ABSTRACT
Objectives
Treatment for adults diagnosed with binge-eating disorder (BED) includes psychotherapy and/or pharmacotherapy and aims to reduce the frequency of binge-eating episodes and disordered eating, improve metabolic-related issues and reduce weight, and address mood symptoms. Data describing real-world treatment patterns are lacking; therefore, this study aims to characterize real-world treatment patterns among patients with BED.
Methods
This retrospective study identified adult patients with BED using natural language processing of clinical notes from the Optum electronic health record database from 2009 to 2015. Treatment patterns were examined during the 12 months preceding the BED recognition date and during a follow-up period after BED recognition (1–3 years for most patients).
Results
Among 1042 patients, 384 were categorized as the BED cohort and 658, who met less stringent criteria, were categorized as probable BED. In the BED cohort, mean ± SD age was 45.2 ± 13.4 years and 81.8% were women (probable BED, 45.9 ± 12.8 years, 80.2%). A greater percentage of patients in the BED cohort were prescribed pharmacotherapy (70.6% [probable BED, 66.9%]) than received/discussed psychotherapy (53.1% [probable BED, 39.2%]) at baseline. In the BED cohort, 54.4% of patients were prescribed antidepressants (probable BED, 52.4%), 25.3% stimulants (probable BED, 20.1%), and 34.4% nonspecific psychotherapy (probable BED, 24.6%) at baseline, with no substantive differences observed during follow-up. Low percentages of patients in the BED cohort received/discussed cognitive behavioral therapy at baseline (12.5% [probable BED, 9.0%) or during follow-up (13.0% [probable BED, 8.8%). Among patients with ≥1 psychotherapy visit, the mean ± SD number of visits in the BED cohort was 1.2 ± 5.9 at baseline (probable BED, 1.7 ± 7.3) and 2.2 ± 7.7 during follow-up (probable BED, 2.6 ± 7.7).
Conclusion
This cohort of patients with BED was treated more frequently with pharmacotherapy than psychotherapy. These data may help inform strategies for reducing differences between real-world treatment patterns and evidence-based recommendations.
1. Introduction
Binge-eating disorder (BED) was first recognized as a distinct eating disorder in the Diagnostic and Statistical Manual of Mental Disorders, Fifth Edition (DSM-5). BED is characterized by the consumption of a larger quantity of food over a particular time frame than most people would consume under similar circumstances, a sense of lack of control over eating during the episode, and marked distress regarding binge eating [Citation1]. A diagnosis of BED requires that, on average, binge eating occurs at least once a week for 3 months; is not associated with the recurrent use of inappropriate compensatory behavior, such as purging or restriction; and does not occur exclusively during the course of bulimia nervosa or anorexia nervosa [Citation1]. The lifetime prevalence of BED is estimated to be 1.9%, with a 12-month persistence rate of 44.3% (95% CI, 38.7%–50.0%) and median episode length of 4.3 (interquartile range [IQR], 1.0–11.7) years [Citation2]. Less than 40% of patients with BED seek treatment for an eating disorder [Citation2].
Binge-eating disorder treatment trials focus on several outcomes: reduction of the frequency of binge-eating episodes and disordered eating, improved metabolic-related issues, weight reduction, and management of mood symptoms [Citation3]. Current treatment practices include psychotherapy, pharmacotherapy, or a combination of these 2 approaches. Multiple behavioral therapies have been used in the treatment of BED [Citation3–6], including nutritional counseling [Citation7–9] and cognitive behavioral therapy (CBT) [Citation5–7]. CBT is among the most well-studied treatments for BED [Citation5–7]. Guidelines suggest that CBT programs should typically consist of approximately 16 sessions [Citation7]. In a systematic review, therapist-led CBT was associated with greater rates of remission from binge eating versus waitlist controls (58.8% vs 11.2%) across 4 trials that used 14 to 16 treatment sessions [Citation3]. Furthermore, qualitative analyses from the same systematic review indicated that partially therapist-led CBT and guided self-help CBT increased binge-eating abstinence and reduced binge-eating frequency, and therapist-led CBT and structured self-help CBT reduced binge-eating frequency [Citation3]. In a meta-analysis comparing CBT (approximately 10‒16 sessions) to behavioral weight loss therapy (approximately 16–20 sessions), greater reductions in being-eating frequency were observed with CBT across 4 studies [Citation10]. However, CBT may not be readily available to all patients with BED and, when available, may not be administered in a standardized manner that is consistent with evidence-based practices [Citation11]. In addition to CBT, other evidence-based psychotherapy treatments for BED include interpersonal psychotherapy (IPT) and dialectical behavior therapy (DBT) [Citation6]. The goal of IPT is for patients to acknowledge negative effects of BED on interpersonal relationships in order to manage negative feelings without food, and similar long-term outcomes have been obtained with IPT compared with CBT. The goal of DBT is for patients to learn to regulate emotions and use more adaptive coping mechanisms, but data on the long-term efficacy of DBT compared with CBT are lacking.
At this time, the only pharmacotherapy approved for the treatment of BED by regulatory agencies is lisdexamfetamine dimesylate (US approval granted by the Food and Drug Administration [FDA] in January 2015) [Citation12]. In short-term efficacy studies, lisdexamfetamine produced significantly greater reductions in binge eating than placebo in adults with moderate-to-severe BED [Citation13,Citation14]. In a pair of double-blind, placebo-controlled studies, the mean reductions in binge-eating days per week were significantly greater with lisdexamfetamine (–3.87 and –3.92, respectively) than with placebo (–2.51 and –2.26, respectively) after 12 weeks of treatment [Citation13]. In a maintenance-of-efficacy study, continued treatment with lisdexamfetamine was associated with a significantly greater time to relapse to binge eating than placebo [Citation15]. In these same studies, lisdexamfetamine treatment was associated with greater reductions in body weight than placebo [Citation13–15]. Although not approved for the treatment of BED, other pharmacotherapies, including antidepressants, anticonvulsants, antipsychotics, and antiobesity agents, have shown efficacy versus placebo in the treatment of BED [Citation16–18]. Some agents, such as topiramate and zonisamide, also resulted in statistically significant weight loss compared with placebo [Citation16]. Nevertheless, the use of topiramate is limited because of its adverse event profile [Citation18]. Antidepressants, which as a class are among the most used nonapproved pharmacotherapies for BED [Citation16], have generally been associated with greater improvement than placebo in a variety of binge-eating outcomes, including reduced frequency of binge-eating days and binge-eating episodes and increased rates of abstinence from binge eating, but have not generally been associated with weight loss [Citation17,Citation18].
Although there have been many clinical investigations of potential therapies for BED [Citation18], data describing real-world treatment patterns in adults diagnosed with BED are lacking. The purpose of this study was to characterize treatment patterns in a cohort of patients identified as having BED in a real-world setting.
2. Materials and methods
2.1 Data source
This retrospective cohort study identified patients with BED using natural language processing (NLP) of clinical notes from 1 January 2009, to 30 September 2015. Previously published studies have shown that NLP of electronic health record (EHR) data is useful for identifying patients, extracting relevant data, and providing quality metrics across several disorders and conditions [Citation19–24], including BED [Citation25,Citation26]. The data were sourced from the Optum EHR database, which is a patient-level database that integrates electronic medical records (eg, medical claims, prescriptions, and practice management data) from US providers and hospital networks. Data from 2007 through 2014 for >36.5 million patients are included in the database, of which 18.4 million patients had ≥1 encounter in 2014. Information for the database is collected from >92,000 providers and 195 hospitals, representing 34 electronic medical record–based providers and hospital networks. The patient population in the Optum EHR database is geographically diverse. Compared with the overall US population, however, the Optum EHR database includes a smaller proportion of individuals <18 years of age and a larger proportion aged >65 years, a smaller proportion of patients from the South and West and a greater proportion of patients from the Midwest, and a greater proportion of uninsured patients.
For the current analyses, all applicable laws and regulations were followed to protect personal health information of patient-level data as specified in the Health Insurance Portability and Accountability Act. The protocol was reviewed by an Optum ethics review committee to ensure the data could not be used to reidentify patients. Because the analyses used deidentified data, an institutional review board review was not required, and informed consent was not required to be obtained from patients. All study procedures followed the principles outlined in the Declaration of Helsinki.
2.2 Patient identification
To be included in the study, patients were required to be aged ≥18 years old. In addition, patients were required to have had ≥1 outpatient encounter with a primary care provider or a healthcare provider who could recognize BED during the 12-month baseline period preceding the cohort entry date (defined as the date of BED recognition) and to have been a patient of a provider who contributed notes to the Optum EHR database. The baseline period included the 12 months before and including the BED cohort entry date.
An International Classification of Diseases (ICD) code for BED was introduced in 2016 in ICD-10. Without a specific code for BED in ICD-9 (previously under the general ICD-9 code 307.50 [Eating Disorder, Not Otherwise Specified]) during the timeframe that this study was conducted, patients with BED were identified using an algorithm comprised of NLP terms. The NLP system was used to extract and organize concepts, attributes, sentiments, and modifiers related to BED from free text clinical notes. The system was developed using vocabulary from the Unified Medical Language System, which includes multiple medical dictionaries (eg, the Logical Observation Identifiers Names and Codes, the Systematized Nomenclature of Medicine–Clinical Terms, and RxNorm [a listing of generic and branded drugs]). The NLP system is updated regularly and supplemented with new terms and information as refinements are identified. The architecture of the NLP system is based on the OASIS Unstructured Information Management Architecture [Citation27] and is similar to other previously described systems [Citation28]. The types of NLP concepts included medications; clinical measurements; diagnostic and therapeutic procedures; and signs, diseases, and symptoms. Modifiers of the NLP concepts included sentiments (eg, negations, affirmations), descriptive attributes (eg, stage, grade, severity, duration), and the notes section (eg, medical history, history of current illness, assessments, plans).
For the current study, separate algorithms () used combinations of NLP terms to identify 2 cohorts: a BED cohort and a probable BED cohort. A third cohort – the all BED cohort – represented all identified patients (the BED cohort + the probable BED cohort). Algorithm creation involved searching NLP fields for terms that represented synonyms of BED (eg, compulsive eating, food addiction) and its symptoms (eg, loss of control, distress, secretive eating), attributes (eg, chronic), and the formal identification of affirmations and negations (eg, negation of purging, negation of eating disorder; ). The BED cohort met criteria that were more stringent (eg, having ≥2 affirmations of BED within a single note or 1 affirmation of BED plus an affirmation of binge eating; ). The probable BED cohort met less stringent criteria, such as having only 1 affirmation of BED in a note or ≥2 neutral mentions of BED (). A neutral mention of BED was one that did not positively or negatively affirm the presence of BED. Patients with an ICD-9 code for bulimia nervosa (code 307.51) were excluded from the study population before application of the BED algorithms. The date of BED recognition was the earliest date in the study period when the patient met the BED algorithm criteria. Hence, we identified both prevalent (patients with existing BED) and incident BED (patients with a new diagnosis during the study period).
Figure 1. Final BED algorithm. BED = binge-eating disorder; N = no; Y = yes. Figure was reproduced with permission from Bulik CM, et al. Suicidality risk among adults with binge-eating disorder. Suicide Life Threat Behav. 2021; doi: 10.1111/sltb.12768 [Epub ahead of print] [Citation29] Copyright © 2021 The Authors. Suicide and Life‐Threatening Behavior published by Wiley Periodicals LLC on behalf of American Association of Suicidology.
![Figure 1. Final BED algorithm. BED = binge-eating disorder; N = no; Y = yes. Figure was reproduced with permission from Bulik CM, et al. Suicidality risk among adults with binge-eating disorder. Suicide Life Threat Behav. 2021; doi: 10.1111/sltb.12768 [Epub ahead of print] [Citation29] Copyright © 2021 The Authors. Suicide and Life‐Threatening Behavior published by Wiley Periodicals LLC on behalf of American Association of Suicidology.](/cms/asset/3ba3ed3d-b736-44aa-8783-f490a25faaf4/ipgm_a_2018255_f0001_b.gif)
Table 1. NLP Concepts Included in Electronic Health Record Algorithms.
Algorithms and sequential listings of NLP terms derived from each clinical note from a subset of patients were reviewed by clinical experts so that the algorithms could be refined in an iterative process. Deidentified clinical notes for 250 patients were sought and reviewed by 3 external clinicians familiar with BED (1 licensed and board-certified psychiatrist; 2 licensed psychologists) to confirm the presence of BED and to validate the EHR algorithms. The external clinicians did not receive any specific training but collectively had 27 years of postgraduate experience in psychiatry/psychology. Positive predictive values (PPVs) were calculated as the number of algorithm-identified patients with confirmed BED divided by the total number of algorithm-identified patients. The identification of patients who received behavioral therapy involved searching NLP fields for terms representing various forms of psychotherapy (eg, CBT, psychotherapy, group therapy; ).
2.3 Data presentation
These analyses report demographic characteristics, lifestyle characteristics, and comorbidities during the 12-month baseline period preceding BED recognition for all study cohorts. Treatment characteristics during the 12-month baseline period preceding BED recognition and during the follow-up period are reported. The follow-up period included the time from BED recognition until departure from the provider group or end of follow-up (30 September 2015). Operational definitions of demographics, lifestyle characteristics, and comorbidities are included in Supplementary Table 1. Receipt of prescriptions for pharmacotherapy was identified using drug class and generic name; receipt of/discussions about psychotherapy were identified using NLP terms. NLP terms for concepts related to CBT and other general types of therapy terms were identified and grouped into the following categories: CBT, behavior therapy, nonspecific therapy, group therapy, and family therapy. Using concept modifiers, patients with affirmations of these flags were further classified as having ‘received’ versus ‘discussed’ psychotherapy. A post hoc analysis was conducted to determine the number of patients who received a first prescription for lisdexamfetamine before and after the date of approval by the FDA for the treatment of BED, stratified by presence or absence of comorbid attention-deficit/hyperactivity disorder (ADHD) at baseline.
Two indices of overall health were calculated: the Framingham Risk Score (FRS) [Citation30] to assess the 10-year risk of cardiovascular disease and the Charlson Comorbidity Index (CCI) [Citation31,Citation32] to assess the 1-year risk of mortality (see Supplementary Table 1). PPVs with 95% CIs are reported for the final BED algorithms and for algorithm-identified behavioral therapies.
Patient attributes were characterized using descriptive statistics (means with SDs for continuous variables, frequencies and percentages for categorical variables). Inferential statistical analyses were not conducted because the goal of the study was to provide a descriptive characterization of how a cross-section of patients with BED are medically managed in a real-world setting.
3. Results
3.1 Algorithm performance
The PPVs were 83% (95% CI, 61% to 95%) for BED and 64% (95% CI, 44% to 81%) for probable BED. PPVs for algorithm-identified psychotherapies are reported in based on review of a sample of notes from 120 patients by the clinicians (1 psychiatrist, 2 psychologists); for CBT, the PPV of algorithm-identified CBT was 50% (95% CI, 21% to 79%), indicating only half of patients classified as receiving or discussing CBT actually had CBT. No NLP terms specific to interpersonal psychotherapy or behavioral weight loss were identified.
Table 2. Positive Predictive Values (PPVs) for Algorithm-Identified Behavioral Therapies.
3.2 Demographic and clinical characteristics
A total of 1042 patients with BED were identified; 384 patients were included in the BED cohort and 658 in the probable BED cohort. Baseline demographics and lifestyle characteristics are summarized in . Across all cohorts, most patients were women and were White. Approximately one-third of the patients were ever smokers (). Approximately one-quarter of the patients had a sedentary level of physical activity; data on physical activity level were missing for 50% to 61% of patients across cohorts (). The frequencies of comorbidities observed during the baseline period are summarized in . The mean ± SD FRS during the baseline period was 9.0 ± 9.6 (n = 276) in the BED cohort (probable BED, 9.3 ± 10.5 [n = 435]; all BED, 9.2 ± 10.2 [N = 711]). The mean ± SD CCI score during the baseline period was 1.5 ± 2.7 (n = 384) in the BED cohort (probable BED, 1.3 ± 2.4 [n = 658]; all BED, 1.4 ± 2.6 [N = 1042]).
Figure 2. Comorbidities reported by ≥5% of patients during the baseline period*†.
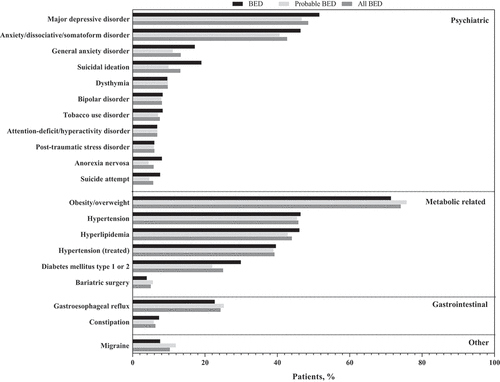
Table 3. Demographics and Lifestyle Characteristics During the Baseline Period*.
3.3 Treatment characteristics
In the BED cohort, 70.6% of patients (271/384) were prescribed any pharmacotherapy (probable BED, 66.9% [440/658]; all BED, 68.2% [711/1042]) during the baseline period (). Among the pharmacotherapies (), 54.4% (209/384) of the BED cohort were prescribed antidepressants (probable BED, 52.4% [345/658]; all BED, 53.2% [554/1042]) and 25.3% (97/384) were prescribed stimulants (probable BED, 20.1% [132/658]; all BED, 22.0% [229/1042]). The most frequently prescribed antidepressants in the BED cohort were selective serotonin reuptake inhibitors (SSRIs) followed by atypical antidepressants (). Among stimulants, amphetamine-like stimulants were prescribed more frequently than nonamphetamine-like stimulants ().
Figure 3. Types of psychotherapy received or discussed or pharmacotherapy prescribed during the baseline period (A) or follow-up period (B)*.
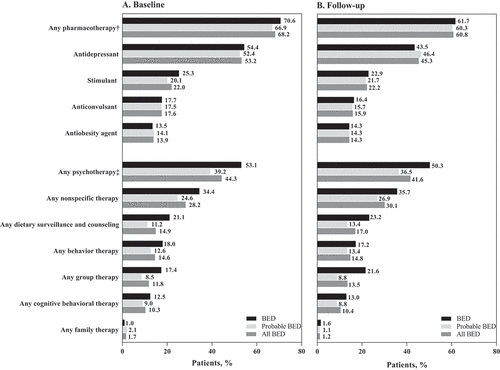
Table 4. Specific Pharmacotherapies Prescribed During the Baseline and Follow-Up Periods*.
Similar pharmacotherapy treatment patterns were observed during the follow-up period. In the BED cohort, 61.7% of patients (237/384) were prescribed any pharmacotherapy (probable BED, 60.3% [397/658]; all BED, 60.8% [634/1042]; ). Among the pharmacotherapies (), 43.5% (167/384) of the BED cohort were prescribed antidepressants (probable BED, 46.4% [305/658]; all BED, 45.3% [472/1042]) and 22.9% (88/384) were prescribed stimulants (probable BED, 21.7% [143/658]; all BED, 22.2% [231/1042]). The most frequently prescribed antidepressants in the BED cohort continued to be SSRIs and atypical antidepressants (). The percentage of patients prescribed any pharmacotherapy during follow-up was numerically lower than during the baseline period, with the largest magnitude decrease being observed for antidepressants ( and ).
Lisdexamfetamine, a stimulant medication for ADHD, received US regulatory approval for the treatment of BED on 30 January 2015, 8 months before the end of the study period for this analysis (30 September 2015). A post hoc analysis of lisdexamfetamine prescriptions before and after approval for BED was carried out to assess for any prescription bias during this 8-month overlap window. Over the course of the entire study period, 9.1% of patients (95/1042) had at least one lisdexamfetamine prescription (see Supplementary Table 2). Before the lisdexamfetamine approval date, 1.9% of patients (20/1,042) had their earliest lisdexamfetamine prescription, of whom 65.0% (13/20) had an ADHD diagnosis at baseline. Following lisdexamfetamine approval, 7.2% of patients (75/1,042) had their earliest lisdexamfetamine prescription, of whom 17.3% (13/75) had an ADHD diagnosis at baseline (see Supplementary Table 2).
Psychotherapy was received by or discussed by 53.1% (204/384) of patients in the BED cohort (probable BED, 39.2% [258/658]; all BED, 44.3% [462/1042]) during the baseline period (). In the BED cohort, 34.4% of patients (132/384) received or discussed nonspecific therapy (probable BED, 24.6% [162/658]; all BED, 28.2% [294/1042]), 21.1% (81/384) received dietary surveillance and counseling (probable BED, 11.2% [74/658]; all BED, 14.9% [155/1042]), and 18.0% (69/384) received or discussed any behavior therapy (probable BED, 12.6% [83/658]; all BED, 14.6% [152/1042]) during the baseline period (). In the BED cohort, 12.5% of patients (48/384) received or discussed CBT (probable BED, 9.0% [59/658]; all BED, 10.3% [107/1042]; ). provides a summary of each psychotherapy type as a function of whether it was discussed or received during the baseline period. The mean ± SD number of psychotherapy visits among patients with ≥1 visit was 1.2 ± 5.9 in the BED cohort, 1.7 ± 7.3 in the probable BED cohort, and 1.5 ± 6.8 in the all BED cohort. Among patients with ≥1 psychotherapy visit, BED patients had a median of 2 (IQR, 1–6) visits, probable BED patients had a median of 4 (IQR, 1–8) visits, and all BED patients had a median of 3 (IQR, 1–8) visits.
Table 5. Psychotherapies Received/Discussed During the Baseline and Follow-Up Periods*.
Similar psychotherapy patterns were observed during the follow-up period. Psychotherapy was received by or discussed by 50.3% (193/384) of patients in the BED cohort (probable BED, 36.5% [240/658]; all BED, 41.6% [433/1042]) during the follow-up period (). In the BED cohort, 35.7% of patients (137/384) received or discussed nonspecific therapy (probable BED, 26.9% [177/658]; all BED, 30.1% [314/1042]) and 17.2% (66/384) received or discussed any behavior therapy (probable BED, 13.4% [88/658]; all BED, 14.8% [154/1042]) during the baseline period (). In the BED cohort, 13.0% of patients (50/384) received or discussed CBT (probable BED, 8.8% [58/658]; all BED, 10.4% [108/1042]) (). provides a summary of each psychotherapy type as a function of whether it was discussed or received during the follow-up period. The mean ± SD number of psychotherapy visits among patients with ≥1 visit was 2.2 ± 7.7 in the BED cohort, 2.6 ± 7.7 in the probable BED cohort, and 2.4 ± 7.7 in the all BED cohort. Among patients with ≥1 psychotherapy visit, BED patients had a median of 2 (IQR, 1–6) visits, probable BED patients had a median of 3 (IQR, 1–9) visits, and all BED patients had a median of 3 (IQR, 1–8) visits. Between the baseline and follow-up periods, there were no substantive changes in the percentages of patients discussing or receiving psychotherapy or in the mean number of psychotherapy visits for patients.
4. Discussion
Our findings indicate that in a cohort of patients identified as having BED, the percentage of patients prescribed pharmacotherapy (either on-label or off-label) for BED was larger than the percentage of patients receiving or having discussed psychotherapy. The most frequently prescribed pharmacotherapies were antidepressants and stimulants. The most frequently received or discussed psychotherapy was nonspecific therapy; very few participants received or discussed CBT. There was a decrease in the percentage of patients prescribed pharmacotherapy during the follow-up period relative to the baseline period, but no substantive change in the percentage of patients who received or discussed psychotherapy was observed across study periods. There was a small increase in the mean number of psychotherapy visits during the follow-up period relative to the baseline period, but in both periods the mean number of visits was low.
In this cohort, antidepressants were the most frequently prescribed class of medications during the baseline and follow-up periods, with SSRIs being the most frequently prescribed type of antidepressant. Given that antidepressants have been assessed for their utility in the treatment of BED [Citation16,Citation18], the relatively high prescription frequency for antidepressants in this study population is not surprising. The prescription frequency for antidepressants is also expected because of the high percentage of patients with comorbid major depressive disorder (MDD) in the current study.
Stimulants were the second most frequently prescribed medication class in this patient population at baseline and during the follow-up period. This was also not unexpected because lisdexamfetamine is the only regulatory agency‒approved pharmacotherapy for BED [Citation12]. Because the initial US approval of lisdexamfetamine for BED occurred on 30 January 2015, the approval of lisdexamfetamine overlapped with the current study only for its last 8 months. FDA approval for the treatment of BED resulted in an increase in the proportion of study patients receiving first lisdexamfetamine prescriptions, from 1.9% before the approval date to 7.2% after the approval date. Prior to approval for BED treatment, most patients receiving lisdexamfetamine had a baseline diagnosis of ADHD, suggesting that lisdexamfetamine was prescribed for comorbid ADHD; additionally, there may have been some off-label use of lisdexamfetamine or other amphetamine-based medications for BED treatment. Following approval, 17.3% (13/75) of patients prescribed lisdexamfetamine had a baseline diagnosis of ADHD and may have received lisdexamfetamine for ADHD rather than BED. In addition, availability of a novel BED medication may have stimulated interest and an early increase in prescriptions, leading to a greater proportion of BED patients receiving lisdexamfetamine during the last 8 months of the study period. Nevertheless, the percentage of patients with a lisdexamfetamine prescription was low (7.2% with a first prescription postapproval, including those who may have received lisdexamfetamine for ADHD). Therefore, approval of lisdexamfetamine during the 8-month overlap window of the study period was unlikely to change the overall interpretation of this analysis. Furthermore, the goal of the current study was not to examine lisdexamfetamine use specifically but to more broadly understand real-world treatment patterns for BED. Further studies will be necessary to more fully assess typical lisdexamfetamine use among patients with BED.
Across all cohorts, approximately 39% to 53% of participants received or discussed any form of psychotherapy during the baseline period and 37% to 50% of participants received or discussed any form of psychotherapy during the follow-up period. Nonspecific therapy was the most frequently reported psychotherapy received or discussed. More importantly, a very low percentage of patients (<15%) received or discussed CBT during the baseline period or during the follow-up period. The lack of use of CBT in this population is consistent with the low number of reported psychotherapy visits across cohorts during the baseline period (mean, 1.2–1.7; median, 2–4) and during the follow-up period (mean, 2.2–2.6; median, 2–3). This number of visits would be considered inadequate according to current treatment guidelines, which typically recommend approximately 16 sessions of CBT over 4 months [Citation7], and based on analyses that have demonstrated the efficacy of CBT for the treatment of BED [Citation3,Citation10]. These results may be limited by the length of follow-up. The majority of patients entered the study in 2012 or later and the study period ended in 2015; therefore, most patients had 1 to 3 years of follow-up.
These findings, especially those related to CBT, are unexpected. CBT is considered to be among the most studied and well-established treatments for BED [Citation5,Citation6], and the American Psychiatric Association [Citation33] and National Institute for Health and Care Excellence [Citation7] recommend that some form of CBT be incorporated into a patient’s BED treatment plan. Therefore, it was expected that CBT would have been used more frequently in this cross-section of patients. Access to care is likely to be an important overarching factor in determining the availability of treatment for BED. Having higher quality insurance and living closer to an academic medical center would be expected to increase the probability of accessing psychotherapies such as CBT, whereas having less insurance coverage and not living near appropriate resources would be expected to increase the probability of being prescribed medication. Of note, patients in this study were predominantly White, and high rates of BED have previously been reported in African Americans [Citation34]. Because race and ethnicity are associated with access to care, the treatment patterns observed in this study may have limited applicability in African American and other minority populations. Furthermore, many patients with BED seek care from a primary care physician [Citation17], and these physicians may only be able to offer limited treatments directly to the patient. It should also be considered that a provider in the EHR capture network may have recognized BED, but a provider outside of the network may have given psychotherapy (and this outside network treatment would not have been captured in this study). It is unlikely that patients in the current population tried, but did not respond to, psychotherapy before the time covered by this study because the mean number of psychotherapy visits among patients with ≥1 visit was less than 3.
With regard to treatment patterns, it is also worth noting that increases in the percentages of patients being treated were not generally observed during the period that followed the recognition of BED relative to baseline. Rather, the percentage of patients prescribed any pharmacotherapy decreased rom 71% during the baseline period to 62% during the follow-up period in the BED cohort (probable BED, from 67%‒60%; all BED, from 68%‒61%). Similar decreases of a smaller magnitude were observed for psychotherapy treatments. The mean number of psychotherapy visits did increase slightly during the follow-up period relative to the baseline period, but the increase of approximately 1 visit is not likely to be clinically meaningful.
Patients identified as having BED also had a high comorbidity burden during the baseline period. The most frequently reported psychiatric comorbidities were MDD and anxiety/dissociative/somatoform disorder, and most frequently reported metabolic-related comorbidities were obesity/overweight, hypertension, and hyperlipidemia. The observation of a high burden of psychiatric [Citation35–41], metabolic [Citation2,Citation42], and gastrointestinal [Citation43,Citation44] comorbidities in patients with BED is consistent with previously published findings.
These findings should be interpreted in light of several limitations. First, EHR data have utility for examining healthcare outcomes and treatment patterns, but these databases are limited in that they have been developed for patient management and not clinical research. Second, if the analyses captured a greater percentage of patients with moderate or severe BED symptoms rather than mild BED symptoms, these findings may not be representative of all individuals with BED. Third, the PPVs of the BED and psychotherapy algorithms were not 100%, so some of the patients included in the cohorts likely did not have BED or did not receive or discuss psychotherapy. Fourth, the diagnostic codes used to assess comorbidities do not necessarily represent the presence of a condition, because the code could be incorrect or associated with a condition that was later ruled out. Fifth, pharmacotherapy and psychotherapy may have been prescribed to treat patient comorbidities other than BED. Lastly, temporality between the onset of BED and the onset of comorbidities cannot be determined because there is likely a delay between the onset of BED symptoms and the recognition of BED by a healthcare provider. Therefore, baseline data may have been affected by the onset of BED, which precludes these results from being interpreted as causal relationships.
5. Conclusion
These data show that treatment patterns in patients identified as having BED based on NLP of clinical notes were characterized by greater percentages of BED patients being prescribed pharmacotherapy – most frequently an antidepressant or stimulant – than receiving or discussing psychotherapy. Although approximately 50% of patients received or discussed psychotherapy during the baseline and follow-up periods, the number of psychotherapy visits was low (on average less than 3) and a small percentage of patients (<15%) received or discussed CBT. These findings were unexpected and could be partially the result of patients with BED seeking care from a primary care physician [Citation17], who may only be able to offer limited treatment options. Another explanation is that some patients may have been recognized as having BED by a provider in the EHR capture network and been treated with psychotherapy by a provider outside of the network. Among patients with BED prescribed pharmacotherapy, a relatively low percentage was prescribed stimulants. This finding could be partially related to the timing of lisdexamfetamine approval for the treatment of BED in the US relative to when this study was conducted, as we did observe a small increase in the proportion of BED patients receiving lisdexamfetamine after FDA approval. Future studies should focus on clarifying the effect of early detection and treatment of BED on associated comorbidities and healthcare use and on identifying the most successful treatment strategies to improve evidence-based recommendations for treatment.
List of Abbreviations
Declaration of funding
This research was funded by Shire Development LLC, a member of the Takeda group of companies, Lexington, MA, USA.
Disclosure of financial/other conflicts of interest
WMS is an employee of Shire, a member of the Takeda group of companies, and holds Takeda stock. MLB is an employee of Optum (a UnitedHealth Group company), which was funded to conduct this study by Shire, a member of the Takeda group of companies. CMB is a grant recipient and advisory board member for Shire, a consultant for Idorsia, and an author for Pearson. She received money from Shire Pharmaceuticals LLC, a member of the Takeda group of companies, for work that is not part of the current manuscript, including for activities that may include consulting, service on advisory boards, and giving speeches. JDS is an employee of Optum (a UnitedHealth Group company), which was funded to conduct this study by Shire, a member of the Takeda group of companies, and owns UnitedHealth Group company stock and/or stock options. The authors have no other relevant conflicts of interest to disclose. Peer reviewers on this manuscript have no relevant financial or other relationships to disclose.
Declarations
Ethics Approval and Consent to Participate
The protocol was reviewed by an Optum ethics review committee to assure the data could not be used to reidentify patients. Because the analyses used deidentified data, an institutional review board review was not required and informed consent was not required to be obtained from patients. All study procedures followed the principles outlined in the Declaration of Helsinki.
Author Contributions
WMS, CMB, and JDS contributed to the design of the study and the interpretation of the data. MLB contributed to the design of the study, the data analysis, and the interpretation of the data. All authors participated in manuscript preparation, provided critical review of each draft, read and approved the final manuscript, and made the decision to submit to Postgraduate Medicine.
Supplemental Material
Download MS Word (94.8 KB)Acknowledgments
Under direction of the authors, writing assistance was provided by Srividya Ramachandran, PhD, and Craig Slawecki, PhD, employees of ICON (North Wales, PA, USA). Shire Development LLC, a member of the Takeda group of companies, Lexington, MA, USA provided funding to ICON for support in writing and editing this manuscript.
Data Availability Statement
The data that support the findings of this study are available from Optum (Boston, MA, USA) but restrictions apply to the availability of these data, which were used under license for the current study, and so are not publicly available. Data are however available from the authors upon reasonable request and with permission of Optum (Boston, MA, USA).
Supplemental data
Supplemental data for this article can be accessed here
References
- American Psychiatric Association. Diagnostic and Statistical Manual of Mental Disorders. Fifth Edition. Washington DC: American Psychiatric Association; 2013.
- Kessler RC, Berglund PA, Chiu WT, et al. The prevalence and correlates of binge eating disorder in the World Health Organization World Mental Health surveys. Biol Psychiatry. 2013;73(9):904–914.
- Brownley KA, Berkman ND, Peat CM, et al. Binge-eating disorder in adults: a systematic review and meta-analysis. Ann Intern Med. 2016;165(6):409–420.
- Wilson GT, Wilfley DE, Agras WS, et al. Psychological treatments of binge eating disorder. Arch Gen Psychiatry. 2010;67(1):94–101.
- Wilson GT, Grilo CM, Vitousek KM. Psychological treatment of eating disorders. Am Psychol. 2007;62(3):199–216.
- Iacovino JM, Gredysa DM, Altman M, et al. Psychological treatments for binge eating disorder. Curr Psychiatry Rep. 2012;14(4):432–446.
- National Institute for Health and Care Excellence. Eating disorders: recognition and treatment (NICE guideline NG69) [Internet]. 2017 [cited 2020 Sept 9]. Available from: https://www.nice.org.uk/guidance/ng69/resources/eating-disorders-recognition-and-treatment-pdf-1837582159813
- Davison KM, Cairns J, Selly C, et al. The Role of Nutrition Care for Mental Health Conditions [Internet]. Dietitians of Canada; 2012 [cited 2020 Nov 16]. Available from: https://www.dietitians.ca/mentalhealth
- Davison KM, Ng E, Chandrasekera U, et al. Nutrition and Mental Health: Therapeutic Approaches [Internet]. Dietitians of Canada; 2012 [cited 2020 Sept 9]. Available from: https://www.dietitians.ca/DietitiansOfCanada/media/Documents/Resources/Nutrition-and-Mental-Health-3.pdf?ext=.pdf
- Palavras MA, Hay P, Filho CA, et al. The efficacy of psychological therapies in reducing weight and binge eating in people with bulimia nervosa and binge eating disorder who are overweight or obese—a critical synthesis and meta-analyses. Nutrients. 2017;9(3):299.
- Brown CE, Nicholson Perry K. Cognitive behavioural therapy for eating disorders: how do clinician characteristics impact on treatment fidelity? J Eat Disord. 2018;6(19): Epub. doi:10.1186/s40337-018-0208-0.
- Vyvanse® (lisdexamfetamine dimesylate). Full Prescribing Information [package insert]. Lexington MA: Shire US Inc.; 2017.
- McElroy SL, Hudson J, Ferreira-Cornwell MC, et al. Lisdexamfetamine dimesylate for adults with moderate to severe binge eating disorder: results of two pivotal phase 3 randomized controlled trials. Neuropsychopharmacology. 2016;41(5):1251–1260.
- McElroy SL, Hudson JI, Mitchell JE, et al. Efficacy and safety of lisdexamfetamine for treatment of adults with moderate to severe binge-eating disorder: a randomized clinical trial. JAMA Psychiatry. 2015;72(3):235–246.
- Hudson JI, McElroy SL, Ferreira-Cornwell MC, et al. Efficacy of lisdexamfetamine in adults with moderate to severe binge-eating disorder: a randomized clinical trial. JAMA Psychiatry. 2017;74(9):903–910.
- Reas DL, Grilo CM. Pharmacological treatment of binge eating disorder: update review and synthesis. Expert Opin Pharmacother. 2015;16(10):1463–1478.
- Berkman ND, Brownley KA, Peat CM, et al. Management and outcomes of binge-eating disorder. Rockville MD: Agency for Healthcare Research and Quality; 2015. p. 15(16)-EHC030-EF.
- McElroy SL, Guerdjikova AI, Mori N, et al. Overview of the treatment of binge eating disorder. CNS Spectr. 2015;20(6):546–556.
- Fonferko-Shadrach B, Lacey AS, Roberts A, et al. Using natural language processing to extract structured epilepsy data from unstructured clinic letters: development and validation of the ExECT (EXtraction of Epilepsy Clinical Text) system. BMJ Open. 2019;9(4):e023232.
- Ramu N, Kolliakou A, Sanyal J, et al. Recorded poor insight as a predictor of service use outcomes: cohort study of patients with first-episode psychosis in a large mental healthcare database. BMJ Open. 2019;9(6):e028929.
- McVay TR, Garrett GC, Celena BP, et al. Natural language processing accurately identifies dysphagia indications for esophagogastroduodenoscopy procedures in a large US integrated healthcare system: implications for classifying overuse and quality measurement. Paper presented at: AMIA Joint Summits on Translational Science Proceedings; Mar 25-28, San Francisco, CA; 2019.
- Patterson BW, Jacobsohn GC, Shah MN, et al. Development and validation of a pragmatic natural language processing approach to identifying falls in older adults in the emergency department. BMC Med Inform Decis Mak. 2019;19(1):138.
- Bozkurt S, Kan KM, Ferrari MK, et al. Is it possible to automatically assess pretreatment digital rectal examination documentation using natural language processing? A single-centre retrospective study. BMJ Open. 2019;9(7):e027182.
- Loda S, Krebs J, Danhof S, et al. Exploration of artificial intelligence use with ARIES in multiple myeloma research. J Clin Med. 2019;8(7):999.
- Bellows BK, LaFleur J, Kamauu AW, et al. Automated identification of patients with a diagnosis of binge eating disorder from narrative electronic health records. J Am Med Inform Assoc. 2014;21(e1):e163–168.
- Bellows BK, DuVall SL, Kamauu AW, et al. Healthcare costs and resource utilization of patients with binge‐eating disorder and eating disorder not otherwise specified in the Department of Veterans Affairs. Int J Eat Disord. 2015;48(8):1082–1091.
- OASIS. Unstructured Information Management Architecture (UIMA) Version 1.0 [Internet]. 2008 [cited 2020 Nov 16]. Available from: https://www.oasis-open.org/committees/download.php/28492/uima-spec-wd-05.pdf
- Savova GK, Masanz JJ, Ogren PV, et al. Mayo clinical Text Analysis and Knowledge Extraction System (cTAKES): architecture, component evaluation and applications. J Am Med Inform Assoc. 2010;17(5):507–513.
- Bulik CM, Bertoia ML, Lu M, et al. Suicidality risk among adults with binge-eating disorder. Suicide Life Threat Behav. 2021;51(5):897–906. Epub ahead of print.
- D’Agostino RB Sr, Vasan RS, Pencina MJ, et al. General cardiovascular risk profile for use in primary care: the Framingham Heart Study. Circulation. 2008;117(6):743–753.
- Deyo RA, Cherkin DC, Ciol MA. Adapting a clinical comorbidity index for use with ICD-9-CM administrative databases. J Clin Epidemiol. 1992;45(6):613–619.
- Romano PS, Roos LL, Jollis JG. Adapting a clinical comorbidity index for use with ICD-9-CM administrative data: differing perspectives. J Clin Epidemiol. 1993;46(10):1075–1079. discussion 81-90.
- Yager J, Devlin M, Halmi K, et al. Guideline watch (August 2012): practice guideline for the treatment of patients with eating disorders, third edition. Focus (Am Psychiatr Publ). 2012;12(4):416–431.
- Goode RW, Cowell MM, Mazzeo SE, et al. Binge eating and binge-eating disorder in Black women: a systematic review. Int J Eat Disord. 2020;53(4):491–507.
- Grilo CM, White MA, Masheb RM. DSM-IV psychiatric disorder comorbidity and its correlates in binge eating disorder. Int J Eat Disord. 2009;42(3):228–234.
- Grilo CM, White MA, Barnes RD, et al. Psychiatric disorder co-morbidity and correlates in an ethnically diverse sample of obese patients with binge eating disorder in primary care settings. Compr Psychiatry. 2013;54(3):209–216.
- Guerdjikova AI, McElroy SL, Kotwal R, et al. Comparison of obese men and women with binge eating disorder seeking weight management. Eat Weight Disord. 2007;12(1):e19–23.
- Jones-Corneille LR, Wadden TA, Sarwer DB, et al. Axis I psychopathology in bariatric surgery candidates with and without binge eating disorder: results of structured clinical interviews. Obes Surg. 2012;22(3):389–397.
- Schulz S, Laessle RG. Associations of negative affect and eating behaviour in obese women with and without binge eating disorder. Eat Weight Disord. 2010;15(4):e287–293.
- Carano A, De Berardis D, Campanella D, et al. Alexithymia and suicide ideation in a sample of patients with binge eating disorder. J Psychiatr Pract. 2012;18(1):5–11.
- Pisetsky EM, Thornton LM, Lichtenstein P, et al. Suicide attempts in women with eating disorders. J Abnorm Psychol. 2013;122(4):1042–1056.
- Hudson JI, Lalonde JK, Coit CE, et al. Longitudinal study of the diagnosis of components of the metabolic syndrome in individuals with binge-eating disorder. Am J Clin Nutr. 2010;91(6):1568–1573.
- Thornton LM, Watson HJ, Jangmo A, et al. Binge-eating disorder in the Swedish national registers: somatic comorbidity. Int J Eat Disord. 2017;50(1):58–65.
- Cremonini F, Camilleri M, Clark MM, et al. Associations among binge eating behavior patterns and gastrointestinal symptoms: a population-based study. Int J Obes. 2009;33(3):342–353.