Abstract
The increasing demand for water in southern Africa necessitates adequate quantification of current freshwater resources. Watershed models are the standard tool used to generate continuous estimates of streamflow and other hydrological variables. However, the accuracy of the results is often not quantified, and model assessment is hindered by a scarcity of historical observations. Quantifying the uncertainty in hydrological estimates would increase the value and credibility of predictions. A model-independent framework aimed at achieving consistency in incorporating and analysing uncertainty within water resources estimation tools in gauged and ungauged basins is presented. Uncertainty estimation in ungauged basins is achieved via two strategies: a local approach for a priori model parameter estimation from physical catchment characteristics, and a regional approach to regionalize signatures of catchment behaviour that can be used to constrain model outputs. We compare these two sources of information in the data-scarce region of South Africa. The results show that both approaches are capable of uncertainty reduction, but that their relative values vary.
Editor D. Koutsoyiannis
Citation Kapangaziwiri, E., Hughes, D.A., and Wagener, T., 2012. Incorporating uncertainty in hydrological predictions for gauged and ungauged basins in southern Africa. Hydrological Sciences Journal, 57 (5), 1000–1019.
Résumé
La demande en eau croissante en Afrique australe nécessite de quantifier correctement les ressources en eau douce actuelles. La modélisation des bassins versants constitue l'outil courant pour générer des estimations continues de débit et d'autres variables hydrologiques. Toutefois, la précision des résultats est rarement quantifiée, et l’évaluation des modèles souffre de la pénurie d'observations historiques. La quantification de l'incertitude des estimations hydrologiques augmenterait la valeur et la crédibilité des prévisions. Nous présentons un cadre indépendant du modèle visant à assurer la cohérence de l'intégration et de l'analyse de l'incertitude dans les outils d'estimation des ressources en eau dans les bassins jaugés et non jaugés. L'estimation de l'incertitude dans les bassins non jaugés est réalisée selon deux stratégies: une approche locale pour une estimation a priori des paramètres du modèle à partir des caractéristiques physiques des bassins, et une approche régionale visant à régionaliser les caractéristiques du régime des bassins pouvant être utilisées pour contraindre les résultats des modèles. Nous avons comparé ces deux sources d'information en Afrique australe où les données sont rares. Les résultats montrent que les deux approches sont susceptibles de réduire l'incertitude, mais que leur valeur relative varie.
1 INTRODUCTION
Water problems are acute in Africa, where an estimated 300 million people are affected by water shortages (WWAP Citation2009). Adequate planning and sustainable management of the continent's freshwater resources are crucial for its socio-economic growth. The transboundary nature of major river basins requires common understanding and approaches that have the potential to benefit all riparian countries. A major constraint to effective and sustainable development of water resources in southern Africa is the lack of reliable baseline information. In many cases, streamflow in the region is impacted by upstream abstractions or return flows, while data are generally unavailable, limited, or of poor quality, effectively rendering most of the basins ungauged. With rapid population growth, expanding urbanization, increased economic development and negative impacts of climate change being predicted for the region (Rosegrant and Perez Citation1997), water will become a limiting resource, especially in the largely semi-arid parts of southern Africa. There is therefore a growing need for accurate monitoring, simulation and long-term forecasting of hydrological variables to support effective water resources management.
While simulation models have been used within the region for nearly four decades, the risks of making decisions based on uncertain and unreliable information have not yet been evaluated. The International Association of Hydrological Sciences (IAHS) Predictions in Ungauged Basins (PUB) initiative aims to increase understanding and to reduce predictive uncertainty in data-sparse regions (Sivapalan et al. Citation2003). Notwithstanding the frequently demonstrated utility of models in water resources management, it is necessary to explicitly acknowledge the limitations of pursuing optimum solutions based on the use of imprecise representations of reality and forcing data (Wagener and Gupta Citation2005). Model prediction uncertainty should be an integral component of model applications (Pappenberger and Beven Citation2006). The incorporation of uncertainty estimation in models should help to quantify the level of confidence in the results, inform and improve decision- and policy-making processes, leading to better risk assessment (Reichert and Borsuk Citation2005).
Many approaches to incorporating uncertainty estimation in hydrological modelling have been proposed (e.g. Beven and Binley Citation1992, Vrugt et al. Citation2003, Kavetski et al. Citation2006, Kuzcera et al. 2006). A common factor of these frameworks is that they use some form of a likelihood measure to assess which models/parameters are potential candidates to represent the hydrological system under study. However, the value of these strategies in poorly gauged regions like southern Africa is at least reduced, since likelihoods cannot be calculated from locally observed data in many cases due to the absence of stream gauges (Wagener and Montanari Citation2011). Two recent uncertainty approaches that are applicable to ungauged or data-poor basins are ensemble predictions (McIntyre et al. Citation2005), and the use of indices of expected basin behaviour to constrain the output of a priori ensembles (Yadav et al. Citation2007). In ensemble modelling, the outcome is a range of possible models (and parameter sets) describing a given system developed through sampling from the feasible parameter space (Beven and Binley Citation1992). Often, different rejection criteria, based on goodness-of-fit-measures, are used to decide whether a feasible parameter set is a possible (a behavioural parameter set) representation of the system under study or not (Freer et al. Citation1996, Beven and Freer Citation2001). Of great practical importance for the application of such an approach to less-developed countries is the development of rejection criteria in the absence of local observations, i.e. providing answers to the problem of transferring information on acceptable model performance to ungauged basins (Wagener and Montanari Citation2011). Yadav et al. (Citation2007) introduced an approach based on the estimation and regionalization of ranges of basin-scale streamflow characteristics. The model output ensembles are compared with the regionalized signatures, and those not consistent with this information (while considering regionalization uncertainty) are rejected. The same principles were also used by Winsemius et al. (Citation2009) in Zambia, by Bulygina et al. (Citation2009) in the UK, by Singh et al. (Citation2011) in the USA, and by Zhang et al. (Citation2008) in the UK, among others.
Thus, reducing uncertainty in hydrological predictions has generally been approached in two ways (Wagener and Montanari Citation2011):
• | a focus on the reduction of uncertainty in the estimation of model parameters either through use of local information on physical catchment characteristics to derive a priori values (local approach), or through the regionalization of model parameters from gauged catchments, and | ||||
• | a focus on the reduction of uncertainty in the expected watershed functional behaviour through the regionalization of streamflow signatures that can subsequently be used to constrain acceptable model behaviour (regional approach). |
This paper explores the value of these two approaches and how they can be combined in order to utilize as much available information in the region as possible in making hydrological predictions in gauged and ungauged basins. The local approach utilizes locally available information on physical catchment characteristics, such as soils and land use, and results in model parameter sets estimated directly from physical basin attributes (Kapangaziwiri and Hughes Citation2008, Kapangaziwiri Citation2011). This avoids the need for model calibration against observations, while the regional approach provides hydrological response indices that can be used to further constrain the model predictions. An alternative to a priori parameter estimation is the regionalization of model parameters from similar catchments (Merz and Blöschl Citation2004, Hartmann and Bárdossy Citation2005, Wagener and Wheater Citation2006). The second strategy uses regionalized streamflow signatures based on gauged catchments. It should be acknowledged that the observed discharge data used for the development of the signature relationships are also uncertain (Di Baldassarre and Montanari Citation2009), which can affect hydrological predictions (e.g. Blazkova and Beven Citation2009). In South Africa, while quality control always forms part of the data preparation process, there will always be inaccuracies in the data and the naturalization process that contribute to uncertainties in the regional relationships.
In this study, the Pitman (Pitman Citation1973, Hughes et al. Citation2006) monthly rainfall–runoff model is applied within an uncertainty framework (Kapangaziwiri et al. Citation2009) to several South African regions which have relatively good data support. The intention is to eventually apply the approach to the whole of southern Africa to harmonise and improve water resources estimation. The main aim of this paper is to assess the applicability of the framework in South Africa. Our specific objectives are to estimate and incorporate uncertainty into an a priori parameter estimation approach for the Pitman model and to develop regional signature equations (including uncertainty). Lastly, we investigate the sensitivity of the a priori parameters in different environments to provide feedback to the estimation process so that it can be improved and uncertainty be reduced even further.
2 A PUB FRAMEWORK FOR MODEL APPLICATION IN GAUGED AND UNGAUGED BASINS IN SOUTHERN AFRICA
Kapangaziwiri et al. (Citation2009) proposed an uncertainty framework for hydrological predictions in both gauged and ungauged basins in southern Africa based on the strategies discussed above (). While this earlier work offered some preliminary results, the current work represents a more thorough testing and critical appraisal of the framework's components. The general framework is intended to be model independent even though the development and testing have focused on a version of the Pitman model widely used in southern Africa (Hughes et al. 2006). The framework consists of a component for directly estimating local parameter priors using physical basin characteristics and their variability. The second part of the framework uses regionalized catchment functional characteristics reflected in hydrological signatures to constrain ensembles of flow predictions generated by sampling from the parameter priors.
Fig. 1 The model-independent model application and evaluation framework that can be used in southern Africa.
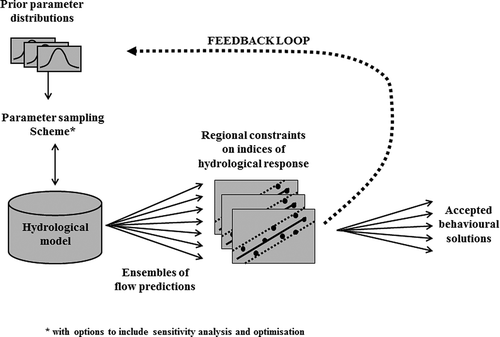
Sensitivity analysis is used to determine how the uncertainty in the model predictions can be apportioned to different parameters (Saltelli et al. Citation2008). Many global sensitivity analysis techniques are derivatives of the regional sensitivity analysis (RSA) idea of Hornberger and Spear (Citation1981), e.g. Freer et al. (1996). The analysis method used here is a variation of the technique used by Wagener et al. (Citation2001), where model outputs are evaluated against a chosen assessment criterion. While Wagener et al. (Citation2001) rank the model output ensembles on the basis of an objective function, the sensitivity analysis tool used in this study extends the approach to include the constraint metrics when observed data are not available to calculate objective functions (Demaria et al. Citation2007, Zhang et al. Citation2008). The output ensembles are ranked based on the selected assessment criterion with no explicit weighting, and a normalized cumulative frequency distribution is plotted for each group (Demaria et al. Citation2007). When using the hydrological constraints, there are potentially seven groups. Two of the groups are those that lie outside the limits of the regional constraints, while the other five are the 20th percentiles lying within the constraints. This approach has similarities with the “limits of acceptability” idea used more recently in approaches based on the GLUE principles (Blazkova and Beven Citation2009).
This use of regionalized constraints described here enables model diagnostic evaluation and sensitivity analysis in ungauged basins where traditional residual-based objective functions cannot be used to determine behavioural thresholds. Accurate historical streamflow records are not readily available in many regions of southern Africa, and regionalized constraints therefore fulfil an important role.
2.1 The Pitman model
The Pitman model is a conceptual, monthly time-step and spatially semi-distributed water balance model that has been widely used as a practical water resource assessment tool in South Africa. The version of the model used in this study has explicit surface water–groundwater interaction routines (Hughes Citation2004). shows the flow diagram of the model, including its parameters (). Runoff is mainly generated by two model functions. The first is an asymmetrical triangular distribution of catchment absorption rates defined by parameters ZMIN, ZAVE and ZMAX. The second function determines the drainage rate from the main moisture storage (S, mm, with a capacity of ST, mm). This storage is depleted by evapotranspiration, interflow and groundwater recharge. The maximum interflow (FT, mm month-1) and recharge (GW, mm month-1) rates occur at S = ST, while two power functions (parameters POW and GPOW) determine these rates at moisture levels below saturation (i.e. S < ST). Recharge is routed through a groundwater storage function that accounts for riparian evapotranspiration losses, drainage to other sub-catchments and contributions to baseflow. The model also has functions to simulate the implications of human impacts in managed basins.
Table 1 List of the parameters of the Pitman model including those of the reservoir water balance model (Hughes et al. 2006)
3 DEVELOPING LOCAL PARAMETER PRIORS
The approaches used to establish prior uncertainty distributions of model parameters are necessarily model-dependent and might also vary from region to region for the same model (Wagener and Wheater Citation2006, McCarthy Citation2007, Munoz-Carpena et al. Citation2007). There is thus huge flexibility in the development of local parameter priors, using anything from user experience regarding the feasible range of a uniform distribution to more formal equations to estimate moments of statistical distributions through parameter regionalization or other strategies (e.g. Hosking and Wallis Citation1997). Marginal probability distribution functions (pdfs) of common environmental properties can be estimated from the scientific literature, physical bounds, surveys, expert judgement and experiments (Saltelli et al. 2008). In this study, the expected parameter values are determined directly from basin physical data, such as topography, soil texture and depth, geology and vegetation cover using the procedure described in Kapangaziwiri (Citation2011) and Kapangaziwiri and Hughes (Citation2008) for the Pitman model.
In South Africa, the Agricultural Geo-referenced Information System (AGIS Citation2007) database provides the most detailed information of physical basin properties. shows a summary of the information typically available in the database that was used in the study. While information on soil hydraulic properties is not explicitly given in the database, it is assumed that these can be inferred from the texture class. The soil texture type information is therefore used to define and quantify some basic soil property values, such as infiltration parameters, porosity and permeability, based on the literature containing typical values (for example Rawls et al. Citation1982, Schulze et al. Citation1985).
Table 2 The information typically included in the South Africa AGIS land type database (AGIS Citation2007)
Physical basin property data cannot be interpreted directly as parameter values, mainly because of the differences in scale between the data and model (Beven Citation1989). The determination of the prior parameter distributions is therefore based on the assumption of some uncertainty in the available estimates of the physical basin characteristics and how these are interpreted at the sub-basin scale. If the distribution properties of the input physical property data can be established, then it is possible to describe the distribution characteristics of each model parameter. Our method uses the spatial variability in the input basin property data to define the uncertainty in these data, and this uncertainty is propagated through the parameter estimation equations (Kapangaziwiri and Hughes Citation2008, Kapangaziwiri Citation2011) into the distribution properties of the parameters at the model scale (). This is achieved through the upscaling of the input data to secondary variables, which are their basin-scale equivalents (). While a number of distribution types for most environmental variables exist (Munoz-Carpena et al. Citation2007), it is generally accepted that the means and variances of the input parameters exert more influence on the output uncertainty than the form of the distribution (Haan et al. Citation1998). In this study, a normal probability distribution was assumed to adequately describe the characteristics of the basin physical characteristics. In basins within the broader southern Africa region, where data-availability and -quality problems are acute, uniform distributions could be used. Simple Monte Carlo sampling of the prior parameter distributions is used to generate a large number of parameter sets assuming independence between the parameters.
4 DEVELOPING REGIONAL CONSTRAINTS
A hydrological signature (or constraint) can be defined as an index of the time series of a basin's dynamic response characteristic (e.g. runoff coefficient) that reflects the basin's functional behaviour (Wagener et al. Citation2007, Yadav et al. Citation2007, Gupta et al. Citation2008, Zhang et al. Citation2008, Bulygina et al. Citation2009, 2011). Appropriate constraints can be regionalized using simple regression relationships, whose prediction limits can be used to define the uncertainty in expected “behaviour”, as model diagnostic tools and to constrain and condition continuous flow simulations at both gauged and ungauged sites. Constraints should be selected to be indicative of how a given catchment differs from others, and examples include common descriptors of hydrograph shape such as runoff ratio, slope of the recession curve and time to peak flow (Shamir et al. Citation2005). The major advantage in the use of constraints is that they are model independent and force the model predictions to be hydrologically realistic in both gauged and ungauged sub-basins (Wagener and Montanari Citation2011). The objective is to achieve a progressive reduction in predictive uncertainty by constraining model output using expected basin behaviour, while maintaining reliable and consistent predictions (Yadav et al. Citation2007). Reliable results therefore require a sufficient number of constraints to encompass the full hydrograph in a multiple-objective assessment (Boyle et al. Citation2003). The important considerations when selecting constraints include:
a. | Data availability and quality: The construction of constraints must be based on reliable information about observed natural hydrological system response. The available historical records in southern Africa are often impacted and require pre-processing to naturalize the observed streamflow. | ||||
b. | Hydrologic relevance: Constraint indices must be hydrologically relevant and measure some aspect of hydrological function corresponding to one or more model components. | ||||
c. | Suitable predictors: The development of constraint relationships is dependent on the availability of suitable basin physical and/or climatic predictors for regionalization. The uncertainty in these relationships will depend on how good these predictors are and 90% prediction limits are employed in this study to define the regional constraint uncertainty. |
The three constraints developed for this study are mean annual runoff ratio, central slope of the monthly flow duration curve and mean annual groundwater recharge (Kapangaziwiri et al. Citation2009, Kapangaziwiri Citation2010). Additional constraints could be developed, but will always be limited by the availability of information.
4.1 The mean annual runoff ratio (Q/P)
This constraint describes the overall water balance of a sub-basin. Budyko (Citation1974) showed that a measure of aridity (P/PE) can be used to predict expected runoff (Q/P), where Q, P and PE are long-term averaged values of runoff, rainfall and potential evapotranspiration, respectively. We use 70 years of simulated runoff data (Midgley et al. Citation1994) that cover the whole of South Africa, to identify five regions with similar response characteristics and to establish initial relationships between P/PE and Q/P in each region. Simulated streamflow was used because available observations are often highly influenced by human intervention. Simulated naturalized flows therefore provide the only data with a national coverage, which allows regionalization at the required scale. Without this approach it would be difficult to include ungauged basins. The second step was to allocate all of the gauging stations for which naturalized observed data are available to the five regions ((b)) and to generate the final regression relationships ( and (a)) and 90% prediction limits. Gauging stations were rejected if they had less than 10 years of observations, if their drainage areas included quaternary catchments from more than one region, or if the amount of missing (and in-filled) data was excessive, i.e. greater than 20% of the months. Some very small (<10 km2) gauged sub-basins were also rejected as they are usually dominated by hillslope processes and exhibit more marked heterogeneity, and were therefore not expected to be representative of quaternary catchment-scale responses (Shaman et al. Citation2004). This process reduced the number of gauges to 270 across the whole country, and the spatial coverage was such that some regions are better represented than others, which might impact on the robustness of the relationships for some parts of the country.
Table 3 Details of the regional Budyko type relationships based on simulated and naturalized observed data. Equations are of the form ln(Q/P) = a × ln(P/PE) + b
Fig. 4 Hydrological metrics used to constrain model output ensembles. The regional relationships between (a) Q/P and P/PE based on observed flows, and (b) the resultant regions. (c) Slope of FDC relationship with aridity and relief, including the 90% prediction limits. (d) Range of groundwater recharge estimates between the lowest and the middle values (grey) and between the middle and the highest values (black).
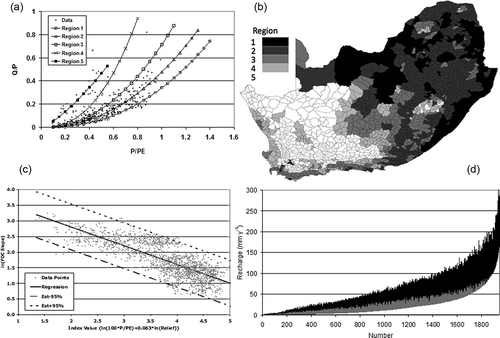
The final equations are very similar to the initial equations (based on simulated data) for regions 1 to 3, but there are quite large differences for regions 4 and 5 (). Possible explanations are that there are fewer streamflow gauges available for these two regions (only 23 and 27, respectively) to develop regression equations with reasonable confidence, that the initial equations are inappropriate, or that there are problems with some of the naturalized flows in these regions. It is also possible that some of the sub-basins that have been included in these regions based on simulated flows should really be allocated to other regions as a result of simulation errors or uncertainties related to poor representation of rainfall in these largely dry areas. The estimated values of P and PE (based on regionalized evaporation pan data; Midgley et al. Citation1994) are also subject to largely unknown estimation errors, which could further contribute to the overall uncertainty in the relationships. These effects will not be evident when using simulated data, as the flow simulations are driven by the same P and PE data. However, these errors will affect the relationships when using naturalized streamflow data. The final regional relationships based on observed data are illustrated in (a) and (b), and are generally consistent with expectations of the variations in regional hydrological behaviour (as controlled by climate and physical basin properties).
4.2 Slope of the mean annual flow duration curve (FDC) for monthly streamflow volumes
The slope of the flow duration curve (FDC) is a measure of the variability of flows and is a useful indicator of hydrological response characteristics in a region such as southern Africa with very diverse flow regime attributes (Smakhtin Citation2000). It may also be used for determining potential levels of sustainable abstraction, identifying the need for artificial storage and for determining environmental flow requirements (Hughes and Hannart Citation2003). For largely perennial river systems, the FDC slope values were defined as:
where Q10 and Q90 are the 10th and 90th percentiles, respectively, of the cumulative frequency distribution of flows. For those sub-basins with periods of zero flow, the Q90 value was replaced with the first non-zero FDC percentile and the difference in flows divided by the appropriate % difference.
After several attempts with various combinations of readily available predictor variables expected to influence FDC shapes, it was evident that there were no obvious regional patterns. (c) illustrates the best current solution. The estimation equation is based on all sub-basin simulated flows using a predictor variable that combines a measure of aridity (P/PE) and a measure of sub-basin slope (relative relief). The equation used is given by:
and “relative relief” is estimated from the highest and lowest points in the sub-basin using a 90-m gridded digital elevation data set (http://csi.cgiar.org). The analysis excluded a number of sub-basins in the country that are strongly influenced by dolomitic geology, and a region in the northeast of South Africa that appears to be anomalous based on the simulated flow data, reducing the number of data points from 1946 to 1911. The two scaling factors (100 and 0.063) were established manually by trial and error (no automatic optimization was attempted) before generating the regression equation with an R 2 value of 0.63. The analysis was based on monthly flow volumes, but it is unlikely that using daily data would have affected the results, as FDC slopes within the range of 90 to 10% are expected to be very similar for daily and monthly data (Smakhtin Citation2000).
4.3 Groundwater recharge
During a revision of the original a priori parameter estimation procedures developed by Kapangaziwiri and Hughes (Citation2008), an attempt was made to estimate the main groundwater recharge parameters in the Pitman model (GW and GPOW) using estimates of mean annual recharge (mm year-1) and an indication of average soil moisture status. Initial tests showed that the estimation equation could not be used with a great deal of confidence. It was therefore considered necessary to constrain the ensembles using available groundwater recharge estimates from a previous groundwater resource assessment project (GRAII, DWAF Citation2005). The GRAII project provides three different estimates of regionalized groundwater recharge for all sub-basins in South Africa, all being based on derivatives of the Chloride Mass Balance (CMB) method, which assumes that drainage (i.e. recharge) is inversely proportional to the chloride content of pore water. The three calculated values are based on output from GIS-calibrated layers, or values calibrated against numerous unpublished results of past small-scale groundwater resources estimation studies. (d) illustrates the ranges of the three different recharge estimates given in the GRAII database (DWAF 2005) for all 1946 sub-basins. The difference between even the two lowest estimates can be as high as 30 mm year-1. Experience with using these three values in water resources estimation suggests that the two lower values produce more reasonable and consistent results compared to the largest values (Kapangaziwiri Citation2010). Initially, the full range was used in the parameter estimation process to illustrate the full range of uncertainty in the GW parameter as it represents the current state of knowledge in the country. However, as this approach appeared to result in excessively large uncertainties in the outputs, the GW parameter uncertainty was then constrained using only the two lower values from the GRAII database (see Section 5.4).
5 APPLICATION OF THE FRAMEWORK
The framework has been applied in 46 test sub-basins (both gauged and ungauged) in South Africa. depicts the possible types of outcome when comparing local (a priori) and regional constraints (A to E). shows the frequency of occurrence of the possible types of outcome (A to E) for the three constraints. The groundwater recharge results are based on outputs before the GW parameter uncertainty was constrained. Outcome type A describes the case where the a priori model output uncertainty is less than the uncertainty in the regional constraints and all ensembles are within the constraint boundaries. This implies that the a priori parameter estimation procedure has produced outputs that are consistent with measured and expected hydrological response and can be considered behavioural (Beven and Binley Citation1992, Beven and Freer Citation2001). However, if the prediction limits of the regionalized indices are quite wide, there would remain a high degree of uncertainty in the definition of behavioural response. In outcome type B the range of model ensembles lies beyond both limits and the constraint boundaries can be used to determine the non-behavioural ensembles that will be rejected. Outcome types C and D refer to situations where the a priori parameter estimation process has produced model results that go beyond the upper and lower limits of the regionalized constraints, respectively. Extreme examples of C and D occur when none of the outputs lie within the constraint limits (outcome type E). Outcome type E can be either below or above the constraint boundaries. This type of outcome is important, as it illustrates an inconsistency in the two sources of information. While neither the local nor the regional approach represents the “truth”, their intersection () represents an area of consistency and agreement between the model and regional constraints, and suggests that the predictions can be used to represent the hydrology of the basin.
Table 4 Percentage frequency of occurrence of possible outcome types A to E in the comparison of the model output ensembles with the constraints
Fig. 5 Possible outcome types (A to E) from an analysis of uncertainty in the parameter estimation process against the uncertainty related to the constraints (* is the intersection between the parameter priors and the regional constraints).
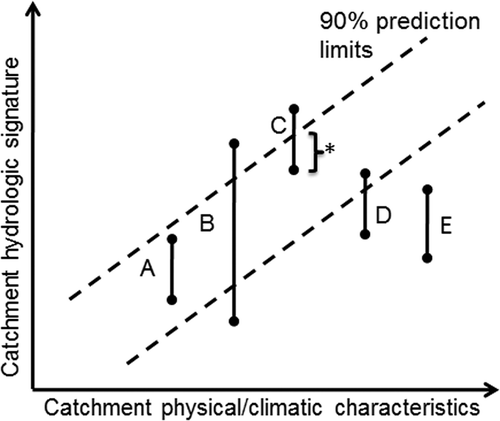
In our results, there are fewer outcome type C and D results for the runoff ratio constraint than for the FDC slope constraint. These types of outcome may indicate an inconsistency between the model and the constraints, and suggests a re-investigation of both the parameter estimation and constraint development processes is needed. There are many possible reasons for the observed inconsistency, including input and output data uncertainty, problems in the regionalization of constraints (e.g. lack of suitable regional predictors), problems in the a priori parameter estimation, or model structural issues with the Pitman model.
5.1 Ensemble analysis using the runoff ratio constraint
Figure 6 illustrates the use of the runoff ratio constraint. The results are generally acceptable with model output ensembles frequently within the ranges of the constraint uncertainty. Twenty-four percent (24%) of the basins produced excessive and unacceptable parameter uncertainty compared to the regional constraints (). There was only one sub-basin where both limits were exceeded (outcome type B). Outcome types C and D included those ensembles whose ranges of variability were narrower than the type B outcome, but included outputs that extended either above or below the constraint limit. The ensemble ranges tended to extend beyond the upper constraint limit rather than the lower one, indicating an over-simulation of the mean volume and a possible systematic error in one or more components of the parameter estimation procedure compared to the regionalized estimates.
Initial investigations based on sensitivity analysis indicate that the interflow, recharge and surface infiltration parameters (FT, GW and ZMIN, respectively) may be causing this problem due to their high a priori variability. The range of variability exhibited by some of the ensembles, even where the ensembles are within the constraint limits, is quite large. In most of these cases, there was substantial variation in the topography and/or the land types, which may have led to misinterpretation of the basin physical characteristics. Since the parameter uncertainty estimates are based on spatial variation in the basin physical attributes, it is inevitable that these sub-basins will have higher levels of uncertainty.
In spite of the problems referred to above, there are many sub-basins where the ensemble results are either within the range of regional constraints (outcome type A, 59%) or only slightly outside this range (outcome types C and D, 17%). The parameter estimation procedures were relatively successful for the example sub-basins, where success is measured against the regional runoff-ratio metric. In the majority of the remaining sub-basins (24%) where the parameter uncertainty is large, most of the ensembles are within the regional constraint values, suggesting that the focus of any improvement in the parameter estimation process should be on the outlier parameter sets. In examining the results in , if many of the observed streamflow data points are located closer to the median of the output ensembles (as is the case with the gauged basins in this study), this would suggest that it is the variance of the parameter uncertainty distributions rather than the mean (or best estimate) values that would require further investigation.
Fig. 6 Results of the comparison of the uncertainty between the runoff ratio constraint and the parameter estimation for the basins in regions 1–5. The observed data points are plotted for comparison with range of simulations, while the upper and lower 90% bounds are 95th and 5th prediction limits of the regression equations of the constraints.
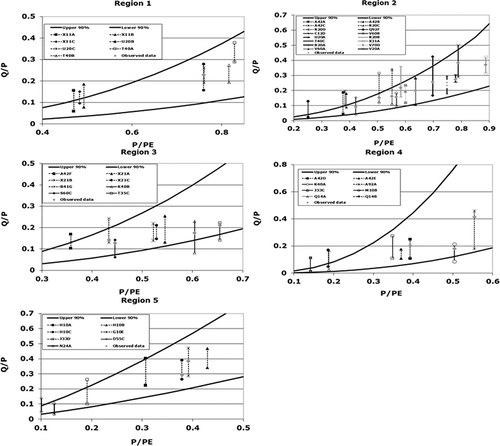
Overall, the parameter estimation process seems to produce fewer uncertain ensembles than the regional constraints, which is possibly a reflection of the limited amount of observed data used to develop the regional constraints, and suggests that a reasonable degree of confidence can be expressed in the parameter estimation approaches.
5.2 Ensemble analysis using the FDC slope constraint
shows the comparison between the a priori ensembles and the FDC slopes for the test basins. shows the distribution of the sub-basins with respect to the five outcome categories. The general tendency is for ensemble FDC slopes to be lower than the constraint boundary (outcome type D rather than C), suggesting that the a priori ensembles are generating excessive low flows compared to the constraints. The observed data points are also generally near the top of, or above, the ensembles. The sub-basins that have some non-behavioural outputs based on the runoff ratio constraint were also problematic with respect to the FDC slope criterion. One possible explanation for the generation of the low FDC gradients is that there is a high degree of uncertainty in the values of the main runoff generation model parameters (e.g. ZMIN, ZMAX, FT and POW). The greater the difference between the two infiltration parameters (ZMIN and ZMAX), the greater the amount of rainfall absorbed by the model, leading to lower runoff rates. Large values of FT and small values of POW also result in lower FDC slopes. As a result of the large variability in these parameters, it is possible that the scenarios described above may occur quite frequently.
Fig. 7 Results of the comparison of the uncertainty between the FDC constraint and the parameter estimation for the test basins.
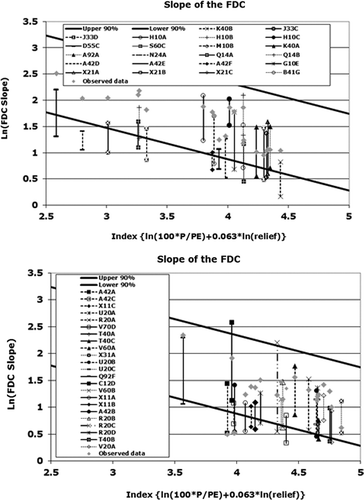
There appears to be a complete failure in the case of one sub-basin (N24A), whose outputs all lay outside the lower constraint boundary (outcome type E). This was surprising given that the monthly runoff volumes simulated for the basin have low uncertainty with only a very small proportion of the outputs lying beyond the lower boundary of the runoff ratio constraint. The sub-basin is only covered by one land type, which means there is little variation in the basin physical property data, minimizing the chances of incorrect interpretation. Sub-basin N24A is an arid basin and has a high proportion of zero flows, while, as clearly shows, excessive recharge is not to blame, suggesting that other sources of uncertainty may be more important than parameter uncertainty. The other dry sub-basins in the study exhibited a similar trend, though not as severe. The general trend was towards lower FDC slopes even in situations where the simulated runoff ratios were behavioural. This result suggests that the FDC slope calculation, and possibly the regionalization of the constraint, should be re-examined, particularly in dry catchments.
A detailed examination of the ensembles reveals that in many of the sub-basins there are instances where non-zero values for the 90% percentile of the simulated FDC are related to some extreme parameter FT values that are unlikely to be behavioural, leading to lower FDC slopes than can be considered realistic. Another possible explanation is that the model is generally poorly simulating high flows. The FDC slope calculation is quite sensitive to the number of zero-flow months and an additional constraint may be required for arid and semi-arid sub-basins where streamflow is intermittent. One of the main problems could be over-estimation of recharge, even if it is constrained by the GRAII values. If the mid value of GRAII is too high then the baseflows might still be excessive.
5.3 Ensemble analysis using the groundwater recharge constraint
Figure 8 shows the comparisons between the three GRAII (DWAF 2005) groundwater recharge estimates and the range of the ensemble outputs for the test basins before the recharge parameter GW was conditioned using only the two lower GRAII recharge values. Fifty-four percent (54%) of the minimum values of the simulated output ensembles are lower than the lowest GRAII estimates of recharge, and 48% of the maximum simulated output ensembles are higher than the maximum recharge estimates given in GRAII. For 10 sub-basins, all output ensembles fall within the GRAII range and can therefore be considered completely behavioural. The highest simulated annual groundwater recharge values were 221 mm (X21C) and 220 mm (T40A) and the lowest was zero for sub-basins that are all in the drier regions of the country.
One of the issues that the study had to take into account is the appropriateness of the three GRAII recharge estimates (DWAF 2005). Firstly, the GRAII values are based on different methods, and the extent to which these can represent uncertainty in the real recharge depends on the validity of the methods used. The current paucity of observed data on recharge makes this difficult. Secondly, experience using the GRAII data suggests that the highest recharge values are not appropriate in many situations. Detailed analysis of model outputs in several sub-basins suggests that such high recharge values result in excessive low flows compared to observed streamflow data (Hughes and Parsons 2005). However, in spite of the potential problems with the high GRAII recharge estimates, the values have been retained in this study as they can be considered to represent the extreme upper limit of recharge and represent the current state of knowledge in the country.
5.4 Exploring parameter sensitivity and feedback mechanisms
One objective of the framework is to identify which parameters are the most uncertain in specific sub-basins, and provide feedback to improve the a priori parameter estimation equations. This step is invaluable for learning about the model, for testing our understanding of process representations within the model, and for investigating both conceptual and structural consistency of the model. If conceptual expectations are at odds with the model outputs, it may be necessary to revisit them for a possible explanation. It is also possible to increase model parsimony by assigning fixed values to insensitive parameters and, hence, have a model that is transferred to ungauged basins more easily (Wagener et al. 2001). The use of the constraints to assess sensitivity is deemed necessary for determining the model's skill in simulating the different components of the hydrograph across regions.
Three sensitivity classes (high, moderate and low) were subjectively defined, based on an analysis of the degree of divergence of the normalized cumulative frequency distribution curves of the parameter values, based, in turn, on either traditional objective functions (for gauged basins) or constraint metrics (for gauged and ungauged basins). provides an example of the sensitivity diagrams. All of the ensembles are divided into five groups (each containing 20% of the ensembles) on the basis of the value of the sensitivity metric (objective function or constraint value). The top (80–100%) and bottom (0–20%) groups contain the 20% having the highest and lowest values of the sensitivity metric, respectively. The sensitivity divergence has been defined by difference between the top (P top) and bottom (P bot) groups at a cumulative frequency (of occurrence of parameter value) of 0.5 divided by the total range of the parameter value (P range):
Fig. 9 Illustration of the sensitivity analysis of the ensembles using a priori parameter estimates for parameter ZMIN for sub-basin R20C, showing the calculation of the sensitivity class of the parameters based on either traditional objective functions or constraint metrics.
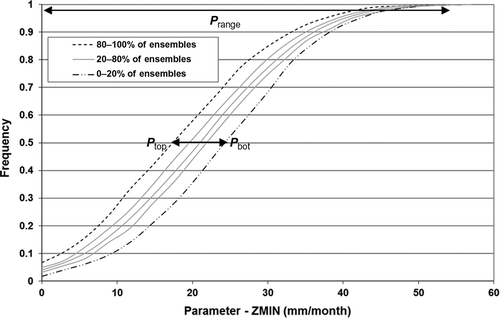
A parameter was deemed highly sensitive if its measure of divergence was greater than 0.25, moderately sensitive for a divergence 0.12–0.25 and less sensitive for a divergence below 0.12. Figure 10 shows the results of the sensitivity analysis for some of the sub-basins and for different assessment criteria (runoff ratio, FDC slope and mean monthly recharge constraints and four traditional objective functions). In general terms, the parameters identified as sensitive (i.e. high and moderate classes) are in agreement with conceptual expectations based on our knowledge of the physical hydrology of the basins.
The aim of the sensitivity analysis was not only to identify the important parameters, but also to test the use of the constraints in sensitivity analysis in gauged basins where traditional objective functions can be calculated. shows that the comparison between runoff ratio (Run Rat) and the % difference between the mean monthly runoff of the untransformed simulated and observed data (MMRE) identified the same sensitive parameters, an indication that the regional constraint indices can be used to identify sensitive parameters in ungauged sub-basins. However, direct comparison between all traditional objective functions and constraints is not possible, given that the criteria do not focus on the same components of the simulated time series. For example, CE focuses mainly on variance error, which none of the current constraints does, but if the two most sensitive classes (high and moderate) are considered together, the same group of parameters was identified by different assessment criteria. Some of the traditional objective functions provide more comprehensive assessments of model performance, while the constraints are restricted to specific aspects of hydrological response, and suggests that the constraints that have been included so far are not adequate for a full assessment of hydrological behaviour.
Fig. 10 Results of the sensitivity analysis for some of the selected sub-basins used in the study. Black indicates a critical (highly sensitive) parameter, grey is important (sensitive) and white is negligible (insensitive).
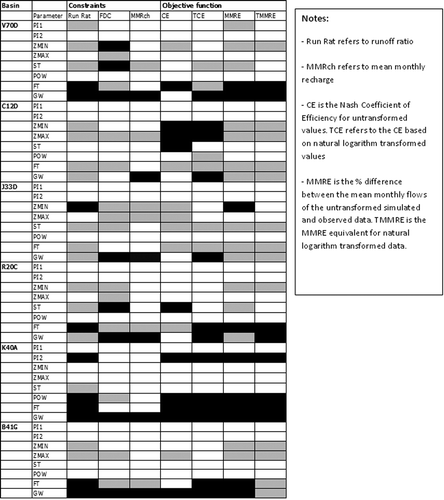
The groundwater recharge parameter (GW) is often identified as highly sensitive in our results, even in the semi-arid regions that are not expected to experience groundwater-related low flows. This result is related to problems with the estimation procedure for parameter GW, and efforts to improve the approach have met with little success. It could also be identified as sensitive because, in semi-arid areas, only very small (or zero) values for this parameter give a good fit and varying the parameter values away from zero has a big impact on the model result. For the estimation of uncertainty in GW, the full range of values of the GRAII database were initially used to condition the simulated mean monthly recharge, and this included the effects of some excessively high values in the GRAII database. The alternative was to manually adjust the GW parameter pdf (mean and standard deviation) to achieve an uncertainty range of the annual recharge that is within the constraint limits suggested by the two lower values in the GRAII data. This represents a calibration (feedback loop) of the GW parameter uncertainty using the regional constraints, and reduces the ranges of variation of both the simulated mean monthly flow () and the FDC slope (Fig. 11(b)) values and reduced bias relative to the constraints. This resulted in most of the sub-basins moving into the low parameter uncertainty category (outcome type A). For those sub-basins that continue to have ensemble outputs outside the constraint boundaries, other factors, such as scale differences between basin physical data and model and interpretation of land type data, may be more important in determining the final model outputs.
6 CONCLUSIONS
This study makes a contribution towards the explicit incorporation of uncertainty in water resources assessments in southern Africa and contributes to the practical use of PUB (Sivapalan et al. 2003). A framework for the incorporation and estimation of uncertainty is tested for basins across South Africa. The framework consists of an a priori parameter estimation approach linked to regional indices of catchment functional behaviour to further constrain the model outputs. A regional sensitivity analysis component provides a feedback loop from the constraint analysis to the a priori parameter estimation method. While this study has been confined to the use of the framework with the Pitman model, the components of the framework are robust enough to enable its use with any model structure—though the a priori method would have to be altered to accommodate different parameters.
This study has demonstrated the merits of the framework and that it is capable of being used in both gauged and ungauged basins. While its successful use outside South Africa will depend on data availability and quality, the integrated use of physically-based parameter estimation methods and hydrological constraints makes use of most of the available information, including physical basin data and regional observed flow response and groundwater recharge data. While neither the local nor the regional approach provides the actual “truth”, their conjunctive use should yield the largest reduction in uncertainty. However, comparing the results to actual observation (with their uncertainties) should help establish the “truth”, and a constraint that quantifies this comparison (e.g. frequency of predictions capturing observations) would be very useful. In this case, the regional constraints act as “limits of acceptability” in the manner of Blazkova and Beven (Citation2009), thus effectively constraining and conditioning the predictions. The framework offers a method of applying hydrological models in ungauged catchments that removes some of the known problems of existing approaches based on parameter regionalization (Wagener and Wheater Citation2006). The model outputs in the intersection between the model and the regional constraints () represent the behavioural outputs, which can be passed on to further water resources analyses.
Our results show that there is a lot of potential for the use of this uncertainty framework for South African basins. The use of physical basin attributes to directly estimate model parameters represents a new approach to regional application of the Pitman model and, except for the groundwater recharge parameter, has been generally successful in the situations tested. In data-scarce regions of the world, such as Africa, regional constraint relationships can be important surrogates for historical observed data and can be used for “calibrating” the behavioural range of model parameters. There are, however, uncertainties related to both the parameter estimation and constraint procedures, which need to be reduced if the full potential of the framework is to be realized. For example, there is large uncertainty in the FDC slope regionalization that needs to be reduced. The constraints used in this study target the total water balance, low flow and groundwater recharge components of simulated flows. While there is room to develop more constraints to address other components (e.g. baseflow indices as used by Yadav et al. Citation2007, or the shape of the recession curve as used by Winsemius et al. Citation2009), their selection should be determined by the objectives of the simulations, such as determining reservoir storage–yield relationships for medium to long-term water resources development planning. Using a suite of carefully chosen constraints, addressing most of the critical components of the streamflow hydrograph, will ensure that outputs accepted as behavioural would have passed more stringent tests of acceptability than can be offered by simple calibration. This should lead to greater confidence in the use of the outputs for any further analyses associated with water resources management decision-making. It is also important to recognize that regional constraint relationships will be uncertain in data-scarce areas and quantifying this uncertainty is an important part of the total framework.
While the three constraints used in this study are insufficient to cover all the components of basin response under the diverse hydro-climatic conditions in South Africa, they represent a good starting point. However, there is a need to address the question of how many constraints are necessary or appropriate. This is not easy to answer and, to a large extent, will depend on the quantity and quality of data available for the development of the constraints, as well as what the simulations will be used for. Constraining as many components of the simulated flow regime as possible in the manner of multi-criteria calibration suggested by Boyle et al. (Citation2003) would seem to be a primary objective. However, there is a need to avoid redundancy (Olden and Poff Citation2003) and to further quantify the level of uncertainty related to the constraints themselves (Kennard et al. Citation2009, Bulygina et al. Citation2011). The framework presented can make use of short records of observations (Seibert and Beven Citation2009) and other observed records of varying quality to reduce the constraint ranges in some basins. However, it is important that the uncertainties associated with these local data are quantified to avoid introducing bias into the framework. Some of the details of the framework will need further development to adequately address the use of observed data with uncertainty. This is an area of continuing research.
Currently, some of the constraints exhibit ranges that may be too wide, resulting in ensemble variability that is too large for effective use in further water resources systems analyses. In a management context, it is important that unambiguous guidance can be provided when uncertain model-generated data are to be used for decision-making. Given that many types of models are used in different parts of the southern African region, and that historical observed data are not always available, it is imperative that a common understanding and approach be developed for achieving the multiple water resources objectives of the region.
This study concentrated on the incorporation and analysis of parameter uncertainty into the Pitman model through a model-independent framework. It is acknowledged that many other sources of uncertainty may influence predictions in ungauged basins and this framework could be developed further to allow for the incorporation of these in the future. An obvious example is the inclusion of uncertainty in the rainfall data used to force the hydrological model. The explicit inclusion of uncertainty offers opportunities to identify where (geographically and in terms of data availability and quality) major sources of uncertainty exist and how these may be effectively reduced.
Acknowledgements
This project was funded by the Water Research Commission (WRC) of South Africa, Project no. K5/1838 and, in part, by the National Research Foundation (NRF). Thorsten Wagener was partially supported by an EPA STAR Early Grant (RD834196). The authors are grateful for this funding. The first author is now based at the Council for Scientific and Industrial Research (CSIR), South Africa.
REFERENCES
- AGIS, 2007. Agricultural Geo-Referenced Information System. http://www.agis.agric.za (http://www.agis.agric.za)
- Beven , K.J. 1989 . Changing ideas in hydrology—the case of physically-based models . Journal of Hydrology , 105 ( 1-2 ) : 157 – 172 .
- Beven , K. 2006 . A manifesto for the equifinality thesis . Journal of Hydrology , 320 : 18 – 36 .
- Beven , K.J. and Binley , A. 1992 . The future of distributed models: Model calibration and uncertainty prediction . Hydrological Processes , 6 : 279 – 298 .
- Beven , K.J. and Freer , J . 2001 . Equifinality, data assimilation, and uncertainty estimation in mechanistic modelling of complex environmental systems . Journal of Hydrology , 249 : 11 – 29 .
- Blazkova , S and Beven , K. 2009 . A limits of acceptability approach to model evaluation and uncertainty estimation in flood frequency estimation by continuous simulation: Skalka catchment, Czech Republic . Water Resources Research , 45 : W00B16 doi: 10.1029/2007WR006726
- Boyle , D. , Gupta , H.V. and Sorooshian , S. 2003 . “ Multicriteria calibration of hydrologic models ” . In Calibration of watershed models, Water Science and Application , Edited by: Duan , S. Vol. 6 , 185 – 196 . Washington , DC : AGU . doi: 10.1029/WS006
- Budyko , M.I. 1974 . Climate and life , New York : Academic Press .
- Bulygina , N. , McIntyre , N. and Wheater , H. 2009 . Conditioning rainfall–runoff model parameters for ungauged catchments and land management impacts analysis . Hydrology and Earth System Sciences , 13 : 893 – 904 .
- Bulygina , N. , McIntyre , N. and Wheater , H. 2011 . Bayesian conditioning of a rainfall–runoff model for predicting flows in ungauged catchments and under land use changes . Water Resources Research , 47 : W02503 doi: 10.1029/2010WR009240
- Demaria , E.M. , Nijssen , B. and Wagener , T. 2007 . Monte Carlo sensitivity analysis of land surface parameters using the Variable Infiltration Capacity model . Journal of Geophysical Research , 112 : D11113 doi: 10.1029/2006JD007534
- Di Baldassarre, , G. and Montanari, , A. 2009 . Uncertainty in river discharge observations: a quantitative analysis . Hydrology and Earth System Sciences , 13 : 913 – 921 .
- DWAF (Department of Water Affairs and Forestry) . 2005 . Groundwater Resource Assessment II , Pretoria , , South Africa : Department of Water Affairs and Forestry, GRAII .
- Freer , J. , Beven , K.J. and Ambroise , B. 1996 . Bayesian estimation of uncertainty in runoff prediction and the value of data: an application of the GLUE approach . Water Resources Research , 32 ( 7 ) : 2161 – 2173 .
- Gupta , H.V. , Wagener , T. and Liu , Y. 2008 . Reconciling theory with observations: elements of a diagnostic approach to model evaluation . Hydrological Processes , 22 : 3802 – 3813 .
- Haan , C.T. 1998 . Effect of parameter distributions on uncertainty analysis of hydrologic models . Transactions of the American Society of Agricultural Engineers , 41 ( 1 ) : 65 – 70 .
- Hartmann , G. and Bárdossy , A. 2005 . Investigation of the transferability of hydrological models and a method to improve model calibration . Advances in Geosciences , 5 : 83 – 87 .
- Hornberger , G.M. and Spear , R.C. 1981 . An approach to the preliminary-analysis of environmental systems . Journal of Environmental Management , 12 ( 1 ) : 7 – 18 .
- Hosking , J.R.M. and Wallis , J.R. 1997 . Regional frequency analysis: an approach based on L-moments , Cambridge , , UK : Cambridge University Press .
- Hughes , D.A. 2004 . Incorporating ground water recharge and discharge functions into an existing monthly rainfall–runoff model . Hydro. Sci. Journ. , 49 ( 2 ) : 297 – 311 .
- Hughes , D.A. and Hannart , P. 2003 . A desktop model used to provide an initial estimate of the ecological instream flow requirements of rivers in South Africa . Journal of Hydrology , 270 : 167 – 181 .
- Hughes , D.A. 2006 . Regional calibration of the Pitman model for the Okavango River . Journal of Hydrology , 331 : 30 – 42 .
- Kapangaziwiri, E., 2010. Regional application of the pitman monthly rainfall–runoff model in southern Africa incorporating uncertainty. Thesis (PhD), Rhodes University, Grahamstown, South Africa http://eprints.ru.ac.za/1777/ (http://eprints.ru.ac.za/1777/)
- Kapangaziwiri , E. 2011 . Physically-based parameter estimation methods for hydrological models: revised calibration and application procedures for the Pitman monthly rainfall runoff model in southern Africa , Berlin : Lambert Academic Publishing . ISBN: 978-3-8443-8737-7
- Kapangaziwiri , E. and Hughes , D.A. 2008 . Towards revised physically-based parameter estimation methods for the Pitman monthly rainfall–runoff model . Water SA , 32 ( 2 ) : 183 – 191 .
- Kapangaziwiri , E. , Hughes , D.A. Wagener , T. 2009 . “ Towards the development of a consistent uncertainty framework for hydrological predictions in South Africa ” . In New approaches to hydrological predictions in data-sparse regions , Edited by: Yilmaz , K. Vol. 333 , 83 – 94 . Wallingford , , UK : IAHS Press, IAHS Publ .
- Kavetski , D. , Kuczera , G. and Franks , S.W. 2006 . Bayesian analysis of input uncertainty in hydrological modelling: 1. Theory . Water Resources Research , 42 ( 3 ) : W03407 doi: 10.1029/2005WR004368
- Kennard , M.J. 2009 . Quantifying uncertainty in estimation of hydrologic metrics for ecohydrological studies . River Research and Applications , 25 ( 3 ) : 137 – 156 .
- Kuczera , G. 2006 . Towards a Bayesian total error analysis of conceptual rainfall–runoff models: characterising model error using storm-dependent parameters . Journal of Hydrology , 331 : 161 – 177 .
- McCarthy , M.A. 2007 . Bayesian methods for ecology , Cambridge , , UK : Cambridge University Press .
- McIntyre , N. 2005 . Ensemble prediction of runoff in ungauged watersheds . Water Resources Research , 41 : W12434 doi: 10.1029/2005 WR004289
- Merz , R. and Blöschl , G . 2004 . Regionalisation of catchment model parameters . Journal of Hydrology , 287 : 95 – 123 .
- Midgley , D.C. , Pitman , W.V. and Middleton , B.J. 1994 . Surface water resources of South Africa 1990, Volumes , Vol. I to IV , Pretoria , , South Africa : Water Research Commission . WRC Report no. 298/1.1/94 to 298/6.2/94
- Munoz-Carpena , R. , Zajac , Z. and Kuo , Y.M. 2007 . Global sensitivity and uncertainty analyses of the water quality model VFSMOD-W . Transactions of the American Society of Agricultural Engineers , 50 ( 5 ) : 1719 – 1732 .
- Olden , J.D. and Poff , N.L. 2003 . Redundancy and the choice of hydrologic indices for characterizing stream flow regimes . River Research and Applications , 19 : 101 – 121 .
- Pappenberger , F. and Beven , K. 2006 . Ignorance is bliss: or seven reasons not to use uncertainty analysis . Water Resources Research , 42 : W05302 doi: 10.1029/2005 WR004820
- Pitman , W.V. 1973 . A mathematical model for generating monthly river flows from meteorological data in South Africa , Johannesburg , , South Africa : Univ. of the Witwatersrand, Hydrological Research Unit, Report no. 2/73 .
- Rawls , W.J. , Brakensiek , D.L. and Saxton , K.E. 1982 . Estimations of soil water properties . Transactions of the American Society of Agricultural Engineers , 25 ( 5 ) : 1316 – 1320 .
- Reichert , P. and Borsuk , M.E. 2005 . Does high forecast uncertainty preclude effective decision support? . Environmental Modelling and Software , 20 ( 8 ) : 991 – 1001 .
- Rosegrant , M.W. and Perez , N.D. 1997 . Water resources development in Africa: a review and synthesis of issues, potentials, and strategies for the future . University of Minnesota: International Food Policy Research Institute, Department of Applied Economics, EPTD Discussion Paper 28. ,
- Saltelli , A. 2008 . Global sensitivity analysis: The primer , New York : John Wiley & Sons .
- Schulze , R.E. , Hutson , J.L. and Cass , A. 1985 . Hydrological characteristics and properties of soils in Southern Africa 2: Soil water retention models . Water SA , 11 ( 3 ) : 129 – 136 .
- Seibert , J. and Beven , K.J. 2009 . Gauging the ungauged basin: how many discharge measurements are needed? . Hydrology and Earth System Sciences , 13 ( 6 ) : 883 – 892 .
- Shaman , J. , Stieglitz , M. and Burns , D.A. 2004 . Are big basins just the sum of small catchments? . Hydrological Processes , 18 : 3195 – 3206 .
- Shamir , E. 2005 . Application of temporal stream flow descriptors in hydrologic model parameter estimation . Water Resources Research , 41 : W06021 doi: 10.1029/2004WR003409
- Singh , R. 2011 . A trading-space-for-time approach to probabilistic continuous streamflow predictions in a changing climate—accounting for changing watershed behavior . Hydrology and Earth System Sciences, , 15 : 3591 – 3603 .
- Sivapalan , M. 2003 . IAHS Decade on Prediction in Ungauged Basins (PUB), 2003–2012: shaping an exciting future for the hydrological sciences . Hydrological Sciences Journal , 48 ( 6 ) : 857 – 880 .
- Smakhtin , V.U. 2000 . Estimating daily flow duration curves from monthly stream flow data . Water SA , 26 ( 1 ) : 13 – 18 .
- Vrugt , J.A. 2003 . A Shuffled Complex Evolution Metropolis algorithm for optimization and uncertainty assessment of hydrologic model parameters . Water Resources Research , 39 ( 8 ) doi: 10.1029/2002WR001642
- Wagener , T. 2001 . A framework for development and application of hydrological models . Hydrology and Earth System Sciences , 5 ( 1 ) : 13 – 26 .
- Wagener , T. and Gupta , H.V. 2005 . Model identification for hydrological forecasting under uncertainty . Stochastic Environmental Research and Risk Assessment , 19 : 378 – 387 . doi: 10.1007/s00477-005-0006-5
- Wagener , T. and Montanari , A. 2011 . Convergence of approaches toward reducing uncertainty in predictions in ungauged basins . Water Resources Research , 47 : W06301 doi: 10.1029/2010WR009469
- Wagener , T. and Wheater , H.S. 2006 . Parameter estimation and regionalization for continuous rainfall–runoff models including uncertainty . Journal of Hydrology , 320 : 132 – 154 .
- Wagener , T. 2007 . Catchment classification and hydrologic similarity . Geography Compass , 1 : 901 – 931 .
- Wagener , T. , Wheater , H.S. and Gupta , H.V. 2004 . Rainfall–runoff modeling in gauged and ungauged catchments , London : Imperial College Press .
- Winsemius , H.C. 2009 . On the calibration of hydrological models in ungauged basins: a framework for integrating hard and soft hydrological information . Water Resources Research , 45 : W12422 doi: 10.1029/2009WR007706
- WWAP (World Water Assessment Programme) . 2009 . Chapter 13: Bridging the observational gap . Water in a changing world. UK: United Nations Educational, Scientific and Cultural Organisation, UNESCO Publishing, The United Nations World Water Development Report , 3 : 226 – 235 .
- Yadav , M. , Wagener , T. and Gupta , H.V. 2007 . Regionalisation of constraints on expected watershed response behaviour . Advances in Water Research , 30 : 1756 – 1774 .
- Zhang , Z. 2008 . Ensemble stream flow predictions in ungauged basins combining hydrologic indices regionalisation and multiobjective optimization . Water Resources Research , 44 doi: 10.1029/2008WR006833