Abstract
Reference intervals (RIs), developed as part of the Nordic Reference Interval Project 2000 (NORIP) are widely used in most European laboratories. We aimed to examine the validity of the NORIP RIs by establishing RIs for 12 frequently used laboratory tests based on data from a local Danish population and compare these local RIs with the NORIP RIs. Using an a posteriori direct sampling approach, blood sample data were assessed from 11,138 participants aged 18+ years in the Lolland-Falster Health Study (LOFUS), of whom 2154 turned out to meet criteria for being healthy for inclusion in establishing RIs according to the NORIP methodology. The 2.5th and 97.5th percentiles were calculated for alanine aminotransferase (ALAT), albumin, alkaline phosphatase, bilirubin, creatinine, hemoglobin, high-density lipoprotein cholesterol, iron, low-density lipoprotein cholesterol, thrombocytes, total cholesterol, and triglycerides. When comparing our estimates with the NORIP, the lower reference limits (RLs) for bilirubin and iron were lower, and higher for ALAT, thrombocytes and triglycerides. Upper RLs were lower for albumin (males and females ≥70 years), bilirubin and iron, but higher for alkaline phosphatase, triglycerides and for creatinine in men. In LOFUS, approximately 20% of the participants were healthy and qualified for inclusion in the establishment of RIs. Several of the local RIs differed from the NORIP RIs.
Introduction
Clinical laboratory testing of blood samples is important for diagnosing and monitoring health and disease status. The evaluation of clinical laboratory test results is based mainly on reference intervals (RIs) which for several laboratory tests are defined as the central 95% of a well-defined reference population [Citation1]. RIs are not universal and are known to differ across laboratory methods, populations, sexes, ages and ethnicities [Citation2,Citation3]. It is therefore recommended that previously established RIs are validated either by transference following the protocol of the Clinical and Laboratory Standards Institute (CLSI) or by establishing RIs based on samples from at least 20 qualified reference individuals [Citation4]. In Europe, most laboratories make use of the common RIs developed as part of the Nordic Reference Interval Project 2000 (NORIP), in which 102 laboratories in the Nordic countries collaborated to produce common RIs for biochemical and hematological parameters [Citation5–7]. The majority of data used in the NORIP originated from Finland and Sweden, while only 13% derived from Denmark. Since the introduction of the NORIP only few studies from the Nordic countries have attempted to undertake the recommended validation of NORIP RIs in use for the general adult population [Citation8–10]. This study provides data from the Lolland-Falster Health Study (LOFUS), a population-based survey undertaken in 2016–2020 in Lolland-Falster, a rural-provincial region in Denmark with approximately 1,03,000 inhabitants [Citation11]. Using the LOFUS data, the purpose of the present study was to establish 12 RIs widely used in clinical practice and compare these RIs with the NORIP RIs using same methodology, following the CLSI and the International Federation of Clinical Chemistry and Laboratory Medicine (IFCC) guidelines [Citation12].
Materials and methods
Study design
LOFUS is a household-based population study where randomly selected persons aged 18 and above were invited with their household members of all ages, if any. A detailed description of the study protocol has been published previously [Citation11]. For this study, we used data for adults aged 18 years and above (n = 11,138) participating from 2016 to 2019, excluding pregnant women.
Self-reported data
From questionnaires [Citation13], we used data on (1) self-perceived health, (2) self-reported medical condition (diabetes, kidney disease, cancer, cardiovascular disease counting stroke, hypertension, atherosclerosis, angina pectoris, and deep vein thrombosis), (3) self-reported medication (antihypertensive, cardiac medications, diuretics, cholesterol-lowering medication, anticoagulants, anti-diabetic medication), and (4) self-reported excessive alcohol consumption. Data on ethnicity was not recorded in LOFUS.
Health examination data and definitions
We used data on (1) blood pressure: No hypertension was defined according to the European Society of Cardiology (ESC) and the European Society of Hypertension (ESH) [Citation14] guidelines as; systolic blood pressure <120 mmHg, diastolic blood pressure <80 mmHg and also no self-reported use of antihypertensive medications. Prehypertension was defined as systolic blood pressure 120–139 mmHg and/or diastolic blood pressure 80–89 and no self-reported use of antihypertensive medications. Known hypertension was defined as using antihypertensive medications. Undiagnosed hypertension was defined as no use of antihypertensive medications and systolic blood pressure above or equal to 140 mmHg and/or diastolic blood pressure above or equal to 90 mmHg. Further, (2) data on body mass index (BMI), calculated according to The World Health Organization (WHO) as weight divided by the square of the height (kg/m2) and categorized as underweight (BMI less than 18.5), normal (BMI 18.5–24.9), overweight (BMI 25.0–29.9), or obese (BMI 30.0 or greater) [Citation15], and 3) on waist-hip ratio (WHR) calculated by waist-circumference divided by hip circumference. Elevated WHR was considered in females with WHR≥ 0.85 and in males with a WHR≥ 0.90.
Blood samples
Blood samples were collected in the non-fasting state between 8:40 a.m. and 6:30 p.m. in vacutainer blood collection tubes (Becton, Dickinson and Company; Franklin Lakes, NJ, USA) and kept at 21 °C (±4 °C) until delivery at 8:00 p.m. for immediate analysis at the Department of Clinical Biochemistry at Nykøbing Falster Hospital, accredited by the standard ISO 15189. The samples were centrifuged for 10 min (at a minimum of 2500 g). Hemoglobin and thrombocytes concentration in venous blood were measured by Sysmex XE-5000. Siemens Dimension Vista 1500 was used to measure plasma concentrations of albumin, alkaline phosphatase, alanine aminotransferase (ALAT), bilirubin, iron, total cholesterol, high-density lipoprotein cholesterol (HDL), creatinine (enzymatically), and triglycerides in venous blood. Low-density lipoprotein cholesterol (LDL) was calculated by using Friedewald formula [Citation16] when the plasma triglyceride concentration was below 4.5 mmol/L. All instruments used were calibrated according to the manufacturer’s instructions. Results of available Serum X analysis are presented in Supplementary Table 1. Accuracy was monitored several times yearly with the Danish Institute for External Quality Assurance for Laboratories in the health sector programme (DEKS) [Citation17].
Dyslipidaemia was defined according to Danish guidelines [Citation18] as at least one of the following criteria; total cholesterol ≥5 mmol/L, HDL-cholesterol ≤1 mmol/L plasma triglycerides ≥2 mmol/L, and LDL-cholesterol ≥3 mmol/L.
Glycated haemoglobin (HbA1c) levels were measured by TOSOH-G8 and were only used to define the reference population.
Reference population
The direct a priori sampling method was used in NORIP, where the exclusion and partitioning criteria were applied before the selection of the reference individuals, while our study was conducted by the a posteriori sampling method, where criteria were applied after the LOFUS data collection was performed. Some criteria used in NORIP were therefore not available for the present study, including information on fasting state, currently breast feeding, blood donor status, smoking during the hour before blood sampling, and consumption of more than 2 units of alcohol in the last 24 hours prior to blood sampling.
The criteria used in the present study were (1) not pregnant, (2) feeling subjectively well, (3) 18+ years of age, (4) no medical diagnosis, (5) no prescribed medications except oral contraceptives or oestrogens, (6) not obese, (7) no excessive alcohol consumption defined by ≥5 units (1 unit = 12 grams) per day. Furthermore, we excluded participants with extreme values of HbA1c ≥48, systolic blood pressure ≥140 or diastolic blood pressure ≥90.
Statistical methods
Data were partitioned according to Rustad et al. to allow for comparison with NORIP [Citation5]. As data were provided by the laboratories with rounded value, random noise was added to them, that is each value x was transformed into x + S(x) * (R − 0.5), where S(x) = 10^(-n), with n number of decimals in x, and R is a random number uniformly sampled in the set {i/10, i = 0,…, 10}. This was done in order to weight the uncertainty of rounded measurement, to avoid the equal value problem, and to ensure the reliability of the statistical procedures performed on the data [Citation5,Citation19]. Following Solberg [Citation20], outliers were detected according to the following procedure. For each (partition) variable, data were first normalized (to have mean 0 and SD 1), then mathematically transformed to approximate a Gaussian distribution. In order to do so, the following transformations, in that specific order, were applied to the (normalized) data: the exponential function described by Manly [Citation21] to correct for skewness, and the modulus function described by John et al. [Citation22] to correct for non-Gaussian kurtosis. The parameters of the above transformations were found by maximum likelihood estimation. Finally, outliers were defined as any value distant more than 1.5 times the interquartile range (IQR) from the lower (Q1) and upper (Q3) quartiles, that is as any x < Q1 − 1.5*IQR or x > Q3 + 1.5*IQR [Citation20]. Following Solberg [Citation20], reference limits (RLs) were calculated by a non-parametric bootstrap method as follows. For each partition 5000 samples, with replacement, were drawn and for each sample the 2.5% and 97.5% percentiles (defined as Definition 5 by Hyndman [Citation23], see also method II in Linnet [Citation24]) were estimated. Thus, the lower and upper RL for the given partition were computed as the mean of the sample estimates. Upper and lower 90% confidence limits were calculated RL ± 1.64 * SD, where RL denotes the RL (computed as above) and SD the standard deviation of the sample estimates.
Comparison of established RIs and NORIP RIs was made by taking the difference of the established RI (point estimate and confidence limits) and compare it with the corresponding NORIP RIs (only point estimate).
The analyses were performed in R 3.5.3 [Citation25], with tidyverse package collection [Citation26], and boot [Citation27,Citation28], robustbase [Citation29], moments [Citation30] and nortest packages [Citation31].
Ethical issues
All participants provided written informed consent and the Region Zealand’s Ethical Committee on Health Research (SJ-421) and the Danish Data Protection Agency (REG-24-2015) approved the study. LOFUS is registered in ClinicalTrials.gov (NCT02482896).
Results
Description of the study population
Basic characteristics of the study population are presented in . Based on a total of 11,138 participants (48.5% males and 51.5% females), the median age of the participants was 58 (IQR 22). Overweight and obesity fractions were 38.6% and 24.5% in males and females combined. 28.9% was found with prehypertension and 18.8% with undiagnosed hypertension. A total of 69.3% was found with dyslipidaemia. Self-perceived health and reports of disease status and medication are presented in Supplementary Table 2. Out of the 11,138 persons, 2154 persons fulfilled the criteria for being included in the reference population for establishing local RIs; 1094 females and 1060 males. Baseline characteristics of the reference population are presented in .
Table 1. Baseline characteristics of participants in Lolland-Falster Health Study aged 18 + years: Determinants, 2016–2019.
Table 2. Baseline characteristics of reference population.
Reference intervals for males and females
RIs for upper and lower limits of 12 laboratory test results in our healthy study population are reported in , together with the corresponding NORIP values [Citation5–7]. When comparing with NORIP, the lower limits for ALAT in females (14.3: 14.0–14.6) and males (17.1: 16.7–17.5) were higher. This was found also for thrombocytes in females (176: 172–181) and males (156: 152–160) and for triglycerides (0.51: 0.49–0.52). The lower limits for bilirubin (4.0: 3.9–4.0) and for iron (6.1: 5.7–6.5) were lower than NORIP values for females and males.
Table 3. Lower and upper limits for reference intervals in apparently healthy males and females.
The upper limits were higher than NORIP for alkaline phosphatase (115.3: 110.8–119.8), and for triglycerides (3.79: 3.57–4.01) in both females and males. This was also found for creatinine in males (106.2: 104.1–108.3). Upper limits lower than NORIP values were found for albumin in females and males ≥70 years (42.8: 42.2–43.5), for bilirubin (22.2: 20.6–23.8), and for iron (29.2: 28.3–30.1). Relative change (%) of LOFUS RIs compared to NORIP RIs, and observations out of the 2.5 and 97.5 percentile or not available are listed in Supplementary Tables 3 and 4.
Discussion
The study had two important outcomes. First, in the population of Lolland-Falster in Denmark, approximately 20% of adult persons were qualified as healthy for inclusion in establishing RIs according to the NORIP methodology [Citation5,Citation6]. When comparing characteristics of the LOFUS participants with that of the participants in other Danish population-based studies such as the Copenhagen General Population Study (CGPS) and the Danish General Suburban Population Study (GESUS) we found LOFUS participants to have higher tendency of obesity, dyslipidaemia, prehypertension and undiagnosed hypertension [Citation32,Citation33]. Previous studies from the present century have reported higher prevalence of lifestyle diseases and hypertension in rural compared with urban areas within given countries [Citation34–36]. Also, persons living in Lolland-Falster have a life expectancy 5.7 years lower than other regions in Denmark [Citation37], and report physical health problems more frequently than people in the rest of the country [Citation38].
Second, our establishment of the 12 RIs commonly used in clinical practice showed that several of these RIs differed from NORIP RIs for either the lower or upper limits ( and ). Using NORIP, lower limits for ALAT and thrombocytes would likely result in overtreatment and for bilirubin under-treatment. Using NORIP, upper limits for alkaline phosphatase and for creatinine in males would probably result in overtreatment while it for albumin in persons ≥70 years and for bilirubin would result in under-treatment.
Figure 1. Relative change and 90% confidence intervals of LOFUS reference values compared to NORIP values, lower limit. Relative change is computed as (XLOFUS - XNORIP)/XNORIP, and similarly for confidence limits. Black dots denote relative changes whose confidence interval overlaps zero (dashed line).
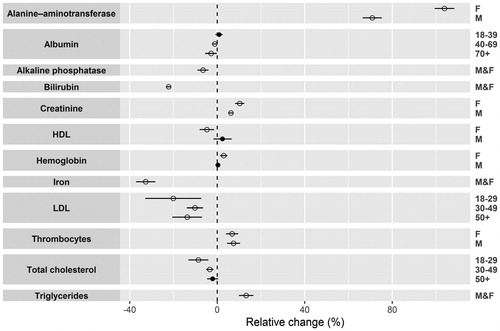
Figure 2. Relative change and 90% confidence intervals of LOFUS reference values compared to NORIP values, upper limit. Relative change is computed as (XLOFUS - XNORIP)/XNORIP, and similarly for confidence limits. Black dots denote relative changes whose confidence interval overlaps zero (dashed line).
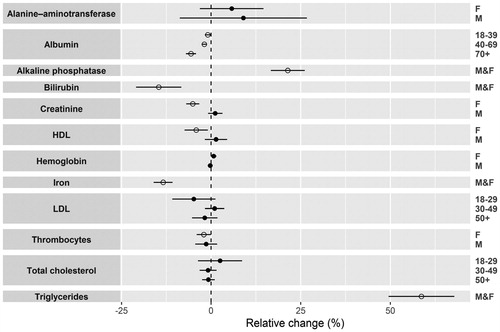
Data on instrument bias estimated by Serum X and provided from the Department of Clinical Biochemistry, Nykøbing Falster Hospital can in general verify transference of the RIs as most deviations were lower than found in the NORIP [Citation5]. Higher deviations were observed for ALAT (21.90%) and albumin (3.50%) however, no correction of data was applied as the Serum X approach estimates bias at a single level only and bias often differs over the measuring range. Correction of data could thus cause uncertainties. The Department of Clinical Biochemistry, Nykøbing Falster Hospital reported lower deviation for ALAT when measurements were above 40 U/L and for albumin in general while using other external and internal quality control programs [Citation39]. The substantial difference found for the lower RL for ALAT and for albumin, when comparing with NORIP may thus only partly be explained by instrument bias.
A previous Danish study by Lykkeboe et al. [Citation8] constructed local RIs by an indirect technique using data from laboratory information systems deriving from outpatients consulting general practitioners in the Northern Jutland region. In this study, only one criterion was used to define a healthy population; infrequent contact to healthcare defined by only one request for biochemical testing in the regional laboratory information system within 18 months retrospective of an included sample. When comparing our study results with their findings, we found similar results; for the lower RL for iron, upper RLs for triglycerides and alkaline phosphatase. Indirect sampling technique for establishing RIs, by using general laboratory stored data is of discussion; this technique is more quick and inexpensive when comparing with the direct sampling approach, but it has also been subject to some criticism due to practical inaccuracy and for producing falsely narrow RIs [Citation40,Citation41].
The higher limit for triglycerides may be due to the nonfasting state, while the lower limit for iron may indicate lifestyle with a sub-optimal diet of the Lolland-Falster population. In a Turkish study, Bakan et al. found regional differences in several RIs when comparing an Eastern Anatolian population with the general Turkish population. These findings were also associated with nutritional and environmental factors [Citation42].
Studies from Sweden have focused on the elderly population and found various laboratory tests outside the 90% CI of the NORIP [Citation43,Citation44]. Our finding on the upper limit for albumin in females and males ≥70 years is in concordance with these studies but the methods, and definitions of a healthy population varied across the studies and also, instrument bias could partly explain this finding. When comparing our overall findings with NORIP, some differences, including lower iron and albumin, and higher ALAT, triglycerides, alkaline phosphatase and creatinine in men could be linked to the fact, that the LOFUS reference population was not as healthy as the NORIP population. However, applying the almost same inclusion criteria, we believe our reference population was valid for the present study. Furthermore, it is important to keep in mind that some statistical methods on outlier removal and transformation were performed differently than in NORIP. To our knowledge, we adopted the most recent IFCC recommendations on the statistical methods for the calculation of RIs. Nonetheless, as many of our results are rather similar to NORIP RIs, we believe that the effect of these choices was relatively small.
Strength and limitations
The strength of this study is the recruitment of the study population over a three-year period and, the low economical costs using an a posteriori method for establishing RIs. Limitations include lack of data on some variables used by the NORIP to define the healthy population due to the a posteriori sampling, but as stated in IFCC-CLSI guidelines: ‘Health is a relative condition lacking a universal definition. Defining what is considered healthy becomes the initial problem in any study’ [Citation12]. It was a limitation that we had only nonfasting blood samples from LOFUS, but as the non-fasting state is more practical in everyday clinical work, this could also be considered as a strength since it does not necessitate a more complicated scheme for blood sampling. The reference individuals in LOFUS were also not completely evenly distributed by age groups as is the case in NORIP; LOFUS participants had an age distribution with 19%, 14%, 23%, 23% and 20%, respectively, for the age-groups 18–29, 30–39, 40–49, 50–59, and 60+ years. Apart from a slight deficit in the age-group 30–39 years, the distribution across age-groups was thus fairly equal.
Furthermore, we cannot rule out the possibility that some of the persons classified as healthy had subclinical disease. Finally, although invitation to LOFUS was population-based, only 34.1% of invited persons participated halfway through the data collection period [Citation45], however, we assume that the attendees were more healthy than nonparticipants [Citation46] so for constructing a reference population, this might constitute a minor issue.
Conclusion
In the Lolland-Falster Health Study, approximately 20% of participants were healthy and qualified for inclusion in the establishment of RIs. Several of the locally established RIs differed considerably from the common NORIP RIs. Our study stressed the difficulty in establishing generalizable RIs for clinical use.
Supplemental Material
Download MS Word (34.2 KB)Acknowledgment
LOFUS is a collaboration between, Region Zealand, Nykøbing Falster Hospital, Lolland and Guldborgsund municipalities. The authors are grateful for permission to use the LOFUS data however, LOFUS bears no responsibility for the analysis or the interpretation conducted within this study. The authors thank the inhabitants of Lolland-Falster and the LOFUS staff for their commitment to the study. Thank you to Mads Skytte Rasmussen, Naestved Hospital for your help and support.
Disclosure statement
No potential conflict of interest was reported by the author(s).
Data availability statement
Data from the study can be made available via Region Zealand following the Danish Data Protection Regulation.
Additional information
Funding
References
- Jones G, Barker A. Reference Intervals. Clin Biochem Rev. 2008;29:S93–S97.
- Tekkeşin N, Bekoz H, Tükenmez F. The largest reference range study for hematological parameters from Turkey: a case control study. JCEI. 2014;5(4):548–552.
- Ichihara K, Ozarda Y, Barth JH, Committee on Reference Intervals and Decision Limits, International Federation of Clinical Chemistry and Laboratory Medicine, et al. A global multicenter study on reference values: 1. Assessment of methods for derivation and comparison of reference intervals. Clin Chim Acta. 2017;467:70–82.
- Rustad P, Felding P, Lahti A, et al. Descriptive analytical data and consequences for calculation of common reference intervals in the Nordic Reference Interval Project 2000. Scand J Clin Lab Invest. 2004;64(4):343–370.
- Rustad P, Felding P, Franzson L, et al. The Nordic Reference Interval Project 2000: recommended reference intervals for 25 common biochemical properties. Scand J Clin Lab Invest. 2004;64(4):271–284.
- Nordin G, Mårtensson A, Swolin B, et al. A multicentre study of reference intervals for haemoglobin, basic blood cell counts and erythrocyte indices in the adult population of the Nordic countries. Scand J Clin Lab Invest. 2004;64(4):385–398.
- Mårtensson A, Rustad P, Lund H, et al. Creatininium reference intervals for corrected methods. Scand J Clin Lab Invest. 2004;64(4):439–441.
- Lykkeboe S, Nielsen CG, Christensen PA. Indirect method for validating transference of reference intervals. Clin Chem Lab Med. 2018;56(3):463–470.
- Lilleng H, Johnsen SH, Wilsgaard T, et al. Are the currently used reference intervals for creatine kinase (CK) reflecting the general population? The Tromsø Study. Clin Chem Lab Med. 2011;50(5):879–884.
- Kristiansen S, Friis-Hansen L, Antonio Juel Jensen C, et al. Verification study on the NORIP LDH reference intervals with a proposed new upper reference limit. Scand J Clin Lab Invest. 2018;78(5):421–427.
- Jepsen R, Egholm CL, Brodersen J, et al. Lolland-Falster Health Study: Study protocol for a household-based prospective cohort study. Scand J Public Health. 2020;48(4):382–390.
- Clinical and Laboratory Standards Institute. EP28-A3c document, Defining, establishing and verifying reference intervals in the clinical laboratory: approved guideline. 3rd ed., vol. 28, No. 30, 2010. (ISBN 1-56238-682-4)
- Egholm CL, Packness A, Stokholm J, et al. Questionnaire development for the Lolland-Falster Health Study, Denmark: an iterative and incremental process. BMC Med Res Methodol. 2020;20(1):52
- European Society of Hypertension-European Society of Cardiology Guidelines Committee 2003 European Society of Hypertension-European Society of Cardiology guidelines for the management of arterial hypertension. J Hypertens. 2003;21(6):1011–1053.
- World Health Organization: Obesity: Preventing and Managing the Global Epidemic. Report of a WHO Consultation on Obesity. 1998. Geneva: WHO, Geneva, 3–5 June 1997. WHO Technical Report Series 894.
- Warnick GR, Knopp RH, Fitzpatrick V, et al. Estimating low-density lipoprotein cholesterol by the Friedewald equation is adequate for classifying patients on the basis of nationally recommended cutpoints. Clin Chem. 1990;36(1):15–19.
- Dansk Institut for Ekstern Kvalitetssikring for Laboratorier i Sundhedssektoren [Danish Institute for External Quality Assurance for Laboratories in the health sector], https://www.deks.dk/en. (accessed 15 August 2020). Danish
- Dansk Cardiologisk selskab [Danish Association for Cardiology]. Clinical Guidelines. Dyslipidemia. https://www.cardio.dk/dyslipidaemi2., 2019, (accessed 5 August 2019. Danish).
- Solberg HE. Approved recommendation (1987) on the theory of reference values. Part 5. Statistical treatment of collected reference values. Determination of reference limits. J Clin Chem Clin Biochem. 1987;170(2–3):p13–p32.
- Solberg HE. The IFCC recommendation on estimation of reference intervals. The RefVal Program. Clin Chem Lab Med. 2004;42(7):710–714.
- Manly BFJ. Exponential Data Transformations. J R Stat Soc. Series D (the Statistician). 1976;25(1):37–42.
- John JA, Draper NR. An Alternative Family of Transformations. J R Stat Soc. Series C (Applied Statistics). 1980;29:190–197.
- Hyndman RJ, Fan Y. Sample Quantiles in Statistical Packages. Am Stat. 1996;50:361–365.
- Linnet K. Nonparametric estimation of reference intervals by simple and bootstrap-based procedures. Clin Chem. 2000;46(6 Pt 1):867–869.
- R Core Team 2019. R: A language and environment for statistical computing. R Foundation for Statistical Computing., Vienna, Austria. Available from: https://www.R-project.org/.
- Wickham H, Averick M, Bryan J, et al. Welcome to the tidyverse. JOSS. 2019;4(43):1686.
- Canty A, Ripley B. Boot: Bootstrap R (S-Plus) Functions. R Package Version. 2017;1:3–20.
- Davison AC, Hinkley DV. 1997. Bootstrap Methods and Their Applications. Cambridge University Press, Cambridge.
- Maechler M, Rousseeuw P, Croux C, et al. Robustbase: Basic Robust Statistics R package version 0.93-6. 2020. Available from: http://CRAN.R-project.org/package=robustbase.
- Komsta L, Novomestky F. Moments: Moments, cumulants, skewness, kurtosis and related tests. R package version 0.14. 2015. Available from https://CRAN.R-project.org/package=moments.
- Gross J, Ligges U. Nortest Tests for Normality. R package version 1.0-4. 2015. Available from https://CRAN.R-project.org/package=nortest.
- Bergholdt HKM, Bathum L, Kvetny J, et al. Study design, participation and characteristics of The Danish General Suburban Population Study. Dan Med J. 2013;60(9):A4693.
- Ellervik C, Tybjaerg-Hansen A, Nordestgaard BG. Total mortality by transferrin saturation levels: two general population studies and a metaanalysis. Clin Chem. 2011;57(3):459–466.
- Ruiz-Castell M, Kandala N-B, Kuemmerle A, et al. Hypertension burden in Luxembourg: Individual risk factors and geographic variations, 2013 to 2015 European Health Examination Survey. Medicine (Baltimore). 2016;95(36):e4758
- Mackenbach JP, Cavelaars AE, Kunst AE, et al. Socioeconomic inequalities in cardiovascular disease mortality; an international study. Eur Heart J. 2000;21(14):1141–1151.
- Kandala NB, Tigbe W, Manda SO, et al. Geographic variation of hypertension in sub-saharan Africa: a case study of South Africa. Am J Hypertens. 2013;26(3):382–389.
- Danmarks Statistik [Statistics Denmark], http://www.statistikbanken.dk/10015. (accessed 17 December 2019. Danish.
- Blaakilde AL, Hansen BH, Olesen LS. Health Profile 2017 for Region Zealand and municipalities - “How are you?”. Sorø, Danmark: Region Zealand, 2018 (1-6-2019). Danish.
- Region Sjaellands Dokumentportal [Region Zealand document portal], http://www.dok.regionsjaelland.dk, document 4,41,339 and 4,48,487 accessed 20 November 2020. Danish
- Ichihara K, Boyd JC, IFCC Committee on Reference Intervals and Decision Limits (C-RIDL)An appraisal of statistical procedures used in derivation of reference intervals. Clin Chem Lab Med. 2010;48(11):1537–1551. 35.
- Siest G, Henny J, Grasbeck R, et al. The theory of reference values: an unfinished symphony. Clin Chem Lab Med. 2013;51(1):47–64.
- Bakan E, Polat H, Ozarda Y, et al. A reference interval study for common biochemical analytes in Eastern Turkey: a comparison of a reference population with laboratory data mining. Biochem Med (Zagreb). 2016;26(2):210–223.
- Carlsson L, Lind L, Larsson A. Reference values for 27 clinical chemistry tests in 70-year-old males and females. Gerontology. 2010;56(3):259–265.
- Helmersson-Karlqvist J, Ridefelt P, Lind L, et al. Reference values for 34 frequently used laboratory tests in 80-year-old men and women. Maturitas. 2016;92:97–101.
- Jepsen R, Wingstrand A, Abild SL, et al. Socio-economic determinants of participation in the Lolland-Falster health study. J Public Health. 2019;17:14034948187996.
- Larsen SB, Dalton SO, Schüz J, et al. Mortality among participants and non-participants in a prospective cohort study. Eur J Epidemiol. 2012;27(11):837–845.