Appreciating Statistics. I chose this title for my Presidential Address with a dual meaning in mind. The most obvious meaning, of course, is that what we do as statisticians should be appreciated, perhaps even more so than it is. But the second meaning has to do with the rapid rise of our profession in all sorts of ways. Our value truly is rapidly appreciating!
Let's look at some examples. The high school Advanced Placement Statistics exam, with which I have been involved since the first year it was offered, was given for the 20th time this past May (). In its first year, 1997, the exam was taken by fewer than 8000 students. This year? Almost two hundred and eight thousand! And that does not count the students who take the course but not the exam. 208,000! That's a lot of potential future statisticians!
Our value in the job market is appreciating as well. According to the Bureau of Labor Statistics, statistician is projected to be one of the fastest-growing jobs in the United States in the next 10 years, growing 34%, when the growth rate for all occupations is projected to be 7%. That places statistician 9th out of the hundreds of occupations listed by BLS (). Considering only occupations requiring at least a college degree, statistician is third in the list, preceded only by nurse practitioner and physical therapist.
The number of degrees awarded in Statistics and biostatistics is growing rapidly as well. The number of bachelor's degrees awarded annually has increased by more than 300% since the 1990s, and the number of Master's and PhD degrees awarded from the year 2000 to the year 2014 increased by 260% and 132%, respectively (; ASA Citation2015).
Yet even this amount of growth is not likely to meet the demand. Again according to the Bureau of Labor Statistics, employment for statisticians grew from about 20,000 positions in 2008 (Crank Citation2010) to about 30,000 in 2014, but that doesn't count many of us, such as professors or managers, who come under different occupational classifications in the BLS database (U.S. Dept. of Labor Citation2015; ASA Citation2015). And neither the current employment statistics nor the future projections include the rapidly growing numbers of other jobs that are not labeled “statistician,” but require substantial expertise in statistics. For instance, in 2014, LinkedIn placed “Statistical Analysis and Data Mining” at the top of its list of 25 Hottest Skills in demand (Murthy Citation2014). At the beginning of 2016 that category had fallen to number 2 overall, preceded by “Cloud and distributed computing,” but as LinkedIn noted in the accompanying report,
“Data isn' t [aren' t!] going anywhere. Our top skill category last year, statistical analysis and data mining, is still sitting comfortably at #2. It is the only skill category that is consistently ranked in the top 4 across all of the countries we analyzed. We still live in an increasingly data-driven world, and businesses are still aggressively hiring experts in data storage, retrieval and analysis.” (Murthy Citation2016)
So our value is appreciating! But with this increased demand comes a big challenge. There are not enough of us to meet it! By now most of you probably have heard the quote from the McKinsey Global Institute, that
“By 2018, the United States alone could face a shortage of 140,000 to 190,000 people with deep analytical skills as well as 1.5 million managers and analysts with the know-how to use the analysis of big data to make effective decisions.”
So what to do? Well, in my role as Chief Reader for Advanced Placement Statistics, I know there are thousands of dedicated high school statistics teachers who would love to learn more about possible careers in statistics for their students, and hundreds of thousands of students who would benefit from hearing from a professional statistician. So, as one of my Presidential initiatives, I put together a committee to formulate ideas for making that happen (). And wow did they come up with ideas! I will highlight some of their work here, and ask you to watch for more information coming soon on how you can get involved. Information will be provided through Amstat News, as well as the ASA Community forums. But first, I want to thank the Committee, led by ASA Board member Anna Nevius, for their amazing work; join me in a round of applause!
The major focus of this group has been to put together resources to prepare professional statisticians to visit high school classrooms. ASA will be rolling out a new website in the next few months, and this list of resources will be rolled out with it. Some of the resources include:
Tips and Tricks for meeting with a high school audience
Links to existing resources, such as the excellent videos and presentations at the website “ThisIsStatistics.org”
Resources and suggestions for making your presentation interactive
Information about “Statistics Plus”—the idea of combining statistics with whatever else interests you, including a (very long) list of college majors that require statistics ()!
I know you can't read this list, but that's because they found over 140 majors! As part of this ongoing project, we hope to create methods for connecting ASA members with local high school teachers. When I announced this initiative to the several hundred high school teachers who work together with college professors at the week-long Advanced Placement Statistics Reading, where the exams are scored, it met with a very enthusiastic response. So I know there are teachers who are ready to host ASA members who are willing to talk with their students, and I encourage you to be one of those members!
I will wrap up this version of “Appreciating Statistics” with an anecdote. When we were interviewing job candidates for a faculty position in my department this past year, one of them told me that it was a visit to her AP statistics class by a biostatistician from MD Anderson Cancer Center that convinced her to become a statistician. So you really can make a difference by visiting a high school classroom!
Now that I have addressed the first aspect of “Appreciating Statistics,” I will address the aspect that you probably thought of first when you heard the “Appreciating Statistics” title of this talk. What we do should be appreciated by everyone! And yet any of us who have responded to a stranger asking the “what do you do” question during casual conversation know that the most common reaction is not “Wow! I really appreciate what you statisticians do for the rest of us!”
In fact, a very common response seems to involve a complete misunderstanding of what we do. Very few people seem to realize how what we do benefits everyone in multiple ways. Let's look at some of those ways. One of my favorite concise accounts is SAS co-founder John Sall's (Citation2013) blog, celebrating the start of the International Year of Statistics. The blog entry was titled “Why Statistics is Essential” and one part of it read:
“Most people don't realize how essential statistics is. Our daily life is surrounded by the products of statistics. You brush your teeth. The fluoride in the toothpaste was studied by scientists using statistical methods to carefully assure the safety and effectiveness of the ingredient and the proper concentration. The toothpaste was formulated through a series of designed experiments that determined the optimal formulation through statistical modeling. The toothpaste production was monitored by statistical process control to ensure quality and consistency, and to reduce variability. The attributes of the product were studied in consumer trials using statistical methods. The pricing, packaging and marketing were determined through studies that used statistical methods to determine the best marketing decisions. Even the location of the toothpaste on the supermarket shelf was the result of statistically based studies. The advertising was monitored using statistical methods. Your purchase transaction became data that was analyzed statistically. The credit card used for the purchase was scrutinized by a statistical model to make sure that it wasn't fraudulent. So statistics is important to the whole process of not just toothpaste, but every product we consume, every service we use, every activity we choose. Yet we don't need to be aware of it, since it is just an embedded part of the process. Statistics is useful everywhere you look.”
Indeed! So why are we not appreciated more by the general public? I think part of it has to do with our lack of visibility. And why are we not more visible? Partly because, as John Tukey famously noted “We get to play in everyone's backyard!” And it's the front yard that everyone sees!
I like to think of us as “The Wizards behind the Curtain!” To illustrate this point, a few weeks ago I did a search of articles for the previous 30 days in the New York Times, I found 201 references to “Economist,” 50 references to “Psychologist,” 31 references to “Biologist,” but only 3 references to Statistician. And unfortunately, one was mentioning the statistician mother of a bride in a wedding announcement (congratulations if you are here!), one was in an article on Prozac and quoted someone saying “One can question whether I'm qualified to make that judgment. I'm neither a psychiatrist nor a statistician. But as the author of a book on mental illness, …” and, finally, congratulations to ASA member and Fellow Rafael Irizarry of Dana Farber and Harvard, who actually was quoted as an applied statistician!
Why are we not more visibly in the news? I think partly because, unlike economists who study issues in economics, psychologists who study issues in psychology, biologists who study issues in biology… we study all of those things! Those things are newsworthy, but we are not the ones the media would naturally think about as experts, even though, often, we are! In fact ASA maintains a media experts list with members willing to talk with the media, covering 148 topics!
Why should we care that we are not more visible? Partly because of the other “appreciating statistics.” As noted already, we need more of us! And one way to attract more young people to our profession is to be more visible about how important our work is to society, how many intriguing problems we get to work on, and how much we contribute to the betterment of almost every aspect of life!
But that's not the only reason I think statisticians need to be more prominent in the news and more involved with the media. Another reason is that statistical information is so often misrepresented in the news, and we are the ones who can help the media get it right. My father was in the newspaper business, so I know a little bit about how it works. For instance, I know that headlines generally are not written by the same person who writes the article. That might help explain why headlines are so often flagrantly misleading. Here are some of my favorite bad headlines, with thanks to Roxy Peck for providing some of them:
“Spanking lowers a child's IQ” (Los Angeles Times, 9/25/09)
“Joining a Choir Boosts Immunity” (Woman's World, 6/27/16)
“6 cups a day? Coffee lovers less likely to die, study finds” (NBC News website, 5/16/12)
“Want to live longer? Start walking—quickly” (Time magazine website, 1/4/11)
What's wrong with these headlines? In all cases, the study being discussed was observational, and I'm sure all of us can think of probable confounding variables in every one of them. And in many of these cases, so could the writer. In some cases the writer quoted the researchers providing examples of confounding variables and explaining that causal claims should not be made. But many people don't read past the headlines, and even those who do may not understand the difference between an observational study and a randomized experiment.
I think our job here is two-fold. First, we need to form better relationships with the media. We have made some headway in getting better representation on editorial boards of subject-matter journals, so journal reports of the studies behind these headlines may do a better job of explaining the shortcomings. But we need to do much more with direct media contact. The second thing we need to do is educate citizens, starting with our students, to be better consumers of statistical information. I will address each of these two “action items” in turn.
First, let's discuss the media. Those of you who attended Joe Palca's talk yesterday will have heard some great ideas for how we can do a better job with our media interactions. And if you missed his talk, it will be available on the JSM website.
In addition, two of my presidential initiatives are geared toward strengthening the presence of statisticians in the media. The first is a Media Training Program for ASA members. This program is designed to provide statisticians with the basic skills required for communicating with journalists and the public. The training will cover various media formats, including print, visual and audio, and will address topics such as how to respond to media inquiries, how to approach telephone, taped and live interviews, and how to initiate and respond to blog posts, op-ed pieces, and so on. A pilot program will take place at ASA headquarters in November. Details will be announced in the coming months. The pilot will be followed by a more permanent resource for all ASA members. Thanks to ASA Vice President Rob Santos, and the work group responsible for developing this initiative (). Please stand if you are here.
The second media initiative is called the Statistical Media Ambassador Program. The work group for this program was led by ASA Council of Chapters Representative to the Board, Wendy Lou, and the other members are shown in . Please stand if you are here.
For the Ambassador program, a select group of ASA members who already have some background in working with the media are being trained in-depth to serve as media spokespeople for ASA. These “Statistical Media Ambassadors,” will pledge to be available to work with the media on stories with statistical content, and will help develop materials for Stats.org. Stats.org is the website ASA currently sponsors, joint with Sense About Science USA, which provides in-depth analysis of statistical stories in the news. We expect to have about a dozen of these Ambassadors at a time, serving for terms of three years. The first Statistical Media Ambassadors training took place two days ago here at JSM, with training provided by the Alan Alda Center for Communicating Science.
We can help members of the media do a better job of providing accurate information to the public. But the other part of the equation is educating the public to be better consumers of that information. Let's look at a familiar set of scenarios. For example, a cartoon published in The New Yorker depicts a somewhat slovenly average Joe in a doctor's office, smoking a cigarette as the doctor warns him that “Lifelong smokers have a one-in-two chance of dying from smoking-related disease,” to which our average Joe responds “It'll never happen to me.” In the next frame Joe is holding a lottery ticket and being told “The odds of winning the powerball lottery are 80 million to one,” to which he responds “This could be my lucky day!”
I think helping people understand statistical studies and make better choices when probability is involved is our most difficult challenge. And that leads to the fourth of my presidential initiatives, which is to establish priorities for statistics education research, to help us figure out how to ensure that K – 16 students are able to develop the conceptual understanding needed to apply statistical thinking to various problem domains. We are working to obtain funding to hold a workshop this fall, to bring together representatives from the statistics education research community and complementary research communities such as science education, learning science, teacher education and computer science education. This initiative is led by ASA Director of Strategic Initiatives and Outcomes, Donna LaLonde. Please join me in thanking the members of the Work Group for this initiative ().
To convey the difficulty of developing statistical thinking, I would now like to spend some time discussing the work of psychologist and winner of the Nobel Prize in Economics, Daniel Kahneman. If you aren't familiar with his work, I strongly encourage you to become familiar with it, especially if you teach statistics. He has a lot to say that can help us understand why students have so much difficulty with what we teach, and why people make choices that as statisticians we may not think are rational.
My favorite of Kahneman's writing is his best-selling, award-winning book, Thinking, Fast and Slow (Citation2011). This book is a gold mine for statisticians who are trying to understand the way people think about what we do, or perhaps more importantly, what we hope they will think about (but don't) when they make choices based on what we do.
Let's look at some of Kahneman's examples of how statistical thinking does not come naturally. The first example was suggested to him by statisticians Howard Wainer and Harris Zwerling, and here I present a somewhat abbreviated version:
A study of kidney cancer in the 3,141 counties in the United States revealed a remarkable pattern. The counties with the lowest incidence are mostly rural, sparsely populated, and located in Republican states.
Now you are probably busy thinking about explanations. I doubt if most of you are giving credit to the Republicans, even if you are one, so you are most likely thinking that rural areas must be better for one's health—better air, perhaps healthier food, and so on.
But now let me give you another result from the same study:
The counties with the highest incidence of kidney cancer revealed a remarkable pattern. They are mostly rural, sparsely populated, and located in Republican states.
What's going on here? Perhaps even as trained statisticians it's not immediately obvious. The key is not any of the rural or Republican attributes of those counties, but rather, that those counties have small populations! And as we all should know, extreme proportions, both high and low, are more prevalent in small samples than in large samples. So even if long-term kidney cancer rates were the same across all counties in the United States, the extremes in any given year are more likely to show up in counties with small populations.
Kahneman goes on to note that the following two statements mean the same thing:
Large samples are more precise than small samples.
Small samples yield extreme results more often than large samples do.
He asserts that “the first statement has a clear ring of truth, but until the second version makes intuitive sense, you have not truly understood the first.”
Lack of understanding of the poor performance of small samples may be partially responsible for the recent and ongoing focus on reproducibility issues in areas such as psychology and medicine. A study published just a month ago in the prestigious journal Psychological Science surveyed 214 research psychologists, and found that a full 89% of them overestimated the power of specific research designs with a small expected effect size, and 95% underestimated the sample size needed to obtain adequate power for detecting a small effect (Bakker et al. Citation2016).
Daniel Kahneman attempts to explain this poor understanding and much more through his basic premise that humans have two mental systems for navigating through life, which he blandly calls “System 1” and “System 2.” System 1 is intuitive, automatic, and “fast thinking.” System 2 is controlled, logical and “slow thinking.” Hence the title of his book, “Thinking, Fast and Slow.”
The fast-thinking System 1 is more influential than we think, and it can often lead us astray. He devotes many chapters in his book to explaining what he calls a major puzzle:
“Why is it so difficult for us to think statistically? We easily think associatively, we think metaphorically, we think causally, but statistics requires thinking about many things at once, which is something that System 1 is not designed to do.”
One of the foils of System 1 for those of us trying to create statistically literate consumers, according to Kahneman, is that it yearns for stories with causal explanations. When given data or study results that don't warrant such explanations, we have a tendency to invent them. That's one reason the headlines I quoted earlier are so appealing (and appalling!). We want to explain the observed relationship, say, between walking faster and living longer in a way that makes sense to us, and the easiest way to do that is to formulate a story that tells us that walking fast is good for your health.
Now I know what you're thinking. You're thinking that you know better than to leap to that conclusion, because, after all, you know you can't make cause and effect conclusions from observational studies. But I bet I know what else at least some of you are thinking—you're trying to come up with a different explanation! For instance perhaps you think that causation is in the reverse direction—that people who are healthier are likely to walk more, and faster. Or you're thinking about possible confounding variables, such as the fact that walking for exercise is often a social activity, and social contact has beneficial health effects.
Our inclination to make up stories to explain the world around us is very, very strong. And in many situations, it's very beneficial. It fact, according to Kahneman (Citation2011), it may even impact our survival:
“System 1 has been shaped by evolution to provide a continuous assessment of the main problems that an organism must solve to survive: How are things going? Is everything normal? Should I approach or avoid? The questions are perhaps less urgent for a human in a city environment than for a gazelle on the savannah, but we have inherited the neural mechanisms that evolved to provide ongoing assessments of threat level, and they have not been turned off.”
Let's look at another example of intuition versus statistical thinking provided by Kahneman and his long-time collaborator, Amos Tversky. This one is so well publicized that you may have heard it, but bear with me for those who have not. It's the “Linda” story, and it goes like this.
“Linda is thirty-one years old, single, outspoken, and very bright. She majored in philosophy. As a student, she was deeply concerned with issues of discrimination and social justice, and also participated in anti-nuclear demonstrations.”
Now for the question:
Which alternative is more probable?
Linda is a bank teller.
Linda is a bank teller and is active in the feminist movement.
The results? By a very large majority, people choose the second option. But it clearly can't be more probable, because it represents an intersection of two features, one of which is given as the first option! The problem, as Kahneman notes, is “the uncritical substitution of plausibility for probability.”
Here's another example of the dominance of the story-telling System 1 over the logical and rational System 2. Which do you think is more probable?
A massive flood somewhere in North America next year, in which more than 1000 people drown.
An earthquake in California sometime next year, causing a flood in which more than 1000 people drown.
You get the idea. People judged the second scenario to be more probable because it contains a plausible and easily understandable story line.
There are numerous other examples of how people are not good at processing statistical and probabilistic information:
Confusing the probability of having a disease conditional on a positive test result with the probability of a positive test result given one has the disease, which can be very different, especially for low prevalence diseases.
Overestimating the risk of catastrophic and rare events that are in the news, and underestimating common risks, such as having a fear of flying but not of driving, even while texting!
Overestimating the likelihood of positive outcomes and underestimating the likelihood of negative ones, as illustrated by the earlier cartoon comparing smoking to buying a lottery ticket.
Embracing data that support our beliefs, and ignoring data that contradict them, called the “confirmation bias.”
Before moving on to what we can do about these issues, here is a summary of how the fast-thinking, intuitive System 1 can interfere with sound statistical System 2 thinking. Again paraphrasing Kahneman, System 1 thinking:
Invents causes and intentions
Focuses on existing evidence and ignores absent evidence
Neglects ambiguity and suppresses doubt
Is biased to believe and confirm (the so-called confirmation bias, in which people hear what they already believe and ignore the rest)
Overweights low probabilities
Frames decision problems narrowly, in isolation from one another
So, now that we know most of our time is spent in the System 1 mode of thinking, and that it can be very misleading when it comes to interpreting data and probability, what can we do about it? As statisticians, and especially addressing those of you who are statistics educators, our challenge is how to teach people to overcome the tendency to jump to unwarranted conclusions and make unwise choices, in spite of their (and our!) natural inclination to do so.
So I contend that our hardest problem is not merely to get people to understand statistics and statistical thinking. But rather, our hard problem is how to overcome eons of evolutionary advantage for System 1 thinking. That system seems to have worked pretty well. But as we have seen, it does not work well for the kinds of information that can help people make better decisions in a complex, multi-variable world.
To help address our challenge, I offer a quote from the book Concepts and Controversies, authored by another of my favorite statistical thinkers, David Moore.
DATA BEAT ANECDOTES
This quote is repeated in the College version of the ASA's Guidelines for Assessment and Instruction in Statistics Education, better known by its acronym, GAISE, as something we hope students will believe and understand after taking a statistics course.
But I would like to question whether anyone actually lives under the guiding principle that Data beat Anecdotes. I certainly haven't seen much evidence of that. Even a cursory knowledge of what's happening in politics should convince us that anecdotes often beat data, and powerfully so.
I can provide a more concrete example based on the research I have done in parapsychology. Parapsychology is concerned with the scientific investigation of potential skills that are commonly known as psychic abilities, such as precognition, telepathy, and so on. For many years I have worked with researchers doing very careful work in this area, including a year I spent working on a classified project for the United States government, to see if we could use these abilities for intelligence gathering during the Cold War. This 20-year project is described in the recent book ESP Wars East and West by physicist Edwin May, the lead scientist on the project, with input from his Soviet counterparts.
At the end of that project I wrote a report for Congress, stating what I still think is true. The data in support of precognition and possibly other related phenomena are quite strong statistically, and would be widely accepted if they pertained to something more mundane. Yet, most scientists reject the possible reality of these abilities without ever looking at data! And on the other extreme, there are true believers who base their belief solely on anecdotes and personal experience. I have asked the debunkers if there is any amount of data that could convince them, and they generally have responded by saying, “probably not.” I ask them what original research they have read, and they mostly admit that they haven't read any! Now there is a definition of pseudo-science—basing conclusions on belief, rather than data!
When I have given talks on this topic to audiences of statisticians, I show lots of data. Then I ask the audience, which would be more convincing to you—lots more data, or one strong personal experience? Almost without fail, the response is one strong personal experience! Of course I'm giving you an extreme example, and I think people are justifiably skeptical, because most people think that these abilities contradict what we know about science. They don't, but that's the subject for a different talk!
So, what do we really mean when we say “Data beat anecdotes?” And if it's not always true even for statisticians, how can we possibly expect to instill it in our students, or the broader public? I would like to propose a modification. Instead of DATA BEAT ANECDOTES, how about:
DATA PLUS ANECDOTES! Or Better Yet, DATA PLUS STORIES!
What do I mean by this? If you read news reports by engaging science writers, you will have noticed that they use this tactic in their stories. They start with a compelling human interest story before delving into the less interesting details of the work they are describing. This technique of combining stories and data can be very effective. Whether you teach statistics, or work as a professional statistician, your work can have a greater impact if you can tell a story to accompany your data.
Let's look at a few examples. Suppose you are trying to teach students about what can go wrong with election polling, certainly a hot topic right now! You could give them a laundry list of why polls aren't what they used to be—fewer landlines, caller ID that keeps people from answering the phone, and so on. Or, you could give them those details accompanied by an interesting example, such as the explanation provided by YouGov the day after the Brexit vote, in which they predicted exactly the opposite of what happened ():
“We do not hide from the fact that YouGov' s final poll miscalculated the result by four points. This seems in a large part due to turnout—something that we have said all along would be crucial to the outcome of such a finely balanced race. Our turnout model was based, in part, on whether respondents had voted at the last general election and a turnout level above that of general elections upset the model, particularly in the North.”
Figure 9. Yougov.com' s predictions over time for the “Brexit” vote held on June 23, 2016, in which 52% voted to leave the European Union.
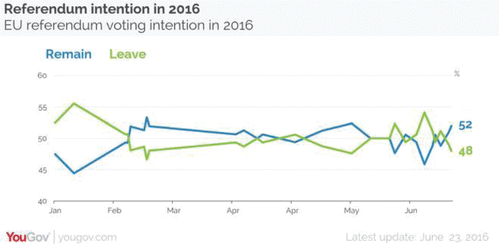
Here is another example. Suppose I want to present the concept of expected value to my students. I can tell them this story:
Last October I was planning a trip for December, but I wasn' t 100% sure I would be able to go because of some possible family obligations for the holidays. I found a hotel that was perfect for my needs, and thought I would reserve a room. But when I entered the dates to find out the cost, I was presented with two options. I could pay $85 now, with no option for a refund, or I could pay $100 on arrival, with no obligation as long as I cancelled at least 24 hours in advance. What to do?
This story provides a simple illustration of the use of expected value in a real life situation, and is much more likely to get students’ attention than simply presenting the formula for expected value. I can then ask them questions, such as, if I think I only have a 50/50 chance of going, is the prepaid option a good choice? What is the minimum probability that makes it a good choice? I can then extend the discussion to issues like extended warranties, insurance, and other situations that rely on an understanding of expected value.
I could go on and on, in fact I could write a whole book about using stories like this—oh wait, I did [Seeing Through Statistics; Utts Citation2015]!
So I encourage those of you who teach introductory statistics to a general audience to stop wasting time on things like why we use (n – 1) instead of n, or presenting formulas that don't help with understanding, and start incorporating stories, especially ones that will help students become educated consumers of statistical information. We have important stories to tell, and for most students we have only one quarter or semester to tell them. Let's not waste that chance.
As statisticians, we all have important stories to tell. No matter where you work or what you do, I encourage you to TELL OUR STORIES to your employers, to your students, to a high school class, to the media, and to the public. Let's help everyone appreciate statistics! Thank you!
References
- American Statistical Association (2015), “More Students Earning Statistics Degrees, But Not Enough to Meet Surging Demands for Statisticians,” press release, available at http://www.amstat.org/asa/files/pdfs/pressreleases/2015-StatsDegreeGrowth.pdf.
- Bakker, M., Hartgerink, C. H. J., Wicherts, J. M., and van der Maas, H. L. J. (2016), “Researchers’ Intuitions About Power in Psychological Research,” Psychological Science, 27, 1069–1077.
- Crank, K. (2010), “Counting Statisticians: How Many of Us Are There?” Amstat News, available at http://magazine.amstat.org/blog/2010/05/13/countingstatisticians510/.
- Kahneman, D. 2011, Thinking, Fast and Slow, New York: Farrar, Straus, and Giroux.
- Murthy, S. (2014), “The 25 Hottest Skills That Got People Hired in 2014,” available on Linkedin at https://blog.linkedin.com/2014/12/17/the-25-hottest-skills-that-got-people-hired-in-2014
- Murthy, S. (2016), “The 25 Hottest Skills That Got People Hired in 2016,” available on Linkedin at https://blog.linkedin.com/2016/01/12/the-25-skills-that-can-get-you-hired-in-2016
- Sall, J. (2013), “Why Statistics is Essential,” JMP Blog, SAS Blogs Home, available at http://blogs.sas.com/content/jmp/2013/01/14/why-statistics-is-essential/
- United States Department of Labor (2015), Labor Force Statistics from the Current Population Survey, available at http://www.bls.gov/cps/cpsaat11.htm
- Utts, J. (2015), Seeing Through Statistics (4th ed.), Boston, MA: Brooks Cole/Cengage Learning.