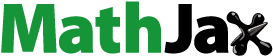
ABSTRACT
The conversion between the two different systems, ISSS and FAO/USDA, of particle size distribution and soil texture classification is useful to characterize soil physical properties and usage of each published. The objective of this study is to test some functions that have been published for conversion from ISSS to FAO/USDA system for Japanese paddy soils and to select the best method. We tested the topsoils of 267 Japanese paddy fields using the log-linear method, log-normal method, multiple regression method, Skaggs et al.’s method, and Minasny and McBratney’s method. The least AIC was obtained using multiple regression method, and the equation derived was given as follows:
siFAO/USDA = 1.305siISSS + 0.396fsISSS−0.100csISSS − 12.323
where si, fs, and cs are the percentage of silt, fine sand, and coarse sand respectively; ISSS and FAO/USDA is the fractionation system; and its RMSE was 3.1%. For the case that only the total sand content (s) is available instead of fine sand and coarse sand, the following equation was obtained:
siFAO/USDA = 1.533siISSS + 0.314sISSS − 20.903 (RMSE = 3.7%)
Among the non-empirical methods, the best estimation method was Skaggs et al.’s method, and its RMSE was 3.3%. The soil texture classification by FAO/USDA system using estimated particle size fractions by the above equation can be classified to correct categories. The accuracy ratio of the classification was 93-97%.
1. Introduction
There are two major soil particle size fraction systems in the world, the system of International Soil Science Society (ISSS) and the system of FAO/USDA (Minasny and McBratney Citation2001). The particle size limit for fractionation of each system is as follows:
ISSS FAO/USDA
clay <2 μm <2 μm
silt 2–20 μm 2–50 μm
sand 20–2000 μm 50–2000 μm
(fine sand 20–200 μm)
(coarse sand 200–2000 μm)
In addition, there are various soil texture classifications in the world (). While the common classification system used in Japan is that of ISSS, the difference in the classification of particle size fractions and texture from that of FAO/USDA forced inconvenience for the immediate adoption of past outcomes based on FAO/USDA system. For instance, Allen et al. (Citation1998) show the relationship between soil texture and field capacity and wilting point (Table 19 in Allen et al.) using the FAO/USDA soil texture classification. It is hard to use these data in Japan because of the two different classification systems.
Figure 1. Ternary diagram of soil texture classification by ISSS and FAO/USDA system (n = 267). Every used soil is plotted by each particle size fraction and texture classification
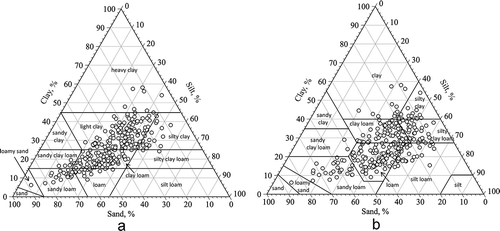
To overcome this problem, several studies are available which support the conversion from one system to the other system (Buchan Citation1989; Shirazi, Boersma, and Johnson Citation2001; Shirazi, Boersma, and Hart Citation1988). The conversion functions from ISSS system to FAO/USDA system are published for the soils in Australia (Minasny and McBratney Citation2001), Bulgaria (Rousseva Citation1997), China (Wei et al. Citation2014), Netherland, and Germany (Nemes et al. Citation1999). However, no study has been reported for conversion functions using Japanese paddy soils. Recently, Global Soil Partnership (GSP) proposes harmonizing soil analytical data for comparative analysis to manage soil resources. According to Pillar five working group of GSP (Citation2017), ‘harmonization could be seen as the next step to standardization, provides the ability to describe, sample, classify, and analyze the soil in a way that allows the use of the results for later scientific use.’ Comparison and evaluation of past attempts to conversion of two texture systems could be valuable as a case study of harmonization of soil data in Japan.
The objective of this study is to test whether the functions that have been published for conversion from ISSS to FAO/USDA system can estimate correct value in the Japanese soil and to select the best method for Japanese paddy soils. Furthermore, we also summarize the relationship between soil textures in FAO/USDA system and some corresponding soil moisture properties (i.e., field capacity and wilting point) in Japanese paddy soils.
2. Materials and Methods
2.1. Materials, soil particle size distribution, and classification of soil texture
For the study, we collected 267 topsoil samples from the eastern parts of many Japanese paddy fields (). The soil type of each soil sample was determined using e-SoilMap (https://soil-inventory.dc.affrc.go.jp/eSoilMap.html), which indicates the soil type using the comprehensive soil classification system of Japan First Approximation (Obara et al. Citation2011; ). The majority of our soil samples belong to Lowland soil type. Of the 267 soil samples, Lowland soil comprised 69% (183), and Organic soils comprised 13% (34). This is a typical distribution of soil type of paddy soils in Japan.
Table 1. Distribution of soil groups of used soils by comprehensive soil classification system of Japan First Approximation (Obara et al. Citation2011)
For pretreatment, the soil organic matter in used soils was removed using H2O2. Dispersion technique was performed using sodium hexametaphosphate for non-volcanic ash soils, and ultrasonic treatment was performed for volcanic ash soils (Gee and Or Citation2002). Then, soil particle size distributions were obtained both as ISSS fractionation system and as FAO/USDA fractionation system. In addition, only for fractionation by ISSS system, fine sand, and coarse sand were separated. The particle size was fractionated using wet sieving for particles of size limit larger than 50 µm and using pipette method for particles of lower size limit (Gee and Or Citation2002).
2.2. Evaluation of different procedures to interpolate particle size distribution
To interpolate particle size distribution, we evaluated five representative procedures, namely log-linear conversion (Nemes et al. Citation1999), log-normal conversion (Shirazi and Boersma Citation1984; Buchan Citation1989), multiple regression, Skaggs et al.’s (Citation2001) method, and Minasny and McBratney’s (Citation2001) method.
Log-linear model is a method used to predict unknown particle fraction from the relationship as EquationEquation (1)(1)
(1) :
where CP is the cumulative amount of particle ratio, i is the upper limit of each fraction (mm), and a and b are the parameters. We calculated a and b by regression for each soil and estimated siFAO/USDA.
Log-normal regression is a model based on the assumption that particle size distribution can be expressed as log-normal equation (Buchan Citation1989).
where i is the upper limit of each fraction, f(i) is the log-normal density, x = log(i), and a and b are the logarithm of geometric mean particle size diameter and geometric particle size standard deviation. In our study, siFAO/USDA was calculated by EquationEquation (2)(2)
(2) and normal distribution function in a spreadsheet application of computers.
The multiple linear regression was also conducted. Each parameter of the explanatory variables was determined using the cross-validation method. First, the total data were split into four groups randomly. And three of the four groups were used for regression analysis and the rest one group was used for validation of the regression equation. This process was conducted four times and the average of the parameters was obtained.
The selection of explanatory variables is important for the above three estimation methods. Logically, we can use a freely selected combination of particle size from four limits (cs, fs, si, and cl. Hereafter we call it ‘case of fs–cs’). However, we are often forced to use total sand content only for the upper limit because fine sand and coarse sand values are not shown as separated fractions (hereafter we call it ‘case of s’). In this study, we examine every combination for the case of fs–cs and s. Because the total of each fraction is 100%, used number of explanatory variables for multiple regression must be available fraction minus 1. For multiple regression, we examined every combination satisfying the above conditions, including single regression.
Skaggs et al. (Citation2001) proposed a simple empirical method to describe particle size distribution.
We used cl, siISSS, and fsISSS to obtain parameters a and b and siFAO/USDA in each soil.
We also tried the method of Minasny and McBratney (Citation2001) that was proposed as the conversion equation from ISSS system to FAO/USDA system using 1210 samples including Australian soils, UNSODA, GRIZZLY, and the USDA–NRCS Soil Survey Laboratory data. The model is shown in EquationEquation (5)(5)
(5) :
where s represents the total sand contents.
Each model was evaluated based on the accuracy of estimation of silt fraction (siFAO/USDA), and we mainly compared the Root Mean Squared Error (RMSE) value of each method to choose the best estimator and also calculated Akaike’s Information Criterion (AIC) to avoid overfitting.
2.3. Estimation of FAO/USDA type soil texture classification
We classified used samples by FAO/USDA system with both measured and estimated particle size distribution. The results were shown as an accuracy ratio that was the ratio that soil texture classified with estimated particle size distribution correspond to soil texture classified with measured particle size distribution correctly.
3. Results
3.1. Distribution of soil particle size and classification of soil texture
The distribution of soil particle size of used soil spreads in a wide range (). When measured using the system of ISSS, the sand, silt, and clay fractions ranged from 4.3% to 88.4%, from 5.4% to 55.5%, and from 6.2% to 58.1%, respectively. When measured using the system of FAO/USDA, the sand and silt fractions ranged from 2.3% to 85.6% and from 8.2% to 67.4%, respectively. Because the fraction of silt is higher in the FAO/USDA system, the distribution of plots shifted to silt fraction slightly (). There are no samples classified by sandy clay, sand, or silt loam with ISSS system, and there are no samples classified by sandy clay, sand, or silt with FAO/USDA system.
3.2. Comparison of conversion methods
shows the comparison of silt contents by measured and estimated values. The selected explanatory variables and the AIC values of each estimation method are indicated in . In log-linear, log-normal, and multiple regression, the least AIC combination among all combinations is indicated for both ‘the case of fs–cs’ and ‘the case of s.’ The least AIC is obtained by multiple regression method for both ‘the case of fs–cs and s.’ In conclusion, the two regression equations were obtained as follows:
Table 2. Comparison of various methods to estimate silt contents by FAO/USDA system from ISSS soil particle size distribution (n = 267)
Figure 3. Comparison of various methods to estimate silt contents of FAO/USDA (siFAO/USDA) from ISSS soil particle size distribution. The alphabet in the parenthesis in the first line of each graph correspond to the symbols in
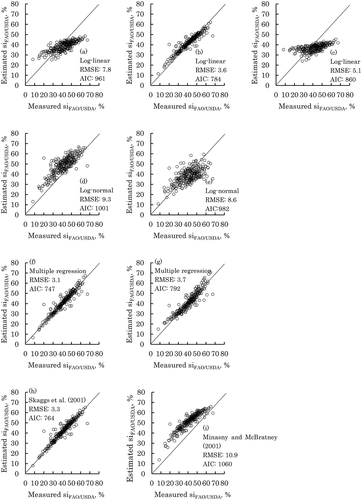
The best equation was EquationEquation (8)(8)
(8) , and the RMSE calculated by this equation was 3.1%. The standard deviations of each coefficient of EquationEquations (8)
(8)
(8) and (Equation9
(9)
(9) ) obtained by cross-validating four times are shown in .
Table 3. The standard deviations (sd) and coefficient of variations (cv) of four cross-validations by using multiple regression EquationEquations (8)(8)
(8) and (Equation9
(9)
(9) )
The estimated silt contents using log-linear method spread in a narrow range (). The best combination of variables for this method is cl + si, fs, and cs (e.g., using a size limit of 20 µm, 200 µm, and 2000 µm, respectively). The log-normal method can estimate the measured values poorly. Skaggs et al.’s method (Citation2001) worked well but the AIC is slightly less than that of EquationEquation (8)(8)
(8) . The estimation of values using Minasny and McBratney’s (Citation2001) study overestimated the measured values.
3.3. Estimation of FAO/USDA type soil texture classification
As used samples were classified by FAO/USDA classification system, they were grouped into nine categories (). The classification using ISSS data with estimation by methods of EquationEquations (8)(8)
(8) and (Equation9
(9)
(9) ), which are the best estimation methods by case of fs–cs and s, respectively, was conducted. Both equations can estimate soil texture classification with high accuracy. Estimation by EquationEquation (8)
(8)
(8) corresponded to correct classification by 97% of accuracy ratio. And it is 93% using EquationEquation (9)
(9)
(9) . The accuracy of estimation about silty clay loam was the least in both equations.
Table 4. Accuracy ratio of classification of soil texture to FAO/USDA system from estimation by multiple regression of EquationEquation (8)(8)
(8) and EquationEquation (9)
(9)
(9) with ISSS soil particle size distribution
4. Discussions
4.1. Recommended method for conversion from ISSS to FAO/USDA system
According to the results of RMSE and AIC values, the method that can convert from ISSS to FAO/USDA system the most precisely is the multiple regression method. The order of RMSE in each method is completely consisting of that of AIC, it means that overfitting does not affect adversely the values of RMSE. Minasny and McBratney (Citation2001) also tested multiple regression and log-linear conversion for 1610 samples including Australian databases, UNSODA, GRIZZLY, and USDA–NRCS, and concluded that empirical regression is the best method to estimate. However, the proposed equation by Minasny and McBratney (Citation2001) did not show good RMSE and AIC values in our samples, and almost all estimated values are over the measured values ( [i]). As the reason that the equation proposed by Minasny and McBratney (Citation2001) did not give a good result for Japanese paddy soils is unclear, we recommend using our empirical regression equations given as EquationEquations (8)(8)
(8) or (Equation9
(9)
(9) ) for Japanese paddy soils.
Empirical equations have the nature that obtained coefficients cannot be generalized unquestionably. The standard deviations of each coefficient obtained by cross-validating four times are shown in . The results indicate that the standard deviation was relatively high in the coefficient of coarse sand and intercept. Adaptability of the equations may have an unknown limit. The alternative to avoid this problem, which is essential in the empirical equation, is the use of Skaggs et al.’s (Citation2001) method. The RMSE obtained by this method (3.3%) is very close to that of the multiple regression (3.1%). If users avoid using an empirical function, this method is used as a probable alternative. Four fractions of particle size are needed for this method, namely only the case of fs–cs is available to estimate. This could be one of the disadvantages.
Classifications by FAO/USDA soil texture using estimated particle size fractions can mostly correspond to these using measured particle size fractions. indicates the required accuracy for the estimated classification. As the accuracy ratio in silty clay loam and silt loam is lower (), there is a tendency that accuracy ratio decreases in the soils in which silt contents are over about 55% (). One advantage of using FAO/USDA classification would be that the soil texture categorization can characterize the soil particle size distribution well because the particle size distributions in the ternary diagram of FAO/USDA system spread in a wider range than that of ISSS (); nearly all soils are classified as light clay or clay loam by the ISSS system.
4.2. Corresponding of some soil moisture properties
Some specific values about water retention properties are often summarized using FAO/USDA soil texture system. For instance, Allen et al. (Citation1998) show the relationship between soil texture and field capacity and wilting point (Table 19 in Allen et al.). It would be convenient to show these relationships for Japanese paddy soils, particularly for field capacity, because field capacity of Japan is determined as water contents at −3.1 kPa or −6.3 kPa water potential (Hasegawa Citation1997), which is quite different from international common value, −33 kPa (Minasny, McBratney, and Bristow Citation1999). In our dataset, 199 data are available to show the relationship between soil texture and water contents at −3.1 kPa, and 244 data can be available to show the relationship between soil texture and water contents at the wilting point, −1500 kPa. In addition, Nakano et al. (Citation2018) show further 151 and 173 data that can relate ISSS particle size fraction with water content at −3.1 kPa and −1500 kPa, respectively. We converted the data of Nakano et al. (Citation2018) to FAO/USDA classification system by EquationEquation (8)(8)
(8) , merged to our dataset, and then summarized the relationship between the water contents at these water potential and soil texture (). is rearranged from for reading each value easily.
Table 5. The relationship between soil texture classification by FAO/USDA and some corresponded moisture properties in Japanese paddy soils and comparison to the ‘typical values’ given by Allen et al. (Citation1998)
Figure 4. Boxplots for the relationship between soil texture categorized by FAO/USDA classification system and field capacity (−3.1 kPa; n = 350) or wilting point (−1500 kPa; n = 417) in Japanese paddy soils
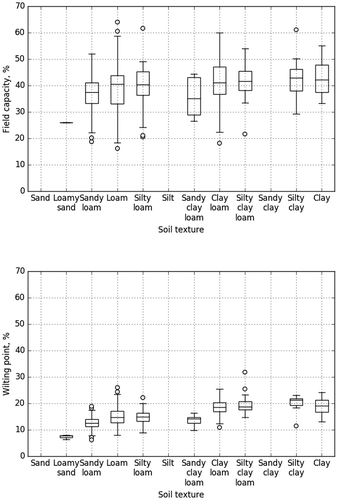
It is clearly shown in that wilting point (volumetric water content at −1500 kPa water potential) is influenced by soil texture, and the results are very close to the ‘typical values’ given by Allen et al. (Citation1998). The soil texture of FAO/USDA system can be classified by texture-by-feel analysis easily (Thien Citation1979) and wilting point could be estimated by the classified soil texture approximately using even in Japanese paddy soils. It is one of the advantages to use FAO/USDA system. On the other hand, the distributions of values of field capacity (−3.1 kPa) are quite higher than the results of Allen et al. (Citation1998) and seem to be difficult to categorize by soil texture (). The field capacity in Japan (−3.1 kPa) is more independent of soil texture than that in international common value (at −33 kPa). The estimation of field capacity in Japanese paddy field still remains for further researches.
Acknowledgments
The authors thank Mr. Yuji Ohashi (Hokkaido Research Organization), Tadayuki Kudo (Agriculture Research Institute, Aomori Prefectural Industrial Technology Research Center), Norimasa Tanikawa (Agriculture Research Institute, Aomori Prefectural Industrial Technology Research Center), Tomohiro Konno (Miyagi Prefectural Furukawa Agricultural Experiment Station), Yuki Kato (Yamagata Integrated Agricultural Research Center), Tadashi Ando (Yamagata Integrated Agricultural Research Center), Makiko Moriya (Yamagata Integrated Agricultural Research Center), Akira Matsuda (Yamagata Integrated Agricultural Research Center), Yoshihumi Nagumo (Niigata Agricultural Research Institute), Masaharu Aoki (Nagano Agricultural Experiment Station), Tadayoshi Uehara (Nagano Agricultural Experiment Station), Kiyoshi Okamoto (Nagano Vegetable and Ornamental Crops Experiment Station), Hideyuki Umemoto (Ishikawa Agriculture Research Center), Yoshihiro Mukai (Ishikawa Agriculture Research Center), and Kenji Nakamura (Ibaraki-ken Agricultural Experiment Institute) for sending soil samples and field capacity data. They also thank Ms. Yuko Itoh for her help in soil analysis.
Disclosure statement
No potential conflict of interest was reported by the authors.
Additional information
Funding
References
- Allen, R. G., L. S. Pereira, D. Raes, and M. Smith. 1998. “Crop Evapotranspiration: Guidelines for Computing Crop Water Requirements.” In: FAO Irrigation and Drainage Paper No. 56. Rome, Italy: FAO.
- Buchan, G. D. 1989. “Applicability of the Simple Lognormal Model to Particle-size Distribution in Soils.” Soil Science 147 (3): 155–161. doi:10.1097/00010694-198903000-00001.
- Gee, G. W., and D. Or. 2002. “Particle-size Analysis.” In Method of Soil Analysis, edited by J. H. Dane and G. C. Topp, 255–293. Madison: Wisconsin.
- Hasegawa, S. 1997. “Water Retention A.” In Methods of Analysis of Soil Environment, edited by Committee of edition of Methods of analysis of Soil environment, 48–52. Tokyo: Hakuyu-Sha.
- Minasny, B., and A. B. McBratney. 2001. “The Australian Soil Texture Boomerang: A Comparison of the Australian and USDA/FAO Soil Particle Classification Systems.” Australian Journal of Soil Research 39 (6): 1443–1451. doi:10.1071/SR00065.
- Minasny, B., A. B. McBratney, and K. L. Bristow. 1999. “Comparison of Different Approaches to the Development of Pedotransfer Functions for Water-retention Curves.” Geoderma 93 (3–4): 225–253. doi:10.1016/S0016-7061(99)00061-0.
- Nakano, K., T. Takahashi, R. Nira, Y. Ohashi, T. Kudo, N. Tanikawa, T. Konno, et al. 2018. “The Relationships between Soil Properties in Farmer’s Field and Soybean Yield in Survey in 16 Prefectures the Relationship between Yield and Soil Physical, and Precipitation Properties, Abstracts of the Annual Meeting.” Japanese Society of Soil Science and Plant Nutrition 64: 85.
- Nemes, A., J. H. M. Wosten, A. Lilly, and O. J. H. Voshaar. 1999. “Evaluation of Different Procedures to Interpolate Particle-size Distributions to Achieve Compatibility within Soil Database.” Geoderma 90 (3–4): 187–202. doi:10.1016/S0016-7061(99)00014-2.
- Obara, H., T. Ohkura, Y. Takata, K. Kohyam, Y. Naejima, and T. Hamazaki. 2011. “Comprehensive Soil Classification System of Japan First Approximation.” Bulletin of National Institute for Agro-Environmental Sciences 29: 1–72. in Japanese.
- Pillar five working group of GSP. 2017. “Plan of Action for Pillar Five of the Global Soil Partnership. Harmonization of Methods, Measurements and Indicators for the Sustainable Management and Protection of Soil Resources.” http://www.fao.org/3/a-az922e.pdf
- Rousseva, S. S. 1997. “Data Transformations between Soil Texture Schemes.” European Journal of Soil Science 48 (4): 749–758. doi:10.1046/j.1365-2389.1997.00113.x.
- Shirazi, M. A., and L. Boersma. 1984. “A Unifying Quantitative Analysis of Soil Texture.” Soil Science Society of America Journal 48 (1): 142–147. doi:10.2136/sssaj1984.03615995004800010026x.
- Shirazi, M. A., L. Boersma, and W. A. Hart. 1988. “Unifying Quantitative Analysis of Soil Texture: Improvement of Precision and Extension of Scale.” Soil Science Society of America Journal 52 (1): 181–190. doi:10.2136/sssaj1988.03615995005200010032x.
- Shirazi, M. A., L. Boersma, and C. B. Johnson. 2001. “Particle-size Distribution: Comparing Texture Systems, Adding Rocks, and Predicting Soil Properties.” Soil Science Society of America Journal 65 (2): 300–310. doi:10.2136/sssaj2001.652300x.
- Skaggs, T. H., L. M. Arya, P. J. Shouse, and B. P. Mohanty. 2001. “Estimating Particle-size Distribution from Limited Soil Texture Data.” Soil Science Society of America Journal 65 (4): 1038–1044. doi:10.2136/sssaj2001.6541038x.
- Thien, S. J. 1979. “A Flow Diagram for Teaching Texture-by-feel Analysis.” Journal of Agronomic Education 8: 54–55.
- Wei, S., D. YongJiu, C. Garcia-Gutierez, and Y. Hua. 2014. “Particle-size Distribution Models for the Conversion of Chinese Data to FAO/USDA System.” The Scientific World Journal 2014: 1–11.