Abstract
Most optimization-based motion planners use a naive linear initialization, which does not use previous planning experience. We present an algorithm called ‘Gaussian mixture spline trajectory’ (GMST) that leverages motion datasets for generating trajectories for new planning problems. Unlike other trajectory prediction algorithms, our method does not retrieve trajectories from a dataset. Instead, it first uses a Gaussian mixture model (GMM) to modelize the likelihood of the trajectories to be inside the dataset and then uses the GMM's parameters to generate new trajectories. As the use of the dataset is restricted only to the learning phase it can take advantage of very large datasets. Using both abstract and robot system planning problems, we show that the GMST algorithm decreases the computation time and number of iterations of optimization-based planners while increasing their success rates as compared to that obtained with linear initialization.
GRAPHICAL ABSTRACT
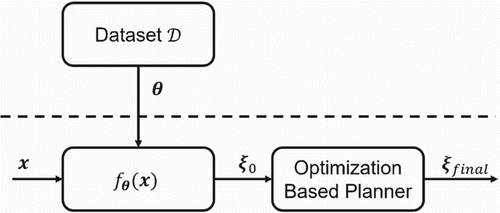
Disclosure statement
No potential conflict of interest was reported by the authors.
ORCID
T. Barbié http://orcid.org/0000-0001-7506-4453
Additional information
Notes on contributors
T. Barbié
T. Barbie received the MSc degree in aerospace from the ISAE-Supaero, Toulouse, France in 2016 and the MSc degree in artificial intelligence from the University Paul Sabatier, Toulouse, France in 2016. He is currently pursuing the PhD degree in robotics at Kyushu Institute of Technology, Kitakyushu, Japan. His research interests include motion planning, trajectory prediction and machine learning.
R. Kabutan
R. Kabutan received his MSc degree from Kyushu Institute of Technology, Kitakyushu, Japan in 2017. His research interests include industrial robotic and motion planning.
R. Tanaka
R. Tanaka received his MSc degree from Kyushu Institute of Technology, Kitakyushu, Japan in 2018. From 2018, he is a PhD student at the Department of Intelligent Interaction Technologies on University of Tsukuba. His research interests include control engineering, path planning, and robotics. He is a student member of the Robotics Society of Japan.
T. Nishida
T. Nishida received his MSc and PhD degrees from Kyushu Institute of Technology in 1999 and 2002, respectively. From 2002 to 2012, he was an Assistant Professor at the Kyushu Institute of Technology. From 2013, he has been an Associate Professor at the same institution. His research interests include control engineering, artificial intelligence, and robotics. He is an active member of the Society of Instruments and Control Engineering, Robotics Society of Japan, and so on.