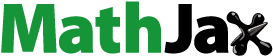
ABSTRACT
Cross-regional mergers and acquisitions (M&A) transfer control and diffuse knowledge across space, which facilitates the integration of business systems. We analyse about 40,000 cross-regional acquisitions in Europe completed between 2003 and 2017 and distinguish innovative and non-innovative M&A. Both types of deals cluster into communities constituted by countries or groups of neighbouring countries. However, an increasing proportion of deals connect different communities, especially for innovative M&A. More populous and richer regions host more acquiring and target companies and thus broker communities. Research and development expenditure and skilled human capital are additional factors favouring brokerage of regions by attracting acquirers.
INTRODUCTION
Cross-border mergers and acquisitions (M&A) are important phenomena of globalization entailing substantial reallocation of economic activities. The global M&A exceeded US$3 trillion between 2014 and 2018, resulting in an unprecedented wave in terms of volumes (Massoudi et al., Citation2017; Platt, Citation2018). Intensifying from the 1990s, an increase in cross-border M&A has been observed between European Union (EU) member states, with a temporary decrease around the financial crisis (Rao & Reddy, Citation2015; Weitzel et al., Citation2014; Wigger, Citation2012). A wide literature has substantially converged in attributing a relevant role in the rise of EU M&A activity to legislative and regulatory steps towards EU monetary and economic integration (Coeurdacier et al., Citation2009; McCarthy & Dolfsma, Citation2015; Moschieri et al., Citation2014). Thus, interregional M&A has indirect consequences on European competitiveness through the processes of economic integration (Krugman & Venables, Citation1990; Rivera-Batiz & Romer, Citation1991). However, besides facilitating knowledge transfer and diffusion (e.g., Albuquerque et al., Citation2019), interregional M&A can also generate new geographical landscapes of production, including the spatial concentration of industries (Boschma & Hartog, Citation2014; Chapman, Citation2003; Colombo & Turati, Citation2014; Zademach & Rodríguez-Pose, Citation2009). Whether M&A spread more prosperity or rather spur regional inequalities is largely unknown (Iammarino et al., Citation2019). Hence, a better understanding is needed of what type of M&A facilitate EU integration in which regions and what regional characteristics favour this process.
In this study, we take a network science perspective to analyse interregional M&A and learn about EU integration. A network science approach has been useful in previous research focusing on innovative collaborations across EU regions to demonstrate that, despite the intensification of international collaborations, the evolution of innovation systems into an integrated European Research Area (ERA) is still incomplete (Chessa et al., Citation2013). Most innovative collaborations are still spatially bounded and fragmented into national innovation systems (Tóth et al., Citation2021). Here we compare over time the spatial boundedness of innovative M&A with that of non-innovative M&A, two types of deals that imply different transfer of knowledge and control (Colombo & Rabiossi, Citation2014) and identify regional determinants that help long-distance transactions across spatially isolated M&A systems. This allows us to contribute to the literature that develops a network brokerage perspective for regions (Martinus et al., Citation2021; Sigler et al., Citation2021) by demonstrating that outstanding broker regions facilitate EU integration into a unified business area.
To achieve these goals, we investigated a new dataset of all completed M&A between 2003 and 2017 involving acquiring and target companies located in the EU, the UK, and the European Free Trade Association (EFTA) countries. Using these data, we were able to identify deals where the target company had a patent portfolio before completion, which we call innovative M&A. We then analysed the spatial patterns of innovative and non-innovative M&A across EU Nomenclature of Territorial Units for Statistics (NUTS)-2 regions, a regional level frequently used in similar analyses (Colombo & Turati, Citation2014; McCarthy & Dolfsma, Citation2015), to interregional M&A. Regions were then grouped with network science tools into communities such that the regions within the communities are densely connected by M&A deals but are sparsely linked across communities. We then applied econometric methods to characterize source and target regions of M&A deals that are brokers between communities and consequently facilitate economic integration.
Our findings indicate that both innovative and non-innovative M&A can be grouped into spatially bounded network communities in which country borders do matter. However, we found a relevant and increasing proportion of cross-community deals, especially for innovative M&A. Overall, we found that more populous and richer regions are the leading intermediaries across communities. The intensity of R&D expenditures in the case of innovative M&A and the presence of a skilled labour force in the case of non-innovative M&A tend to attract acquirers that invest across communities of regions and thus further facilitate European integration through the transfer of control and knowledge.
LITERATURE REVIEW
This study contributes to ongoing discussions on the broker role of regions in facilitating economic interactions between distant actors (Martinus et al., Citation2021; Sigler et al., Citation2021). We investigate processes of spatial transfer of knowledge and control by looking at interregional M&A and uncover how hub regions broker the European integration process. This section reviews previous literature in these domains and highlights the contribution of the study.
M&A as processes of spatial knowledge transfer
The process of M&A is thought to require strong interactions between the involved companies and third parties (Caiazza & Volpe, Citation2015), such as customers, suppliers, partners and competitors, typically mediated by professional experts (Angwin, Citation2001; Das & Kapil, Citation2012). This exchange of control therefore leads to flows of knowledge regarding combinations of management philosophies, business cultures, strategies, goals, working organizations and technological expertise (Bathelt & Henn, Citation2021).
Our aim is to characterize such transfers of control and knowledge embedded in geographical space and thus borrow a spatial learning argument from the fields of economic geography and international business. This literature provides an image of the globalized world economy as a set of locations with ‘local buzz’ (Storper & Venables, Citation2004) connected by ‘global pipelines’ (Bathelt et al., Citation2004). Companies, in particular multinational enterprises, and ultimately people within them, are the key actors shaping such connections, serving as conduits for multidirectional knowledge flows between places (e.g., Cano-Kollmann et al., Citation2016; Crescenzi & Iammarino, Citation2017; Iammarino & McCann, Citation2013; Song, Citation2014). While most interactions take place between geographically close agents, creating in some cases clusters (or buzz) with especially dense activity, cross-regional connections (or pipelines) are key to allow combinations of different knowledge inputs and avoid cognitive lock-in (Boschma, Citation2005; Giuliani & Bell, Citation2005). M&A that involve different locations are an important tool for building pipelines and, eventually, promoting processes of mutual learning and transfer of organizational control across space (Chapman, Citation2003).
Empirical evidence for such knowledge transfer by M&A across space is still limited but growing. Studying the research and development (R&D) activity of French firms acquired by foreign companies, Bertrand (Citation2009) found a positive impact of foreign acquisition on R&D of local providers of target companies. Bruhn et al. (Citation2017) documented positive spillovers of inward and outward foreign M&A with regard to the technological upgrading of Brazilian industries. Albuquerque et al. (Citation2019) showed using firm-level data from 64 countries that foreign M&A activities improve the quality of governance, valuation and productivity of local competitors of the target companies. However, there is a lack of understanding of how such connections impact economic systems by bridging locations that would be otherwise isolated.
A network brokerage approach to interregional M&A
It is widely documented in the economic geography literature that the transfer of control across space through M&A leads to increasing spatial concentration of economic activities in regional hubs (Ashcroft & Love, Citation1993; Colombo & Turati, Citation2014; Chapman, Citation2003; Green, Citation1990). For example, taking a historical perspective, Boschma and Hartog (Citation2014) documented the role of M&A activity in the creation of a financial hub in Amsterdam. Regions emerge as hubs by concentrating control in urban agglomerations mostly from neighbouring or geographically proximate regions (Böckerman & Lehto, Citation2006; Rodríguez-Pose & Zademach, Citation2003; Zademach & Rodríguez-Pose, Citation2009). However, these hubs can also connect regional economic systems by long-distance M&A.
Here we propose a network brokerage approach to address the notion that some regions emerge and stand out as hubs where control and knowledge transfer across distant places. These locations are gateways that surrounding small regions are connected to and that intermediate flows between their local economy and distant places (Derudder et al., Citation2010). More recently, the idea that such cities, regions, or countries are brokers of network flows has gained popularity in research on global networks of organizational control (Martinus et al., Citation2021; Neal, Citation2012; Sigler, Citation2013). Brokers in networks are important agents that bridge otherwise loosely connected parts of the network and thus can control flows (the tertius gaudens orientation) (Granovetter, Citation1973) and can also facilitate new connections (the tertius iungens orientation) (Burt, Citation2004). The growing literature on regional brokerage concentrates more on this latter approach by distinguishing the types of intermediation of brokers zooming into triadic relations (Gould & Fernandez, Citation1989). However, brokerage is at play at more aggregate levels of network structures as well. For example, brokers can facilitate interaction across communities (Kwon et al., Citation2020), the integration of segregated groups (Stovel & Shaw, Citation2012), and the mixing of network peripheries with the network core (Juhász et al., Citation2020; Pan et al., Citation2018).
The network brokerage approach allows us to assess the role of regions in linking otherwise loosely connected local economic systems. Despite the well-documented spatial concentration of the M&A process, this role of M&A interactions has received less attention. This new approach enables us to contribute to the discussion by analysing regional characteristics that facilitate brokerage by attracting investments or by hosting acquirer companies.
Understanding European integration with interregional networks of M&A
The context of the study is the integration of the European market that has speeded up by the launch of the Economic and Monetary Union in 1992 and the introduction of the euro in 2002. Many studies converge in finding that these steps, mainly through increasing market size and decreasing transaction costs and political uncertainty, have led to a rise in cross-border M&A activity and performance (Coeurdacier et al., Citation2009; McCarthy & Dolfsma, Citation2015; Moschieri et al., Citation2014). Even in the years following the financial crisis, market integration continued despite M&A activity decreased (Weitzel et al., Citation2014) and the patterns of M&A activity during the financial crisis did not deviate substantially from those during periods of expansions. In sum, M&A intensification go hand in hand with monetary and economic integration of the EU and facilitates the transfer of control and knowledge in the common market (Coeurdacier et al., Citation2009; McCarthy & Dolfsma, Citation2015; Moschieri et al., Citation2014). The regional analysis by McCarthy and Dolfsma (Citation2015) shows a rising geographical spread of deals and connections with increasingly distant locations, especially because of an increase in the number of targets in peripheral regions.
Previous studies take the increase of cross-regional M&A activity as a sign of growing economic integration of the European market. However, cross-regional interactions may contribute very differently to knowledge transfer and economic integration depending on the extent to which the same regions repeatedly interact between each other, or, by contrast, many regions evenly interact with a large number of other regions. In fact, integration of national business systems into a European area is not trivial despite the increasing number of international M&A. For example, the empirical evidence is mixed on the impact on integration of the ERA, which promotes an integrated research market with the free circulation of researchers, scientific knowledge and technology. Some observers argue that integration efforts of research and innovation systems have been largely unsuccessful (e.g., Chessa et al., Citation2013; Morescalchi et al., Citation2015) because majority of innovative collaboration still remains within country borders.
In this study, we aim to better understand how regional brokerage – the tertius iungens orientation – and what type of regions facilitate integration of the M&A network across European regions. Our regional brokerage approach builds on previous results that identified communities of regions in innovative knowledge production (Chessa et al., Citation2013; Tóth et al., Citation2021). To reflect potential differences between knowledge-intensive from other M&A, that can be expected due to distinct investment motivations (Zademach & Rodríguez-Pose, Citation2009), we investigate interregional networks of innovative versus non-innovative M&A. The communities identified on these networks are non-overlapping groups of regions that are more densely connected within than across communities (Blondel et al., Citation2008; Newman, Citation2006). Thus, inter-community M&A represent brokerage by definition because they bridge otherwise loosely connected parts of the network (Girvan & Newman, Citation2002) and thereby facilitate integration of the business system. The framework allows us to identify regional characteristics as determinants of regional brokerage in the network by estimating regions position in the network with their characteristics.
DATA AND METHODS
Data
The analysis is based on a sample of 39,346 acquisitions completed between 2003 and 2017 where acquiring and target companies are located in different NUTS-2 regions of the EU, the UK and EFTA, which includes Iceland, Lichtenstein, Norway and Switzerland. The data preparation process started with the extraction of 102,896 acquisitions involving a change in the control of the target company (more than 50% of the shares of the target is acquired) from Zephyr, a database managed by Bureau van Dijk. We then used postal codes to geolocate the companies, assigning them to NUTS-2 regions using the Eurostat Postcode Directory.Footnote1 After discarding 11,256 acquisitions because either the acquiring or the target company had invalid postcodes, we remained with 35,615 local M&A (target and acquiring companies located in the same NUTS-2 region of EU or EFTA countries), 16,679 extra-European M&A (target or acquiring company located outside EU and EFTA countries), as well as the 39,346 cross-regional intra-European acquisitions (target and acquiring companies located in different NUTS-2 regions of EU or EFTA countries) that we used in the analysis.
The choice of NUTS-2 is dictated by the level of spatial aggregation of some important variables used in the regression analysis to measure regional innovation capabilities, namely the expenditure in R&D and the availability of skilled human capital, which are available at the NUTS-2 level of regional disaggregation. Similarly, data availability supports the choice of using political/administrative rather than economic/functional criteria for the spatial disaggregation of countries into regions. While the former criteria may capture local economic activities and interactions less accurately than the latter ones, the lack of a regional disaggregation based on economic areas is a general problem in regional analysis in Europe (e.g., Belderbos & Somers, Citation2015; Bottazzi & Peri, Citation2003; Tappeiner et al., Citation2008).
To distinguish innovative from non-innovative acquisitions, we identified the patent applications of each target company using data from Orbis Intellectual Property, a database managed by Bureau van Dijk with information on companies’ patent applications. We defined an acquisition innovative if the target company made one or more patent applications in the 20 years before deal completion. This yields a sample of 4182 innovative and 35,164 non-innovative cross-regional acquisitions. Limitations of patents in capturing innovations are well known (Hall et al., Citation2014). For instance, firms may prefer not to patent their inventions to keep them secret (Hussinger, Citation2006). Yet, patents have the appealing advantage to allow identifying technological innovations. Accordingly, an increasing number of studies relies on patents to track and analyse innovative M&A based on the idea that the presence of patents among the assets of the target company is an important signal of the technological capability of the target company (Breitzman & Thomas, Citation2002; Morton & Shapiro, Citation2014; Park et al., Citation2013).
Network structure
To compare the spatial structure of innovative and non-innovative M&A across European regions, we aggregated individual M&A at the level of NUTS-2 regions by year and constructed networks of regions in which the directed links (or edges) between regions are weighted by the number of M&A deals. For both networks, we calculate standard measures of network structure, comparing networks over the full period as well as by year, documenting the dynamics of these measures over the 2003–17 period.
We characterize the degree of integration of the network by grouping regions into countries or network groups named communities and compute the following key metrics:
The fraction of cross-country M&A quantified into foreign edges (i.e., the share of international links over the total number of links in the network) and foreign deals (i.e., the share of international deals over the total number of deals).
The fragmentation index based on a partition of regions into groups (or communities) that maximizes the density of links within groups as compared with the density of links across groups. A high fragmentation index means that M&A transactions are concentrated within loosely connected communities and thus the level of integration in the whole system is low.
The share of deals that span network community boundaries.
To calculate the fragmentation index, we use the Louvain algorithm (Blondel et al., Citation2008) that partitions regions by hierarchical clustering. This algorithm uses information on the network structure to find the best grouping of vertices (or nodes) – in our case, NUTS-2 regions – into communities by maximizing the density of the links within communities as compared with the density of links across communities, a measure called modularity in network science (Newman, Citation2006). The modularity Q of the network’s partition can be written as:
where L is the total number of M&A transactions in the network;
is the total transactions of group k; and
is the number of transactions within group k. Because modularity is highly dependent on the size and density of a network, the comparison of the measure alone from two networks – such as the innovative M&A network versus the non-innovative M&A network – can be misleading. Following Sah et al. (Citation2017), we calculated the fragmentation index of the two networks by dividing Q by the theoretical Qmax that would be achieved if all edges were within the communities. Similar values of fragmentation would indicate that differences in community structures are just an artefact of differing network sizes and densities.
The identification and characterization of these communities allow us to understand how M&A transactions are fragmented in groups of European regions. Previous literature on spatial innovative collaboration networks highlighted that network communities are in most cases spatially bounded. For example, Chessa et al. (Citation2013) and Tóth et al. (Citation2021) document that groups of interregional co-inventor collaboration are bounded by national borders or groups of neighbouring countries. Therefore, EU regions grouped into communities of the innovative M&A network can be also expected to follow this characteristic spatial pattern.
Estimation strategy to characterize broker regions
To better understand the drivers behind the integration in the M&A networks, we regressed network centrality indicators considering the direction, weighting method and community structure defined in the previous section on regional characteristics. We apply a fixed-effect panel regression specified by:
(1)
(1) where
is the vector of parameters associated with the vector of time-varying regional characteristics
;
is a region-specific time-invariant fixed effect; dt denotes year fixed effects; and ϵr,t is the error term. The following network centrality measures are used as dependent variable denoted by yr,t for region r (r = 1, … , 288) at year t (t = 2003, … , 2017):
Strength-in characterizes the volume of incoming M&A transactions. These measures quantify the attractiveness of a region.
Strength-out is an indicator of the volume of outgoing M&A transactions. These indices capture the capacity of a region to invest somewhere else.
To characterize regions’ brokerage across network communities, we decompose strength-in and strength-out measures into the number of deals within and across communities. As a robustness check, we model the determinants of betweenness centrality of regions with a Heckman selection method. For the empirical approach and results of the betweenness models, see Appendix E in the supplemental data online.
We use standard regional variables x as in prior literature on regional determinants of cross-regional M&A activity in Europe (e.g., Zademach & Rodríguez-Pose, Citation2009; McCarthy & Dolfsma, Citation2015). These include population (in logs) to measure size effects and per capita gross domestic product (GDP, in logs) to measure economic development. Regional innovation capabilities are measured by R&D intensity (R&D expenditure as a share of GDP) and the share of scientists and engineers in the workforce. The effect of business cycle fluctuations (including the global financial crisis) is modelled through the unemployment rate. Cost factors complete the specification, namely population density, related to congestion costs and pressure on land prices and rents, and corporate tax rates. All independent variables come from the Eurostat online database, except for the corporate tax rates which are taken from the Organisation for Economic Co-operation and Development’s (OECD) online Tax Database.
The estimation sample is an unbalanced panel due to the presence of a few missing values in R&D expenditures and human capital variables implying the loss of 53 region-year observations. All independent variables enter the model with one-year lag to reduce simultaneity issues. Their correlations (see Appendix D in the supplemental data online) indicate that collinearity is not a problem in the models. We did not use models accounting for spatial autocorrelation because they are not appropriate to take into account the clustering of regions into communities, since neighbouring and close regions may both fall within the same as well in a different community of the focal region. Moreover, standard Lagrange multiplier tests of the form proposed by Anselin et al. (Citation1996) indicate the absence of spatial autocorrelation issues in the estimated models.
RESULTS
Network properties of cross-regional M&A
We start the analysis by aggregating M&A transactions across pairs of regions over the 2003–17 period to compare the network of regions generated from innovative deals with the network generated from non-innovative ones.
describes differences in the overall structure of the two networks. The number of regions included in the networks is approximately the same. However, the number of links between regions in the innovative network (non-weighted) is only 32% of that of the non-innovative network. This is the result of the smaller volume of innovative deals, which is only 12% of the volume of non-innovative deals. Links and triads in the innovative network are less likely and paths in the network are therefore longer. We provide further evidence on the spatial concentration of cross-regional M&A activity in Appendix A in the supplemental data online.
Table 1. Networks’ characteristics.
To illustrate how the networks are fragmented by country borders, reports the percentages of M&A deals between companies located in distinct countries and the percentages of interregional edges across countries. The values indicate that innovative deals are more international but that these deals occur more frequently between the same region pair such that the fraction of foreign edges is lower in the innovative network than in the non-innovative network. Moreover, the fragmentation index shows that the innovative network is less fragmented into communities than the non-innovative network. Conversely, there is a higher percentage of deals connecting different communities in the innovative network than in the non-innovative network.
In line with previous research focusing on innovative collaborations (Chessa et al., Citation2013; Tóth et al., Citation2021), substantially yet not completely overlapping spatial communities emerge in innovative and non-innovative M&A networks. As shown in , large countries or groups of neighbouring countries constitute these communities. While national economies of large countries and in some cases groups of neighbouring countries are the primary arenas of M&A transactions, deals across communities are about 27% of all deals. The fragmentation indices are relatively low in both networks (a frequently applied threshold to distinguish between low and high modularity is 0.3; for instance, see Clauset et al., Citation2004). This suggests that although network communities resemble spatially bounded groups of regions, the fraction of transactions that span country or community borders are high (especially in the innovative network), and thus can indeed strengthen the integration of national economies into a unified European ecosystem.
Figure 1. Cross-regional networks of innovative and non-innovative mergers and acquisitions (M&A) and their community structures.
Note: Graphs are created for the networks of M&A deals (edges) completed between 2003 and 2017 that connected different NUTS-2 regions (vertices). Directed edges from the region of the acquirer to the region of the target are weighted by the number of M&A deals. Communities are tightly knit groups of regions and are detected using the Louvain algorithm.
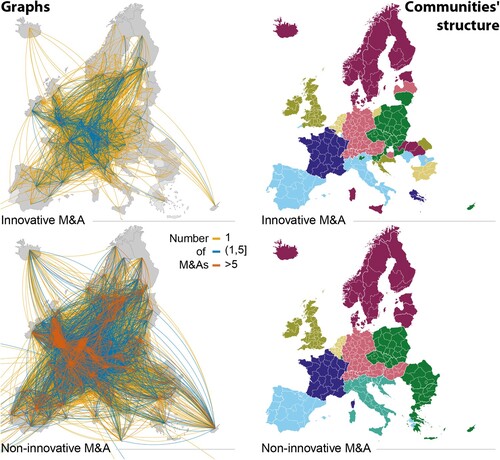
illustrates patterns of integration by showing how the main characteristics of both networks have evolved between 2003 and 2017. To enable the comparison of network dynamics, we standardized each variable by setting the mean over the period to zero and scaling temporal values by the standard deviation from the mean.
Figure 2. Dynamics of networks’ characteristics.
Note: Statistics are computed for the yearly networks created by merger and acquisition (M&A) deals (edges) completed between 2003 and 2017 that connected different NUTS-2 regions (vertices). Directed edges from the region of the acquirer to the region of the target are weighted by the number of M&A deals. All characteristics are standardized by subtracting the average and dividing by the standard deviation in order to make yearly changes comparable across the two networks. Fragmentation is an index that characterizes the concentration of edges in tightly knit groups of regions or communities and detected using the Louvain algorithm. Between communities is the percentage of deals that connect different communities.
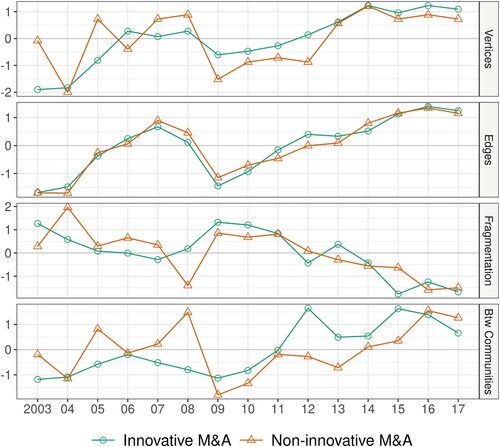
We observed that the scale of M&A transactions increased over the full period but the years of the financial crisis. The fragmentation index decreased over this period, suggesting that the dominance of spatially bounded communities has diminished over time. Integration in the M&A network occurred in periods of more intense M&A activity, before and after the financial crisis. These periods of M&A expansions imply the presence of more interactions across communities. Following the financial crisis, innovative M&A restarted connecting spatial communities faster than non-innovative M&A. This finding is intuitively confirmed by the trend in the share of deals connecting different communities (between communities in ), with innovative cross-community M&A clearly increasing after 2010.
Appendix B in the supplemental data online provides additional information on the network characteristics and the community structure. Figure B1 in Appendix B illustrates that the non-innovative spatial communities are stable over time, while innovative network communities are more volatile. Table B1 in Appendix B contains the raw values of network indicators over the full period.
Regions brokering network communities
M&A transactions are often motivated by access to new markets and new assets, such as skilled labour and technologies, and are more likely to occur from developed regions that have higher volumes of capital to invest. For an overview on the dynamics of network positions and hub regions, see Appendix C in the supplemental data online. The incoming and outgoing M&A create connections with other regions, increasing the centrality of the region in the network. Consequently, when investigating the determinants of the integrating role of regions, we need to, first, understand the drivers of incoming and outgoing M&A deals and, second, distinguish the determinants of the strength of a region in the local community and in connecting different communities; the latter allows regional attributes related to spatial integration to be distinguished.
provides fixed-effect regression models in which strength centrality is the dependent variable. Models 1 and 2 investigate centrality in innovative M&A and models 3 and 4 investigate centrality in non-innovative M&A; models 1 and 3 investigate outflows (acquirer regions) and models 2 and 4 investigate inflows (target regions). The model estimates show that the key drivers of regional centrality with respect to acquirers are population and per capita GDP, which have significant and positive associations for innovative deals and even significantly higher estimates for non-innovative deals. In terms of attracting M&A to target regions, corporate tax rate has a significant negative effect for both types of deals, R&D intensity has a significant positive effect only for innovative deals and per capita GDP has a significant positive effect only for non-innovative deals. For non-innovative deals, the unemployment rate is associated with significant positive parameter estimates for strength of both acquirer and target regions.
Table 2. Regional fixed-effect models on strength centrality.
The results of the models suggest that, in both networks, size of regions and economic development drive cross-regional acquisitions. Yet, the magnitude of the effects is much stronger for non-innovative than innovative M&A. For innovative M&A, the effect of per capita GDP on acquisitions of local companies is even not statistically significant. Another important distinction between the types of deals is that R&D intensity positively affects acquisitions of local target companies in the case of innovative M&A but not in the case of non-innovative M&A. Corporate taxation, by contrast, influences the attractiveness of target companies in both types of M&A.
To better understand which regional characteristics favour bridging across network communities, we decomposed strength into a component of M&A within the network community and a component of M&A between communities. Appendix E in the supplemental data online provides complementary evidence on the characteristics that strengthen the role of regions in economic integration by estimating Heckman selection models on betweenness centrality. Betweenness centrality alone does not indicate whether a region bridges spatially bounded communities because it disregards the community structure of the networks. However, it is a fair approximation of such a role because it captures whether a region is a bridge across tightly knit parts of the network. Model estimates provide similar results to those of the fixed effects models on the strength between communities, further supporting our findings.
shows the results of fixed-effect panel regressions on the decomposed within- and between-communities strength measures. For innovative M&A, regional determinants of strength centrality within and between communities differ substantially. Innovative acquisitions by local companies in regions belonging to the same communities (model 1) depends positively on the population and per capita GDP of the region of the acquiring company and negatively on the share of scientists and engineers in the regional workforce and the corporate tax rate. In contrast, when looking at innovative M&A connecting different communities (model 3), only population has a positive significant effect. As for the number of acquisitions of regional targets made by acquirers (model 2), the workforce share of scientists and engineers and population density are significant determinants of the number of deals within the local community, negatively affecting the number of deals. In contrast, targets acquired by companies located in other communities (model 4) are positively affected by regional population, population density and R&D intensity while the corporate tax rate has a significant negative effect. The difference in the determinants of strength centrality within and between communities is more nuanced for non-innovative M&A. Population and per capita GDP are significant for the connection of regions both within and between communities, having a significant positive effect in all models (5–8). The corporate tax rate and unemployment rate respectively have a negative and a positive effect for the regions of targets acquired by companies located in both the same and different communities (models 6 and 8), as well as for the regions of the acquirers within the same community (model 5). The population density of the region of the acquirer increases M&A between regions within the same community (models 5), while the proportion of skilled human capital in the target region increases the number of deals between different communities (model 8). Models with alternative measures of human capital quality such as the share of people with tertiary education and/or employed in science and technology provided the same results.
Table 3. Regional fixed-effect models on strength centrality within and between communities.
In sum, the results of the models indicate that high population levels in the regions of the acquirer and target companies facilitate the occurrence of M&A deals that connect different communities in both networks, and high levels of economic development in the non-innovative networks. Other characteristics of the region of the target have a significant role in connecting communities. Namely, high corporate tax rate reduces the connection of communities in both networks. The intensity of regional R&D expenditure and population density positively influence the connection of regions in different communities in the innovative network, the presence of a skilled labour force and high unemployment in the non-innovative one.
DISCUSSION
Despite being an important source of economic integration in the EU, we find in this study that M&A transactions across European regions are fragmented by country borders or by groups of neighbouring countries. The spatially bounded M&A systems follow similar patterns that were previously found in innovative European collaborations (Chessa et al., Citation2013; Tóth et al., Citation2021), signalling that the process of European integration is still incomplete. However, our results confirm that intensifying interregional M&A mitigate spatial fragmentation and facilitate the integration of European regions into a unified economic area (McCarthy & Dolfsma, Citation2015).
We provide a network brokerage approach to identify regional attributes that play a critical role in bridging segregated economic systems. In this approach, the broker regions are those hubs that attract investments or host acquirers such that M&A transactions bridge otherwise loosely connected communities. We find that these large and wealthy regions, sometimes accused of reaping most benefits from the processes of innovation and globalization, not only concentrate economic power by transferring control from proximate regions (Böckerman & Lehto, Citation2006; Zademach & Rodríguez-Pose, Citation2009), but are also leading European integration by linking distant communities.
We also show that regions’ potential of brokerage differs by M&A type. Innovative M&A deals, motivated by new knowledge access, connect different network communities to a larger extent than non-innovative M&A that are more market oriented. However, foreign innovative deals are also more concentrated in the same pairs of developed regions, which is line with geography of innovation literature that argues for the spikiness of innovation as few places are suitable for innovation (e.g., Castellani et al., Citation2013; Castellani, Citation2018; Crescenzi et al., Citation2020). We highlight that regional R&D expenditure can attract distant innovative investments across communities and thus strengthen integration of knowledge-intensive business environment in the EU. In the case of non-innovative M&A, the presence of skilled human capital is found decisive. These results support the idea that policies promoting human capital development and R&D – and especially those targeted to less developed regions – can help economic integration by facilitating knowledge transfer between developed and less developed regions.
Our approach on regional brokerage contributes to the ongoing discussion on how regions bridge otherwise loosely connected parts of the global economy (Martinus et al., Citation2021). Unlike in Sigler et al. (Citation2021) that describe the diversity of brokerage, our focus is on a specific type of brokers that link domestic business systems. The long-distance bridges in the interregional M&A network transfer massive bodies of knowledge across regions (Bathelt & Henn, Citation2021). Thus, besides facilitating economic integration in the EU, the broker regions might also act as gatekeepers between national systems (Breschi & Lenzi, Citation2015) and can be important engines of economic development by enabling novel combinations of knowledge resided in national systems. In this sense, whether the transaction links complementary knowledge bases is critical for EU competitiveness and Smart Specialisation Strategies (Balland et al., Citation2019; Balland & Boschma, Citation2021; McCann & Ortega-Argilés, Citation2015) and requires further research. Our approach presented here is not without limitations and demands further improvements. First, we do not consider the direction of knowledge transfer; hence it is difficult to assess whether M&A means knowledge flow to the target or the source region. The overlap of patent portfolios of the acquirer and target companies and subsequent patenting dynamics might allow for inferring directions of knowledge transfer across regions. Second, generalizability of these results on regional brokerage in other context like collaborations in patents and scientific publications, in different M&A categories (e.g., industries), or in other macro regions and different time periods is not clear and calls for further investigations. Third, individual M&A transactions might be looked at more in detail to better understand what types of deals bridge economic systems and what are their local consequences for subsequent economic dynamics. Finally, to better reflect the divide between more and less developed regions in the network, the brokerage approach should be further developed for example by considering core/periphery structures (Juhász et al., Citation2020).
Supplemental Material
Download PDF (9.3 MB)ACKNOWLEDGEMENTS
We are very grateful to the guest editors and two anonymous referees for extensive comments that helped to improve the paper considerably. We also thank Sara Amoroso and Dániel Vértesy for their contributions to the inception of the study; Davide Castellani for in-depth comments on a previous version of the study; Nicola Pontarollo for in-depth discussions on the methods; Andrea Marcucci for excellent research assistance; and Antonella Ferrara, Athina Karvounaraki, Tiago Pereira and the participants at the 2020 workshops on the Internationalization of Innovation (Reading, UK) and the 2020 INNOVA MEASURE IV workshops for helpful comments and suggestions.
DISCLOSURE STATEMENT
No potential conflict of interest was reported by the authors. The scientific output expressed here does not imply a policy position of the European Commission. Neither the European Commission nor any person acting on its behalf is responsible for the use which might be made of this study.
Additional information
Funding
Notes
1. The Eurostat Postcode Directory (https://ec.europa.eu/eurostat/web/nuts/correspondence-tables/postcodes-and-nutshttps://ec.europa.eu/eurostat/web/nuts/correspondence-tables/postcodes-and-nuts) provides a link between postcodes and NUTS-3 codes. The 2013 version of the NUTS classification is used in this study.
REFERENCES
- Albuquerque, R., Brandão-Marques, L., Ferreira, M. A., & Matos, P. (2019). International corporate governance spillovers: Evidence from cross-border mergers and acquisitions. The Review of Financial Studies, 32(2), 738–770. https://doi.org/10.1093/rfs/hhy053
- Angwin, D. (2001). Mergers and acquisitions across European borders: National perspectives on preacquisition due diligence and the use of professional advisers. Journal of World Business, 36(1), 32–57. https://doi.org/10.1016/S1090-9516(00)00053-5
- Anselin, L., Bera, A. K., Florax, R., & Yoon, M. J. (1996). Simple diagnostic tests for spatial dependence. Regional Science and Urban Economics, 26(1), 77–104. https://doi.org/10.1016/0166-0462(95)02111-6
- Ashcroft, B., & Love, J. H. (1993). Takeovers, mergers, and the regional economy. Edinburgh University Press.
- Balland, P. A., & Boschma, R. (2021). Complementary interregional linkages and Smart Specialisation: An empirical study on European regions. Regional Studies, 55(6), 1059–1070. https://doi.org/10.1080/00343404.2020.1861240
- Balland, P. A., Boschma, R., Crespo, J., & Rigby, D. L. (2019). Smart Specialisation policy in the European Union: Relatedness, knowledge complexity and regional diversification. Regional Studies, 53(9), 1252–1268. https://doi.org/10.1080/00343404.2018.1437900
- Bathelt, H., & Henn, S. (2021). Knowledge exchanges, trust, and secretive geographies in merger and acquisition processes. Environment and Planning A, https://doi.org/10.1177/0308518X211013360.
- Bathelt, H., Malmberg, A., & Maskell, P. (2004). Clusters and knowledge: Local buzz, global pipelines and the process of knowledge creation. Progress in Human Geography, 28(1), 31–56. https://doi.org/10.1191/0309132504ph469oa
- Belderbos, R., & Somers, D. (2015). Do technology leaders deter inward R&D investments? Evidence from regional R&D location decisions in Europe. Regional Studies, 49(11), 1805–1821. https://doi.org/10.1080/00343404.2015.1018881
- Bertrand, O. (2009). Effects of foreign acquisitions on R&D activity: Evidence from firm-level data for France. Research Policy, 38(6), 1021–1031. https://doi.org/10.1016/j.respol.2009.03.001
- Blondel, V. D., Guillaume, J.-L., Lambiotte, R., & Lefebvre, E. (2008). Fast unfolding of communities in large networks. Journal of Statistical Mechanics: Theory and Experiment, 2008(10), P10008. https://doi.org/10.1088/1742-5468/2008/10/P10008
- Boschma, R. (2005). Proximity and innovation: A critical assessment. Regional Studies, 39(1), 61–74. https://doi.org/10.1080/0034340052000320887
- Boschma, R., & Hartog, M. (2014). Merger and acquisition activity as driver of spatial clustering: The spatial evolution of the Dutch banking industry, 1850–1993. Economic Geography, 90(3), 247–266. https://doi.org/10.1111/ecge.12054
- Bottazzi, L., & Peri, G. (2003). Innovation and spillovers in regions: Evidence from European patent data. European Economic Review, 47(4), 687–710. https://doi.org/10.1016/S0014-2921(02)00307-0
- Böckerman, P., & Lehto, E. (2006). Geography of domestic mergers and acquisitions (M&A): evidence from matched firm-level data. Regional Studies, 40(8), 847–860. https://doi.org/10.1080/00343400601043365
- Breitzman, T., & Thomas, P. (2002). Using patent citation analysis to target/value M&A candidates. Research Technology Management, 45(5), 28–36. https://doi.org/10.1080/08956308.2002.11671519
- Breschi, S., & Lenzi, C. (2015). The role of external linkages and gatekeepers for the renewal and expansion of US cities’ knowledge base, 1990–2004. Regional Studies, 49(5), 782–797. https://doi.org/10.1080/00343404.2014.954534
- Bruhn, N. C. P., Calegário, C. L. L., Carvalho, F. M., Campos, R. S., & dos Santos, A. C. (2017). Mergers and acquisitions in Brazilian industry: A study of spillover effects. International Journal of Productivity and Performance Management, 66(1), 51–77. https://doi.org/10.1108/IJPPM-11-2014-0179
- Burt, R. (2004). Structural holes and good ideas. American Journal of Sociology, 110(2), 349–399. https://doi.org/10.1086/421787
- Caiazza, R., & Volpe, T. (2015). M&A process: A literature review and research agenda. Business Process Management Journal, 21(1), 205–220. https://doi.org/10.1108/BPMJ-11-2013-0145
- Cano-Kollmann, M., Cantwell, J., Hannigan, T. J., Mudambi, R., & Song, J. (2016). Knowledge connectivity: An agenda for innovation research in international business. Journal of International Business Studies, 47(3), 255–262. https://doi.org/10.1057/jibs.2016.8
- Castellani, D. (2018). The changing geography of innovation and the multinational enterprise. In G. Cook, J. Johns, F. McDonald, J. Beaverstock, & N. Pandit (Eds.), The Routledge companion to international business and economic geography (pp. 454–474). Routledge.
- Castellani, D., Jimenez, A., & Zanfei, A. (2013). How remote are R&D labs? Distance factors and international innovative activities. Journal of International Business Studies, 44(7), 649–675. https://doi.org/10.1057/jibs.2013.30
- Chapman, K. (2003). Cross-border mergers/acquisitions: A review and research agenda. Journal of Economic Geography, 3(3), 309–334. https://doi.org/10.1093/jeg/3.3.309
- Chessa, A., Morescalchi, A., Pammolli, F., Penner, O., Petersen, A. M., & Riccaboni, M. (2013). Is Europe evolving toward an integrated research area? Science, 339(6120), 650–651. https://doi.org/10.1126/science.1227970
- Clauset, A., Newman, M. E., & Moore, C. (2004). Finding community structure in very large networks. Physical Review E, 70(6), 066111. https://doi.org/10.1103/PhysRevE.70.066111
- Coeurdacier, N., De Santis, R. A., & Antonin, A. (2009). Cross-border mergers and acquisitions and European integration. Economic Policy, 24(57), 55–106. https://doi.org/10.1111/j.1468-0327.2009.00218.x
- Colombo, L. V., & Turati, G. (2014). Why do acquiring banks in mergers concentrate in well-developed areas? Regional development and mergers and acquisitions (M&A) in banking. Regional Studies, 48(2), 363–381. https://doi.org/10.1080/00343404.2011.653337
- Colombo, M. G., & Rabiossi, L. (2014). Technological similarity, post-acquisition R&D reorganization, and innovation performance in horizontal acquisitions. Research Policy, 43(6), 1039–1054. https://doi.org/10.1016/j.respol.2014.01.013
- Crescenzi, R., & Iammarino, S. (2017). Global investments and regional development trajectories: The missing links. Regional Studies, 51(1), 97–115. https://doi.org/10.1080/00343404.2016.1262016
- Crescenzi, R., Iammarino, S., Ioramashvili, C., Rodríguez-Pose, A., & Storper, M. (2020). The geography of innovation and development: Global spread and local hotspots (Geography and Environment Discussion Paper Series 4). London School of Economics and Political Science.
- Das, A., & Kapil, S. (2012). Explaining M&A performance: A review of empirical research. Journal of Strategy and Management, 5(3), 284–330. https://doi.org/10.1108/17554251211247580
- Derudder, B., Taylor, P., Ni, P., De Vos, A., Hoyler, M., Hanssens, H., Bassens, D., Huan, J., Witlox, F., Shen, W., & Yang, X. (2010). Pathways of change: Shifting connectivities in the world city network, 2000–08. Urban Studies, 47(9), 1861–1877. https://doi.org/10.1177/0042098010372682
- Girvan, M., & Newman, M. E. J. (2002). Community structure in social and biological networks. Proceedings of the National Academy of Sciences, USA, 99(12), 7821–7826. https://doi.org/10.1073/pnas.122653799
- Giuliani, E., & Bell, M. (2005). The micro-determinants of meso-level learning and innovation: Evidence from a Chilean wine cluster. Research Policy, 34(1), 47–68. https://doi.org/10.1016/j.respol.2004.10.008
- Gould, R. V., & Fernandez, R. M. (1989). Structures of mediation: A formal approach to brokerage in transaction networks. Sociological Methodology, 19, 89–126. https://doi.org/10.2307/270949
- Granovetter, M. (1973). The strength of weak ties. American Journal of Sociology, 78(6), 1360–1380. https://doi.org/10.1086/225469
- Green, M. B. (1990). Mergers and acquisitions: Geographic and spatial perspectives. Routledge.
- Hall, B., Helmers, C., Rogers, M., & Sena, V. (2014). The choice between formal and informal intellectual property: A review. Journal of Economic Literature, 52(2), 375–423. https://doi.org/10.1257/jel.52.2.375
- Hussinger, K. (2006). Is silence golden? Patents versus secrecy at the firm level. Economics of Innovation and New Technology, 15(8), 735–752. https://doi.org/10.1080/10438590500510467
- Iammarino, S., & McCann, P. (2013). Multinationals and economic geography. Location, technology, and innovation. Edward Elgar.
- Iammarino, S., Rodríguez-Pose, A., & Storper, M. (2019). Regional inequality in Europe: Evidence, theory and policy implications. Journal of Economic Geography, 19(2), 273–298. https://doi.org/10.1093/jeg/lby021
- Juhász, S., Tóth, G., & Lengyel, B. (2020). Brokering the core and the periphery: Creative success and collaboration networks in the film industry. PLoS ONE, 15(2), e0229436. https://doi.org/10.1371/journal.pone.0229436
- Krugman, P., & Venables, A. J. (1990). Integration and the competitiveness of peripheral industry (Discussion Papers No. 363). CEPR.
- Kwon, S. W., Rondi, E., Levin, D. Z., De Massis, A., & Brass, D. J. (2020). Network brokerage: An integrative review and future research agenda. Journal of Management, 46(6), 1092–1120. https://doi.org/10.1177/0149206320914694
- Martinus, K., Sigler, T., Iacopini, I., & Derudder, B. (2021). The brokerage role of small states and territories in global corporate networks. Growth and Change, 52(1), 12–28. https://doi.org/10.1111/grow.12336
- Massoudi, A., Fontanella-Khan, J., & Weinland, D. (2017). Global M&A exceeds $3tn for fourth straight year. The Financial Times. December 28. https://www.ft.com/content/9f0270aa-eabf-11e7-bd17-521324c81e23
- McCann, P., & Ortega-Argilés, R. (2015). Smart Specialization, regional growth and applications to European Union Cohesion Policy. Regional Studies, 49(8), 1291–1302. https://doi.org/10.1080/00343404.2013.799769
- McCarthy, K. J., & Dolfsma, W. (2015). The Euro and its impact on the number, size, performance and regional spread of European mergers and acquisitions. Regional Studies, 49(8), 1407–1422. https://doi.org/10.1080/00343404.2015.1007936
- Morescalchi, A., Pammolli, F., Orion Penner, O., Petersen, A. M., & Riccaboni, M. (2015). The evolution of networks of innovators within and across borders: Evidence from patent data. Research Policy, 44(3), 651–668. https://doi.org/10.1016/j.respol.2014.10.015
- Morton, F. M. S., & Shapiro, C. (2014). Strategic patent acquisitions. Antitrust Law Journal, 79(2), 463–499.
- Moschieri, C., Ragozzino, R., & Campa, J. M. (2014). Does regional integration change the effects of country-level institutional barriers on M&A? The case of the European Union. Management International Review, 54(6), 853–877. https://doi.org/10.1007/s11575-014-0206-7
- Neal, Z. P. (2012). The connected city: How networks are shaping the modern metropolis. Routledge.
- Newman, M. E. J. (2006). Modularity and community structure in networks. Proceedings of the National Academy of Sciences, USA, 103(23), 8577–8582. https://doi.org/10.1073/pnas.0601602103
- Pan, F., He, Z., Sigler, T., Martinus, K., & Derudder, B. (2018). How Chinese financial centers integrate into global financial center networks: An empirical study based on overseas expansion of Chinese financial service firms. Chinese Geographical Science, 28(2), 217–230. https://doi.org/10.1016/j.regsciurbeco.2018.04.009
- Park, H., Yoon, J., & Kim, K. (2013). Identification and evaluation of corporations for merger and acquisition strategies using patent information and text mining. Scientometrics, 97(3), 883–909. https://doi.org/10.1007/s11192-013-1010-z
- Platt, E. (2018). Global M&A activity hits new high. The Financial Times. September 28. https://www.ft.com/content/b7e67ba4-c28f-11e8-95b1-d36dfef1b89a
- Rao, N. V., & Reddy, K. S. (2015). The impact of the global financial crisis on cross-border mergers and acquisitions: A continental and industry analysis. Eurasian Business Review, 5(2), 309–341. https://doi.org/10.1007/s40821-015-0028-y
- Rivera-Batiz, L. A., & Romer, P. M. (1991). Economic integration and endogenous growth. The Quarterly Journal of Economics, 106(2), 531–555. https://doi.org/10.2307/2937946
- Rodríguez-Pose, A., & Zademach, H.-M. (2003). Rising metropoli: The geography of mergers and acquisitions in Germany. Urban Studies, 40(10), 1895–1923. https://doi.org/10.1080/0042098032000116031
- Sah, P., Leu, S. T., Cross, P. C., Hudson, P. J., & Bansal, S. (2017). Unraveling the disease consequences and mechanisms of modular structure in animal social networks. Proceedings of the National Academy of Sciences, 114(16), 4165–4170. https://doi.org/10.1073/pnas.1613616114
- Sigler, T. J. (2013). Relational cities: Doha, Panama City, and Dubai as 21st century entrepôts. Urban Geography, 34(5), 612–633. https://doi.org/10.1080/02723638.2013.778572
- Sigler, T. J., Neal, Z. P., & Martinus, K. (2021). The brokerage roles of city-regions in global corporate networks. Regional Studies, https://doi.org/10.1080/00343404.2021.1950914.
- Song, J. (2014). Subsidiary absorptive capacity and knowledge transfer within multinational corporations. Journal of International Business Studies, 45(1), 73–84. https://doi.org/10.1057/jibs.2013.55
- Storper, M., & Venables, A. J. (2004). Buzz: Face-to-face contact and the urban economy. Journal of Economic Geography, 4(4), 351–370. https://doi.org/10.1093/jnlecg/lbh027
- Stovel, K., & Shaw, L. (2012). Brokerage. Annual Review of Sociology, 38(1), 139–158. https://doi.org/10.1146/annurev-soc-081309-150054
- Tappeiner, G., Hauser, C., & Walde, J. (2008). Regional knowledge spillovers: Fact or artifact? Research Policy, 37(5), 861–874. https://doi.org/10.1016/j.respol.2007.07.013
- Tóth, G., Juhász, S., Elekes, Z., & Lengyel, B. (2021). Repeated collaboration of inventors across European regions. European Planning Studies, 29(12), 2252–2272. https://doi.org/10.1080/09654313.2021.1914555
- Weitzel, U., Kling, G., & Gerritsen, D. (2014). Testing the fire-sale FDI hypothesis for the European financial crisis. Journal of International Money and Finance, 49, 211–234. https://doi.org/10.1016/j.jimonfin.2014.03.011
- Wigger, A. (2012). The political interface of financialization and the regulation of mergers and acquisitions in the EU. Journal of European Integration, 34(6), 623–641. https://doi.org/10.1080/07036337.2012.707364
- Zademach, H.-M., & Rodríguez-Pose, A. (2009). Cross-border M&A and the changing economic geography of Europe. European Planning Studies, 17(5), 765–789. https://doi.org/10.1080/0965431090277t8276