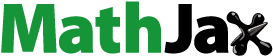
The subject of Statistics can be broadly classified into two components, namely theoretical statistics and applied statistics. Excelling in the latter area is not possible if one does not have a solid knowledge of the foundational concepts in the former area. The soul of theoretical statistics is the field of statistical inference, where one uses probabilistic and mathematical tools to infer about the unknown characteristics of a population based on some data at hand. Hence, developing a comprehensive background in the theory of statistical inference is mandatory for every statistician/data scientist, irrespective of the actual field they are working on. While there is no shortage of excellent books on this particular subject, aimed at audiences at the undergraduate or even the masters level, for example, Casella and Berger (Citation2001), it is, in my opinion, significantly harder to find a good book unifying all the concepts in statistical inference at the advanced (Ph.D.) level. While there are excellent books available on individual areas in statistical inference such as the theory of estimation, the theory of hypothesis testing, decision theory, or linear models, finding a good book that combines all these fundamental fields at an advanced level can be quite challenging. The present textbook Theory of Statistical Inference, in my opinion, fills this gap quite nicely, and is in fact, accessible to students at the masters or even at the undergraduate level, having a comparatively advanced background.
Theory of Statistical Inference, in my opinion, provides an excellent background on a wide variety of areas in statistical inference, starting from the basic and fundamental areas such as methods of estimation, hypothesis testing and decision theory, and ranging to the much more advanced areas such as group structure and invariant inference. This large variety of topics will make this textbook suitable for students at all level, and make it an extremely useful resource for lecturers to prepare their class-notes from.
The book starts with brief introductions on some standard topics in probability, such as distribution theory and multivariate analysis (Chapters 1 and 2), which is crucial for students lacking sufficient background in probability theory, but otherwise interested in learning theoretical statistics. Chapter 1 covers all the fundamental areas in advanced probability very briefly, such as probability measures, expectation, independence, conditional distributions, probabilistic inequalities, -spaces, quantiles and basic distribution theory. Chapter 2 gives a very nice account of multivariate distributions, the Cramer-Wold device, multivariate transformations of distributions (the Jacobian technique), order statistics, the Fisher-Cochran theorem for quadratic forms, and various test statistics based on the sample mean and sample covariance. The exercises in my opinion, also provide a very detailed practice in these areas, and are particularly useful for students who do not have a previous background in these fields.
Chapter 3 is from where the topic of statistical inference really starts. It provides a very detailed discussion on statistical models, covering topics from as basic as parametric families and likelihood functions, to some of the core areas of parametric inference, such as sufficiency, completeness, exponential families and various inference principles. In this chapter, the author also gives a very brief introduction on Bayesian models. Although the idea of combining all these important topics into one single introductory chapter might be arguable, in my opinion, this is a good idea, since it gives a brief glimpse to every aspect of inference to the reader at one glance.
Chapters 4 and 5 together teach the two central pillars of inference, namely estimation and hypothesis testing. These are the chapters everyone interested in studying statistical inference, should learn. They cover important concepts like the maximum likelihood estimator, method of moments estimator, equivariant and shrinkage estimators, likelihood ratio tests, etc. The central concepts in estimation and hypothesis testing, namely UMVU estimation, UMP tests and the Neyman-Pearson lemma are not studied in these chapters, but instead deferred to later portions of the book (Chapters 8 and 10, respectively). This is something about which I feel that it might have been a somewhat better idea to present these in chapters 4 and 5 themselves, but once again, it’s just a matter of taste.
Chapter 6 is on linear models, an area that is not so widely seen in textbooks on statistical inference, yet of paramount interest in both theory and applications. It covers the basic aspects of linear regression, such as distribution theory of the estimates of the regression coefficient and error variance and its applications in constructing confidence intervals, generalized least squares estimates and ANOVA. I really like how in spite of being a textbook on theoretical statistics, the author keeps the application side in mind, which is in particular evident from Exercise 6.28, where the reader is asked to perform a numerical study using the statistical software R.
Chapter 7 lays the foundation of decision theory, by describing notions of performance of statistical estimators, such as the MSE, risk and admissibility. It also discusses a slightly general version of the celebrated Karlin’s theorem due to Lehmann and Casella (Citation1998), which provides a useful sufficient condition for admissibility in one-parameter exponential families. It would be nice to see a proof of this result presented in the book, at least in one of the appendix sections, though.
Chapters 8 and 10, as mentioned earlier, provide detailed discussions on two fundamental concepts in estimation and testing, namely UMVU estimators and UMP tests. The main technical tool in Chapter 8 is the Lehmann-Scheffé theorem which provides a highly applicable sufficient condition for a statistic to be the unique UMVU estimator of a parameter. The central object of Chapter 10 is the Neyman-Pearson lemma, which constructs the most powerful test in terms of the likelihood ratio. Some nice applications of this result have been shown in context of the monotone likelihood ratio property of a parametric family. There is also a separate subsection dedicated to the generalized Neyman-Pearson lemma, that makes Chapter 10 even more suitable for the advanced reader willing to gather detailed knowledge on hypothesis testing. These two chapters basically summarize the important aspects of the two pioneering books Theory of Point Estimation by E. Lehmann and G. Casella (Citation1998) and Testing Statistical Hypothesis by E. Lehman and J. Romano (2005).
Chapter 9 on group structure and invariant inference, is definitely the most theory-intensive part of the entire book. It requires knowledge of group theory from the reader. The reader should also note that if there is any chapter in the entire book that might be skipped, it is this one. This chapter covers Minimum Risk Equivariant (MRE) estimators for location and scale parameters, invariant density families and invariant hypothesis testing.
Chapter 11, once again, digresses a bit from the topic of statistical inference, and discusses some of the important areas in advanced probability theory, related to convergence of random variables and laws of large numbers. Apart from the classical results, I appreciate the idea of the author to include a separate subsection on CLT for triangular arrays, a class of results that is often useful in many statistical applications. The purpose of this chapter, in my opinion, is to prepare the reader for the next three chapters on large sample theory.
Chapter 12 describes various tools involved in large sample estimation and their applications, for example the -method and variance-stabilizing transformations (which have obvious applications in construction of confidence intervals using pivots), their multivariate versions, Bahadur representation of quantiles, large sample theory for U-statistics, and asymptotic efficiency. This chapter is once again nicely composed, the only comment that one can possibly have, is that probably it would have been nice to see a proof of Bahadur’s representation theorem in the book itself.
Finally Chapters 13 and 14, in my opinion, are also addressed to the more advanced reader willing to pursue a research career in statistics. Chapter 13 is on the asymptotic theory for estimating equations, and talks about consistency and asymptotic normality of M-estimators, with applications in maximum likelihood estimation and regression. In this chapter, the author also discusses a bit about generalized linear models. Chapter 14 talks about large sample hypothesis testing, and describes large sample asymptotics of common hypothesis tests, such as the score test, the likelihood-ratio test, the Wald test and Pearson’s goodness-of-fit test. I would like to see an expansion of Section 14.8 on the power analysis of approximate -tests in future editions of the book.
The three greatest strengths of this textbook are:
The exceptionally wide variety of topics that it covers, ranging from preliminary to a quite advanced level,
The solid probabilistic background that it gives (Chapters 1, 2, and 11) to prepare the reader who is not so much familiar with probability theory in general, for his journey in learning theoretical statistics, and
The comprehensive collection of exercises that it has to offer for practice to the readers.
The large variety of topics covered in the current version allows students at all level, be it bachelor, master or Ph.D. to find topics suitable for their own study. On one hand, the book contains fairly advanced topics to help researchers in their own research areas, and on the other hand, it provides enough materials for lecturers to prepare lecture notes and design an entire course on theoretical statistics. Overall, I am extremely excited about Theory of Statistical Inference and eagerly waiting for numerous students, researchers, lecturers and even working professionals to enjoy the immense benefit coming out of its publication.
National University of Singapore
[email protected]
References
- Casella, G., and Berger, R. L, (2001), Statistical Inference (2nd ed.), Pacific Grove, CA: Duxbury, Thomson Learning.
- Lehmann, E., and Casella, G. (1998), Theory of Point Estimation, New York: Springer.
- Lehmann, E., and Romano, J. (2005), Testing Statistical Hypotheses, New York: Springer.