Abstract
In this study, a knee-assisted exoskeleton that can assist the wearer's knee joints at the appropriate phase of the gait cycle is designed. A convolutional neural network (CNN) model is used in the gait control to identify the locomotion modes of the exoskeleton. In order to verify the CNN-based locomotion mode identification method, an offline CNN model was created in MATLAB. The experiment was conducted and seven healthy subjects’ hip, knee, and ankle joint angles were captured and six common locomotion modes were recognized in the experiments. The recorded data were segmented with overlapping sliding windows of varying sizes and were tested using a stratified 5-fold cross-validation technique. The experimental findings showed that the appropriate window size was 1.25T (T stands for the normal adult gait cycle). The lowest and the highest accuracies of the test sets at the window size of 1.25T were 96.47% ± 0.83% and 99.67% ± 0.49% on different subjects. It also showed that the model has strong generalization. Thus, the algorithm had proved useful to extract the characteristics of lower limb joint angles in various locomotion modes and the approach in this paper can be extended to the recognition of motion patterns in lower limb exoskeletons.
GRAPHICAL ABSTRACT
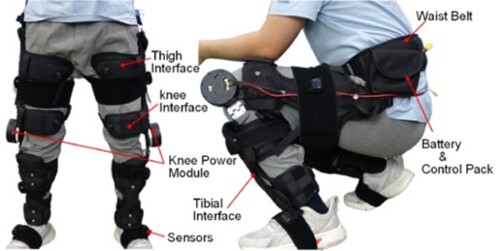
Acknowledgements
The authors thank the anonymous reviewers for their constructive remarks and suggestions for improving this paper.
Disclosure statement
No potential conflict of interest was reported by the author(s).
Additional information
Notes on contributors
Aimin Xu
Aimin Xu, born in 1998. He received his B.E. degree in Mechanical Engineering from Lanzhou University of Technology, China, in 2016. He is currently working towards the master degree in South China University of Technology, China. His research interests include wearable robotics, lower limb exoskeleton control, and man-machine system.
Chenxi Qu
Chenxi Qu received his B.E. degree from Jilin University, China, in 2016. He is currently a PhD candidate at School of Mechanical, Aerospace and Civil Engineering, University of Manchester, Manchester. His research interests include intelligent robotics, prosthetic system control, and anthropomorphic robotic hand.
Liang Yang
Liang Yang was born in Wuhan, Hubei, P.R. China, in 1981. He received master degree from University of Sunderland, United Kingdom. Now, he is currently a PhD candidate at School of South China University of Technology, China. His interests include exoskeleton design, wearable robotics, work-related musculoskeletal disorders, and applied computing.
Peng Yin
Peng Yin, born in 1990. He received the master and Ph.D. degree in School of Mechanical and Automotive Engineering, South China University of Technology, China, in 2016 and 2020, respectively. His research interests include man-machine system and intelligent robotics.
Jiliang Lv
Jiliang Lv, born in 1996, is currently a PhD candidate at School of South China University of Technology, China. He received his master degree from School of South China University of Technology, China, in 2020. His research interests include exoskeleton design, motion control of intelligent robotics.
Shengguan Qu
Shengguan Qu, born in 1966. He received his Ph.D. in Mechanical Engineering from South China University of Technology, China. He has been a tutor at the School of Mechanical and Automotive Engineering, South China University of Technology since 2005. In 2009, he has been elevated as a doctoral supervisor. His research interests include exoskeleton design, man-machine system, intelligent robotics, and industrial automation.