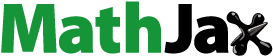
The book belongs to the Statistics in the Social and Behavioral Sciences Series, and it is devoted to problems of political sciences, spatial voting theory, measurement of political parties’ positions on multiple issues, methodologies for comparative politics in aspects of ideology, psychology, and sociology. The authors are renown scientists from several north American universities, and in this 2nd edition of the book they present more methods for structuring relations among the variables describing political phenomena and processes; particularly, an entire chapter is added on the Bayesian estimations. In Preface, the authors note that the spatial voting models have a long history, and virtually all political conflicts can be represented in spatial terms of left-right division, which was originated in the Jacobins and Girondists sitting in the National Assembly in times of revolution in France, and can be observed in the U.S. Congress as well. The data modeling and visualization is performed by various multivariate statistical techniques implemented in the R software. The book consists of six chapters, each divided to many sections and subsections presenting theory and examples of application.
Chapter 1, Introduction, explains that the term “spatial” can be used not only in the actual spatial statistics for the modeling of physically proximate units, but also for finding the relative geometric positions or distances among the points representing political relational data. In political science, a spatial model can be useful in measuring latent characteristics built from the observed indicators, for example, a level of a respondent conservatism can be defined by the position on the ideological dimension of the political perceptual map. Such mapping helps to evaluate a level of polarization in legislature and electorates, ideological makeup of campaign contributors, and quality of representation of voter preferences. The spatial models contain different methods developed in the fields of psychology, economics, and political science. Particularly, they include various techniques of multidimensional scaling (MDS) developed to analyze similarity and preferential choice data from the respondents. MDS produces spatial maps in a low-dimensional space with the axes representing latent dimensions which account for variation in the data and could serve to understanding how humans process information and make decisions. The spatial theory of voting had been founded by H. Hotelling, A. Smithies, A. Downs, D. Black, and developed in numerous works.
In the spatial theory, voters are assumed to have ideal points marking their most preferred outcome, and also to have utility functions across the range of policy alternatives that specify the amount of utility voters obtain from each outcome. Utility functions are assumed to be single-peaked, with the maximum at the voter’s ideal point, and symmetric monotonically decreasing in the distance from the ideal point, similar to the Gaussian bell-curve, quadratic or triangle concave curves. Spatial models help to clarify problems of aggregation of individual choices, including even the 18th century Marquis de Condorcet’s paradox of transitive preferences cycles, when a majority of voters prefer candidate A over candidate B, candidate B over candidate C, but also candidate C prevails over candidate A. In his famous work Social Choice and Individual Values, Arrow (1951) developed the so-called impossibility theorem proving that the Condorcet paradox, or the possibility of preferences’ cycles can occur in any aggregation method that satisfies a set of minimal conditions, such as complete and transitive individual preferences, Pareto optimality, independence of irrelevant alternatives, and non-dictatorship. Such result is troubling for democratic theorists because it contradicts to the certainty of stable majority rule. Later it was shown that the majority rule can produce a stable outcome at the location of the median voter if preferences are ordered along a single dimension, and even in a multidimensional space if the ideal points are radially symmetric around the equivalent of the median voter. However, McKelvey’s chaos theorem shows that such results do not hold in a multidimensional policy space when ideal points are distributed not radially symmetric, so in more realistic cases there is an absence of a stable equilibrium. In practical terms, it means that losers in a single-dimensional situation could raise new advantageous dimensions to upset the existing equilibrium. This political maneuver was considered by W. Riker and named her-esthetic, which is designed to strategically manipulate the choice space, for instance, in the legislative arena, for example, “killer” amendments, and other such kind of strategy for political campaigns.
The book focuses on proximity models, although there are models considering centripetal forces, directional voting to the ideal point, effects of convergence break with entry of another party, and other specific models. Empirical statistical estimations for voting spatial models use scaling methods of factor and cluster analyses to find latent dimensions which were applied to the U.S. Congress and the French Parliament. Other approaches include the so-called feeling thermometer for measuring affinity of voters for candidates and parties in 0-100 scale, NOMINATE scaling procedure for estimating ideological positions of members of Congress throughout the span of American history, a modified DW-NOMINATE generating standard errors for ideal points in bootstrap procedure, nonparametric Optimal Classification to scale political actors in various contexts, and more. The basic space theory aims to reduce the original high-dimensional observations into a low-dimensional space by projecting them into a few latent variables keeping an approximate structure of the mutual positioning of the voters. The dimensions of such a low-dimension space are referred as basic, predictive, or ideological dimensions. For example, the voters’ opinions by numerous issues can be reduced to the plane of political ideology with the horizontal x-axis of economic attitude (economic left and right are positioned to the left and to the right from the center, respectively) and the vertical y-axis of social views (social left and right positioned below and above the center, respectively), where the conservatives and liberals would group in the first and third quadrant of this map.
Chapter 2, Analyzing Issue Scales, considers measuring used in public opinion surveys to ask respondents to place themselves and political parties, candidates, and public figures on an issue. The well-known in applied psychology and sociology Likert scales are commonly used, for instance, of seven-point with endpoints of “strongly agree” and “strongly disagree.” The American National Election Study (ANES) applies seven-point scale with the endpoints labeled “extremely liberal” and “extremely conservative”, and respondents locate themselves and political stimuli on the scale. Issue scales can be used to collect both preferential (what is my position?) and perceptual (what are the positions of others?) data, which are employed in spatial voting models and in estimation of the ideal points of political figures. Guttman cumulative scaling, also known as scalogram analysis, is also used. This chapter covers several widely used methods for the analysis of issue scales included in the basicspace package in R: Aldrich-McKelvey scaling, Blackbox scaling, and Blackbox transpose scaling.
Aldrich-McKelvey (A-M) scaling is used to diminish the so-called interpersonal incompatibility, to reduce the differential item-functioning (DIF), in other words to make a sample more heterogeneous in discrepancy between the high- and low-raters. The A-M technique is derived in the closed-form solution for an individual rater adjustment. The aldmck() function in basicspace R package is described and the whole R scripts are given for several examples which include: French module of the 2009 European Election Study (EES), with graphs on density of left-right self-placement of respondents and of political parties; two issue scales used in the 1968 American National Election Study urban unrest and Vietnam War, with density of liberal-conservative distributions of opinions on different candidates. The basic space techniques of Blackbox scaling and Blackbox transpose scaling correspond to the data dimensionality reduction to low rank matrices by methods of the singular value decomposition kind. They are implemented in the blackbox() and blackbox_transpose() functions for the individual scale data of preference or perception, respectively, and demonstrated on several examples. The U.S. Party 2000 Convention Delegate Study with multiple scales is described, and the results are shown on the two-dimensional scatter plots of democrats and republicans grouping, and density plots of liberal-conservative distributions. Other examples include: Swedish parliamentary candidate survey 2010 with data on 10- parties, and distribution of the ideal points presented; data from the 2000 and 2006 Mexican modules of the Comparative Study of Electoral Systems (CSES), with the left-right placements of parties in the plane projection; data with large number of respondents from the California module of the 2010 Cooperative Congressional Election Study. The bootstrap estimates of the standard errors are obtained in these examples as well.
The Ordered Optimal Classification (OOC) method presents a nonparametric approach to Basic Space scaling technique for binary choice data in estimating a latent ideological configuration of legislators without parametric assumptions on individual utility functions or the error term. OOC allows for ordinal or combined ordinal/binary issue scale data. An example is taken from the 2004 ANES data about the respondents’ preferences on issues ranging from diplomacy and defense issues to government spending and abortion. The OOC is performed in R with use of the ooc() function in the ooc library, with the results shown in the scatter plot of the Bush vs. Kerry vote choice. Combining data from several countries could create the DIF problem, occurring because of cross-cultural differences, demographic variables, education or political sophistication, etc., that can require using the “anchoring vignettes”, described on the example of the Chapel Hill Expert Survey (CHES). Its 2010 wave consists of 118 parties and 224 experts in 14 member countries of the European Union. Over 160 experts placed all three vignette parties and between 8 and 17 experts placed each of the actual parties. The blackbox_transpose() routine estimates several dimensions from the data allowing also for missing values. The results of the DIF-corrected scale to the raw data are graphically compared, and left-moderate-right party groups are visualized on two-dimension scatter plots of the latent variables with maximum variation.
Chapter 3, Analyzing Similarities and Dissimilarities Data, focuses on the techniques of Multidimensional Scaling (MDS). It describes original data of respondents (observations) by stimuli (variables), and its compression into a square matrix of squared distances between stimuli. The classical metric MDS also double-centers the matrix, and finds its eigenproblem solution. An example of similarity between 12 countries is presented with visualization of the nations’ mutual closeness in a plane of the main eigenvectors. Other optimizing procedures are also described, particularly, the Genetic Optimization using Derivatives (GENOUD), and proposed by de Leeuw iterative Scaling by MAjorizing a COmplicated Function (SMACOF) which minimizes the Stress criterion, implemented in the rgenoud and smacof R packages, respectively.
While metric MDS methods use the similarities data represented by ratio or interval-level positions, the nonmetric MDS procedures assume that the data represent only the ordinal relationships between the stimuli, hence, in the estimated point configuration the inter-point distances reproduce the rank ordering of the observed dissimilarities. The same data on 12 nations is used to perform nonmetric MDS by the majorization algorithm with original and rotated metric via smacof package, showing that metric and nonmetric MDS yield very similar locations in biplots of the countries mutual positioning. Another example is considered on the legislative roll call data used to compute a symmetric matrix of agreement scores between each pair of legislators of the U.S. Senate in 1967–68, with two dimensions of liberal-conservative and region/civil-rights voting during this period. The scatter plots of the legislators’ mutual disposition on the scales of metric and nonmetric MDS are presented, and the corresponding Shepard diagrams of the reproduced vs observed distances are discussed. The formalism of the individual differences MDS (INDSCAL) method by Carrol and Chang is described in detail and applied to the EES data from chapter 2, with the results presented in two-dimensional plots of ideological placements of French political parties.
Chapter 4, Unfolding Analysis of Rating Scale Data, operates with rectangular matrices of the individuals on the rows and the stimuli on the columns, used in political science for feeling thermometers and propensity to vote measures. Feeling thermometer questions are used the ANES to ask respondents to rate their affect towards various political stimuli (parties, candidates, and sociopolitical groups) on a 100-point scale. Propensity to vote questions, commonly included in EES, ask respondents to rate how likely they would vote for a given party or candidate, usually on 10- or 11-point scale. Such data are the best available measures of voters’ expected utility from political alternatives, capturing the considerations that voters use to make political choices: policy positions, party labels, valence or personal affect, etc. Coombs’ unfolding model to analyze this type of preferential choice data, uses the idea of a most preferred point (ideal point) and a single-peaked preference function to account for the observed rank orderings. The unfolding analysis arranges the individuals’ ideal points and points representing the stimuli on the so-called evaluative J scale so that the distances between the ideal points and the stimuli points reproduce the observed rank orderings. Coombs called this an unfolding analysis because the researcher “unfolds” the rank orderings, and the unfolding model is an ideal point model consistent with the spatial (geometric) model of choice and well-suited to the analysis of political choice data.
The Thermometer problem is derived, the Multidimensional Least-Squares Metric Unfolding (MLSMU6) developed by Poole and the SMACOF metric unfolding procedures are described. An example is given on the data for various interest group ratings of U.S. Senators in 1981, using a function to run MLSMU6 approach in the asmcjr package, and bi-plotting the estimated configuration of Senators and 12 selected interest groups. The results reveal, for instance, that the groups are distributed along the liberal-conservative continuum, with the very liberal groups American Federation of Teachers and Americans for Democratic Action and the very conservative groups Conservative Coalition and the National Tax-payers’ Union furthest apart on this dimension. The results also show the relative ideological positions of interest groups; for example, that the National Federation of Independent Business is more moderate than the Chamber of Commerce. An alternative method of SMACOF procedure in smacofRect() function is used for 2009 European Election on the Danish module of EES data, where 1000 respondents rated their propensity to vote for each of 8 parties in 0-10 point scale of “not at all possible” to “very probable”. The biplot presents voters and parties and permits interpretation of the axes as the left-right ideology and viability directions. Features of MLSMU6 and SMACOF procedures are discussed and the latter one is recommended as having a higher recovery of the true individual and stimuli ideal points when missing data was present.
Chapter 5, Unfolding Analysis of Binary Choice Data, continues with studying behavior of legislative, judicial, and other settings where members cast a series of binary (i.e., “Yea” or “Nay”) votes. This analysis uses the assumption that legislators or other political actors such as judges or voters have ideal points in a latent policy space and vote for the policy alternative closest to it, subject to a random error. A roll call vote is understood as the product of the distance between a legislator’s ideal point and a policy proposal, and the observed roll call votes data is used to recover the locations of the legislators’ ideal points and the policy alternatives in the latent space. This is the basic idea underlying spatial analyses of binary choice data. Beginning with MacRae’s work on the structure of Congressional voting, the fields of ideal point estimation and legislative studies have grown, giving numerous insights into the structure and dynamics of legislative and judicial voting, voting in historical legislative bodies like the Constitutional Convention and contemporary political phenomena of partisan polarization. New scaling methods to perform unfolding analysis of binary choices have been developed, including parametric NOMINATE, α-NOMINATE or MCMC (Markov Chain Monte Carlo), and nonparametric Optimal Classification (OC) procedures.
Geometry and data formatting as an object of class rollcall are described and procedures employed in wnominate, anominate, pscl, MCMCpack, and oc R packages are presented in all detail with the utility functions and optimization criteria. Consideration is given on the data for the 108th (2003-2005) U.S. House with 440 Representatives conducted 843 roll calls, with the results on density plots of partisan polarization, biplots of individual roll calls and party groups, graphs of the so-called partial Coombs mesh, and histogram of cutline angles for displaying configuration of votes. Other data are taken on the 1st European Parliament 1979-1984, and the first five European Parliaments 1979-2001, with the parametric bootstrap executed to obtain standard errors for the ideal point estimates, presenting biplots for the members of the parliament and parties, with their ideal points’ confidence contours. The OC application is performed for the French National Assembly during the 4th Republic (1946-1958), with the results presented in biplots of ideal point estimations of deputies by parties. Another OC example considers 2008 American National Election by feeling thermometer data. Various modifications, such as D-, W-, DW-NOMINATE are compared with OC procedure which is preferable when the distribution of the error is unknown, but in stable legislative bodies conducting regular roll call votes with reasonable rates of voting errors, choice of scaling method has little effect on the results, that is demonstrated by the example on roll call data from the 111th U.S. Senate.
Chapter 6, Bayesian Scaling Models, is devoted to revisiting the various methods with Bayesian approach, which is convenient for working with latent variables because those can be sampled directly from the derived posterior distribution for these quantities, thus, it is easy to make inferences about functions of parameters (e.g., predicted probabilities). Another practical advantage of the Bayesian framework is that new models can be estimated with relative ease with help of JAGS, BUGS, or Stan software. The Bayesian A-M (BAM) scaling is performed using rjags package in R, calling the separate program which can also be downloaded from JAGS - Just Another Gibbs Sampler (sourceforge.io). The same example from the chapter 2 on French political parties in the 2009 EES is used, and the results of classical maximum likelihood (ML) and Bayesian procedures are compared, revealing almost identical left-right positions of political parties with the correlation 0.999, and the standard errors smaller in BAM than in bootstrap estimates for A-M. Bayesian MDS (BMDS) methods are described and applied to the same 12 nations similarities data considered in the chapter 3, with the codes given in detail and the results plotted and compared. Bayesian multidimensional unfolding is presented in formulae and applied to the 1968 ANES data discussed in the chapter 2, with comparison by SMACOF and Bayesian BFGS (Broyden-Fletcher-Goldfarb-Shanno) optimization procedure, and bi-plotting the results.
The Item Response Theory (IRT), developed in psychometrics for the measurement of skills-based tests and test subjects, has been adapted to political sciences as well. In the IRT model, a series of test questions (items) are used to measure a latent level of ability that subjects possess. The subjects are assumed to answer correctly if they have a level of ability above some threshold by each item, which is called the item difficulty parameter. The one-parameter IRT model, or the Rasch model, contains the individual ability and item difficulty variables, and the two-parameter model adds a discrimination parameter measuring how well each item discriminates subjects by their latent ability. The IRT model can be extended to the ideal point estimation of legislators if to substitute political ideology for ability (both being latent individual attributes), legislators for subjects, roll calls for test items, the difficulty parameter can be related to the cutting plane (i.e., how left- or right-wing a legislator needs to be in order to be classified as a Yea/Nay vote), and the discrimination parameter indicates how well a roll call vote separates legislators based on ideology. In contrast to the IRT testing applications focused on the estimated values of the item parameters to determine how well test items are constructed, the main interest in political sciences is usually the individual parameters defining the legislator ideal points. The Guttman scaling is closely related to the IRT as both correspond to cumulative scaling techniques. The IRT has been employed by political scientists in the CJR (Clinton-Jackman-Rivers) model to perform unfolding analysis using the random utility model with quadratic deterministic utility, where the recovered dimensions are policy dimensions, with the individuals’ ideal points and the midpoints of the Yea and Nay alternatives recovered.
Bayesian IRT methods are attractive because they allow for the joint estimation of the ability, difficulty, and discrimination parameters. The Gibbs sampler (the workhorse of the MCMC world – in words by Robert and Casella, 2010) breaks down the high-dimensional posterior density into a series of tractable components and samples from the conditional densities for each component, offering an efficient way to analyzing high-dimensional posterior densities, like those regularly produced from legislative roll call data. The extension of the two-parameter IRT model to the analysis of legislative roll call data is developed in the Bayesian parametric CJR model, with its formalism presented, and the software described. The MCMCpack package allows for Bayesian estimation of models common in the social sciences, from the linear regression to more complex models involving hierarchical regression, limited dependent variables, and the measurement of latent variables (e.g., factor analysis and IRT). The sampling of the posterior density is implemented via the C programming language, allowing for very efficient estimation. The functions MCMCirt1d() and MCMCirtKd() for the Bayesian estimation of the uni- and multi-dimensional IRT models are described. Another pscl package includes the functions to create rollcall objects in R, together with the versatile ideal() function for performing Bayesian IRT in one or more dimensions using the CJR model.
Bayesian unidimensional IRT with the MCMCirt1d() function is demonstrated using data from the 2000 Term of the U.S. Supreme Court, with the detail scripts by all steps of estimation and presentation of results on the posterior densities in the ideology scale. For example, Justices Stevens, O’Connor, Rehnquist, and Scalia occupy ideological positions directed from the liberal to the conservative locations. Running multiple Markov chains in MCMCpack and pscl packages, and the diagnostic procedure Potential Scale Reduction Factor (PSRF) are described. The point estimates by posterior means and 95% credible intervals for all nine Justices are plotted and discussed.
To show how to plot voting patterns on individual roll call votes in the Bayesian IRT framework, the data is taken from the 100th US Senate’s vote on the confirmation of President Reagan’s nominee Judge Robert H. Bork to the U.S. Supreme Court. The Bayesian IRT convergence diagnostic is considered, and the probit model is applied to estimate the voting choice probability of each legislator. The multidimensional IRT is demonstrated on the roll call data from the 89th U.S. Senate during 1967-1969 period of congressional history, used also in the chapter 3. Two-dimensional plotting of the 90% HPD (highest posterior density) is shown, with the x-axis of liberal-conservative ideology, and the y-axis of regional/racial differences in legislators’ views. In the same scales, the results on the legislators and party groups’ locations from the IDEAL and W-NOMINATE application of different packages and functions are presented. Problems of identification of the model parameters and a minimally restricted solution are also discussed.
Different functional forms to model legislators’ utility functions are described, including the Gaussian normal function and quadratic function used in DW-NOMINATE and in Bayesian IRT, respectively. The so-called α-NOMINATE uses a mixture model that nests the Gaussian and quadratic forms in the legislator utility function with an added α-parameter. This approach is applied via the anominate package in R for analyzing the structure of legislators’ utility functions in the 111th U.S. Senate.
The IRT in application to the analysis of ordinal choice data and the measurement of latent ideology over time in dynamic models are also considered. The Bayesian mixed factor analysis model implemented in the MCMCordfactanal() function in MCMCpack package is equivalent to the standard two-parameter IRT model with a probit link, for the polytomous or ordinal data. This function is applied to the data from the 2004 ANES, used also in the chapters 2 and 4. The results of the ordinal IRT scaling of responses is presented in the scatter plot of ideal points in voting for Bush vs Kerry, and the outcomes of the probit model choice probability estimates are given by Democratic, Independent, and Republican parties. Estimating latent constructs over time and exploiting the temporal structure is accomplished by incorporating a random walk prior on the latent variable, that is demonstrated using data from the Comparative Manifestos Project which codes political party manifestos in every election across roughly 50 countries since 1945.
The massive and novel data sets appear in more exotic quantities such as word usage by political candidates and parties or the network of relationships between political actors on social media platforms, when researchers encounter heavy computational costs and convergence issues. For analyzing large data sets, the EM (Expectation-Maximization) algorithm is efficient in estimating Bayesian IRT models, including the basic two-parameter model for binary choice data and its extension to ordinal choice data. For them, a closed-form version of the EM algorithm is derived that exactly maximizes the posterior distributions of the unknown parameters. In cases of dynamic, hierarchical, textual, and network IRT models, a closed-form EM algorithm is not possible, but a variational EM algorithm to approximately maximize the posterior distributions can be applied. The corresponding software of the emIRT package in R with implementation of the Poisson IRT (or “Wordfish”) model to analyze text data from presidential State of the Union (SOTU) addresses between 1985 and 2019 is described. In the Wordfish model, the data are organized as word frequencies in a matrix with the words on the rows and the documents on the columns. The word frequencies are assumed to be generated by a Poisson process that accounts for a general prevalence of a specific word in the latent ideological position of a document. The inferential framework and the scripts for using emIRT and two text-processing packages quanteda and tm in R are presented, with the results by six recent presidents’ speech ideological characteristics visualized and discussed.
The book is completed with a list of several hundred references and a detailed index. Many exercises are given at the end of chapters. The R package for the data and new functions used in the book can be installed by library(devtools) and install_github(“davidaarmstrong/asmcjr”),and ggplot2 package is applied for visualization of the results. Multiple R codes are given throughout the book, helping to practical implementation of the considered techniques. The book can be interesting to students and researchers not only in political sciences, but it can actually enrich other applied socio-psychological and economical studies by new approaches and innovative statistical methods. Some additional related topics are considered in the sources given in the references below.
Stan Lipovetsky
Minneapolis, MN
[INLINEFIGURE]
References
- Camparo J., and Camparo L.B. (2013), “The Analysis of Likert Scales Using State Multipoles: An Application of Quantum Methods to Behavioral Sciences Data,” Journal of Educational and Behavioral Statistics, 38, 81–101. DOI: https://doi.org/10.3102/1076998611431084.
- Lipovetsky S. (2017), “Factor Analysis by Limited Scales - Which Factors to Analyze,” Journal of Modern Applied Statistical Methods, 16, 233–245. DOI: https://doi.org/10.22237/jmasm/1493597520.
- Lipovetsky S. (2020), Modern Psychometrics with R, by P. Mair, Technometrics, 62, 135–137.
- Lipovetsky S. (2021), Understanding Elections through Statistics: Polling, Prediction, and Testing, by O.J. Forsberg, Technometrics, 63, 143–144.
- Lipovetsky S. (2021), Handbook of Item Response Theory, in 3 volumes, by W. J. van der Linden, Ed., Technometrics, 63, 428–437.
- Lipovetsky S., and Conklin M. (2018), “Decreasing Respondent Heterogeneity by Likert Scales Adjustment via Multipoles”, Stats, 1, 169–175. DOI: https://doi.org/10.3390/stats1010012.