeling, which is a general framework used in estimating times series models (e.g., Durbin & Koopman, Citation2012; Harvey, Citation1989); and (b) dynamic factor analysis, which is a factor analytic approach to time series data (e.g., Molenaar, Citation1985; Zhang, Hamaker, & Nesselroade, Citation2008). But where these time series techniques are traditionally concerned with N = 1 data (i.e., data from a single case; see Box, Jenkins, Reinsel, & Ljung, Citation2016; Chatfield, Citation2004; Hamilton, Citation1994; Kim & Nelson, Citation1999; Shumway & Stoffer, Citation2017), DSEM encompasses both N = 1 time series analysis and multilevel extensions of this (Asparouhov et al., Citation2017, Citation2018).
In this section, we present some of the basics of DSEM that are relevant to the analyses of the COGITO affective data that we present afterward. We begin by discussing the general model structure, consisting of a model at the within-person level, and a model at the between-person level. Subsequently, we discuss three key issues, that is: the estimation used in DSEM, how missing data are handled, and how we can account for unequal intervals between observations.
2.1. The model
If we have longitudinal multilevel data, this implies that there is more than one person, dyad, or other unit, from which repeated measures have been obtained. If we want to analyze these data using DSEM in Mplus, the data should be in long format, which means that the repeated measures of a person should form separate rows in the data file, and there should be a cluster variable to indicate which rows belong to the same case.
Multilevel DSEM is based on decomposing the data into a within-person part and a between-person part, and modeling each of these parts with their own model. Let yit be the vector with p observed variables for individual i at time t. This is decomposed into a within-person vector with means μi, and a time-specific within-person vector with deviation from these mean y(W)it, where the superscript (W) stands for “within,” Hence, the decomposition can be expressed as
(2)
(2) similar to what was shown for the COGITO affect measures in Equation (Equation1
(1)
(1) ).
The temporal within-person deviations y(W)it are modeled with the within-person level model, which consists of a measurement equation that can relate observed variables to latent variables, and a structural equation in which latent variables can be related to each other. But in addition to such standard structural equation modeling properties, the within-person level model also allows for lagged relationships between variables, that is, regression paths between variables at different time points. These lagged relationships can be between observed variables, between latent variables, or a combination of these. Moreover, factor loadings, regression coefficients, and variances are allowed to be random, meaning that these parameters can take on different values for different individuals. These random effects thus allow for individual differences in, for instance, lagged relationships or residual variances, as we will show later on.
The random effects from the within-person level model and the random means from the decomposition automatically end up as latent variables in the between-person level model. Here they can be further modeled together with observed time-invariant (i.e., level 2 or between level) variables that have been included in the analysis. The between-person level model also consists of a measurement equation and a structural equation, allowing for the definition of factor models, path models, or a combination of these two based on all between-person variables.
2.2. Estimation in DSEM
DSEM as it is implemented in Mplus version 8 is based on Bayesian estimation. This makes it possible to have a large number of random effects, which would be intractable in a frequentist framework. Bayesian estimation is based on combining the likelihood of the data with prior distributions for the unknown model parameters to obtain posterior distributions for these unknown parameters (Gelman et al., Citation2014; Lynch, Citation2007). Typically, estimation consists of an iterative process in which parameters are sampled from conditional distributions according to a Markov chain Monte Carlo (MCMC) procedure (Gelman et al., Citation2014). The priors can be used to incorporate prior knowledge or subjective expectations into the model, but one can also specify flat, diffuse priors that contain little prior information; in the latter case, the results are asymptotically equivalent to maximum likelihood estimation. Mplus allows the user to specify the priors, but it also provides default priors that are diffuse so that the data dominate the results (Asparouhov & Muthén, Citation2010; Gelman et al., Citation2014); this is the option we will use here.
When using an MCMC algorithm to estimate parameters, we have to decide on the number of iterations. In Mplus we can either specify this number, or use the potential scale reduction (PSR) criterion, which is computed for each model parameter separately by dividing the total variability across two chains of the MCMC algorithm, by the variance within a chain (Asparouhov & Muthén, Citation2010; Gelman et al., Citation2014). This quantity should be very close to one, as the between-chain variance should be close to zero, such that the total variance across two chains becomes (almost) identical to the within-chain variance if enough iterations are used. Furthermore, it is considered good practice in Bayesian estimation to check the trace plots of each parameter, to see whether there are signs of nonconvergence.
In the end, a burn-in period is discarded to erase the influence of starting values used by the MCMC algorithm. The remaining MCMC samples are considered an empirical approximation of the posterior distribution of the parameters. Point estimates are obtained by taking the mean or median for each parameter. In addition, credible intervals (CI) are obtained based on the posterior distributions through finding the lower and upper cut-off values of a particular interval (e.g., the 95% CI). Hence, these intervals are not based on the assumption that each parameter has a normal or even symmetric distribution. Moreover, it is easy in Bayesian analysis to compute additional quantities of interest based on model parameters (e.g., indirect effects or standardized parameters), and obtain a CI for this, as the quantity can be computed at each iteration of the MCMC algorithm, thus providing a posterior distribution for the quantity.
2.3. Missing data
Missing data are very common in intensive longitudinal data; how we handle these may have serious consequences for the quality of our results. In Bayesian analyses, missing data are typically treated in the same way as random effects and model parameters, which implies that at each iteration of the MCMC algorithm, missing data are sampled from their conditional posterior. This conditional distribution takes the autocorrelation structure of the individual’s data into account. For instance, if one is estimating a first-order autoregressive process and there is a missing value for individual i at occasion t, the conditional posterior of this missing value will depend on: (a) the neighboring observations (i.e., at occasions t − 1 and t + 1); (b) the individual’s autoregressive parameter at the current iteration of the MCMC algorithm; and (c) the uncertainty that is expressed by the residual variance (to be discussed in more detail below). This approach is similar to the way that missing data in single-subject time series analysis are handled with a Kalman smoother based on the state-space model (see Särkkä, Citation2013), and it guarantees consistent estimation as long as the missing data are missing at random.
Clearly, when there is a structural reason for certain missingness—such as no measurement during weekends as in the COGITO study, or no measurements during the night in experience sampling—it may be questionable whether this amounts to missing at random. We will elaborate on this in the discussion.
2.4. Unequal time intervals between observations
A particular challenge when dealing with intensive longitudinal data is unequal intervals between observations. These may result from missing observations, for instance, when we have daily measurements but participants sometimes forget to fill out the end-of-day questionnaire. However, nonequidistant observations may also be a part of the research design: In experience sampling and ecological momentary assessments, participants are prompted at random time points during the day to ensure they are caught in their daily life rather than that they prepare for the next measurement. In the COGITO data, the majority of observations were obtained with an interval of 1 day, but there were also larger intervals, for instance because people missed a day, or because there were no measurements taken on the weekend or during holidays.Footnote3
Unequal intervals are a concern when the interest is in lagged relationships, because the strength of a lagged effect depends on the size of the time interval between the measurements (see Gollob & Reichardt, Citation1987). An elegant way of dealing with this issue is the use of continuous time models based on differential equations (Deboeck & Preacher, Citation2015; Voelkle, Oud, Davidov, & Schmidt, Citation2012), which we elaborate on in the discussion. In contrast, in discrete time models we can account for different intervals by adding missing values between subsequent observations that are made further apart in time; in doing so we create a data set in which the cases are approximately equidistant.
Heuristically, it implies that we may have a continuous time dimension with observations located on this dimension at unequal intervals. By choosing a grid, this continuous time dimension is divided into segments of the length we specified; a smaller grid results in shorter segments. These segments are numbered 1, 2, 3, et cetera, and they either contain an observation or are assigned a missing value. Note that the actual observations could have been taken at any time within the segment they fall into, which is why the approach results in approximately equally spaced observations. Haan-Rietdijk, Voelkle, Keijsers, and Hamaker (Citation2017) found that such an approach leads to very similar results as using a modeling approach that treats time as truly continuous such that different intervals between observations are naturally accounted for. Asparouhov et al. (Citation2018) also include a simulation study that shows that choosing a finer grid, such that there is a better agreement with observed times, results in good estimates despite inserting a large amount (e.g., more than 80%) of missing data.
In the current example, a logical choice is a grid based on intervals of 1 day, which implies that if there is an interval between two consecutive measurements of more than 1 day, missing values are inserted (i.e., as many as there are missing days). However, for other forms of data, such as experience sampling, the choice is less obvious, as we discuss at the end of this paper.
3. Model 1: A multilevel VAR(1) model for PA and NA
We begin by considering a multilevel model based on a first-order vector autoregressive (VAR(1)) model, which is represented in . The VAR(1) model is one of the most basic time series models (for N = 1 data), and consists of regressing a vector with observations at occasion t on the vector of observations at the preceding occasion. Multilevel extensions of this basic time series model have emerged in the psychological literature, allowing for the analysis of multivariate time series of multiple individuals simultaneously (see Bringmann et al., Citation2013; Schuurman, Ferrer, Boer-Sonnenschein, & Hamaker, Citation2016). Another way to view this model is as a multilevel extension of a cross-lagged panel model, allowing for individual differences in means and in lagged effects.
Figure 2. Representation of the multilevel VAR(1) model. Left part contains the decomposition into within-person (time-varying) and between-person (time-invariant) components. Top right contains the within-person level model, which is a VAR(1) model. Bottom right contains the between-person level model, which includes the between-person components from the decomposition, as well as all the random effects of the model, corresponding to the solid black circles in the within-person level model.
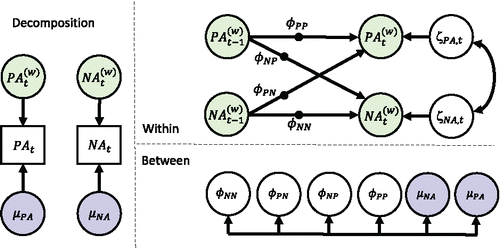
Fundamental to the use of dynamic multilevel models is that the data are decomposed into a within-person and a between-person component, as shown in Equation (Equation1(1)
(1) ). This decomposition is also shown in the left part of . The within-person means μPA, i and μNA, i can be thought of as a person’s long-run equilibria for the variables PA and NA, respectively. Such equilibria have also been interpreted as a person’s trait scores, or—if we are taking a dynamic system’s perspective—as the attractor of the system to which an individual returns in the absence of external perturbations. The temporal deviations from these within-person means, PA(W)it and NA(W)it, can be thought of as the affective states of a person at occasion t.
We discuss the model specification of both parts below. Then we discuss the priors that are used in this model. Subsequently we discuss the results from these analyses for both samples.
3.1. Within-person level model
The within-person deviations PA(W)it and NA(W)it are regressed on themselves and each other at the preceding occasion, PA(W)it − 1 and NA(W)it − 1, as represented in the top right part of . Hence, the level 1 or within-person equations can be expressed as
or (using the general matrix notation yit = Φiyit − 1 + ζit) as
(3)
(3) where φPP, i and φNN, i are the autoregressive parameters for PA and NA, respectively; φPN, i is the cross-lagged regression effect from NA to PA at the next occasion; and φNP, i is the cross-lagged regression coefficient from PA to NA at the next occasion.
The ζ’s represent the residuals, often referred to as innovations, or alternatively as random shocks, disturbances, system noise, or dynamic errors. The latter term is to distinguish it from measurement error, which tends to affect the measurements only at one occasion; in contrast, dynamic error tends to change the actual course of the system, because it also affects future measurements through the lagged relationships (Schuurman, Houtveen, & Hamaker, Citation2015). By definition, these innovations have a mean of zero both within and between individuals. They are assumed to come from a multivariate normal distribution, that is,
(4)
(4) Hence, at the within-person level, we estimate two variances and a covariances for the innovations.
All the lagged parameters in Equation (Equation3(3)
(3) ) have a subject index i to indicate that individuals may differ with respect to the magnitude of these parameters. The autoregressive parameter indicates how quickly a person restores equilibrium after being perturbed. For this reason, this parameter has also been referred to as inertia,Footnote4 carryover, and regulatory weakness. Typically, its value lies between 0 and 1, although negative values may also occur. The further away from zero this parameter is, the longer it takes a person to return to his/her equilibrium. Individual differences in autoregressive parameters have been an important topic in empirical research. For instance, inertia in affect has been shown to be positively related to person characteristics, such as current and future depression, being female, and neuroticism (see Brose, Voelkle, et al., Citation2015; Koval, Kuppens, Allen, & Sheeber, Citation2012; Kuppens, Allen, & Sheeber, Citation2010; Suls et al., Citation1998). Others have investigated individual differences in autoregression in daily drinking behavior (Rovine & Walls, Citation2006).
The cross-lagged parameters reflect predictive relationships, and are especially interesting because they potentially represent causal mechanisms. For this reason, they are sometimes referred to as spill-over, as they may represent the cascade effect of functioning or behavior in one domain into another domain (Almeida, Wethington, & Chandler, Citation1999; Bolger, DeLongis, Kessler, & Wethington, Citation1989; Masten & Cichetti, Citation2010). However, there are two major concerns we should be aware of when considering cross-lagged parameters as evidence for causal mechanisms. First, it is very likely that there are omitted variables that vary over time and that affect both variables; the relationships found with a particular model are likely to change when such an omitted variable is included in the analysis. Second, lagged effects are specific to a particular interval between the observations, which is known as the “lag-problem” (Gollob & Reichardt, Citation1987); hence, a cross-lagged parameter should never be considered “the” effect of one variable on another, but merely the effect at a particular interval (see Deboeck & Preacher, Citation2015). We elaborate on both concerns in the discussion.
3.2. Between-person level model
While the initial decomposition in Equation (Equation1(1)
(1) ) suggests that at the between-person level we have two variables—that is, the within-person means for PA and NA (i.e., μPA, i and μNA, i)—closer inspection of Equation (Equation3
(3)
(3) ) shows that we have four additional random effects, that is: two random autoregressive parameters (φPP, i and φNN, i), and two random cross-lagged regression parameters (φPN, i and φNP, i). If we simply consider these to be related to each other without any particular constraint, we have
(5)
(5) where the six γ’s represent the fixed or averaged effects, and the six u’s represent the individual deviations from these means. These individual deviations are assumed to come from a multivariate normal distribution, that is,
(6)
(6) where Ω is a 6 × 6 covariance matrix. Hence, there are 6 variances (i.e., the random effects), 15 covariances, and 6 fixed effects, resulting in 27 parameters at the between-person level. Combined with the three parameters estimated at the within-person level, we now have a total of 30 model parameters for this bivariate multilevel VAR(1) model.
3.3. Priors
All unknown parameters need to be given a prior distribution in Bayesian estimation. Here, we make use of the defaults included in Mplus.Footnote5 For means and intercepts, the default is a univariate prior with mean zero and variance equal to 1010. The default diffuse prior that is used in Mplus for covariance matrices is an inverse Wishart with a zero matrix for scaling and degrees of freedom equal to minus the number of variables minus 1.
For the current model, we thus have the following priors. For the covariance matrix of the innovations at the within-person level ,we use an inverse Wishart distribution, that is,
(7)
(7) For the fixed effects (or average random effects, we use univariate normal distributions, that is,
(8)
(8) and for the covariance matrix of the random effects at the between-person level, we use an inverse Wishart, that is,
(9)
(9)
In addition to priors for these model parameters, there are also priors needed for the missing observations, and the individual parameters (i.e., the uji’s). The latter is necessary because the random effects are not integrated out.
3.4. Results
We used 50,000 iterations and two chains. Because the first half of each chain is discarded as burn-in, and because we used a thinning of 10 iterations (meaning that only 1 in 10 iterations is saved), our results here are based on 5000 iterations (see Gelman et al., Citation2014). Model convergence is checked using the proportional scale reduction and by checking the trace plots for an absence of trends, spikes, or other irregularities. The parameter estimates for the fixed and random parameters, and their 95% credible intervals (CI) are presented in the left part of .
Table 1. Point estimates (posterior means) and 95% credible intervals for fixed effects (i.e., means) and random effects (i.e., variances) in both groups.
Only the CIs for the fixed effect φNP, i in the older sample contains zero, indicating that there is no evidence that there is an effect of PA on NA over time on average in this group. This does not necessarily imply that this is not an important parameter in the model: As it is included as a random parameter, there may be meaningful individual differences that are centered around zero. In this case, however, the variance for this parameter also seems rather small in this group, implying that the individual φNP, i’s are all close to zero.Footnote6
To interpret the magnitude of the cross-lagged effects, we consider the standardized results that Mplus provides, which are based on standardizing the parameters per person first, and then taking the average of these. This approach was proposed in Schuurman et al. (Citation2016) and takes into account that individuals have different cross-lagged parameter values, and that they may have different variances.Footnote7 The latter stems from the fact that the variance of a variable in the VAR(1) model is a function of the lagged parameters, which are allowed to differ across individuals. The average individually standardized lagged parameters prove to be all rather small: The average standardized effect from NA to PA is 0.034 (CI = [0.008, 0.059]) in the younger sample and 0.089 (CI = [0.059, 0.119]) in the older sample; the average standardized effect from PA to NA is 0.038 (CI = [0.017, 0.059] in the younger sample, and 0.026 (CI = [0.001, 0.051]) in the older sample. Note that for the latter effect, the corresponding fixed effect had a CI that contained zero. This seemingly contradictory result stems from the fact that the averaged individually standardized parameter always has a smaller CI than the corresponding model parameter, which reflects the kind of inferences that can be based on them: The averaged individually standardized parameter allows for inferences conditional on the individuals in the sample (i.e., other observations within the same clusters), whereas the conventional model parameters allow for inferences to other individuals from the population. In this case, the lower bound of the CI of the average standardized effect is very close to zero, so we do not have to consider this an important contradiction.
Related to the average individually standardized regression coefficients, the standardized results also include the averaged within-person proportion of explained variance: This is 0.18 (CI = [0.17, 0.20]) for PA and 0.21 (CI = [0.19, 0.23]) for NA in the younger sample, and 0.26 (CI = [0.24, 0.28]) for PA, and 0.16 (CI = [0.14, 0.17]) for NA in the older sample. Furthermore, the standardized results also reveal that the average within-person correlation between the innovations is −0.19 (CI = [−0.21, −0.17]) in the younger sample, and −0.27 (CI = [−0.29, −0.25]) in the older sample.
In addition to these averaged within-person standardized results, the standardized results also include the between-person level correlations between the six random effects included in the models. To represent these for both groups in a convenient and concise way, we make use of the function qgraph in R (Epskamp, Cramer, Waldorp, Schmittmann, & Borsboom, Citation2012). The resulting visual representations included in the upper part of contain the correlation estimates that have credible intervals that do not include zero. Red connections represent positive (warm) correlations, and blue connections represent negative (cold) correlations; the strength of these correlations is represented by the thickness of the connections. The correlations in the younger sample ranged from 0.22 to 0.46 (in absolute values), and in the older sample they ranged from 0.25 to 0.49 (in absolute values).
Figure 3. Visual representations of the correlation structure of the random effects of the multilevel VAR(1) models in the two samples. Upper part contains the correlations between the six random effects in model 1, while the bottom part contains the correlations between the nine random effects of model 2. Only correlations whose 95% credible interval did not include zero are included here. Red connections represent positive correlations, blue connections represent negative correlations. Strength of the correlations is represented by the thickness of the connection.
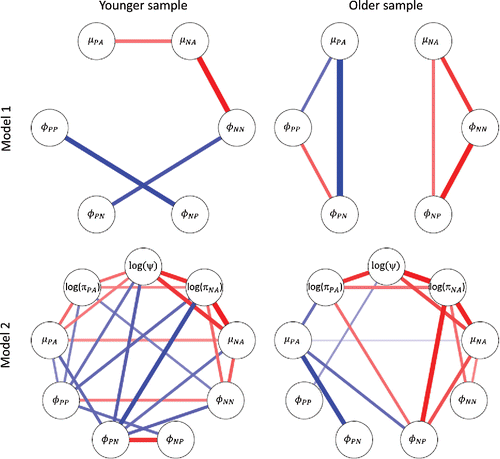
These representations show that, while there are equally strong and many correlations in both samples, the patterns are very different. The two negative relationships in the correlation structure of the younger sample indicate that individuals with a higher autoregressive parameter for PA tend to have lower cross-lagged parameters from PA to NA, and individuals with a higher autoregressive parameter for NA tend to have lower cross-lagged parameters from NA to PA. The positive correlation between the autoregressive parameter for NA and the mean of NA indicates that individuals with a higher trait level for NA also tend to have more carry-over in their NA from 1 day to the next. Finally, the positive connection between the two means of PA and NA runs counter to the results we obtained before in the decomposition, where the between-person correlations were close to zero. The currently positive correlation implies that individuals who have a higher mean on PA also tend to have a higher mean on NA; note however that this relationship is quite weak, and further inspection of the corresponding CI indicated that the lower bound was quite close to zero.
In contrast, in the older sample we see the correlation structure consists of two separate parts: One part connecting the parameters associated with PA, including the mean μPA, i, and the lagged regression coefficients φPP, i and φPN, i (on the left side), and another part connecting the parameters associated with NA, including the mean μNA, i, and the lagged regression coefficients φNN, i and φNP, i (on the right side). Higher mean PA is associated with less carry-over in PA, and a smaller effect from NA on PA. This pattern seems to suggest that high levels of PA are not resulting from the maintenance of PA, but that they may emerge from the ability to shield NA from spill-over into PA. In contrast, higher mean NA is associated with more carry-over in NA, and a stronger effect from PA to NA. Finally, the autoregressive parameter is positively correlated to the cross-lagged parameter in the same part, meaning that carry-over and spill-over are positively related.
4. Model 2: Extending the multilevel VAR(1) model with random innovation variances and covariance
In the model presented above, we considered random means and random lagged parameters. In contrast, the innovation variances and their covariance were fixed to be equal across individuals and estimated at the within-person level (see Equation (Equation4(4)
(4) )). While typically within-person (or level 1) residuals are not given much thought in multilevel modeling, they are actually of great interest here: They contain everything that was not measured explicitly, but that affects the course of the variables that were observed, including social interactions, weather conditions, demands at work or at home, and physiological factors such as sugar or caffeine intake, hormones, and health.
Here, we begin by providing a brief rationale for random innovation variances and covariance. Then we discuss how to adjust the previous model to include random innovation variances and covariance. Subsequently, we discuss the priors for this adjusted model. Finally, we present the results for these analyses for both samples.
4.1. Rationale for individual differences in innovation variances and covariance
As Jongerling, Laurenceau, and Hamaker (Citation2015) argued, we may expect individual differences in the variance of this collective term, due to two kinds of differences between individuals. First, there may be individual differences in reactivity to unobserved influences. For instance, there are a number of studies that show that individuals who are depressed are characterized by larger increases in negative affect in response to external negative events than nondepressed individuals, a phenomenon that is referred to as stress sensitivity (Wichers et al., Citation2009). Similarly, nondepressed individuals are characterized by a stronger increase in positive affect in response to a positive event in comparison to depressed individuals, which is referred to as the reward experience (Wichers et al., Citation2009). Second, there may be individual differences in variability of unobserved influences. That is, some people lead more constant lives than others, and this difference in exposure to variability in external factors may also be captured by individual differences in the innovation variance.
While Jongerling et al. (Citation2015) considered a univariate model and thus only needed a random innovation variance to account for individual differences in this part of the model, the current model is based on two variables, such that we also have a covariance between the innovations to consider. Individual differences in the covariance between innovations can be understood as resulting from three possible sources: (a) within-day effects from PA to NA; (b) within day effects from NA to PA; and (c) unobserved (or “third”) variables that vary over time and that affect both variables (for instance, the weather, social interactions, external demands, physiological states, etc.). In most instances, the covariance will be the result of a mix of these three sources. If we want to actually distinguish between these different explanations, we should obtain multiple measurements within a day, for instance through the use of experience sampling (see Conner et al., Citation2009). For our purposes, it is sufficient to assume that correlations between innovations are likely to result from all three sources, that is, from unobserved causes as well as within-day reciprocal effects between PA and NA. Moreover, because we believe that all these sources are likely to be characterized by individual differences, it makes sense to extend the model presented above with a random covariance between the innovations as well as random innovation variances.
4.2. Random innovation variances and covariance in the within-person level model
In the previous model, the distribution of the innovations was assumed to be identical across individuals, as is clear from the matrix Ψ(W) in Equation (Equation4(4)
(4) ). Now we want to allow for individual differences in the variances and covariance of the innovations, which implies Ψ(W) gets a subject index i. To account for such individual differences in variances, we can use a normal distribution for the log of the innovation variance, which is the same as saying that the innovation variance comes from a log normal distribution. There are two reasons to use a log normal distribution here: (a) it ensures that each individual will get a positive value for the innovation variance; and (b) it allows us to include this random effect in a multivariate normal distribution such that the random log of the innovation variance can be correlated with the other random effects such as the individual means or lagged parameters.
Extending the model with a random covariance is more complicated though, as the covariance matrix of the innovations has to be positive definite for each individual. To ensure this is the case, we make use of an additional latent variable ηit that represents what the two innovations have in common, while the unique parts are modeled as residuals. Specifically, the innovations are modeled as
(10)
(10) where the latter two residuals e are by definition uncorrelated; these form the unique parts of the innovations. The variances and covariance of the innovations for individual i can now be expressed as functions of the variances of the common factor and the residuals, that is,
(11)
(11)
(12)
(12)
(13)
(13) This shows that the variances of the innovations are the sum of the unique variances and the shared variance, while the covariance between the innovations is determined by the negative variance of the common factor.
The latter is the result of choosing the factor loadings of this common factor to be 1 and −1: This implies that for each individual, the covariance between the innovations will be negative. In the current model specification, we have to specify the innovation covariance to be either positive or negative for all individuals. This may seem needlessly restrictive or even an undesirable limitation. Alternatively, one can fix the variance of the common factor ηit to 1, and allow one of the factor loadings to be estimated freely and to vary randomly across individuals: This would allow for both positive and negative covariances between the innovations. However, this alternative specification does not guarantee that every covariance matrix can be fitted well, and in our case it caused convergence problems. Another approach would be to use a Cholesky decomposition of the covariance matrix, but this option is currently not available in Mplus.
The model defined in Equations (Equation1(1)
(1) ) and (Equation3
(3)
(3) ), combined with Equation (Equation10
(10)
(10) ), is visually represented in . It shows that we do not estimate any parameters at level 1, but that instead, we have nine random effects, that are estimated at the between level, which we discuss below.
Figure 4. Representation of the multilevel VAR(1) model with random innovation variances and covariance. Left part contains the decomposition into within-person (time-varying) and between-person (time-invariant) components. Top right contains the within-person level model, which is a VAR(1) model. Bottom right contains the between-person level model, which includes the between-person components of the observed data as well as all the random effects of the model, corresponding to the solid black circles in the within-person level model. It shows that random variances at the within-person level are modeled using their log at the between-person level (which are then assumed to come from a multivariate normal distribution); this is done to ensure that the individual variances are positive for all individuals.
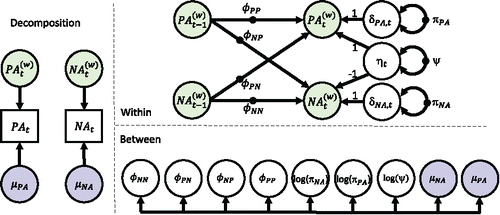
4.3. Between-person level model
As indicated above, random variances are given a log normal distribution at the between-person level, such that we end up estimating the average log of the variance as the fixed effect and the variance of the log of the variance as the random effect. This way we get three additional fixed effects, that is and
for the unique parts of the innovation variances, and γlog (ψ) for the negative covariance between the innovations. Furthermore, we get three additional random effects, that is, two for the unique parts of the innovations, and one for the shared part.
If we simply consider all nine random effects to be related to each other without any particular constraints, the between-person level model is
(14)
(14) where the nine γ’s represent the fixed or averaged effects, and the nine u’s represent the individual deviations from these means. These individual deviations are assumed to come from a multivariate normal distribution, that is,
(15)
(15) where Ω is a 9 × 9 covariance matrix, which implies there are 9 variances (i.e., the random effects), and 36 covariances. Combining this with the nine fixed effects, we now have a model with 54 parameters at the between-person level.
4.4. Priors
The current model only has parameters at the between-person level. The default priors for the nine fixed effects are
(16)
(16) where j = {P, N, PP, PN, NP, NN, log (πPA, i), log (πNA, i), log (ψi)}. The default prior for the covariance matrix of the random effects is
(17)
(17)
4.5. Results
Again, we used 50,000 iterations and a thinning of 10. The parameter estimates and their 95% credible intervals are presented in the right part of . Note that none of these intervals includes zero, indicating that there is ample evidence that these fixed effects differ from zero.
We also visually represented the correlations between the nine random effects in the lower part of . When comparing these to the correlation structures based on Model 1, it is striking that the relationships between the random effects that are included in both models (i.e., means and lagged parameters) change depending on whether or not the variances and covariance of the innovations are included as random effects. This underscores the need to consider potential randomness in these residual variances, because ignoring these additional sources of individual differences may result in biased estimates of the other model parameters, as was also shown by Jongerling et al. (Citation2015).
When comparing the correlation structures of the two samples based on Model 2, it is clear that the younger sample is characterized by more correlations than the older sample. However, there are also some similarities across the two groups. First, what is interesting to see is that both groups are characterized by strong positive connections between the individual’s mean on NA (i.e., μNA, i), the unique part of the innovation variance of NA (i.e., log (πNA, i)), and the log variance of the factor that represents the negative covariance between the innovations of PA and NA (i.e., log (ψi)). This implies that individuals with a relatively high mean on NA also tend to be characterized by a larger innovation variance (as this is the sum of πNA, i and ψi, see Equation (Equation12(12)
(12) )), and a stronger negative covariance between the innovations (as the covariance between the innovations is obtained by taking the exponential of log (ψi) and multiplying this by −1, see Equation (Equation13
(13)
(13) )).
Second, in both groups there are also relatively strong positive relationships between the autoregressive coefficient for NA (i.e., φNN, i), the mean of NA (i.e., μNA, i), and the unique part of the innovation variance of NA (i.e., log (πNA, i)). This implies that individuals who are relatively high on NA on average, tend to be characterized by a stronger carry-over effect for their NA, and they have larger (unique) innovation variances.
Third, in both groups there are positive relationships between the log of the unique variances of PA and NA (i.e., log (πPA, i) and log (πNA, i)) on the one hand, and the log of the negative covariance between the innovations of PA and NA (i.e., log (ψi). This implies that ψi is also positively related to πPA, i and πPA, i, which means that individuals who are more reactive to external influences and/or who experience more variability in external influences, tend to do so for both PA and NA (as the innovation variance of PA is the sum of πPA, i and log (ψi), and the innovation variance of NA is the sum of πNA, i and log (ψi); see Equations (Equation11(11)
(11) ) and (Equation12
(12)
(12) )).
Fourth, there is a negative correlation between the mean of PA (i.e., μPA, i) and the lagged effect from NA to PA (i.e., φPN, i) in both samples. This implies that individuals who are relatively high on PA on average, tend to be characterized by a lower cross-lagged effect from NA to PA.
Finally, there is a negative relationship in both groups between the autoregressive parameter of PA (i.e., φPP, i), and the negative covariance between the innovations. This implies that when individuals are characterized by more carry-over in their PA, they also tend to have a smaller value for the log of the negative covariance, which corresponds to larger negative covariance between the innovations.
While the current approach makes it easy to investigate the relationships between all the random effects in the model, this also leads to an increased complexity: Where we started with just two observed variables in the original decomposition, we now have nine random effects and 36 correlations between them. Alternatively however, one may also argue that where we started out with a data set of 2 × 100 × 100 (i.e., variables by persons by time points), we have now reduced this to a 9 by 9 correlation matrix.Footnote8 Either way, this is still a large number of relationships, which we may want to model further, for instance by using predictors or latent variables to account for their interrelatedness. We consider one particular approach next.
5. Model 3: Indirect effects through the random effects
As in most intensive longitudinal data sets, the COGITO study includes diverse person characteristics that were measured prior and/or after the intensive longitudinal measurement period. Here, we consider depressive symptoms measured by the Center for Epidemiologic Studies Depression (CESD) scale both before and after the daily measurement phase. Prior research, including analysis of this issue with the data from the COGITO study (see Brose, Schmiedek, Koval, & Kuppens, Citation2015), has shown that the autoregressive parameter of affective measurements is positively related to current levels of depression, but also that it is predictive of depression two and a half years later, suggesting that it flags an important malfunctioning regulation mechanism (Houben, Noortgate, & Kuppens, Citation2015; Koval et al., Citation2012; Kuppens et al., Citation2010; Kuppens et al., Citation2012). To see whether we can replicate these two findings in a single model, we include pre-CESD as a predictor of the nine random effects of the dynamic multilevel model described above, and post-CESD as an outcome variable of these random effects and of pre-CESD. We compute additional parameters in Mplus, based on a product of the two parameters that form an indirect effect through a particular random effect. This allows us to investigate these nine indirect effects, using their posterior distributions.
In , we have included the point estimates and the 95% CIs for the nine indirect effects and the direct effect. It shows that in both samples there is a positive direct effect of pre-CESD on post-CESD, with the effect in the older sample being about twice as large as that in the younger sample. Furthermore, in the younger sample there is evidence that two of the nine indirect effects are different from zero. The first of these is through the autoregressive parameter of NA (i.e., φNN), which confirms previous findings (Brose, et al., Citation2015; Kuppens et al., Citation2012): People with more depressive symptoms at the start (i.e., higher pre-CESD), tend to have more carryover of negative affective states (i.e., higher autoregression in NA), which in turn is associated with more depressive symptoms at the post-assessment (i.e., higher post-CESD). This effect exists after controlling for previous depression (i.e., the direct effect). The second indirect effect is through the covariance of the innovations, and illustrates the importance of looking past the usual suspects of means and autoregression: It implies that higher pre-CESD precedes a stronger negative covariance between the innovations (either because of within day reciprocal effects or because of an unmeasured common cause), which is followed by higher post-CESD. This latter result is in agreement with the idea that people who differentiate less in their affective responses (i.e., individuals with a larger negative covariance between innovations), run more risk of becoming depressed (see Borkulo, Boschloo, Borsboom, Penninx, & Schoevers, Citation2015).
Table 2. Point estimates (posterior means) and 95% credible intervals for direct and indirect effects of pre-CESD on post-CESD in both groups.
The two indirect effects added together are 0.199, which is about two-thirds of the direct effect, which is 0.290. Moreover, note that there were no indirect effects through the individual means of PA and NA of prior depressive symptoms on later depressive symptoms. In the younger sample, only the mean of NA was related to pre-CESD (point estimate and 95% CI: 0.78 [0.34,1.25]), whereas in the older sample the mean of PA was related to pre-CESD (point estimate and 95% CI: −2.00 [−2.94, −1.02]); none of the means were predictive of post-CESD. Although highly speculative, the largely absent association between depression scores and average PA and NA from the daily measurements may reflect a form of adaption in scale use, where depressed individuals grow used to feeling more NA and less PA, and thus change their reference point when filling out daily diaries.
6. Discussion
We have used the COGITO affect data to showcase some of the exciting new possibilities there are to analyze intensive longitudinal data. While dynamic multilevel modeling has been applied more often in recent years, the current approach forms a unique combination of a number of key features that make it particularly appealing, that is: (a) it is based on multivariate data, allowing for multiple dependent or outcome variables; (b) it handles missing data using MCMC sampling; (c) it handles unequal intervals by making the observations (approximately) equally spaced through adding missing values; (d) it gives results that are standardized per person, such that the relative strength of cross-lagged parameters can be compared; (e) it allows for random variances and covariances, which are to be expected from a substantive viewpoint; and (f) it allows for modeling the random effects at the between-person level, through considering these as outcome variables, but also as predictors.
Along the way, we have identified a number of questions that are likely to arise when taking a dynamic multilevel modeling approach. As we stated at the start, there are very few guidelines and rules of thumb for this approach; this stems from the fact that dynamic multilevel modeling is still in its infancy. To some extent, practices from other fields—such as traditional single-subject time series analysis—may be appropriate. However, we need to carefully evaluate how these will apply in the context of multilevel extensions. Moreover, we also need to consider their relevance for psychological research, as the goals one pursues here may differ from the goals in disciplines like econometrics.
Here, we provide a brief treatment of the issues identified before, and include a few additional concerns that we believe to be most urgent. For ease of presentation, we have grouped these into three categories: (a) data requirements; (b) model evaluation; and (c) theoretical concerns. Although we should not expect easy and clear cut solutions for many of these problems, it is paramount to the field and its progress to consider these issues in the years ahead.
6.1. Data requirements
6.1.1. Sample size
More needs to be known about the trade-off between the number of people, the number of time points, the number of variables, and the number of random effects, and other parameters in the model. This will clearly depend on the circumstances, and we should not expect a one-size-fits-all rule of thumb. However, general knowledge regarding reasonable ratios between these quantities would be extremely useful for researchers who wish to set up an intensive longitudinal study and wonder whether they should invest in more participants or more time points per participant.
One way in which such knowledge can be developed is through simulation studies that investigate the accuracy and reliability of parameter estimates, coverage rates, and efficiency. This can be done for basic models that are likely to be used frequently, but it could also be tailored to a particular research design, similar to a power study. For a first simulation study of this kind, see Schultzberg and Muthén (Citation2017). They focused on a series of univariate AR(1) models, with a random mean, autoregressive parameter, and innovation variance, which were predicted from a between-person level variable and used to predict another between-person level variable. One of their core findings was that samples with many persons and few time points outperform samples with few persons and many time points, and thus that it is easier to compensate few time points with more persons than vice versa. Furthermore, having random effects as dependent variables at the between-person level requires less data than having random effects serve as predictors of between-level outcome variables. They concluded that when random autoregression and random innovation variance are of interest and are used as predictors for a between level outcome, a sample of 200 or more persons and 100 or more occasions is recommended.
6.1.2. Nonnormal data
As indicated in the introduction, we assumed all data were normally distributed, both within and between individuals. However, it is well known that measures of negative affect tend to be nonnormal. More specifically, the within-person distribution for some individuals may look quite normal, but many other individuals are characterized by a strong floor effect in that they indicate at a large number of occasions that they experience no negative affect at all (see Brose, De Roover, Ceulemans, & Kuppens, Citation2015).
How to deal with such distributions continues to be a challenging question. Potential solutions could be to handle such variables as categorical (i.e., ordinal) rather than as continuous, to use latent variables with multiple indicators rather than sum scores, or a combination of these two (i.e., use ordinal indicators of a continuous latent variable). All these options are available in the current version of DSEM in Mplus, but they have not been studied extensively and compared yet. Another possible approach would be to use a regime-switching model, in which individuals switch between different states according to a hidden Markov model, which can be estimated using a continuous observed variable (see Hamaker, Grasman, & Kamphuis, Citation2016; Kim & Nelson, Citation1999). A multilevel extension of this approach will be possible with a future version of Mplus (see Asparouhov et al., Citation2017).
6.2. Model evaluation
6.2.1. Alternative models
First-order autoregressive models—like the ones used in the current empirical application, but also first-order differential equations—can be considered a very basic approach to study dynamics, and it is without a doubt the most frequently used approach for studying processes in the social sciences. However, there are many alternative models in the dynamic modeling literature on time series analysis and differential equations. These include higher-order autoregressive (or differential) models, models with moving average terms, integrated models (based on difference scores), models with measurement error, and models that allow for regime switching (Chatfield, Citation2004; Hamilton, Citation1994).
While some of these models have been used in dynamic mutlilevel analyses, allowing for individual differences in the dynamics parameters through modeling these as random effects at level 2 (see Asparouhov et al., Citation2017, Citation2018; Haan-Rietdijk, Gottman, Bergeman, & Hamaker, Citation2016; Wang et al. Citation2012), this area is still largely unexplored. However, the need to move beyond first-order autoregressive models is undeniable, whether the interest is in mere prediction or in actually understanding the underlying mechanism. As we expand the kinds of models we wish to consider, the need will increase to evaluate their fit and compare them.
6.2.2. Model comparison
There is a need for measures that can be used to compare nested and nonnested models. A commonly used measure in Bayesian analysis is the deviance information criterion (DIC; Spiegelhalter, Best, Carlin, & van der Linde, Citation2002), which is also reported when using DSEM. However, it is not functioning optimally for these models, due to the many parameters that need to be sampled: All the individual parameters are also treated as unknown parameters in the Bayesian context, which have to be sampled at each iteration, and this makes the DIC very unstable, even when very large numbers of iterations are used. Moreover, because the DIC is based on a likelihood in which the random effects are included, the DIC is not always comparable across models due to the parameterization (see Celeux, Forbes, Robert, & Titterington, Citation2006).
Alternatively, we can check whether there is evidence that a parameter differs from zero based on its CI, but for variance terms, these will never include zero by definition. Hence, at this point there is no easy to use tool to determining whether a random effect is necessary, or that the variance across individuals can be fixed to zero.
6.2.3. Model fit
Related to model comparison, there is also a need for measures to evaluate model fit. This may prove rather challenging, as even in N = 1 time series analysis, there is not a strong tradition of summarizing model fit. This may be because the focus in time series analysis is not specifically on the proportion of explained variance (as it tends to be in regression analysis), but also on the autocovariance structure of the data, and this includes all lags (from lag 0 up to lag T − 1 for a series of length T). As a result, there is no saturated model that could serve as a basis for comparison as is typically done in structural equation modeling.
Evaluating the appropriateness of a time series model has often relied on investigating whether the residuals (i.e., innovations) behave like white noise, meaning there are no autocorrelations in these series (Box et al., Citation2016): This illustrates the strong focus on trying to capture the autocovariance structure in the observed data. In multilevel multivariate extensions, this implies we have to consider the autocorrelation and partial autocorrelation functions for each variable and the cross-lagged correlations functions for each pair of variables for each person: Clearly, it will not be easy to keep track of the many functions, and even more so, it would also require the development of new rules of thumb regarding—for instance—the number of acceptable violations of the white-noise assumption.
6.3. Theoretical concerns
6.3.1. Causality
Although the topic is often not discussed explicitly, researchers in the social sciences who use longitudinal data are often interested in the causal relationships between variables. While longitudinal data allows for temporal order—such that a potential cause precedes its assumed effects in time—these are nevertheless observational data, which implies that the omitted variable problem hampers causal conclusions. In this regard, it is helpful to distinguish between time-invariant and time-varying omitted variables.
Time-invariant omitted variables are of no concern when using either a single subject approach, because it is a constant in that context. Similarly, when we have multilevel longitudinal data, the decomposition into a between-person and a within-person part like we discussed at the beginning of this paper, ensures that the time-invariant omitted variables are separated from the within-person dynamics (see also Allison, Citation2009; Halaby, Citation2004; Hamaker, Kuiper, & Grasman, Citation2015; Ousey, Wilcox, & Fisher, Citation2011). However, time-varying omitted variables are still a major concern when our interest is in causal mechanisms that we hope to understand better by evaluating the within-person part.
In the time series literature, the concept of Granger causality is often brought up, but the opinions are divided on how useful it is as a basis for causal conclusions. Eichler (Citation2013) and Eichler and Didelez (Citation2010) have connected diverse definitions of causality, including Granger causality and interventionism in the context of time series. There have also been innovations in the area of design, referred to as micro-randomization, in which an intervention is randomly assigned to time point (Klasnja et al., Citation2015). This approach has been used in health research, for instance to stimulate participant to be more physically active. Other recent developments have used time series models in combination with impulse response functions to generate feedback for participants about how they may generate, for instance, a 10% increase in one variable by changing another variable (Blaauw, van der Krieke, Emerencia, Aiello, & de Jonge, Citation2017). Hence, in the latter case, the time series model is used to generate hypotheses that professionals may use to design a person-tailored intervention for a particular individual.
6.3.2. Trends and cycles
Closely related to the concern of omitted time-varying variables, is the issue of trends and cycles in the data. In the time series literature, the convention is to de-trend data before considering further relationships between variables (e.g., Chatfield, Citation2004), and this approach has also been advocated by some in panel research and intensive longitudinal studies (e.g., Berry & Willough by, Citation2017; Curran & Bauer, Citation2011; Wang et al., Citation2012). Wang and Maxwell (Citation2015) showed that when the interest is in the effect of a predictor on an outcome in longitudinal multilevel models, it does not matter whether one de-trends the predictor, de-trends the predictor and the outcome, or includes time as a covariate without de-trending the predictor or the outcome. However, the results can differ starkly from those obtained when the trend is not accounted for in one of these ways.
As pointed out by Wang and Maxwell (Citation2015) and Liu and West (Citation2016), how we handle potential trends and cycles in the data, is likely to have serious consequences for the parameter estimates that describe the dynamics of the process and the predictive relationships between variables over time: It should therefore reflect our conviction of the underlying mechanism, and whether we believe trends and cycles are something that happens separately from the dynamics, or as an intrinsic part of it. In the latter case, one may actually prefer not to explicitly model trend or cycles, but simply allow these to emerge as part of the dynamics of a model. The latter approach is common in the dynamic systems literature, where long-term trends are assumed to result from the same underlying dynamics as the short-term fluctuations (see Bisconti et al., Citation2004; Van der Maas et al., Citation2006; Voelkle et al., Citation2012).
The models used in this paper could be extended with trends or cycles over time by adding this as a within-person level predictor. As shown by Wang and Maxwell (Citation2015), whether this approach is used, or the relationships are modeled between the residuals of the variables (after the trends are account for), makes little difference (see also Hamaker, Citation2005). Alternatively, trends could be accounted for through the cross-classification option offered by DSEM (see Asparouhov et al., Citation2018); this approach accounts for the average (across individuals) trend over time in an exploratory manner, meaning the researcher does not have to decide on the shape such as linear, sine wave, etcetera. Alternatively, we can add time as a predictor and allow for a random slope so that individuals can be characterized by different trends. However, when individuals are characterized by (very) different trends, or are not in sync in their cycles, this approach would not be appropriate, and it is better to use the explicit modeling of it at the within-person level.
6.3.3. Between-person differences
As shown in Models 1 and 2, even with a relatively simple bivariate data set, we can easily obtain a rather complex covariance structure at the between-person level. Some have proposed to use the within-person means to construct between-person networks that can be used as a tool for making causal inferences (Epskamp, Waldorp, Mottus, & Borsboom, Citation2017). However, like the time-varying omitted variables are a threat to causal inference at the within-person level, time-invariant omitted variables are a major concern when wishing to draw causal conclusions at the between-person level.
Nevertheless, these modeling opportunities challenge researchers to think about their theories in new ways, and to become more precise about their hypotheses: Do they assume their theory pertains to the within-person level or the between-person level, and at what timescale do they expect particular relationships to appear? Such considerations can guide the modeling approach, but may also have important implications for the way the data should be gathered.
6.3.4. Discrete versus continuous time
The results that are obtained with discrete time models such as the VAR(1) models used in this study are specific to a particular time interval. While for daily diary data, where individuals are asked to provide an average of their experiences for the entire day, this approach may be considered appropriate, it is more problematic when the data consist of momentary experiences, such as obtained with, for instance, experience sampling or ecological momentary assessments. In such approaches, the measurements are taken at random intervals, which reflects the underlying assumption that the process actually evolves continuously over time and could be measured at any time point.
An alternative modeling approach that has been used for such data is continuous time modeling (Oravecz, Tuerlinckx, & Vandekerckhove, Citation2011; Voelkle et al., Citation2012). These models are based on differential equations and instead of estimating the lagged effects for a particular interval as we do in discrete time models, continuous time models result in a drift matrix that represents the expected change (i.e., the first-order derivatives) as a function of the current values of the variables in the system (Boker & Laurenceau, Citation2006; Oud, Citation2007). Thus, compared to discrete time models, continuous time models have the advantage that they naturally account for unequal intervals between the observations (including missing observations), and that the resulting parameters do not pertain to a particular interval that was used in the analysis.
It should be noted however that there are mathematical relationships between the drift matrix that is obtained with continuous time analysis, and the matrix for lagged coefficients from a discrete time analysis, such that even when a discrete time analysis is executed, the results can be converted to what they would be when a continuous time analysis had been run instead (Deboeck & Preacher, Citation2015; Haan-Rietdijk et al., Citation2017; Voelkle et al., Citation2012). At a more fundamental level, however, the discrete versus continuous time distinction raises the question of how the results of lagged models are best represented: Should we provide the result that are related to a particular lag, or consider an alternative representation related to the continuous time perspective, such as the drift matrix, vector fields (Boker & McArdle, Citation2005), or curves of the value particular parameters take on at different intervals (e.g., Deboeck & Preacher, Citation2015)? While all these representations are based on the same information, the relation between them may be hard to grasp for researchers who are not that familiar with dynamic systems analyses, and it is not clear which of these representations is most intuitive and valuable in the context of applied research. Nevertheless, it is important that researchers in this area recognize the limitations of only focusing on the lagged parameters obtained from a discrete time analysis.
6.3.5. Structural missings due to nighttime or weekends
In the COGITO data, no observations were made on Sundays (and for many participants Saturdays) or holidays. Such structural missing data are quite common in intensive longitudinal data. For instance, in experience sampling, which is based on multiple measurements per day, typically no measurements are taken during the night. In the current approach, we assumed that the process just continued during the missing episodes. Alternatively, instead of dealing with these data as two-level with observations nested in individuals, we can also consider them as three-level with repeated measures nested in days or weeks, which are subsequently nested in individuals.
Haan-Rietdijk, Kuppens, and Hamaker (Citation2016) compared the two-level and three-level approach in the context of experience sampling data, where the two-level model consists of measures nested in persons, and the three-level model consists of beeps nested in days which are nested in persons. The results showed that autoregression in a two-level model may result from ignoring the three-level structure of the data. Conversely, it is also possible to find day-level variance, which would seem to point to a three-level model, but that actually resulted from autoregression in a two-level model. Their empirical application showed more evidence for the two-level than the three-level model, but clearly more research is needed with diverse data sets and data collection protocols.
6.4. Conclusion
With the many novel modeling opportunities that have appeared recently for the analysis of intensive longitudinal data, we also see many new challenges and unresolved issues that require solutions. However, we should not let this discourage us at this point. Rather, it is our hope that the rapid technological and statistical developments that are taking place now will inspire many of us to pursue a research line in this innovative field: We need psychometricians, applied statisticians, quantitative psychologists, and substantive researchers to explore this exciting new frontier, so that 10 years from now we can look back and smile at how little was known today.
Article information
Conflict of Interest Disclosures. Each author signed a form for disclosure of potential conflicts of interest. Three of the authors (ELH, TA, and BM) indicated that they have contributed to the development of dynamic structural equation modeling (DSEM) in Mplus, which was used to analyze the data.
Ethical Principles. The authors affirm having followed professional ethical guidelines in preparing this work. These guidelines include obtaining informed consent from human participants, maintaining ethical treatment and respect for the rights of human or animal participants, and ensuring the privacy of participants and their data, such as ensuring that individual participants cannot be identified in reported results or from publicly available original or archival data.
Funding. This work was not funded.
Role of the Funders/Sponsors. None of the funders or sponsors of this research had any role in the design and conduct of the study; collection, management, analysis, and interpretation of data; preparation, review, or approval of the manuscript; or decision to submit the manuscript for publication.
Acknowledgments. The ideas and opinions expressed herein are those of the authors alone, and endorsement by the authors’ institutions is not intended and should not be inferred.
HMBR_A_1446819_Supplementary.docx
Download MS Word (20.5 KB)Notes
1 At this point, multiple group analyses are not part of DSEM in Mplus version 8. Alternatively, we could treat the data as one sample, and have a grouping variable that is used at the between-person level to see whether there are differences between the groups. However, as the two samples were sampled from different age populations, we decided to treat them as two separate samples here.
2 Brose et al. (Citation2013) actually investigated the effect of current affective state on the way individuals answer questions with respect to what they are like in general (i.e., when they are given a trait instruction), using data from the COGITO study. The results showed that the current affective state a person is in contributed substantially to the way a person evaluates their general affect. It is likely that the relative contributions of trait and state depend on the instruction that is given (Steyer, Ferring, & Schmitt, Citation1992), but studies like the one by Brose et al. (Citation2013) show that using a particular instruction does not eliminate the potential contamination of other sources of variability.
3 In the younger sample, for instance, the intervals were 1 day for 10,066 cases, 2 days for 7321 cases, 3 days for 1580 cases, 4 days for 636 cases, 5 days for 168 cases, 6 days for 89 cases, 7 days for 54 cases, and so on, with a maximum interval of 54 days.
4 While inertia is a term that has been used extensively to refer to autoregression and autocorrelation in affective research, starting with Suls, Green, and Hillis (Citation1998) and Gottman, Murray, Swanson, Tyson, and Swanson (Citation2002), this should not be confused with the usage of this term in the context of differential equations and physics.
5 These are obtained as part of the output in Mplus when asking for the TECH1 output.
6 Note that the CI of a variance term will never include zero, as the prior is specified to be larger than zero. Hence, using the CI of a variance estimate to investigate whether there is evidence that a parameter differs from zero, is not as straightforward as it is for other parameters.
7 The standardization is based on the assumption that the random effects are normally distributed, which implies that the standardized effects most probably are not normally distributed. However, preliminary simulations have shown that if the random effect itself is not normally distributed, this level 2 assumption is quickly overruled as sample size (at level 1) increases.
8 The authors thank Nilam Ram for pointing out this alternative perspective.
References
- Allison, P. D. (2009). Fixed effects regression models. Thousand Oaks, CA: Sage. doi: 10.4135/9781412993869
- Almeida, D. M., Wethington, E., & Chandler, A. L. (1999). Daily transmission of tension between marital dyads and parent-child dyads. Journal of Marriage and the Family, 61, 49–61. doi: 10.2307/353882
- Asparouhov, T., Hamaker, E. L., & Muthén, B. (2017). Dynamic latent class analysis. Structural Equation Modeling, 24, 257–269. doi: 10.1080/10705511.2016.1253479
- Asparouhov, T., Hamaker, E. L., & Muthén, B. (2018). Dynamic structural equation models. Structural Equation Modeling, 25, 359–388. doi: 10.1080/10705511.2017.1406803
- Asparouhov, T., & Muthén, B. (2010). Bayesian analysis of latent variable models using Mplus (version 3)(Technical Report). Retrieved from http://statmodel.com/download/Bayes3.pdf
- Beltz, A. M., Wright, A. G. C., Sprague, B. N., & Molenaar, P. C. M. (2016). Bridging the nomothetic and idiographic approaches to the analysis of clinical data. Assessment, 23, 447–458. doi: 10.1177/1073191116648209
- Berry, D., & Willoughby, M. T. (2017). On the practical interpretability of cross-lagged panel models: Rethinking a developmental workhorse. Child Development, 88, 1186–1206. doi: 10.1111/cdev.12660
- Bisconti, T., Bergeman, C. S., & Boker, S. M. (2004). Emotional well-being in recently bereaved widows: A dynamical system approach. Journal of Gerontology, Series B: Psychological Sciences and Social Sciences, 59, 158–167. doi: 10.1093/geronb/59.4.P158
- Blaauw, F. J., van der Krieke, L., Emerencia, A. C., Aiello, M., & de Jonge, P. (2017). Personalized advice for enhancing well-being using automated impulse response analysis — AIRA. Retrieved from https://arxiv.org/abs/1706.09268
- Boker, S. M., & Laurenceau, J.-P. (2006). Dynamic system modeling: An application to the regulation of intimacy and disclosure in marriage. In T. A. Walls & J. L. Schafer (Eds.), Models for intensive longitudinal data (p.195–218). New York, NY: Oxford University Press. doi: 10.1093/acprof:oso/9780195173444.003.0009
- Boker, S. M., & McArdle, J. J. (2005). Vector field plot. In P. Armitage & P. Colton (Eds.), Encyclopedia of biostatistics (Second Edition, pp. 5700–5704). Chichester, England: Wiley. doi: 10.1002/0470011815.b2a12068
- Boker, S. M., Neale, M., Maes, H., Wilde, M., Spiegel, M., Brick, T., … J., F. (2011). OpenMx: An open source extended structural equation modeling framework. Psychometrika, 76, 306–317. doi: 10.1007/S11336-010-9200-6
- Bolger, N., DeLongis, A., Kessler, R. C., & Wethington, E. (1989). The contagion of stress across multiple roles. Journal of Marriage and the Family, 51, 175–183. doi: 10.2307/352378
- Bolger, N., & Laurenceau, J.-P. (2013). Intensive longitudinal methods: An introduction to diary and experience sampling research. New York, NY: The Guilford Press.
- Borsboom, D., Mellenbergh, G. J., & Van Heerden, J. (2003). The theoretical status of latent variables. Psychological Review, 110, 203–219. doi: 10.1037/0033-295X.110.2.203
- Box, G. E. P., Jenkins, G. M., Reinsel, G. C., & Ljung, G. M. (2016). Time series analysis: Forecasting and control (5 ed.). Hoboken, NJ: Holden-Day. doi: 10.1111/jtsa.12194
- Bringmann, L. F., Vissers, N., Wichers, M., Geschwind, N., Kuppens, P., Peeters, … Tuerlinckx, F. (2013). A network approach to psychopathology: New insights into clinical longitudinal data. PLoS ONE, 8, e60188, 1–13. doi: 10.1371/journal.pone.0060188
- Brose, A., De Roover, K., Ceulemans, E., & Kuppens, P. (2015). Older adults’ affective experiences across 100 days are less variable and less complex than younger adults’. Psychology and Aging, 30, 194–208. doi: 10.1037/a0038690
- Brose, A., Schmiedek, F., Koval, P., & Kuppens, P. (2015). Emotional inertia contributes to depressive symptoms beyond perseverative thinking. Cognition and Emotion, 29, 527–538. doi: 10.1080/02699931.2014.916252
- Brose, A., Schmiedek, F., & Lindenberger, U. (2013). Affective states contribute to trait reports of affective well-being. Emotion, 13, 940–948. doi: 10.1037/a0032401
- Brose, A., Voelkle, M. C., Lövdén, M., Lindenberger, U., & Schmiedek, F. (2015). Differences in the between-person and the within-person structures of affect are a matter of degree. European Journal of Personality, 29, 55–71. doi: 10.1002/per.1961
- Cattell, R. B. (1952). The three basic factor-analytical research designs: Their interrelations and derivatives. Psychological Bulletin, 49, 499–520. doi: 10.1037/h0054245
- Celeux, G., Forbes, F., Robert, C. P., & Titterington, D. M. (2006). Deviance information criteria for missing data models. Bayesian Analysis, 1, 651–674. doi: 10.1214/06-BA122
- Chatfield, C. (2004). The analysis of time series: An introduction (6th ed.). London, UK: Chapman and Hall. doi: 10.1007/978-1-4899-2923-5
- Chow, S.-M., Nesselroade, J. R., Shifren, K., & McArdle, J. J. (2004). Dynamic structure of emotions among individuals with Parkinson’s disease. Structural Equation Modeling: A Multidisciplinary Journal, 11, 560–582. doi: 10.1207/s15328007sem1104_4
- Chow, S.-M., Ram, N., Boker, S. M., Fujita, F., & Clore, G. (2005). Emotion as a thermostat: Representing emotion regulation using a damped oscillator model. Emotion, 5, 208–225. doi: 10.1037/1528-3542.5.2.208
- Conner, T. S., Tennen, H., Fleeson, W., & Feldman Barrett, L. (2009). Experience sampling methods: A modern idiographic approach to personality research. Social and Personality Psychology Compass, 3, 292–313. doi: 10.1111/j.1751-9004.2009.00170.x
- Curran, P. J., & Bauer, D. J. (2011). The disaggregation of within-person and between-person effects in longitudinal models of change. Annual Review of Psychology, 62, 583–619. doi: 10.1146/annurev.psych.093008.100356
- Deboeck, P., & Preacher, K. (2015). No need to be discrete: A method for continuous time mediation analysis. Structural Equation Modeling: A Multidisciplinary Journal, 23, 1–15. doi: 10.1080/10705511.2014.973960
- de Haan-Rietdijk, S., Gottman, J. M., Bergeman, C. S., & Hamaker, E. L. (2016). Get over it! A multilevel threshold autoregressive model for state-dependent affect regulation. Psychometrika, 81, 217–241. doi: 10.1007/s11336-014-9417-x
- de Haan-Rietdijk, S., Kuppens, P., & Hamaker, E. L. (2016). What’s in a day? A guide to decomposing the variance in intensive longitudinal data. Frontiers in Psychology, 7, 891. doi: 10.3389/fpsyg.2016.00891
- de Haan-Rietdijk, S., Voelkle, M., Keijsers, L., & Hamaker, E. L. (2017). Discrete- vs. continuous-time modeling of unequally spaced experience sampling method data. Frontiers in Psychology, 8, 1849. doi: 10.3389/fpsyg.2017.01849
- Driver, C. C., Oud, J. H. L., & Voelkle, M. C. (2017). Continuous time structural equation modeling with R package ctsem. Journal of Statistical Software, 77, 1–35. doi: 10.18637/jss.v077.i05
- Durbin, J., & Koopman, S. J. (2012). Time series analysis by state space methods (2 ed.). New York, NY: Oxford University Press. doi: 10.1093/acprof:oso/9780199641178.001.0001
- Eichler, M. (2013). Causal inference with multiple time series: Principles and problems. Philosophical Transactions of the Royal Society A, 371, 2011.0613. doi: 10.1098/rsta.2011.0613
- Eichler, M., & Didelez, V. (2010). On Granger-causality and the effect of interventions in time series. Life Time Data Analysis, 16, 3–32. doi: 10.1007/s10985-009-9143-3
- Epskamp, S., Cramer, A. O. J., Waldorp, L. J., Schmittmann, V. D., & Borsboom, D. (2012). qgraph: Network visualizations of relationships in psychometric data. Journal of Statistical Software, 48, 1–18. doi: 10.18637/jss.v048.i04
- Epskamp, S., Deserno, M. K., & Bringmann, L. F. (2017). mlVAR: Multi-level vector autoregression [computer software manual]. (R package version 0.4). Retrieved from https://cran.r-project.org/web/packages/mlVAR/mlVAR.pdf
- Epskamp, S., Waldorp, L. J., Mottus, R., & Borsboom, D. (2017). Discovering psychological dynamics: The Gaussian Graphical Model in cross-sectional and time-series data. Retrieved from https://arxiv.org/abs/1609.04156
- Gates, K. M., & Molenaar, P. C. M. (2012). Group search algorithm recovers effective connectivity maps for individuals in homogeneous and heterogeneous samples. NeuroImage, 65, 310–319. doi: 10.1016/j.neuroimage.2012.06.026
- Gelman, A., Carlin, J. B., Stern, H. S., Dunson, D. B., Vehtari, A., & Rubin, D. (2014). Bayesian data analysis (3 ed.). Boca Raton, FL: CRC Press, Taylor & Francis Group.
- Gollob, H. F., & Reichardt, C. S. (1987). Taking account of time lags in causal models. Child Development, 58, 80–92. doi: 10.1111/j.1467-8624.1987.tb03492.x
- Gottman, J. M., Murray, J. D., Swanson, C. C., Tyson, R., & Swanson, K. R. (2002). The mathematics of marriage: Dynamic nonlinear models. Cambridge, MA: MIT Press.
- Grice, J. W. (2004). Bridging the idiographic-nomothetic divide in ratings of self and others. Journal of Personality, 72, 203–241. doi: 10.1111/j.0022-3506.2004.00261.x
- Halaby, C. N. (2004). Panel models in sociological research: Theory intro practice. Annual Review of Sociology, 30, 507–544. doi: 10.1146/annurev.soc.30.012703.110629
- Hamaker, E. L. (2005). Conditions for the equivalence of the autoregressive latent trajectory model and a latent growth curve model with autoregressive disturbances. Sociological Methods and Research, 33, 404–418. doi: 10.1177/0049124104270220
- Hamaker, E. L. (2012). Why researchers should think “within-person” a paradigmatic rationale. In M. R. Mehl & T. S. Conner (Eds.), Handbook of research methods for studying daily life (p.43–61). New York, NY: Guilford Publications. doi: 10.1177/1468794113484441
- Hamaker, E. L., Dolan, C. V., & Molenaar, P. C. M. (2002). On the nature of SEM estimates of ARMA parameters. Structural Equation Modeling, 9, 347–368. doi: 10.1207/S15328007SEM0903_3
- Hamaker, E. L., Dolan, C. V., & Molenaar, P. C. M. (2003). ARMA-based SEM when the number of time points T exceeds the number of cases N: Raw data maximum likelihood. Structural Equation Modeling, 10, 352–379. doi: 10.1207/S15328007SEM1003_2
- Hamaker, E. L., Dolan, C. V., & Molenaar, P. C. M. (2005). Statistical modeling of the individual: Rationale and application of multivariate time series analysis. Multivariate Behavioral Research, 40, 207–233. doi: 10.1207/s15327906mbr4002_3
- Hamaker, E. L., Grasman, R. P. P. P., & Kamphuis, J. H. (2016). Modeling BAS dysregulation in bipolar disorder: Illustrating the potential of time series analysis. Assessment, 23, 436–446. doi: 10.1177/1073191116632339
- Hamaker, E. L., Kuiper, R., & Grasman, R. P. P. P. (2015). A critique of the cross-lagged panel model. Psychological Methods, 20, 102–116. doi: 10.1037/a0038889
- Hamaker, E. L., Nesselroade, J. R., & Molenaar, P. C. M. (2007). The integrated trait-state model. Journal of Research in Personality, 41, 295–315. doi: 10.1016/j.jrp.2006.04.003
- Hamaker, E. L., Schuurman, N. K., & Zijlmans, E. A. O. (2017). Using a few snapshots to distinguish mountains from waves: Weak factorial invariance in the context of trait-state research. Multivariate Behavioral Research, 52, 47–60 doi: 10.1080/00273171.2016.1251299
- Hamaker, E. L., & Wichers, M. (2017). No time like the present: Discovering the hidden dynamics in intensive longitudinal data. Current Directions in Psychological Science, 26, 10–15. doi: 10.1177/0963721416666518
- Hamilton, J. D. (1994). Time series analysis. Princeton, NJ: Princeton University Press.
- Harvey, A. C. (1989). Forecasting, structural time series models and the Kalman filter. Cambridge, UK: University Press. doi: 10.1017/CBO9781107049994
- Houben, M., Noortgate, W. Van den, & Kuppens, P. (2015). The relation between short-term emotion dynamics and psychological well-being: A meta-analysis. Psychological Bulletin, 141, 901–930. doi: 10.1037/a0038822
- Jongerling, J., Laurenceau, J.-P., & Hamaker, E. L. (2015). A multilevel AR(1) model: Allowing for inter-individual differences in trait-scores, inertia, and innovation variance. Multivariate Behavioral Research, 184, 334–349. doi: 10.1080/00273171.2014.1003772
- Kim, C.-J., & Nelson, C. R. (1999). State-space models with regime switching: Classical and Gibbs-sampling approaches with applications. Cambridge, MA: The MIT Press. doi: 10.2307/2669796
- Klasnja, P., Hekler, E. B., Shiffman, S., Boruvka, A., Almirall, D., Tewari, A., & Murphy, S. A. (2015). Micro-randomized trials: An experimental design for developing just-in-time adaptive interventions. Health Psychology, 34, 1220–1228. doi: 10.1037/hea0000305
- Koval, P., Kuppens, P., Allen, N. B., & Sheeber, L. (2012). Getting stuck in depression: The roles of rumination and emotional inertia. Cognition and Emotion, 26, 1412–1427. doi: 10.1080/02699931.2012.667392
- Kuppens, P., Allen, N. B., & Sheeber, L. B. (2010). Emotional inertia and psychological maladjustment. Psychological Science, 21, 984–991. doi: 10.1177/0956797610372634
- Kuppens, P., Sheeber, L. B., Yap, M. B. H., Whittle, S., Simmons, J., & Allen, N. B. (2012). Emotional inertia prospectively predicts the onset of depression in adolescence. Emotion, 12, 283–289. doi: 10.1037/a0025046
- Lamiell, J. T. (1998). ‘Nomothetic’ and ‘idiographic’: Contrasting Windelband’s understanding with contemporary usage. Theory and Psychology, 8, 23–38. doi: 10.1177/0959354398081002
- Liu, S. (2017). Person-specific versus multilevel autoregressive models: Accuracy in parameter estimates at the population and individual levels. British Journal of Mathematical and Statistical Psychology, 70, 480–498. doi: 10.1111/bmsp.12096
- Liu, Y., & West, S. G. (2016). Weekly cycles in daily report data: An overlooked issue. Journal of Personality, 84, 560–579. doi: 10.1111/jopy.12182
- Lynch, S. M. (2007). Introduction to applied Bayesian statistics and estimation for social scientists. New York, NY: Springer Science & Bussiness Media.
- Masten, A. S., & Cichetti, D. (2010). Developmental cascades. Development and Psychopathology, 22, 491–495. doi: 10.1017/S0954579410000222
- Molenaar, P. C. M. (1985). A dynamic factor model for the analysis of multivariate time series.Psychometrika, 50, 181–202. doi: 10.1007/bf02294246
- Molenaar, P. C. M. (1987). Dynamic assessment and adaptive optimization of the psychotherapeutic process. Behavioral Assessment, 9, 389–416. doi: 10.1007/BF00959854
- Molenaar, P. C. M. (2004). A manifesto on psychology as idiographic science: Bringing the person back into scientific psychology - this time forever. Measurement: Interdisciplinary Research and Perspectives, 2, 201–218. doi: 10.1207/s15366359mea0204_1
- Molenaar, P. C. M., Huizenga, H. M., & Nesselroade, J. R. (2003). The relationship between the structure of interindividual and intraindividual variability: A theoretical and empirical vindication of developmental systems theory. In U. M. Staudinger & U. Lindenberger (Eds.), Understanding human development: Dialogues with lifespan psychology (p.339–360). Norwell, MA: Kluwer Academic Publishers. doi: 10.1007/978-1-4615-0357-6_15
- Nesselroade, J. R. (2002). Elaborating on the differential in differential psychology. Multivariate Behavioral Research, 37, 543–561. doi: 10.1207/S15327906MBR3704_06
- Oravecz, Z., Tuerlinckx, F., & Vandekerckhove, J. (2011). A hierarchical latent stochastic difference equation model for affective dynamics. Psychological Methods, 16, 468–490. doi: 10.1037/a0024375
- Oravecz, Z., Tuerlinckx, F., & Vandekerckhove, J. (2016). Bayesian data analysis with the bivariate hierarchical Ornstein-Uhlenbeck process model. Multivariate Behavioral Research, 51, 106–119. doi: 10.1080/00273171.2015.1110512
- Ou, L., Hunter, M., & Chow, S.-M. (2018). dynr: Dynamic modeling in R. (R-package version 0.1.12-5). Retrieved from: https://cran.r-project.org/web/packages/dynr/
- Oud, J. H. L. (2007). Continuous time modeling of reciprocal relationships in the cross-lagged panel design. In S. M. Boker & M. J. Wenger (Eds.), Data analytic techniques for dynamic systems in the social and behavioral sciences (p.87–129). Mahwah, NJ: Lawrence Erlbaum Associates. doi: 10.4324/9780203936757
- Ousey, G. C., Wilcox, P., & Fisher, B. S. (2011). Something old, something new: Revisiting competing hypothesis of the victimization-offending relationship among adolescents. Journal of Quantitative Criminology, 27, 53–84. doi: 10.1007/s10940-010-9099-1
- Plummer, M. (2003). JAGS: A program for analysis of Bayesian graphical models using Gibbs sampling. Retrieved from https://www.r-project.org/conferences/DSC-2003/Drafts/Plummer.pdf
- Ram, N., Chow, S.-M., Bowles, R. P., Wang, L., Grimm, K., Fujita, F., & Nesselroade, J. R. (2005). Examining interindividual differences in cyclicity of pleasant and unpleasant affects using spectral analysis and item response modeling. Psychometrika, 70, 773–790. doi: 10.1007/s11336-001-1270-5
- Rovine, M. J., & Walls, T. A. (2006). Multilevel autoregressive modeling of interindividual differences in the stability of a process. In T. A. Walls & J. L. Schafer (Eds.), Models for intensive longitudinal data (pp.124–147). New York, NY: Oxford University Press. doi: 10.1093/acprof:oso/9780195173444.003.0006
- Sadler, P., Ethier, N., Gunn, G. R., Duong, D., & Woody, E. (2009). Are we on the same wavelength? Interpersonal complementarity as shared cyclical patterns during interactions. Journal of Personality and Social Psychology, 97, 1005–1020. doi: 10.1037/a0016232
- Särkkä, S. (2013). Bayesian filtering and smoothing. Cambridge, UK: Cambridge University Press. doi: 10.1017/CBO9781139344203
- Schmitz, B. (2000). Auf der Suche nach dem verlorenen Individuum: Vier Theoreme zur Aggregation von Prozessen [In search of the lost individual: Four theorems regarding the aggregation of processes]. Psychologische Rundschau, 51, 83–92. doi: 10.1026//0033-3042.51.2.83
- Schmitz, B., & Skinner, E. (1993). Perceived control, effort, and academic performance: Interindividual, intrainidividual, and multivariate time-series analyses. Journal of Personality and Social Psychology, 64, 1010–1028. doi: 10.1037/0022-3514.64.6.1010
- Schultzberg, M., & Muthén, B. (2017). Number of subjects and time points needed for multilevel time series analysis: A simulation study of dynamic structural equation modeling. Structural Equation Modeling. doi: 10.1080/10705511.2017.1392862
- Schuurman, N. K., Ferrer, E., Boer-Sonnenschein, M. de, & Hamaker, E. L. (2016). How to compare cross-lagged associations in a multilevel autoregressive model. Psychological Methods, 21, 206–221. doi: 10.1037/met0000062
- Schuurman, N. K., Houtveen, J. H., & Hamaker, E. L. (2015). Incorporating measurement error in n = 1 psychological autoregressive modeling. Frontiers in Psychology, 6, 1038. doi: 10.3389/fpsyg.2015.01038
- Shumway, R. H., & Stoffer, D. S. (2017). Time series analysis and its applications: With R examples (4 ed.). New York, NY: Springer. doi: 10.1007/978-3-319-52452-8
- Spiegelhalter, D. J., Best, N. G., Carlin, B. P., & van der Linde, A. (2002). Bayesian measures of model complexity and fit. Journal of the Royal Statistical Society, 64, 1–34. doi: 10.1111/1467-9868.00353
- Spiegelhalter, D. J., Thomas, D., Best, N. G., & Lunn, D. (2003). WinBUGS version 1.4 user manual. Cambridge, UK: MRC Biostatistics Unit. Retrieved from http://www.mrc-bsu.cam.ac.uk/bugs/
- Stan Development Team. (2017). Stan modeling language: User’s guide and reference manual (version 2.16.0). Retrieved from http://mc-stan.org/users/documentation/
- Steyer, R., Ferring, D., & Schmitt, M. J. (1992). States and traits in psychological assessment. European Journal of Psychological Assessment, 8, 79–98.
- Suls, J., Green, P., & Hillis, S. (1998). Emotional reactivity to everyday problems, affective inertia, and neuroticism. Personality and Social Psychology Bulletin, 24, 127–136. doi: 10.1177/0146167298242002
- Trull, T. J., & Ebner-Priemer, U. (2013). Ambulatory assessment. Annual Review of Clinical Psychology, 9, 151–176. doi: 10.1146/annurev-clinpsy-050212-185510
- Van der Maas, H. L. J., Dolan, C. V., Grasman, R. P. P. P., Wicherts, J. M., Huizenga, H. M., & Raijmakers, M. E. J. (2006). A dynamical model of general intelligence: The positive manifold of intelligence by mutualism. Psychological Review, 113, 842–861. doi: 10.1037/0033-295X.113.4.842
- van Borkulo, C. D., Boschloo, L., Borsboom, D., Penninx, B. W. J. H., & Schoevers, R. A. (2015). Association of symptom network structure and the course of depression. JAMA Psychiatry, 72, 1219–1226. doi: 10.1001/jamapsychiatry.2015.2079.
- Voelkle, M., Brose, A., Schmiedek, F., & Lindenberger, U. (2014). Toward a unified framework for the study of between-person and within-person structures: Building a bridge between two research paradigms. Multivariate Behavioral Research, 49, 193–213. doi: 10.1080/00273171.2014.889593
- Voelkle, M., Oud, J. H. L., Davidov, E., & Schmidt, P. (2012). An SEM approach to continuous time modeling of panel data: Relating authoritarianism and anomia. Psychological Methods, 17, 176–192. doi: 10.1037/a0027543
- Walls, T. A., & Schafer, J. L. (Eds.). (2006). Models for intensive longitudinal data. New York, NY: Oxford University Press.
- Wang, L., Hamaker, E. L., & Bergman, C. S. (2012). Investigating inter-individual difference in short-term intra-individual variability. Psychological Methods, 17, 567–581. doi: 10.1037/a0029317
- Wang, L., & Maxwell, S. E. (2015). On disaggregating between-person and within-person effects with longitudinal data using multilevel models. Multivariate Behavioral Research, 20, 63–83. doi: 10.1037/met0000030
- Watson, D., Clark, L. A., & Tellegen, A. (1988). Development and validation of brief measures of positive and negative affect: The PANAS scales. Journal of Personality and Social Psychology, 54, 1063–1070. doi: 10.1037//0022-3514.54.6.1063
- Wichers, M. C., Barge-Schaapveld, D. G. C. M., Nicolson, N. A., Peeters, F., de Vries, M., Mengelers, R., & van Os, J. (2009). Reduced stress-sensitivity or increased reward experience: The psychological mechanism of response to antidepressant medication. Neuropsychopharmacology, 34, 923–931. doi: 10.1038/npp.2008.66
- Zhang, Z., Hamaker, E. L., & Nesselroade, J. R. (2008). Comparisons of four methods for estimating a dynamic factor model. Structural Equation Modeling: A Multidisciplinary Journal, 15, 377–402. doi: 10.1080/10705510802154281