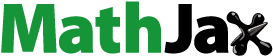
ABSTRACT
This study evaluates the quality of the digital platform economy at the global scale by employing a network model rooted in nonparametric linear techniques (data envelopment analysis) on a sample of 116 countries for 2019. The proposed model is in accordance with the geographic diversity (country heterogeneity) and the multilayered structure characterizing the interactions between system participants: governments, digital platforms, platform-dependent firms, and end users. The core findings indicate that the configuration of countries’ platform economy is very heterogeneous, which suggests that an informed, tailor-made approach to policy might produce more effective outcomes. Policies aimed at enhancing the digital platform economy should emerge from the analysis of its main factors if the development of a strategy seeking qualitative improvements in the system is the desired goal.
Introduction
Over the past decades scholars and policymakers have witnessed how digitalization – that is, the development and application of digital technologies that are widely exploited by platforms, tech entrepreneurs and users – has evolved from a disruptive wave affecting specific industries to become a fundamental driving force of economic growth around the globe (for example, Acs et al., Citation2022; Cutolo & Kenney, Citation2021; Goldfarb & Tucker, Citation2019; McKinsey, Citation2016; Yoo et al., Citation2010).
By embracing the properties of the digital technology, for example, data availability, enhanced computer power, improvements in network connectivity or reduced transaction costs (Brynjolfsson & McAfee, Citation2014) digital markets ranging from smartphone applications to different forms of digital products and services have consolidated in today’s business scenario and, consequently, digital platforms – for example, the “Big Five” tech giants: Alphabet-Google, Amazon, Apple, Facebook, and Microsoft – are nowadays among the most successful organizations at the global scale.
Digital platforms play a decisive role in creating value to stakeholders in the digital platform economy by redefining the way to do business and contributing to develop new products and services. As a result, digital platforms have accumulated significant power, and the resulting imbalances between platforms and other actors are causing concerns in policy circles interested in preserving stakeholders’ interests as platforms pursue their economic goals (for example, privacy, personal data protection, and cyber security; Cutolo & Kenney, Citation2021; Van Dijck et al., Citation2018).
As a reaction to the consolidation of the digital platform economy, policymakers are progressively devoting efforts both for improving digital infrastructures and for regulating the relationships between platforms and other system actors (that is, platform-dependent firms that sell their products and services through platforms, and users). For example, many governments are developing policies that facilitate the digitalization of society and economic activities (see, for example, European Commission, Citation2020 for a description of the EU’s Digital Europe program).
In addition, we have observed how countries have recently endorsed different regulations in order to govern their relationships with platforms. These policies have sometimes produced tensions between governments and digital platforms with relevant repercussions for other market agents that do not take part in the negotiations between platforms and governments, namely: firms whose business model relies on the commercialization of their products and services through platforms – that is, platform-dependent firms or complementors – and digital users. Examples of such tensions include, among others, the imposition of digital taxes in various EU countries with different reactions from digital platforms (The Economist, Citation2019a), the fierce debate between Google and Australia’s authorities as a result of the announcement of the country’s plan for regulating digital markets (The Guardian, Citation2021), and Meta’s announcement in early 2022 to weigh the possibility of withdrawing social media platforms – that is, Facebook and Instagram – from Europe over data privacy and transfer disputes with EU authorities (Business Insider, Citation2022).
From the observation of these events it becomes evident that, as a national phenomenon, the digital platform economy is characterized by multiple interactions between regulators, platforms, users, and platform-dependent firms (Cennamo, Citation2021; Goldfarb & Tucker, Citation2019; Palmié et al., Citation2020). Following this logic, digital policy – for example, regulation and investments in technology – as well as the interaction between governments and platforms set the “rules of the game” and the economic potential of digital markets (Acs et al., Citation2022; Goldfarb & Tucker, Citation2019). At the local level, the regulatory framework and technological possibilities of digital markets – which can be partially inferred from the interaction between governments and platforms – condition both users’ capacity to maneuver in digital markets and the economic and technological incentives to entrepreneurs for effectively exploiting the distinctive properties of digital markets via the creation of platform-dependent firms (Cutolo & Kenney, Citation2021). In this sense, despite its utter simplicity, our model is in line with prior work suggesting a multilayered structure of the platform economy in which governments and platforms shape the structure of the digital platform economy which, in turn, conditions the interactions between platform-dependent firms and users (Cennamo, Citation2021; Cutolo & Kenney, Citation2021; Goldfarb & Tucker, Citation2019; J. Li et al., Citation2019; Parker et al., Citation2017).
It should be noted that prior work has focused on either the governance of platforms’ activities (for example, Cennamo, Citation2021; Halaburda & Yehezkel, Citation2013; Rochet & Tirole, Citation2003; Wareham et al., Citation2014), or the analysis of the strategies adopted by system participants, namely digital platforms, platform-dependent firms, and users (for example, Athey & Gans, Citation2010; Brynjolfsson et al., Citation2018; De Cornière, Citation2016; Cutolo & Kenney, Citation2021; Palmié et al., Citation2020; Parker et al., Citation2017).
Despite the relevance of existing research, there are nonetheless various concerns related to the digital platform economy that remain unaddressed: what are the main characteristics and drivers of countries’ digital platform economy? Moreover, in light of the properties of digital markets and the central role of platforms as global intermediaries that ultimately interconnect and accelerate economic processes (Cennamo, Citation2021; Cutolo & Kenney, Citation2021), does the proposed analysis help to unveil strategic policies which can contribute to improve countries’ digital platform economy, conditional on the interplay between governments and digital platforms?
This is the core of this study. To address these questions empirically, we evaluate the quality of the digital platform economy at the global scale by applying a network (two-stage) efficiency model rooted in nonparametric linear techniques (Charnes et al., Citation1978; Grifell-Tatjé & Lovell, Citation2015). The empirical application uses a unique data set including information on the properties of the digital platform economy – measured by the digital platform ecosystem (DPE; Szerb et al., Citation2022) – for a sample of 116 countries for the year 2019.
At this point it is worth questioning why the proposed study of the digital platform economy is needed, and why our analysis constitutes a valid approach to scrutinize the digital platform economy at the global scale. The “platformization” of the economy has reshaped the structure of markets. In this new context, digital platforms have become the leaders of the digital platform economy with private regulatory power (Boudreau & Hagiu, Citation2009), while participation in this system has become decisive for warranting the market success of many businesses whose competitive advantage mostly relies on digital technologies.
For the specific purpose of this study, the proposed network model is suitable for our analysis for, at least, two interconnected reasons. First, as mentioned above, from a methodological point of view the network model used to evaluate countries’ digital platform economy – proxied by the DPE – melds together two relevant properties of this system: the two-layer structure governing the relationships between participating actors, and the country-level heterogeneity in the configuration of the platform economy, that is, in terms of the relative weight of system constituents (Cutolo & Kenney, Citation2021; Parker et al., Citation2017). The DPE index has attractive properties that validate its accuracy to assess the platform economy (Szerb et al., Citation2022). But, the DPE trends in a single-layer framework and profiling countries across the DPE values may offer limited material for analytical purposes. This problem exacerbates once we account for one of the key assumptions of the DPE: the use of fixed (across countries) and homogeneous (across variables) weights for system constituents. Thus, instead of canonical analyses based on the DPE results that ignore the multilayer structure of the platform economy, our approach is coherent with system’s characteristics by proposing that the structure of the platform economy is first determined by the interplay between governments and platforms which, in turn, directly affects the relationships between (new and incumbent) platform-dependent firms and users. By modeling countries’ digital platform economy as a multilayer structure, our analysis provides a rich framework to understand how the digital platform economy is nurtured by the different interactions that exist between system actors.
Second, from a policy viewpoint, governments are increasingly supporting digitization plans to improve digital infrastructures and the functioning of digital markers (for example, European Commission, Citation2020). In this sense, backing digital entrepreneurship is essential for consolidating emerging platform-dependent sectors which complement other industries (for example, app developers and the “fintech” sector), and facilitate the economic relationships between platforms, individuals, and other platform-dependent firms (Cumming & Schwienbacher, Citation2018; Nambisan & Baron, Citation2021). Furthermore, each country has its own socioeconomic reality and digital priorities (for example, digital inclusion and digital infrastructures), and policies embraced by one country may not be effective in other contexts. The properties of our model (that is, country-specificity and network structure) support this argument. For example, mimicking policies adopted by advanced countries in developing economies without proper adaptation to the local context would not help to improve the quality of the platform economy in these countries. Models like ours – which acknowledge the geographic diversity of digital markets and partially capture the complexity of the digital platform economy – can prove themselves effective in equipping policymakers with the means to better grasp the properties of the platform economy, while allowing countries to identify key aspects of the platform economy that should be prioritized based on their specific institutional and market conditions.
The objective of our network model is not to merely identify the overall efficiency level of countries’ digital platform economy. Rather, our model seeks to assess the quality of the digital platform economy by computing the best efficiency level that countries can achieve through collaboration among system participants in the proposed layered structure: governments and digital platforms on the one hand, and on the other hand, platform-dependent firms and users, conditional on the efficiency resulting from the relationship between governments and platforms.
In this sense, what is the positioning of our study within the literature? Building a universally accepted and generalizable metric to assess the digital platform economy is a hard, challenging task; however, the detailed nature of the data (DPE) and the proposed network analysis should provide enough feedback into the generation of economically meaningful results. Therefore, the relevance of this study surpasses computational exercises and advances what we consider a rich analysis with valuable implications. From the perspective of the digital platform economy, our study contributes to appreciation of the drivers of countries’ platform economy by identifying priorities that can inform policymakers how to orchestrate resources to promote quality-led improvements in the platform economy. Also, our analysis provides nuanced guidance on how to support both digital entrepreneurship and the connections between platform-dependent firms and users by developing tailor-made policies that, at the same time, help strengthen the local platform economy.
The plan of the paper follows. The actors and market properties of the digital platform economy are described, followed by the model used to evaluate the quality (efficiency) of countries’ digital platform economy. The data and sample are set out and the results are presented. Concluding remarks, implications, and future research lines complete the paper.
Characterizing countries’ digital platform economy
At country level, the digital platform economy has gained centrality in today’s economic landscape. With the dawn of the twenty-first century we have witnessed how different technology developments – for example, smart phones and mobile services, social media, big data, cloud computing, or the Internet of things (Acs et al., Citation2022; European Commission, Citation2017) – have modified the competitive environment by reshaping countries’ digital policy, as well as supporting new ways of allocating resources in the economy and developing new products that accommodate contemporary market demands (Acs et al., Citation2022; Nambisan & Baron, Citation2021; Sussan & Acs, Citation2017).
To better understand the functioning of countries’ digital platform economy, in this section we discuss three critical aspects of this system: the key actors of the digital platform economy, the distinctive market characteristics of the digital platform economy, and the policy issues that emerge from the interactions between system actors.
Actors of the digital platform economy
As a result of the rapid improvement in digital infrastructures, computing power and connectivity, the “digitization” of the economy – in terms of increased digital infrastructures and digital trade – is a growing trend that is transforming the functioning of economies (Brynjolfsson & McAfee, Citation2014; Parker et al., Citation2017). Building on the growing literature dealing with digital platform economy (for example, Acs et al., Citation2022; Goldfarb & Tucker, Citation2019; Kenney & Zysman, Citation2016; Song, Citation2019), in this study we conceptualize the digital platform economy as the collection of multiple digitally enabled activities that take place in the economy between different stakeholders, namely: two stakeholders primarily situated in the external environment (that is, governments and digital platforms), and two market agents (that is, platform-dependent firms and users).
Governments as facilitators of digital infrastructures
Governments, by themselves or in close collaboration with private organizations, create and/or develop digital infrastructures that support the functioning of the digital economy (Acs et al., Citation2022; Jullien, Citation2012). Digital infrastructures include specific digital technologies (for example, Internet backbone, fixed broadband, local servers, and mobile telecommunication networks) as well as the regulatory framework that governs the social and economic transactions that occur within the digital ecosystem (Hanseth & Lyytinen, Citation2016; Henfridsson & Bygstad, Citation2013). The world is today a more digitally integrated place. In this sense, digital technologies are essential to warrant digital inclusion by supporting the access to the Internet to the population and, subsequently, to help citizens and businesses to carry out social and economic activities, including, among others, access to information, e-commerce, remote education, remote work, remote health care, and digital banking services.
Digital regulation is decisive to support digital integration among the population by promoting specific policies that regulate digital transactions, improve data privacy, and enhance cyber protection. Besides the role of digital infrastructures as facilitators of an open and secure digital economy, we have recently observed how governments are promoting ways to curb the power of digital platforms, and how tensions between governments and platforms have materialized in the enactment of regulations guided by policymakers’ interest for controlling the key input of the digital platform economy, namely the data (Business Insider, Citation2022; The Economist, Citation2021).
Digital platforms
Digital platforms (for example, Alphabet-Google, Amazon, Apple, Facebook, and Microsoft) are a “layered architecture of digital technology” (Yoo et al., Citation2010, p. 725) that facilitate communications, interactions between system participants, as well as the development of innovations that support economic and social activities through the Internet or private networks (Cennamo, Citation2021; Gawer, Citation2014). The intense use of hardware and software to generate standards, interfaces, and rules that enable the provision of value-adding goods and services is a key characteristic of platforms’ business model (Teece, Citation2018). By providing structures that reduce information costs of products and services, platforms are digital hubs or “matchmakers” that trigger economic- and information-based exchanges between users and businesses (Brynjolfsson & Saunders, Citation2010; Goldfarb & Tucker, Citation2019). Digital technologies are at the core of the value proposition of platforms, and advances in such technologies reduced experimentation costs, thus promoting innovations (Rysman, Citation2009; Von Briel et al., Citation2018). By exploiting digital technologies, platforms orchestrate different layers – that is, device layer, network layer, service layer, and content layer (Parker et al., Citation2017) – to enable economic transactions between system actors, namely complementors who provide complementary products and services (for example, platform-dependent firms developing apps or software), and users who consume the products, services and content offered digitally by platforms and complementors (Cennamo, Citation2021; Goldfarb & Tucker, Citation2019).
By positioning digital platforms at the center of the digital platform economy as bridge builders intermediating between actors on both sides of the system (Goldfarb & Tucker, Citation2019), recent studies have identified three types of interactions occurring in this system (Nooren et al., Citation2018; Parker et al., Citation2017; Teece, Citation2018): between digital platforms (for example, Apple’s iOS vs Google’s Android); between platforms and other system actors (for example, the cases of Microsoft capturing part of the value from Internet browsers; and the collaboration between EU authorities, platforms and digital users for developing the Digital Services Act to regulate online relationshipsFootnote1); and between platform-dependent firms that operate through platforms (for example, app developers, business software developers, and other forms of high-tech organizations). Regardless of whether the analyzed platform is transactional (that is, exchange facilitator), innovative (that is, providing base technologies and systems to other businesses), or hybrid (that is, transactional and innovative; Gawer, Citation2014), recent work shows the increased economic and societal relevance of digital platforms (for example, Hagiu, Citation2009; Jullien, Citation2012; Parker et al., Citation2017; Saadatmand et al., Citation2019; Teece, Citation2018).
Platform-dependent firms
This group includes entrepreneurial and incumbent technology-intensive firms that populate the supply-side of the digital platform economy (for example, app developers and software developers). These new and incumbent firms exploit hardware and software to support experimentation, innovation, and value creation processes (Cutolo & Kenney, Citation2021).
Within the platform economy, platform-dependent firms simultaneously play two roles (Acs et al., Citation2022; Goldfarb & Tucker, Citation2019; Nambisan & Baron, Citation2021). First, in their pursuit of market profits, these businesses nurture platforms with a large variety of products or services that connect to other actors of the digital platform economy. Here, platforms act as global intermediaries connecting businesses to potential customers (users). Second, from the perspective of the digital platform these businesses are complementors that offer important benefits both to platforms – whose structures facilitate the commercialization of products and services – and to users who now have a digital marketplace to access firms’ offering. In this latter case, the economic outcome of platform-dependent firms is highly reliant on the dynamics generated by digital platforms – for example, traffic, power relationship between the platform and the business, and platform’s rules and constraints (Boudreau & Hagiu, Citation2009) – which, following our argument line, are also interwoven with the properties of the competitive playing field determined by the governance mechanisms set by regulators and digital platforms (Acs et al., Citation2022; song, Citation2019).
End users
In the context of the digital platform economy, end users encompass agents actively exploiting platforms’ services, that is, individual consumers and, in some cases, producers. Digital users connect to each other for economic and social activities through the Internet and mobile devices on various digital platforms. Examples of platforms with mostly differentiated producers and users are Facebook, Amazon, and Netflix: these platforms interact with digital businesses and other firms (advertisers, suppliers, and filmmakers, respectively) to generate their products and services. In some platforms agents can also act as users and producers. Airbnb represents a relevant case. Data available for 2021 indicate that Airbnb has more than 150 million users (demand-side) and more than four million hosts (supply-side) worldwide who can also be users of Airbnb services (https://ipropertymanagement.com/research/airbnb-statistics). Uber and Didi Chuxing are additional examples of platforms – in this case, in the private transport sector – where users can also provide services through the digital platform.
Platforms’ penetration rate is highly reliant on both the willingness of users to adopt digital platforms and on the platforms’ perceived utility. Therefore, digital trust (for example, data privacy and data protection policies) and digital expertise (for example, e-reviews and rating scores of movies or restaurants) are relevant aspects that explain users’ online participation (Acs et al., Citation2022).
Market properties of the digital platform economy
Concerning the dynamics of the digital platform economy, we pay special attention to what we consider the three most relevant properties of this system, namely platform multisidedness (related to product offering in the system), network effects (which we link to role of platforms as matchmakers connecting system actors), and the “winner-take-all” competition logic.
Platform multisidedness
The rise and consolidation of digital platforms is one of the more representative events of the last two decades. By May 2021, the combined market value of the “Big Five” club – that is, Alphabet-Google, Amazon, Apple, Facebook, and Microsoft – was over US$8 trillion (The Wall Street Journal, Citation2021). Figures are not surprising. The “platformization” of the economy has produced vast benefits to the economy in the form of new ways to do business, as well as new products, services and content. However, platforms’ activities also introduced new challenges that demand policy action. Contrary to standard markets, in which competition is defined at the product level, in the digital platform economy competition is between markets with unclear boundaries as it covers multiple products and industries (Rochet & Tirole, Citation2003).
Digital platforms capitalize on digitization capabilities (that is, availability of digital data resulting from advances in creating, storing and analyzing digital data) and digitalization processes (that is, application of digital technologies by organizations and users) to create a networked technology architecture that connects numerous external, independent businesses that deliver their products and services to end users (Brennen & Kreiss, Citation2016; Cennamo, Citation2021).
This principle governs the functioning of digital markets in many diverse industries, including, among others: sectors inherently tied to the Internet (for example, search engines such as Google search and media streaming such as YouTube), e-commerce (for eample, Amazon and eBay), social media (for example, Facebook, Twitter, and Instagram), communication (for example, WhatsApp and Zoom), mobility (for example, MyTaxi and Uber), hotels (for example, Airbnb), and delivery services (for example, Glovo and Deliveroo). Platforms are the lead agent of the digital platform economy by providing architectures to guide the evolution of the system and, at the same time, they interact with governments to organize and regulate the transactions between complementors and users (Rochet & Tirole, Citation2003; Teece, Citation2018).
This implies that digital platforms have the capacity to generate new technologies to re-shape the digital economy characterized by a strong interdependence between different industries (Yoo et al., Citation2010). In a context of systems dominated by platform leaders, the increased interconnectedness between platform-dependent firms (that is, complementors) facilitates the creation of an integrated product/service system for end users (Jacobides et al., Citation2018; Sussan & Acs, Citation2017).
Network effect
The dominance of large platforms observed in the digital economy can be attributed to the platforms’ capacity for exploiting indirect network effects (that is, matchmaking) by facilitating matching and improving the efficiency of multisided tradeoffs (De Cornière, Citation2016; Jullien, Citation2012). The benefits for users are clear: the access to a vast amount of filtered information (see, for example, Tucker and Zhang (Citation2011) for an analysis of popularity information effects in e-retail markets, and Agrawal et al. (Citation2015) for a study on how popularity information affects online investment patterns). Examples of matchmaking platforms include, among others: search engines (for example, Google), social media (for example, Facebook), dating sites (for example, eHarmony), and travel agencies (for example, TripAdvisor). Network effects are also evident when analyzing how platforms facilitate multisided economic transactions. In this case, platforms develop structures so that complementors (app developers) can centralize information to connect a large number of sellers and buyers more efficiently, that is, by minimizing search, reproduction and verification costs (Halaburda & Yehezkel, Citation2013). The access to a wide variety of products, services and content is the core benefit to users (Hagiu, Citation2009). Amazon (e-commerce sector) and Uber (mobility sector) are notable examples of platforms performing this intermediation role.
It should be noticed that the relationships between platforms, complementators and end users are mostly cooperative. On the one hand, platforms are increasingly becoming innovation engines that influence the complementors’ innovation efforts by facilitating the access to the platform’s architecture (for example, Apple iOS and Android that work with specific market infrastructures: AppStore and GooglePlay, respectively); and by offering more attractive deals (for example, favorable licensing agreements for new apps). On the other hand, part of the value of the digital economy relies on the capacity of platforms for generating, storing, and exploiting data so that platform-dependent firms and users can fully realize the benefits of the digital platform economy.
The “winner-take-all” competition logic
In strict connection to the previous point, it has been argued that network effects might result in a “winner-take-all” outcome in digital markets characterized by overlapping multisided platforms in terms of offering, strong economies of scope, and very low benefits from market specialization (Lee et al., Citation2006; Rochet & Tirole, Citation2003).
Underlying this competition logic is the presumption that size (for example, users’ network size and complementors’ network size) is the most important factor driving platform’s competitive advantage (Hagiu, Citation2009). Contrary to conventional markets where businesses use aggressive pricing strategies to generate market value by damaging competitors’ position, in the “winner-take-all” logic platforms that reach a critical mass of users are better able to produce a self-reinforcing loop between users’ network and complementors’ network and content which, in turn, increases the value of the platform. As a result, platforms with a large network size will likely dominate the entire system, which helps explain why the digital platform economy is dominated by tech giants operating as leaders of a digital market that tends to a monopolistic structure (Lee et al., Citation2006).
This theoretical approach has been empirically corroborated by verifying how large platforms with a solid competitive advantage – for example, Alphabet-Google, Amazon, Apple, Facebook, and Microsoft – have won market share and increased their value via the development of solid (and growing) networks of complementors (products, services, and content) and users, which produces that potential new competitors would operate under diseconomies of scale (Cennamo, Citation2021).
Policy issues in the digital platform economy: Interaction between governments and digital platforms in an evolving environment
The digital platform economy is mostly an unregulated environment, but different scandals involving digital platforms – for example, the case of the Equifax breach (The Economist, Citation2019b) and the Cambridge Analytica-Facebook scandal (The Guardian, Citation2018) – raise concerns about the need to regulate and more closely monitor platforms’ activities. These concerns primarily include policy issues (for example, digital freedom, data privacy, and cyber protection) as well as economic aspects related to the platforms’ market power (for example, market structure and operational transparency).
Different initiatives sponsored by governments – as in the case of the EU and UK (Nooren et al., Citation2018) – or by independent committees – as in the case of the USA (Stigler Committee on Digital Platforms, Citation2019) – have taken form aiming at discussing and proposing different approaches to govern the relationship between governments and digital platforms. Although platforms share economic and technical properties, each digital platform has its own specific characteristics, which creates substantial problems when it comes to design a uniform regulation (Nooren et al., Citation2018).
As the policy debate on how to regulate platforms continues to develop, the close interaction (and sometimes collaboration) with platforms as well as the cross-border regulatory enforcement are examples of strategies adopted by developed economies to safeguard public interests (for example, privacy and data control), while guaranteeing the functioning of the digital economy (Nooren et al., Citation2018). In parallel, the US and EU authorities have recently started to regulate certain activities of some specific platforms through, for example, taxation, disclosure of pricing terms, labor issues, the handling of personal data, and the relationship between the platform and its partners (that is, complementors; for example, Eaton et al., Citation2015; Rochet & Tirole, Citation2003; Van Dijck et al., Citation2018).
To sum up, digital platform economies are environments characterized by the lack of regulation and monopolistic competition. In this setting, digital platforms dominate their relationships with platform-dependent firms and end users. The “platformization” of the economy has undoubtedly produced large benefits to the market: platforms support innovation efforts of complementators, and provide increased offering of digital goods and services at minimum search, reproduction, and verification costs (for example, Goldfarb & Tucker, Citation2019; Sussan & Acs, Citation2017). These benefits are also evident at the territorial level, in terms of the higher adoption of ICTs in urban settings (agglomeration effects), the increased flow of digital and physical goods in rural or low-density areas, and the reduced need for a task-specific workplace which favors that tech entrepreneurs locate their businesses in rural areas (for example, Kolko, Citation2012; Lafuente et al., Citation2010).
Nevertheless, the “platformization” of the economy might produce negative effects on society’s welfare which raise important policy concerns. For example, the dominant position of digital platforms might manifest itself through lower quality of content (for example, Lynch & Ariely, Citation2000), fewer innovation incentives (for example, Brynjolfsson & Saunders, Citation2010), lower privacy and data protection (even if consumers pay in kind by transferring their data; Goldfarb & Tucker, Citation2011), and the monopolization of other markets, such as news media which can potentially affect news quality, objectivity, and plurality (for example, Calzada & Gil, Citation2020; Chiou & Tucker, Citation2017).
Because digital platforms are powerful players of the digital economy, governments are increasingly interested in interacting with platforms in order to safeguard public interests as platforms pursue their economic goals, as well as ensure an efficient functioning of the system in terms of the connections between platforms, platform-dependent firms, and end users.
Building on this logic and evidence, by proposing a framework to the digital platform economy in which a network structure and geographic heterogeneity play a key role, our approach offers valuable insights for the design of a more informed policy. Contrary to the single-level interaction approach proposed by Acs et al. (Citation2022) and Song (Citation2019), a closer inspection of both the dynamics of the digital economy (for example, Cutolo & Kenney, Citation2021; Goldfarb & Tucker, Citation2019; Rochet & Tirole, Citation2003) and the evolution of the relationships between governments and platforms (for example, Business Insider, Citation2022; Stephens et al., Citation2019; The Economist, Citation2019b; The Guardian, Citation2021) support our two-layer framework to evaluate the interactions between the actors of the digital platform economy.
To move toward the model estimations, the next section presents the proposed network model used to evaluate the quality of countries’ digital platform economy.
The model
In this section, the description of the proposed framework to evaluate the efficiency of countries’ digital ecosystem is presented, followed by the details of the estimation strategy used to compute the relevant parameters of our model.
The network process shaping countries’ digital platform economy
The primary characteristic of the digital platform economy that is common to all countries is the presence of a digital platform that can be seen as a “digital infrastructure” playing two roles in the market. First, digital platforms, in their role as matchmakers, act as data hubs that accumulate and generate information flows between platform-dependent firms and users. Second, in their intermediation role, platforms facilitate economic tradeoffs by providing a market structure that connects users and suppliers of goods and services (Cennamo, Citation2021; Rochet & Tirole, Citation2003).
Based on this concept and the economic situations presented, we argue that the digital platform economy follows a leader-follower logic in which the platform leader interacts with two different groups of actors. On the one hand, governments are increasingly reacting to platforms’ strategic moves by taking actions to regulate both platform’s operations and the Internet content available to users. Notable examples of these actions include, among others, the creation of a digital tax in various EU countries (The Economist, Citation2019a), and the online “Great Firewall” created by China’s government to block Internet contents and various platforms (for example, Facebook, Google, Dropbox, among others; Business Insider, Citation2019). These barriers undermine the efficiency of the platform economy by deterring investments and limiting the access to several products, services, and content. However, it has been argued that the development of strong institutions, digital infrastructures, and enhanced coordination mechanisms is necessary to build a solid and efficient digital platform economy that favors platforms’ operations, as well as accurately protects public goods linked to privacy and citizens’ personal data (for example, Cennamo, Citation2021; Stigler Committee on Digital Platforms, Citation2019). Thus, this approach will likely increase the system’s efficiency.
On the other hand, platforms’ strategic actions, jointly with the interaction between governments and platforms, definitely impact platform-dependent firms and users. The strategy adopted by Apple and Google to manage their relationships with app developers is a good example of how platform choices affect platform-dependent firms and users. Apple iOS is a relatively closed operating system, and complementators interested in monetizing their work by publishing iOS applications must go through Apple’s rigorous quality checks. Besides, Apple controls the only distribution channel (App Store). On the contrary, part of the success of Apple’s main competitor (Google) relies on the open source license of Google’s Android system and on the support (guidelines and reference designs) that Google offers to developers to reduce their costs, provided that the developer subscribes to Google Play (Parker et al., Citation2017). But, similar to Apple, Google controls the distribution channel. The innovation incentives to platform-dependent businesses condition the development of new applications and software which, in turn, affects the stock and the quality of the products, services and content offered to end users.
In summary, the interactions characterizing the digital platform economy sketched herein can be modeled as a network-based relationship between governments, platforms, (digital infrastructure governance and digital multisided platforms) and digital agents (users and platform-dependent firms). presents the network (two-stage) process framework proposed in this study to evaluate the efficiency of the digital platform ecosystem.
Stage 1 deals with the relationship between governments and digital platforms. Here, countries employ their (observed or unobserved) economic and political resources (input: x = 1, …,J) to produce digital infrastructures and regulation (output: z = 1, …,D) seeking to create the conditions for an effective digital institutional setting that can be capitalized by multisided platforms. By jointly evaluating the characteristics of digital institutions – in terms of digital access, freedom and protection – and relevant properties of digital platforms – for example, networking and matchmaking – we can compute the efficiency level of the relationship between institutions and platforms .
As we argued previously, the relationship between governments and platforms conditions the relationships between platform-dependent firms and users. Thus, the estimated output of Stage 1 is used as the input of the production process of Stage 2 in which governments and platforms shape the structure of the digital setting where platform-dependent firms and users develop their activities (output: y = 1, …,M). Similarly, from the analysis of the latter process we can identify the efficiency gains that can be achieved by improving the interactions between platform-dependent firms and end users, conditional on the relationship between governments and digital platforms.
Because the proposed framework imposes the assumption of network coordination to the model, the overall efficiency level of the digital platform economy can be computed, for each country, as the multiplication of the efficiency level of Stage 1 (digital institutions and platforms) and Stage 2 (platform-dependent firms and users): .
We show below how the proposed network model can be estimated via standard frontier models rooted in nonparametric techniques, that is, data envelopment analysis (DEA).
Estimation strategy: Network data envelopment analysis (DEA)
Building on our framework sketched in earlier (), in which governments and platforms shape the digital setting (that is, regulation, infrastructures, and market structures), the relationship between institutions, digital platforms, and economic agents can be understood as a two-stage process that is characteristic of a network data envelopment analysis (DEA) model (Kao & Hwang, Citation2008; Y. Li et al., Citation2012). In the specific context of this study and following the generic two-stage process presented in , we assume that a group of observations – in our case countries – uses a set of inputs
to produce a set of outputs
in the first stage of the process (Stage 1). The outputs of the first stage (z) are considered the inputs – intermediate measures – used to produce the outputs
in Stage 2. For each country the efficiency level for the first and second stage of the process are defined as
and
, respectively.
Building on nonparametric techniques, the standard radial DEA model (Charnes et al., Citation1978) is used to compute, for each country (i = 1, …,N), a distance function that evaluates the first stage of the proposed digital ecosystem framework (Grifell-Tatjé & Lovell, Citation2015; Ray, Citation2004):
subject to:
In Equationequation (1)(1)
(1) the drawn technology
exhibits constant returns to scale, is homogeneous of degree +1 and is convex in the outputs (z). The efficiency of the digital institutions and platforms (Stage 1) is
, and for efficient countries on the frontier
while for inefficient countries
(
is the inefficiency level). The terms
and
are the non-negative weights that point to the degree of importance of the variables included in the first stage (digital institutions and platforms). Weights in Equationequation (1)
(1)
(1) are the core of our study as they reveal a countries’ strategy, in terms of the prioritization of pillars of the platform economy ().
An additional assumption underlying the technology described in Equationequation (1)(1)
(1) is that the vector of outputs is observable whereas the input vector is not directly observable. In many occasions input data is not available and, in the specific context of nonparametric analyses, the literature proposes the use of a single constant vector of 1s as input as a solution to this problem (see, Karagiannis & Lovell, Citation2016; Lovell & Pastor, Citation1999). There are two main motivations to evaluate countries’ digital platform economy using a DEA model without explicit input data. First, some economic problems do not require input data and the performance analysis relative to best practices becomes the main objective. Examples of such problems include the analysis of countries’ Olympic performance (Soares de Mello et al., Citation2009), and the analysis of the Kyoto protocol target achievement (Lo, Citation2010). Second, in some applications the outputs are aggregate variables (for example, GDP per capita), and the data do not permit to distinguish the input levels necessary to produce the outputs. Examples include the analysis of macroeconomic figures (Cherchye et al., Citation2004) or composite indicators such as the OECD Better Life Index (Mizobuchi, Citation2014), and the study of metrics related to the public sector (for example, Araya-Solano, Citation2019; Lafuente et al., Citation2022; Lefebvre et al., Citation2018).
The analysis presented in this study falls into the latter category. Countries can deploy a wide array of economic and political resources to enhance their digital platform economy (Acs et al., Citation2022; Cutolo & Kenney, Citation2021; Song, Citation2019). Therefore, in models like ours – that is, where composite indicators are the output and the specific inputs linked to the output are hard to identify – the use of DEA models with a single constant input – that is, the technology includes a vector of 1s (x = 1) – may prove itself suitable for evaluating the performance of countries’ platform economy relative to the best practice frontier.
To ensure the networked coordination proposed in our model (), in the technology of the second stage (Stage 2) we treat
as the single input subject to the constraint that the efficiency level of the first stage remains at
. Thus, the DEA model that computes the efficiency of the second stage of our model
is the following:
subject to:
In Equationequation (2)(2)
(2) the efficiency of the digital setting (institutions and platforms) (Stage 1) is
and
, while for Stage 2 (platform-dependent firms and users)
and
. The overall efficiency of the digital ecosystem
is obtained as
.
Solution values for both Stage 1 and Stage 2
can be represented in a hyper-plane depicting the two technologies
of the digital platform economy index (DPE). This analysis based on the visual representation of the solution values – which is represented as a 2 × 2 matrix in – can offer valuable information about the factors driving the efficiency of the digital platform economy among the sampled countries.
Description of the output set and sample
The data used in this study to evaluate countries’ digital platform economy at global scale come from the digital platform economy (DPE) index databases made available by the Global Entrepreneurship and Development Institute (www.thegedi.org; Szerb et al., Citation2022).
The DPE index (Acs et al., Citation2022; Szerb et al., Citation2022) situates at the center of the digital platform economy variables related to four different economic agents: institutions, digital platforms, platform-dependent firms, and digital users. The DPE index works under the assumption that for technology to be successfully introduced the interactions taking place within the digital platform economy need to be developed simultaneously by participating actors. This way, the proposed analysis based on the DPE index data allows for a more realistic modeling of the countries’ platform economy and contributes to identify country-specific policy priorities that should be targeted in order to produce quality improvements in the platform economy. A detailed description of the DPE methodology and computation is offered by Szerb et al. (Citation2022) (see Appendix 1).Footnote2
Concerning the measurement issues, the DPE integrates 12 pillars grouped in four interconnected system constituents (): (1) “Institutions” leading digital investments as well as the development of the regulation governing the activities taking place within digital markets (DPE sub-index: digital technology infrastructure); (2) “Digital multisided platforms” that coordinate social and economic activities between users and platform-dependent firms (DPE sub-index: digital multisided platforms); (3) “Platform-dependent firms” that incorporate relevant properties of (new and incumbent) businesses that contribute to innovation processes and commercialize their products and services through platforms (DPE sub-index: digital technology entrepreneurship); and (4) “Digital users” which includes users on the demand-side and the supply-side of the platform economy (DPE sub-index: digital user citizenship).
The Institutions sub-indicator captures the mechanisms that facilitate the functioning of the platform economy. This variable includes aspects related to the technologies of the digital age jointly with the regulations that govern their effective implementation. Appropriate technological infrastructures are essential prerequisites for the efficient operation of digital markets that is also responsible for ensuring an open and secure digital platform economy (Nooren et al., Citation2018; Van Dijck et al., Citation2018). Digital access, digital freedom, and digital protection are the components included in this sub-indicator.
The Digital multisided platforms sub-indicator highlights the key role played by digital platforms as innovation bridges of the information technology revolution (for example, Gawer, Citation2014; Rochet & Tirole, Citation2003). Saadatmand et al. (Citation2019) describe “digital platforms as an emergent organizational form characterized by technology and social processes.” By governing the mutually dependent relationships between the economic agents that consolidate the platforms’ value proposition (for example, app developers and users), digital platforms operate in multiple markets that are essential to most consumers in our contemporary economy. In order to take into account the various properties of platforms’ economic reality, this sub-indicator includes a number of constructs, including: networking, matchmaking, and financial facilitation ().
Third, the sub-indicator dealing with Digital users emphasizes the value of users’ privacy protection for a healthy and active digital ecosystem. This building block of the DPE addresses the explicit legitimization and implicit social norms that enable users to participate in the digital society in order to increase public trust and the sustainability of the digital ecosystem (Sussan & Acs, Citation2017, p. 64). Variables related to digital literacy, digital openness and digital rights are critical aspects of this sub-indicator ().
The role of Platform-dependent firms in the platform economy is included in the fourth DPE sub-indicator (digital technology entrepreneurship). This sub-indicator brings forth innovation and, subsequently, increases platforms’ efficiency. For a sustainable digital platform economy, digital entrepreneurship is critical for supporting innovation processes (for example, app and software developers), while knowledge exchange contributes to narrow the gap between supply opportunity and demand needs within platforms that increase the system’s efficiency (Cutolo & Kenney, Citation2021; Parker et al., Citation2017). In this case, decisive elements of a solid industry of platform-dependent firms include: digital adoption, technology absorption, and technology transfer ().
For analytical purposes, the four DPE sub-indices are the outputs used in our network DEA model to scrutinize countries’ digital platform economy: digital institutions (y1: “digital institutions”), digital platforms (y2: “digital multisided platforms”), end users (y3: “digital users”), and platform-dependent firms (y4: “platform-dependent firms”).
The final sample used in this study includes information for 116 countries for the year 2019 (Szerb et al., Citation2022). Summary statistics for the output variables (DPE sub-indicators) are presented in . Notice that shows the outputs for the sampled countries grouped by continent, while the country data (DPE sub-indicators) is presented in Appendix 2, Table A3
Table 1. Output set used to assess the digital platform economy: Descriptive statistics for 2019.
It is worth noting that the representativeness of the sample is ensured insofar as it includes 42 European countries (Albania, Armenia, Austria, Azerbaijan, Belgium, Bosnia and Herzegovina, Bulgaria, Croatia, Cyprus, Czech Republic, Denmark, Estonia, Finland, France, Georgia, Germany, Greece, Hungary, Iceland, Ireland, Israel, Italy, Latvia, Lithuania, Luxembourg, North Macedonia, Malta, Moldova, Montenegro, Netherlands, Norway, Poland, Portugal, Romania, Serbia, Slovak Republic, Slovenia, Spain, Sweden, Switzerland, Ukraine, and UK), 31 Asian countries (Bahrain, Bangladesh, Cambodia, China, Hong Kong, Iran, Indonesia, Iran, Japan, Jordan, Kazakhstan, Republic of Korea, Kuwait, Kyrgyzstan, Lebanon, Malaysia, Mongolia, Nepal, Oman, Pakistan, Philippines, Qatar, Russia, Saudi Arabia, Singapore, Sri Lanka, Taiwan, Thailand, Turkey, United Arab Emirates, and Vietnam), 23 African countries (Algeria, Benin, Botswana, Burundi, Cameroon, Egypt, Ethiopia, Kenya, Madagascar, Malawi, Mali, Mauritius, Morocco, Namibia, Nigeria, Rwanda, Senegal, South Africa, Tanzania, Tunisia, Uganda, Zambia, and Zimbabwe), 18 American countries, including both North America and Latin America and the Caribbean islands (Argentina, Brazil, Canada, Chile, Colombia, Costa Rica, Dominican Republic, Ecuador, El Salvador, Guatemala, Honduras, Jamaica, Mexico, Panama, Paraguay, Peru, United States, and Uruguay), and two Oceania economies (Australia and New Zealand).
Results
This section presents the findings of the efficiency measures based on Equationequations (1)(1)
(1) and (Equation2
(2)
(2) ), as well as the analysis of the strategic trajectory of countries’ digital platform economy. Finally, we illustrate the case-specific implications of the proposed network model by showing how our estimations can usefully reveal policy priorities with the potential to enhance the local platform economy in three highly heterogeneous countries, in terms of both macroeconomic figures and the level and configuration of the digital platform economy.
Analysis of the digital platform economy: Baseline results
This section presents the baseline results of the efficiency estimations. presents summary statistics of the efficiency scores (Equationequations (1)(1)
(1) and (Equation2
(2)
(2) )), while shows the performance patterns of the digital platform economy for the analyzed countries. Keep in mind that our network model does not merely compute the efficiency level of countries’ platform economy. Instead, our approach identifies the greatest efficiency gain that countries can achieve through collaboration among system participants in the proposed layered structure ().
Table 2. Network DEA model: Results.
Overall, the findings in reveal that, on average, the efficiency of the countries’ digital platform economy is 16.79% , which indicates that if the participating actors work together (for example, collaboration) they can improve the overall efficiency of the digital platform economy by 83.21%. Also, notice that the digital platform economy presents a balanced configuration among the sampled countries, in terms of digital institutions and platforms
and platform-dependent firms and users
. The USA is the only country reporting an efficient digital platform economy in terms of the two dimensions included in our model
. Also, results indicate that the Netherlands has an efficient digital setting (Stage 1: institutions and platforms), and that the inefficiency of its system exclusively comes from the relationship between platform-dependent firms and users
.
By continent, Europe and the two Oceania economies (Australia and New Zealand) report the highest average level of efficiency, in terms of both models: digital institutions and digital platforms (Stage 1) and platform-dependent firms and users (Stage 2). Also, in these countries the result of the digital institutions and platforms (Stage 1) is significantly higher than the efficiency scores for Stage 2 (Wilcoxon signed-ranked test for both continents: Z-value = 4.31 and p-value < 1%). The group of American countries shows a balanced system . A similar configuration pattern is reported for Asia
; however, notice that among Asian economies the result of the model linking the digital setting to complementators and users (Stage 2) is slightly higher than that observed for the first stage (Stage 1).
Finally, African countries report the lowest efficiency scores, in terms of both digital institutions and platforms and digital agents (platform-dependent firms and users)
. At this point it should be noted that, although the specific analysis of the links between economic development and the efficiency level of the platform economy falls outside the scope of our study, the results for African economies can be attributed either to imperfect policies or to the “less developed” status of most African countries which implies a shortage of potentially critical resources to implement specific digital policies.Footnote3
plots the estimations of the platform economy and its components. Findings indicate that the group of top performing countries is formed by European economies (Denmark, Estonia, Finland, Germany, France, Iceland, Luxembourg, Netherlands, Norway, Sweden, Switzerland, and UK), two North American countries (USA and Canada), the two Oceania countries (Australia and New Zealand), and Japan.
In addition, we identify a group of countries with an average efficiency results. This group includes various Eastern European countries (for example, Croatia, Hungary, Slovenia, Slovak Republic, among others), Asian countries (China and Korea), and the three top-performing Latin American countries (Uruguay, Chile, and Costa Rica). Finally, from the figure it is possible to observe a group of countries with low efficiency results – in terms of both dimensions of our network model – which includes mostly developing nations from Africa, Asia, and several European countries outside the EU (for example, Albania, Bosnia, Moldova, and Serbia).
Identifying strategic trajectories driving countries’ digital platform economy
In line with our first research question, “what are the main characteristics and drivers of countries” digital platform economy?”, this section focuses on the analysis of the strategic pillars driving countries’ digital platform economy. To aid in the analysis of the strategic patterns that characterize countries’ platform economy, we employ the weights computed via Equationequation (2)(2)
(2)
in order to map countries’ strategic priorities, in terms of the four variables analyzed in our network model. This way, by identifying what factors of the digital platform economy are prioritized by countries, constitutes the empirical representation of . The figure also shows, for each observed strategic trajectory – which we link to the drivers of the platform economy (DPE sub-indicators) – the overall efficiency of countries’ platform economy. To complement the results reported in , presents summary statistics of the overall efficiency level for the four quadrants represented in .
Table 3. Overall efficiency for the different configurations of the digital platform economy.
The first group (Cluster 1) includes 34 countries with a digital platform economy characterized by a strong emphasis on variables linked to digital institutions and digital users. The average overall efficiency of this cluster is 19.71% , which suggests that policy efforts can help improve the platform economy of these countries by 80.29% (). Europe is the most representative continent in the group (18 countries), and from we identify three groups within this cluster: a group of countries with a high-performing system (Australia, Denmark, Estonia, Finland, France, Germany, Japan, Netherlands, and Norway), a group of Eastern European countries whose platform economy shows an average overall efficiency (Bosnia, Croatia, Czech Republic, Hungary, Latvia, Moldova, Romania, Slovak Republic, and Slovenia), and a low-efficiency group of eight African countries.
For the 18 countries included in Cluster 2, digital institutions and the factor linked to the platform-dependent firms are the most important drivers of their digital platform economy. Countries with a healthy platform economy in this group are important financial hubs in Europe (Iceland, Luxembourg, and Switzerland) and Asia (Hong Kong) which, based on a tentative intuition supported by recent work (see, for example, Claessens et al., Citation2018; Cumming & Schwienbacher, Citation2018), may help explain the greater importance given to platform-dependent firms among these countries, in terms of estimated weights (for example, growth of “fintech” services, and development of regulation to monitor e-banking activities and financial operations online). This cluster also includes a group of developing countries mostly from Africa (Algeria, Egypt, and South Africa) and Latin America (Colombia, Dominican Republic, and Peru).
The digital platform economy of the third cluster (Cluster 3) is mostly dominated by factors linked to digital platforms and digital users. Similar to the case of Cluster 1, countries in this cluster can be split into three groups: a small group of countries with a solid digital platform economy (Canada, New Zealand, and UK), a group of countries from Europe, Latin America and Asia with average efficiency results (Chile, Italy, Korea, Lithuania, Malaysia, Spain, Taiwan, and Uruguay); and, finally, a group of developing countries mostly from Africa and Asia with low efficiency results (Benin, Cameroon, Lebanon, Mongolia, Namibia, Nepal, and Tanzania).
Finally, the digital platform economy of the fourth group (Cluster 4) is driven by a solid digital platform setting (“digital platforms”) and a robust sector of platform-dependent firms. Not surprisingly, the USA – the only country with an efficient digital platform economy (see Appendix 3) – falls into this cluster. This result can be explained by the long tradition of the country in supporting innovation processes (for example, Boston and Silicon Valley; Saxenian, Citation1991; Stephens et al., Citation2019), as well as by the fact that the “Big Five” tech giants are headquartered in the USA (Alphabet-Google, Amazon, Apple, Facebook, and Microsoft).
Besides the USA, in this cluster Belgium, Ireland, Israel, Singapore, and Sweden are countries with a highly efficient digital platform economy (). Additionally, 22 out of the 41 countries in the cluster are from Asia, and they mostly show average efficiency results. Notable examples of Asian countries in this cluster are China, Qatar, Saudi Arabia, Thailand, and United Arab Emirates. Within this cluster, a group of developing countries mostly from Africa (for example, Ethiopia, Kenya, Nigeria, Rwanda, Uganda, and Zimbabwe) and Asia (for example, Bangladesh, Cambodia, Pakistan, and Sri Lanka) with low efficiency results was identified.
Country-specific analysis: Improving the digital platform economy
So far we have described the main drivers of countries’ digital platform economy and discussed the advantages of the proposed network model. With these results at hand, we now turn to the country-level analysis by showing how our estimations can be used by social planners for policy purposes. Specifically, in connection with our second research question, “does the proposed analysis help to unveil strategic policies which can contribute to improve countries” digital platform economy, conditional on the interplay between governments and digital platforms?”, this section briefly illustrates the changes in the digital platform economy resulting from a policy based on the prescription of the proposed model in three countries with remarkable differences in terms of geography, institutions, economic development, as well as in the level and configuration of the platform economy: Norway, Singapore, and Costa Rica.
Results are presented in . Notice that estimations in are based on a hypothetical situation in which countries proportionally allocate additional resources equivalent to 10 index points to the policy priority – that is, the platform economy output variable – identified by our network model (Equationequations (1)(1)
(1) and (Equation2
(2)
(2) )). In computational terms, keep in mind that for each analytical stage the efficiency scores are the weighted sum of the rescaled “pie shares”: Stage 1
; and Stage 2
The endogenous weights and “pie shares” needed to compute the efficiency scores are then used to determine the policy priority of each analyzed country.
Table 4. Estimated improvements in the digital platform economy for the selected countries.
In addition, to further validate the results of this analysis we contrast the estimations based on the policy prescribed by our model against alternative approaches based on improvements in the other output variables included in the analysis. In the context of this example, the proposed comparisons represent a valid case to show the potential effects over the digital platform economy of a more directed policy (that is, strategic emphasis based on the prescription of our model) vis-à-vis alternative strategies focused on improving other constituents of the platform economy.
Norway, the case with a developed platform economy, has a relatively balanced digital platform economy in terms of the configuration of its constituents, which ranges between 63.68 (“platform-dependent firms”) and 84.40 (“institutions”) (see Appendix 2). From the inspection of the computed weights and “pie shares” it was found that “digital users” (y3) is the key variable driving Norway’s platform economy: Stage 1: ; and Stage 2:
(). By re-computing Equationequations (1)
(1)
(1) and (Equation2
(2)
(2) ) under the assumption that Norway allocates additional resources equivalent to 10-index points to the “digital users” variable, we find that a directed policy targeting aspects related to this variable would translate into a qualitative improvement of 24.05% in the efficiency level of the digital platform economy (Panel D in ).
From a policy viewpoint, examples of actions that Norwegian policymakers can take in this direction – which are summarized in Appendix 1 and described in great detail by Szerb et al. (Citation2022) – include the development of “digital literary” programs that improve digital skills (for example, computer skills, digital reading) among the population, or the promotion of actions linked to users’ “digital protection” to simultaneously educate users on how to do safe searches online and reduce the net infection ratio among users. Following the logic of our analytical approach, these actions based on a focused digital strategy might also enhance the relationships between users and (new and incumbent) platform-dependent firms. By comparing the outcomes of the focused digital policy to those obtained from alternative digital strategies, we find that the strategy based on investments in the “digital users” variable outperforms – in terms of the potential impact on the digital platform economy – the results of policies supporting other pillars of the platform economy ().
For Singapore, the country with a mid-level digital platform economy result, the findings for the “pie shares” indicate that “digital multisided platforms” (y2) is the main variable shaping the country’s digital platform economy: Stage 1: ; and Stage 2:
().
By replicating the resource allocation exercise used in this section, investments in aspects that have an impact on platforms’ operations – equivalent to 10-index points – might potentially enhance the digital platform economy index by 38.74% (Panel C in ). Similar to the Norwegian case, strategic emphasis pays off and a policy targeting “digital multisided platforms” would produce a greater improvement in the digital platform economy, compared to the results obtained from alternative policies affecting other constituents of the platform economy (). In this case, policies supporting human capital formation might help increase the number of developers in the country, which represents a key indicator of the “matchmaking” between labor markets and the human capital requirements of both platforms and entrepreneurial platform-dependent firms.
“Financial facilitation” is another decisive factor for a healthy connection between platforms, users and platform-dependent firms (Appendix 1). Thus, reforms that stimulate venture capital markets as well as the involvement of business angels in entrepreneurial digital projects are examples of policies that can enhance the ”financial facilitation” indicator of the platform economy. These policies have the potential to support new digital-led (or technology-intensive) firms that add value to both platforms and other businesses, being smart-phone apps, software development, and the ”fintech” industry good cases of growing activities primarily driven by digital entrepreneurship (for example, Cumming & Schwienbacher, Citation2018; Gazel & Schwienbacher, Citation2021).
In the case of the third country example, Costa Rica presents a relatively weak digital platform economy index and the scrutiny of the ”pie shares” estimated via Equationequations (1)(1)
(1) and (Equation2
(2)
(2) ) shows that ”institutions” (y1) is the main factor driving the country’s digital platform economy (): Stage 1:
; and Stage 2:
. Again, if additional resources – that is, investments equivalent to 10-index points – are deployed to the ”institutions” indicator, the quality of the platform economy would improve by 45.93% (Panel C in ).
In terms of policy, improvements in the ”institutions” variable can be achieved by promoting both investments in digital infrastructures that improve citizens’ connectivity (Appendix 1: “digital access”: fixed broadband and internet bandwidth) and reforms that increase competition in digital markets (Appendix 1: “digital freedom”).
Once more, as reported for Norway and Singapore, a digital policy logic based on the prescription of our model (that is, investments in the “institutions” factor) produces the greatest efficiency improvement, relative to the results reported for alternative digital strategies ().
Overall, the results presented in this section show that countries should prioritize different aspects of the platform economy to support quality improvements in the platform economy.
In sum, the analysis presented in this section leads us to conclude that the local configuration of the digital platform economy should be taken into account in order to produce a more meaningful digital policy. Policy design based on more informed models, such as the proposed network model, that account for territorial heterogeneity as well as the multilayered configuration of the digital platform economy constitutes an ideal scenario for policymakers. The proposed analytical tool for identifying optimal platform economy policy has the potential to offer valuable guidance to policymakers on how to direct the allocation of additional resources in order to optimize the quality of their local platform economy.
Concluding remarks, implications and future research lines
Concluding remarks
In this paper we employ a network (two-stage) model rooted in nonparametric techniques (data envelopment analysis) to analyze the digital platform economy at the world scale. By studying the performance of the digital platform economy – measured via the variables included in the DPE index (Acs et al., Citation2022; Szerb et al., Citation2022) – in 116 economies for 2019, our effort sought to shed light on the value to policymakers both of evaluating the quality of the digital platform economy using objective, data-driven methods that fit the properties of the platform economy (that is, multilayered structure and geographic heterogeneity), and of identifying key system factors that constitute strategic priorities driving countries’ digital platform economy.
Overall, the results of our model indicate that countries have a lot of room for improving their digital platform economy by implementing specific policies that enhance digital institutions (that is, infrastructures and regulation), as well as by promoting collaborations with the players leading the system, that is, digital platforms and platform-dependent firms. In addition, we found that the configuration of countries’ digital platform economy is very heterogeneous, which suggests that “blind” investments by governments are not enough to improve system. Rather, digital policy should emerge from the analysis of the factors driving the digital platform economy if the development of a more purposeful strategy seeking quality improvements in the system is the desired goal.
Implications
What policy implications can be drawn from our analysis of countries’ digital platform economy? In an increasingly competitive digital environment, different demands surface countries’ platform economy and policymakers struggle for selecting accurate measures to evaluate this system. The policy implications discussed in this section emerge from the results of the study, and are strictly connected to our research questions.
Valuable information based on accurate (data-driven) methods to analyze countries’ digital platform economy
Research on the digital platform economy has primarily focused on the governance of platform ecosystems (for example, Cennamo, Citation2021; Halaburda & Yehezkel, Citation2013; Rochet & Tirole, Citation2003), or the analysis of the strategic paths adopted by platforms, platform-dependent firms and users (for example, Brynjolfsson et al., Citation2018; De Cornière, Citation2016; Palmié et al., Citation2020; Parker et al., Citation2017). These studies have unquestionably contributed to increase our knowledge on the behavior of platforms and system participants. Nevertheless, the design of objectives measures that match system properties to evaluate countries’ digital platform economy is an important gap that we address in this study. At country level, understanding the properties of the digital platform economy is a complex, challenging task mostly because this system is characterized by multiple interactions between regulators, platforms, users and platform-dependent firms (Cennamo, Citation2021; Cutolo & Kenney, Citation2021; Goldfarb & Tucker, Citation2019). The dominant position of platforms and the increased interest of governments for regulating platforms’ activity add complexity to the analysis (Nooren et al., Citation2018; Van Dijck et al., Citation2018).
In connection to our first research question, “what are the main characteristics and drivers of countries” digital platform economy?”, the two-stage model used in this study to evaluate countries’ digital platform economy offers valuable information and insights that cannot be fully captured by simply using ratios or standard additive measures. In addition, our model is in line with existing studies that emphasize the layered structure of the digital platform economy in which the relationship between governments and digital platforms shape the configuration of the digital setting which, in turn, affects the interaction between platform-dependent firms and users (Cutolo & Kenney, Citation2021; Goldfarb & Tucker, Citation2019; Parker et al., Citation2017). The results of this research may usefully be made more central to inform policymakers on what specific policy actions are potentially conducive to a more efficient digital platform economy.
Policy isomorphism is not an optimal approach to enhance the digital platform economy
In a closely related manner, an additional implication of our analysis is that effective digital policy should not be based on ill-informed policy isomorphism, that is, the mere replication of action plans or strategies adopted by other, often more developed, countries. Policymakers need detailed and accurate information about what elements of the digital platform economy are more relevant in the local context when it comes to design economically meaningful actions.
Our findings highlight the geographic heterogeneity of the configuration of the digital platform economy. Countries prioritize different aspects of their digital platform economy and, consequently, the relationships between platform-dependent firms and users are affected by the interaction between governments and digital platforms. In line with our core results, we argue that a newly re-defined digital policy should emphasize selective improvements in the system.
The digital platform economy is highly influenced by its context, and multiple actors interact in the fabrication of a healthy platform economy (Acs et al., Citation2022; Goldfarb & Tucker, Citation2019). Besides, digital platforms are decisive players in this system as they are global intermediaries that ultimately interact with and interconnect different economic actors (that is, governments, platform-dependent firms, and users; for example, Boudreau & Hagiu, Citation2009; Cennamo, Citation2021; Gawer, Citation2014). Thus, the approach to digital policy should not be a “yes” or “no” to actions aligned with one or more actors of the digital platform economy, but rather to seek a new form of governance of the digital platform economy more compatible with the characteristics of the local economy. The support to digital entrepreneurship is a good example of how governments can channel innovations and economic activity to digital markets (for example, Cumming & Schwienbacher, Citation2018; Nambisan & Baron, Citation2021; Acs et al., Citation2022). This tailor-made approach to policy should promote actions targeting those elements of the digital platform economy that would produce even more positive outcomes. This aspect is especially relevant when we observe how governments are increasingly promoting regulations to govern digital markets and platforms’ activities, while ensuring the protection of users’ privacy and data (Van Dijck et al., Citation2018). This argument is in strict connection to our second research question, “does the proposed analysis help to unveil strategic policies which can contribute to improve countries” digital platform economy, conditional on the interplay between governments and digital platforms?”.
Future research
As with any study, the findings presented in this study are open to future verification. In this sense, it would be valuable to extend the proposed analysis in various directions. First, the data do not permit the direct analysis of the decision-making process preceding the generation and implementation of policies linked to the digital platform economy. Further work can address this issue by exploring the economic- and policy-led antecedents of support actions aimed at enhancing the digital platform economy. A second open issue concerns the impact of the digital platform economy on national outcomes. Future studies can address this by analyzing countries’ economic response to improvements in the digital platform economy. In addition, future studies can evaluate the connections between policies oriented to enhance the digital platform economy, the local entrepreneurial ecosystem and entrepreneurship-related outcomes.
Third, our model partially captures the complexity of the relations taking place with the digital platform economy. Extensions in this direction constitute a promising research avenue. Future work should address this point by proposing and empirically testing alternative models that more accurately reflect different aspects that underlie the multiple connections between system actors – that is, governments, platforms, platform-dependent firms and users – which are not fully represented in our two-stage model.
Finally, future research should assess the economic response to different system-enhancing policies in different geographic contexts. The analysis of the effectiveness of policy instruments in different settings would help to understand the extent to which the connections between governments, digital platforms, platform-dependent firms, and users condition the configuration and evolution of the digital platform economy across territories and across time.
Acknowledgments
Esteban Lafuente acknowledges financial support from the Spanish Ministry of Science and Innovation (Ministerio de Ciencia e Innovación, Agencia Estatal de Investigación) (grant: PID2020-115018RB-C32). László Szerb received financial support from the National Research, Development and Innovation Fund of Hungary (Project no. TKP2021-NKTA-19 and Project No. NKFIH OTKA K 131935).
Disclosure statement
No potential conflict of interest was reported by the authors.
Additional information
Funding
Notes
1 For more details on the Digital Services Act go to: https://digital-strategy.ec.europa.eu/en/policies/online-platforms
2 Szerb et al. (Citation2022) offer a comprehensive description of both all variables included in the DPE (see, in Szerb et al. Citation2022, pp. 51–59) and the computation of the DPE (see, Szerb et al., Citation2022, pp. 61–64).
3 We thank one of the anonymous reviewers for highlighting this relevant point.
References
- Acs, Z. J., Autio, E., & Szerb, L. (2014). National systems of entrepreneurship: Measurement issues and policy implications. Research Policy, 43(3), 476–494. https://doi.org/10.1016/j.respol.2013.08.016
- Acs, Z. J., Lafuente, E., & Szerb, L. (2022). A note on the configuration of the digital ecosystem in Latin America. TEC Empresarial, 16(1), 1–19. https://doi.org/10.18845/te.v16i1.5926
- Agrawal, A., Catalini, C., & Goldfarb, A. (2015). Crowdfunding: Geography, social networks, and the timing of investment decisions. Journal of Economics and Management Strategy, 24(2), 253–274. https://doi.org/10.1111/jems.12093
- Araya-Solano, M. (2019). Efficiency assessment of Costa Rica’s counties: A non-parametric analysis of the county competitiveness index. TEC Empresarial, 13(3), 78–92. http://dx.doi.org/10.18845/te.v13i3.4599
- Athey, S., & Gans, J. (2010). The impact of targeting technology on advertising markets and media competition. American Economic Review, 100(2), 608–613. https://doi.org/10.1257/aer.100.2.608
- Boudreau, K. J., & Hagiu, A. (2009). Platform rules: Multi-sided platforms as regulators. In A. Gawer (Ed.), Platforms, markets and innovation (pp. 163–191). Edward Elgar.
- Brennen, S. J., & Kreiss, D. (2016). Digitalization. In K. B. Jensen, R. T. Craig, J. D. Pooley, & E. W. Rothenbuhler (Eds.), The international encyclopedia of communication theory and philosophy (pp. 1–11). John Wiley & Sons.
- Brynjolfsson, E., & Saunders, A. (2010). Wired for innovation: How information technology is reshaping the economy. MIT Press.
- Brynjolfsson, E., & McAfee, A. (2014). The second machine age: Work, progress, and prosperity in a time of brilliant technologies. W.W. Norton & Company.
- Brynjolfsson, E., Eggers, F., & Gannamaneni, A. (2018). Measuring welfare with massive online choice experiments: A brief introduction. American Economic Association Papers and Proceedings, 108(May), 473–476. https://doi.org/10.1257/pandp.20181035
- Business Insider (2019). Here are all the major US tech companies blocked behind China’s ‘Great Firewall. Retrieved October 10th, 2019, from https://www.businessinsider.com/major-us-tech-companies-blocked-from-operating-in-china-2019-5
- Business Insider (2022). Meta warns it could pull Instagram and Facebook in Europe if it loses a data-sharing ruling. Retrieved February 8th, 2022, from https://www.businessinsider.com/meta-could-pull-instagram-facebook-europe-data-sharing-ruling-2022-2
- Calzada, J., & Gil, R. (2020). What do news aggregators do? Evidence from google news in Spain and Germany. Marketing Science, 39(1), 134–167. https://doi.org/10.1287/mksc.2019.1150
- Cennamo, C. (2021). Competing in digital markets: A platform-based perspective. Academy of Management Perspectives, 35(2), 265–291. https://doi.org/10.5465/amp.2016.0048
- Charnes, A., Cooper, W. W., & Rhodes, E. (1978). Measuring the efficiency of decision making units. European Journal of Operational Research, 2(6), 429–444. https://doi.org/10.1016/0377-2217(78)90138-8
- Cherchye, L., Moesen, W., & Van Puyenbroeck, T. (2004). Legitimately diverse, yet comparable: On synthesizing social inclusion performance in the EU. Journal of Common Market Studies, 42(5), 919–955. https://doi.org/10.1111/j.0021-9886.2004.00535.x
- Chiou, L., & Tucker, C. (2017). Content aggregation by platforms: The case of the news media. Journal of Economics and Management Strategy, 26(4), 782–805. https://doi.org/10.1111/jems.12207
- Claessens, S., Frost, J., Turner, G., & Zhu, F. (2018, September). Fintech credit markets around the world: Size, drivers and policy issues. BIS Quarterly Review. https://ssrn.com/abstract=3288096
- Cumming, D. J., & Schwienbacher, A. (2018). Fintech venture capital. Corporate Governance: An International Review, 26(5), 374–389. https://doi.org/10.1111/corg.12256
- Cutolo, D., & Kenney, M. (2021). Platform-dependent entrepreneurs: Power asymmetries, risks, and strategies in the platform economy. Academy of Management Perspectives, 34(4), 584–605. https://doi.org/10.5465/amp.2019.0103
- de Cornière, A. (2016). Search advertising. American Economic Journal: Microeconomics, 8(3), 156–188. https://doi.org/10.1257/mic.20130138
- Eaton, B., Elaluf-Calderwood, S., Sørensen, C., & Yoo, Y. (2015). Distributed tuning of boundary resources: The case of apple’s iOS service system. MIS Quarterly, 39(1), 217–244. https://doi.org/10.25300/MISQ/2015/39.1.10
- The Economist (2019a). France’s digital tax riles the white house. Retrieved July 11th, 2019a, from https://www.economist.com/finance-and-economics/2019/07/11/frances-digital-tax-riles-the-white-house
- The Economist (2019b). The big data breach suffered by equifax has alarming implications. Retrieved September 16th, 2019b, from https://www.economist.com/finance-and-economics/2017/09/16/the-big-data-breach-suffered-by-equifax-has-alarming-implications
- The Economist (2021). China has become a laboratory for the regulation of digital technology. Retrieved September 11th, 2021, from https://www.economist.com/china/2021/09/11/china-has-become-a-laboratory-for-the-regulation-of-digital-technology
- European Commission (2017). Digital transformation scoreboard 2017. https://ec.europa.eu/growth/tools-databases/dem/monitor/scoreboard
- European Commission (2020). Commission welcomes political agreement on €7.5 billion digital Europe programme. Press release, Brussels, Retrieved December 14, 2020, from https://www.europarl.europa.eu/news/en/press-room/20201211IPR93656/digital-europe-programme-meps-strike-deal-with-council
- Gawer, A. (2014). Bridging differing perspectives on technological platforms: Toward an integrative framework. Research Policy, 43(7), 1239–1249. https://doi.org/10.1016/j.respol.2014.03.006
- Gazel, M., & Schwienbacher, A. (2021). Entrepreneurial fintech clusters. Small Business Economics, 57(2), 883–903. https://doi.org/10.1007/s11187-020-00331-1
- Goldfarb, A., & Tucker, C. (2011). Privacy regulation and online advertising. Management Science, 57(1), 57–71. https://doi.org/10.1287/mnsc.1100.1246
- Goldfarb, A., & Tucker, C. (2019). Digital economics. Journal of Economic Literature, 57(1), 3–43. https://doi.org/10.1257/jel.20171452
- Grifell-Tatjé, E., & Lovell, C. A. K. (2015). Productivity accounting: The economics of business performance. Cambridge University Press.
- The Guardian (2018). Revealed: 50 million Facebook profiles harvested for Cambridge Analytica in major data breach. Retrieved March 17th, 2018, from https://www.theguardian.com/news/2018/mar/17/cambridge-analytica-facebook-influence-us-election
- The Guardian (2021). Google threatens to shut down search in Australia if digital news code goes ahead. by Josh Taylor. Retrieved January 22th, 2021, from https://www.theguardian.com/media/2021/jan/22/google-threatens-to-shut-down-search-in-australia-if-digital-news-code-goes-ahead
- Hagiu, A. (2009). Two-sided platforms: Product variety and pricing structures. Journal of Economics & Management Strategy, 18(4), 1011–1043. https://doi.org/10.1111/j.1530-9134.2009.00236.x
- Halaburda, H., & Yehezkel, Y. (2013). Platform competition under asymmetric information. American Economic Journal: Microeconomics, 5(3), 22–68. https://doi.org/10.1257/mic.5.3.22
- Hanseth, O., & Lyytinen, K. (2016). Design theory for dynamic complexity in information infrastructures: The case of building internet. In L. P. Willcocks, C. Sauer, & M. C. Lacity (Eds.), Enacting research methods in information systems (pp. 104–142). Palgrave Macmillan.
- Henfridsson, O., & Bygstad, B. (2013). The generative mechanisms of digital infrastructure evolution. MIS Quarterly, 13(4), 907–931. https://doi.org/10.25300/MISQ/2013/37.3.11
- Jacobides, M. G., Cennamo, C., & Gawer, A. (2018). Towards a theory of ecosystems. Strategic Management Journal, 39(8), 2255–2276. https://doi.org/10.1002/smj.2904
- Jullien, B. (2012). Two-Sided B to B Platforms. In M. Peitz & J. Waldfogel (Eds.), The Oxford Handbook of the Digital Economy (pp. 161–185). Oxford University Press.
- Kao, C., & Hwang, S. N. (2008). Efficiency decomposition in two-stage data envelopment analysis: An application to non-life insurance companies in Taiwan. European Journal of Operational Research, 185(1), 418–429. https://doi.org/10.1016/j.ejor.2006.11.041
- Karagiannis, G., & Lovell, C. A. K. (2016). Productivity measurement in radial DEA models with a single constant input. European Journal of Operational Research, 251(1), 323–328. https://doi.org/10.1016/j.ejor.2015.12.013
- Kenney, M., & Zysman, J. (2016). The rise of the platform economy. Issues in Science and Technology, 32(3), 61.
- Kolko, J. (2012). Broadband and local growth. Journal of Urban Economics, 71(1), 100–113. https://doi.org/10.1016/j.jue.2011.07.004
- Lafuente, E., Vaillant, Y., & Serarols, C. (2010). Location decisions of knowledge-based entrepreneurs: Why some Catalan KISAs choose to be rural? Technovation, 30(11–12), 590–600. https://doi.org/10.1016/j.technovation.2010.07.004
- Lafuente, E., Araya, M., & Leiva, J. C. (2022). Assessment of local competitiveness: A composite indicator analysis of Costa Rican counties using the ‘benefit of the doubt’ model. Socio-Economic Planning Sciences, 81(June), 100864. https://doi.org/10.1016/j.seps.2020.100864
- Lee, E., Lee, J., & Lee, J. (2006). Reconsideration of the winner-take-all hypothesis: Complex networks and local bias. Management Science, 52(12), 1838–1848. https://doi.org/10.1287/mnsc.1060.0571
- Lefebvre, M., Perelman, S., & Pestieau, P. (2018). Productivity and welfare performance in the public sector. In E. Grifell-Tatjé, C. A. K. Lovell, & R. Sickles (Eds.), The oxford handbook of productivity analysis (pp. 557–592). Oxford University Press.
- Li, Y., Chen, Y., Liang, L., & Xie, J. H. (2012). DEA models for extended two-stage network structures. Omega, 40(5), 611–618. https://doi.org/10.1016/j.omega.2011.11.007
- Li, J., Chen, L., Yi, J., Mao, J., & Liao, J. (2019). Ecosystem-specific advantages in international digital commerce. Journal of International Business Studies, 50(9), 1448–1463. https://doi.org/10.1057/s41267-019-00263-3
- Lo, S.-F. (2010). The differing capabilities to respond to the challenge of climate change across annex parties under the Kyoto protocol. Environmental Science & Policy, 13(1), 42–54. https://doi.org/10.1016/j.envsci.2009.09.005
- Lovell, C. A. K., & Pastor, J. T. (1999). Radial DEA models without inputs or without outputs. European Journal of Operational Research, 188(1), 46–51. https://doi.org/10.1016/S0377-2217(98)00338-5
- Lynch, J. G., & Ariely, D. (2000). Wine online: Search costs affect competition on price, quality, and distribution. Marketing Science, 19(1), 83–103. https://doi.org/10.1287/mksc.19.1.83.15183
- McKinsey. (2016). Digital globalization: The new era of global flows.
- Mizobuchi, H. (2014). Measuring world better life frontier: A composite indicator for OECD better life index. Social Indicators Research, 118(3), 987–1007. https://doi.org/10.1007/s11205-013-0457-x
- Nambisan, S., & Baron, R. A. (2021). On the costs of digital entrepreneurship: Role conflict, stress, and venture performance in digital platform-based ecosystems. Journal of Business Research, 125(March), 520–532. https://doi.org/10.1016/j.jbusres.2019.06.037
- Nooren, P., van Gorp, N., van Eijk, N., & Fathaigh, R. Ó. (2018). Should we regulate digital platforms? A new framework for evaluating policy options. Policy & Internet, 10(3), 264–301. https://doi.org/10.1002/poi3.177
- Palmié, M., Wincent, J., Parida, V., & Caglar, U. (2020). The evolution of the financial technology ecosystem: An introduction and agenda for future research on disruptive innovations in ecosystems. Technological Forecasting and Social Change, 151(February), 119779. https://doi.org/10.1016/j.techfore.2019.119779
- Parker, G., Alstyne, M. V., & Jiang, X. (2017). Platform ecosystems: How developers invert the firm. MIS Quarterly, 41(1), 255–266. https://doi.org/10.25300/MISQ/2017/41.1.13
- Ray, S. C. (2004). Data envelopment analysis: Theory and techniques for economics and operations research. Cambridge University Press.
- Rochet, J. C., & Tirole, J. (2003). Platform competition in two-sided markets. Journal of the European Economic Association, 1(4), 990–1029. https://doi.org/10.1162/154247603322493212
- Rysman, M. (2009). The economics of two-sided markets. Journal of Economic Perspectives, 23(3), 125–143. https://doi.org/10.1257/jep.23.3.125
- Saadatmand, F., Lindgren, R., & Schultze, U. (2019). Configurations of platform organizations: Implications for complementor engagement. Research Policy, 48(8), 103770. https://doi.org/10.1016/j.respol.2019.03.015
- Saxenian, A. (1991). The origins and dynamics of production networks in Silicon Valley. Research Policy, 20(5), 423–437. https://doi.org/10.1016/0048-7333(91)90067-Z
- Soares de Mello, J. C. C. B., Angulo-Meza, L., & Branco da Silva, B. P. (2009). A ranking for the Olympic games with unitary input DEA models. IMA Journal of Management Mathematics, 20(2), 201–211. https://doi.org/10.1093/imaman/dpn025
- Song, A. K. (2019). The digital entrepreneurial ecosystem—A critique and reconfiguration. Small Business Economics, 53(3), 569–590. https://doi.org/10.1007/s11187-019-00232-y
- Stephens, B., Butler, J. S., Garg, R., & Gibson, D. V. (2019). Austin, Boston, Silicon Valley, and New York: Case studies in the location choices of entrepreneurs in maintaining the Technopolis. Technological Forecasting and Social Change, 146(September), 267–280. https://doi.org/10.1016/j.techfore.2019.05.030
- Stigler Committee on Digital Platforms (2019). Final report. https://www.chicagobooth.edu/research/stigler/news-and-media/committee-on-digital-platforms-final-report
- Sussan, F., & Acs, Z. J. (2017). The digital entrepreneurial ecosystem. Small Business Economics, 49(1), 55–73. https://doi.org/10.1007/s11187-017-9867-5
- Szerb, L., Komlosi, E., Acs, Z. J., Lafuente, E., & Song, A. (2022). The digital platform economy index: 2020. Springer Briefs in Economics. https://doi.org/10.1007/978-3-030-89651-5
- Teece, D. J. (2018). Profiting from innovation in the digital economy: Enabling technologies, standards, and licensing models in the wireless world. Research Policy, 47(8), 1367–1387. https://doi.org/10.1016/j.respol.2017.01.015
- Tucker, C. E., & Zhang, J. (2011). How does popularity information affect choices? A field experiment. Management Science, 57(5), 828–842. https://doi.org/10.1287/mnsc.1110.1312
- van Dijck, J., Poell, T., & De Waal, M. (2018). The platform society: Public values in a connective world. Oxford University Press.
- von Briel, F., Davidsson, P., & Recker, J. (2018). Digital technologies as external enablers of new venture creation in the IT hardware sector. Entrepreneurship Theory and Practice, 42(1), 47–69. https://doi.org/10.1177/1042258717732779
- The Wall Street Journal (2021). Five tech giants just keep growing. https://www.wsj.com/articles/five-tech-giants-just-keep-growing-11619841644
- Wareham, J., Fox, P. B., & Cano-Giner, J. L. (2014). Technology ecosystem governance. Organization Science, 25(4), 1195–1215. https://doi.org/10.1287/orsc.2014.0895
- Yoo, Y., Henfridsson, O., & Lyytinen, K. (2010). The new organizing logic of digital innovation: An agenda for information systems research. Information Systems Research, 21(4), 724–735. https://doi.org/10.1287/isre.1100.0322
Appendix 1.
The digital platform economy (DPE) index
Structure of the digital platform economy (DPE) index
Szerb et al. (Citation2022) suggest a five-level composite indicator building methodology including (1) indicators (2) variables, (3) pillars, (4) sub-indices, and (5) the index. The index is called the digital platform economy (DPE) index and its sub-indices are the four frameworks (see below). The 12 components are called pillars. Pillars are the most important constituents of the model. Pillars are comprised from 24 variables, representing digital ecosystem (12) and entrepreneurship ecosystem (12). Variables are built from 61 indicators that are the elementary building blocks of DPE index. Szerb et al. (Citation2022) offer a full description of the structure of the DPE.
Table A1. The Digital Platform Economy index (DPE).
Data and variable normalization process
All pillars used to build the sub-indices of the DPE index include two variables: a variable representing the digital ecosystem (digital technology and digital users), and a variable accounting for the entrepreneurial ecosystem (institutions/infrastructures and businesses). briefly describes the data sources employed to generate the DPE variables and sub-indices. Because the data used to create the DPE variables result from multiple methodologies and have different scales, next we summarize the variable normalization process employed to calculate the DPE index. Szerb et al. (Citation2022) offer a comprehensive description of all variables included in the DPE (see, -A4 in Szerb et al., Citation2022, pp. 51–59), as well as full details on the computation of the DPE (see, Szerb et al., Citation2022, pp. 61–64).
Table A2. Data used to build the Digital Platform Economy index (DPE).
Following Szerb et al. (Citation2022), for each analyzed country (i = 1, … N) the 24 variables (j = 12 pillars) included in the DPE index (DPE_pillar) result from multiplying the variable representing the digital ecosystem (DE) and the variable capturing the entrepreneurship ecosystem (EE) as follows: .
Then, raw pillar scores are normalized using the distance methodology as follows:
Differences in the average of the normalized pillar values imply that reaching the same pillar value requires different efforts and resources. Consequently, the effect of additional resources to achieve the same marginal improvement of the pillar values is different and it is problematic for using the pillar values to public policy purposes. The average pillar adjustment methodology developed by Acs, Autio and Szerb (Citation2014) reduces but not fully eliminates this problem. Equations A1a–A1c present the calculation steps.
First, we calculate the average value of the normalized pillar values:
where is the average value of all j = 12 normalized pillars
Second, values are transformed such that their values fall in the (0,1) range:
where t is the “strength of adjustment,” the t-th moment of is exactly the needed average,
Third, the root of the following equation is identified for t:
For solution, the Newton–Raphson method is used with an initial guess of 0. After obtaining t, the computations are straightforward. After these transformations, the penalty for bottleneck methodology was used to create pillar-adjusted PFB values. A bottleneck is defined as the worst performing pillar or a limiting constraint in a particular country’s digital entrepreneurship system. Here, bottleneck is defined as the lowest level of a particular pillar, relative to other pillars in a particular country. We define our penalty function following as:
where is the modified, post-penalty value of pillar j in country i
is the normalized value of index component j in country i
Note, that the multiplication by 100 is purely practical to get a 0–100-point scale instead of the 0–1 range.
Sub-index calculation is simple, just taking the arithmetic average of its PFB-adjusted pillars for that sub-index.
where
= Digital technology infrastructure score for country i
= Digital user citizenship score for country i
= Digital multisided platform score for country i, and
= Digital technology entrepreneurship score for country i
Finally, the digital platform economy index (DPE) score is calculated as the simple arithmetic average of the four sub-indices.
Appendix 2
Table A3. Digital platform economy index (DPE) 2019: Country data.
Appendix 3
Table A4. Digital platform economy: Efficiency results (Stage 1: θ1; Stage 2: θ2; Overall: θ*).