Abstract
Droughts are an inevitable consequence of climate variability and are pervasive across many regions. Their effects can vary on an extensive scale, depending on the type of drought and people's vulnerability. Crucial characteristics of both hydrological (groundwater, streamflow) and meteorological (precipitation) droughts are related to their durations and severities, and these characteristics are typically correlated. While many studies have addressed the dependencies between these characteristics for either the meteorological or hydrological drought, the cross-dependence between meteorological and hydrological drought characteristics is barely investigated. The development of meteorological drought characteristics to hydrological drought characteristics is often hard to model and their connection is not definitively established. In order to better understand and explain this relationship, this study seeks to apply statistical tools and models. Drought characteristics data from areas in Europe with different climates are analysed. Two approaches of identifying related meteorological and hydrological drought are explored and compared. Classical linear correlation techniques do not provide promising results, indicating that any statistic of a hydrological drought is not a straightforward function of a preceding meteorological drought. Subsequently, the application of the concept of copulas to explore this dependence between meteorological and hydrological drought characteristics is investigated. The more comprehensive approach of copulas shows that the meteorological drought contains probability information of the successive hydrological drought.
Editor Z.W. Kundzewicz
Citation Wong, G., van Lanen, H.A.J., and Torfs, P.J.J.F., 2013. Probabilistic analysis of hydrological drought characteristics using meteorological drought. Hydrological Sciences Journal, 58 (2), 253–270.
Résumé
Les sécheresses sont une conséquence inévitable de la variabilité du climat et sont omniprésentes dans de nombreuses régions. Leurs effets peuvent énormément varier, en fonction du type de la sécheresse et de la vulnérabilité des populations. Les caractéristiques essentielles des sécheresses hydrologiques (eaux souterraines, débits) et météorologiques (précipitations) sont liées à leur durée et à leur sévérité, et ces caractéristiques sont généralement corrélées. Alors que de nombreuses études ont porté sur les dépendances entre ces caractéristiques soit pour les sécheresses météorologiques soit pour les sécheresses hydrologiques, la dépendance croisée entre les caractéristiques des sécheresses météorologiques et hydrologiques a à peine été abordée. Le passage des caractéristiques des sécheresses météorologiques à celles des sécheresses hydrologiques est souvent difficile à modéliser et leur relation n'est pas définitivement établie. Afin de mieux comprendre et expliquer cette relation, cette étude vise à appliquer des outils statistiques et des modèles. Des données des caractéristiques de sécheresses provenant de régions européennes de climats différents ont été analysées. Deux approches d'identification des sécheresses météorologiques et des sécheresses hydrologiques qui leur sont associées ont été étudiées et comparées. Les techniques classiques de corrélation linéaire n'ont pas donné de résultats prometteurs, ce qui indique que toute statistique d'une sécheresse hydrologique n'est pas une fonction simple de la sécheresse météorologique qui l'a précédée. Par la suite, nous avons étudié l'application du concept de copule pour explorer la dépendance entre lescaractéristiques des sécheresses météorologiques et hydrologiques. L'approche plus globale des copules a montré qu'il y a dans la sécheresse météorologique des informations de nature probabiliste concernant la sécheresse hydrologique consécutive.
Citation Wong, G., van Lanen, H.A.J., and Torfs, P.J.J.F., 2013. Probabilistic analysis of hydrological drought characteristics using meteorological drought. Hydrological Sciences Journal, 58 (2), 1–18.
INTRODUCTION
A drought is a sustained and regionally extensive occurrence of below-normal, natural water availability due to climate variability (Wilhite Citation2000, Tallaksen and van Lanen Citation2004). Droughts occur everywhere and can develop over rather short periods of months, or much longer periods of several seasons (multi-year droughts). Drought affects all components of the hydrological cycle, resulting in, for example, low soil moisture, declines in groundwater levels and reductions in spring and river flows. Drought may refer to meteorological drought (precipitation), soil water drought, or hydrological drought (groundwater, streams and lakes) (Tallaksen and van Lanen Citation2004, EEA 2009).
Droughts regularly lead to large socio-economic and environmental impacts (EC Citation2007, EEA 2009, UNESCO Citation2009). Drought is one of the most severe natural hazards, and climate change makes it likely to become more frequent and extensive in many areas across the world in the 21st century (Bates et al. Citation2008). The severity of drought impacts requires targeted management with monitoring and forecasting as essential elements. Drought monitoring poses challenges, because, in many cases, drought develops gradually, making it difficult to identify. Hence, drought forecasting with adequate lead times (e.g. months, season) is even more challenging (van den Hurk and Jacobs Citation2009). Drought forecasting comprises two steps, namely providing probabilities on the spatio-temporal development of: (a) meteorological drought that covers large areas, e.g. parts of continents (Tallaksen and van Lanen Citation2004) and is driven by persistent weather circulation types (Fleig et al. Citation2011), and (b) hydrological drought that shows up as a patchy pattern (Hisdal et al. Citation2001, Stahl et al. Citation2010) within areas of ongoing or preceding meteorological droughts.
The study of each drought type (meteorological or hydrological), and its respective characteristics has been extensive (labelled (1) in ). To describe the probabilistic nature of drought, a substantial amount of research has been devoted to describing the relationship between drought characteristics for each drought type, as well as its spatio-temporal behaviour. A familiar approach to describing the relationships between drought characteristics is to use the multivariate joint distribution of these characteristics. Most such studies have focused on the relationship between drought duration and severity for meteorological and hydrological droughts separately (Shiau and Shen Citation2001, Kim et al. Citation2003, Tallaksen et al. Citation2004, Salas et al. Citation2005, Mishra et al. Citation2009, Serinaldi et al. Citation2009, Cancelliere and Salas Citation2010, Song and Singh Citation2010a, Citation2010b). For example, Shiau and Shen (Citation2001) formulated a methodology for frequency and risk analysis of hydrological droughts using streamflow, and obtained a theoretical derivation of the recurrence interval of hydrological droughts above a certain severity. Other statistical relationships, such as the nonparametric kernel estimator used by Kim et al. (Citation2003) and the alternative renewal process by Mishra et al. (Citation2009), have also been reported.
In recent years, the bivariate approach has been extended to the multivariate aspect to include more associated drought characteristics, using the concept of copulas. However, this was still limited to studying the characteristics within each drought type. Several studies have applied copula-based distributions to both streamflow and rainfall variables (Favre et al. Citation2004, Salvadori and De Michele Citation2004b, Zhang and Singh Citation2006, Citation2007). For instance, Shiau (Citation2006) employed the bivariate copula to model the joint distribution of meteorological drought duration and severity. Similarly, he used a copula to investigate hydrological droughts of the Yellow River in China (Shiau et al. Citation2007). Song and Singh (Citation2010a) extended this to a trivariate case by including the inter-arrival time as a third variable and used a Plackett copula. In the meteorological case, Wong et al. (Citation2010) investigated trivariate Gumbel-Hougaard copulas for drought characteristics in Australia, conditional on different climatic states. Other quantitative relationships between characteristics for one individual drought type were explained using severity–area–duration (SAD) curves over different time scales. Andreadis et al. (Citation2005) used SAD curves to investigate hydrological drought (runoff) over the conterminous USA, and Sheffield et al. (Citation2009) applied these to study the most extreme soil moisture droughts across the globe. This was similarly done by Soule (Citation1992) using data from three Palmer indices to examine patterns of drought frequency and duration based on multiple definitions of drought events in the contiguous USA.
So far, these studies have concentrated on describing the relationships between characteristics of individual drought types. Limited studies have attempted to explain the links (i.e. cross-dependencies) between drought characteristics of a meteorological and hydrological drought, and such attempts have been fairly rudimentary (labelled (2) and (3) in ).
Our understanding of these cross-dependencies is still limited because: (a) the time lag between meteorological and hydrological drought follows from the complexity of storage and response times in the soils and aquifers, and is certainly not constant; (b) not all meteorological drought develops into hydrological drought, or severe hydrological drought can develop from a series of preceding rather minor meteorological droughts (van Lanen and Tallaksen Citation2008); and (c) the pattern of hydrological drought characteristics is patchy due to the spatial distribution of soils and aquifers. Drought propagation is not well understood; deterministic approaches are proposed by Peters et al. (Citation2003) and Schaake et al. (Citation2006, Citation2007), but are not very successful yet in quantitatively describing the link between the two drought types. Therefore, in this paper, we investigate, as an alternative, statistical relationships between hydrological drought and the preceding meteorological ones. This is not straightforward, because the characteristics for each drought type occur at different times and do not have similar sample sizes (Tabrizi et al. Citation2010). Some early research by Santos et al. (Citation2001) made a comparison between computed hydrological (runoff) drought and meteorological drought. Also, Lloyd-Hughes and Saunders (Citation2002) analysed the correlation between the standardized precipitation index (SPI) for measuring meteorological drought and the Palmer drought severity index (PDSI) for soil water drought, and found that the SPI based on the one-year-ahead moving average corresponds closely to the PDSI. Wanders et al. (Citation2010) calculated the correlation between several drought indices, which showed that relationships between indices of different drought types are weak.
Another recent development was the use of the Wilcoxon-Mann-Whitney rank-sum test, which is a non-parametric method in which the null hypothesis assumes that the two samples are independent. This test was used to evaluate the relationships between corresponding drought types, based on ranked values (Tabrizi et al. Citation2010). This method overcame the issue of different sample sizes for different drought types. Using data from Iran, the SPI was used to identify meteorological droughts, while streamflow droughts were described by drought intensities, using the threshold level approach. For most of the raingauge and hydrometric stations, significant relationships were observed between time-based SPI values and streamflow drought intensities. For applicability reasons, comparisons and verification were confirmed against historical droughts (Tabrizi et al. Citation2010).
In this study, we seek to statistically forecast drought duration and severity, starting with meteorological droughts that propagate through the hydrological cycle into hydrological drought (Peters et al. Citation2003, Citation2006). If this transformation could be quantified, timely forecasting of hydrological drought would be possible for a known meteorological drought, and this is urgently needed for water resources management (Wilhite Citation2000, Tallaksen and van Lanen Citation2004, EC 2007). The aim of this paper is to present preliminary explorative statistics by providing and assessing the information gathered from hydrological drought statistics that can be drawn from those of the preceding meteorological drought (shown in as the relationships (2) and (3)).The study areas, their climatic properties and basic statistics are given in the next section, following which, a description of the model used to obtain the data of meteorological and hydrological drought characteristics is given. Two approaches are presented for identifying the prior meteorological drought, which can provide information of the impending hydrological drought. In the subsequent section, standard and classical correlation tools are employed for correlation analysis between the meteorological and hydrological drought catchments for two catchment areas. The concept of copulas is introduced to carry out further correlation analysis and this is compared to classical correlation techniques. Finally, the application of the copulas is demonstrated using the conditional probability distribution function (pdf) of a meteorological drought characteristic to explain the corresponding hydrological drought characteristic.
DROUGHT STUDY AREAS
Three study areas in Europe were selected, each of which reflects different climatic properties. The Nedožery study area is located in the upper part of the Nitra catchment, Prievidza district, in central Slovakia (). The area has a moderately warm, humid continental climate (Köppen-Geiger climate Dfb), with mean annual precipitation of 873 mm and mean annual air temperature of 7.6°C (van Loon et al. Citation2010). The Upper-Metuje study area is situated in the northeast corner of the Czech Republic, on the border with Poland (). The area also has a Dfb climate, but mean annual precipitation and temperature are lower (Rees et al. Citation2004, van Huijgevoort et al. Citation2010)—748 mm and 5.8°C, respectively. The third study area is Guadiana, located in central Spain (). The climate is classified as warm Mediterranean (Köppen-Geiger climate Csa); mean annual precipitation varies between 400 and 500 mm and the mean annual temperature is 14–15°C (Conan et al. Citation2003).
For each area, series of meteorological and hydrological drought characteristics: duration and deficit volume, were obtained. The meteorological drought data were derived from time series of daily precipitation using the variable threshold approach (Yevjevich Citation1967, Hisdal et al. Citation2004). The start of a drought event is indicated by the point in time when the precipitation falls below the given threshold, and the event continues until the threshold is exceeded again. The difference between drought termination and onset gives the duration of the drought event. The deficit volume is calculated by summing up the differences between actual precipitation and the threshold level over the drought event. A monthly threshold derived from the monthly duration curves was applied. The discrete monthly threshold values are smoothed by applying a centred moving average of 30 days (van Loon et al. Citation2010). To eliminate minor droughts a minimum duration of 3 days was used. Threshold values of 70, 80 and 90% were used (Hisdal et al. Citation2004, Fleig et al. Citation2006, Hisdal Citation2009), meaning that the variable is equalled or exceeded, for instance, for 70% of the time in the time series for a particular month. Due to the absence of a widely accepted standardized definition of a drought event, values between 70 and 90% were used as the drought thresholds. Since the three study catchments exhibit substantially different climatic conditions, it is expected that the hydrological drought responses to meteorological drought will also differ. To investigate whether a single joint threshold can provide sufficient predictive power across all study catchments, different combinations of hydrological and meteorological drought thresholds were examined.
Drought event-based data (duration and deficit volumes) were determined using the threshold level approach rather than the popular SPI, since, to our knowledge, there is currently no equivalent transformation index for characterizing the severity of a hydrological drought.
Hydrological drought data were taken from the time series of groundwater discharge into the stream using the variable threshold approach similar to precipitation. Groundwater discharge was simulated using a synthetic hydrological model, which combines a soil-water balance model and a conceptual groundwater model (van Lanen and Tallaksen Citation2008, van Lanen et al. Citation2012). The soil-water balance model subdivides the soil column into topsoil and subsoil to account for differences in water storage and availability, and assumes no capillary rise. The downward flux across the bottom of the soil (i.e. recharge) drives the groundwater model. The groundwater model is based on linear reservoir theory. The responsiveness of the reservoir, j, is characterized by the transmissivity and storativity of the groundwater body and the distance between the streams. A low value of j implies a quick response of the groundwater body to recharge, whereas high j represents slow response.
The soil-water balance model uses time series of daily precipitation and potential evapotranspiration as input. WATCH Forcing Data (Weedon et al. Citation2011) for the period 1958–2001 were used for the precipitation series and to calculate the potential evapotranspiration series according to the FAO Penman-Monteith approach (van Huijgevoort et al. Citation2010). In addition, soil moisture retention data and thickness of soil layers are required to compute soil-water availability. Soil with a light silty loam texture (soil water availability: 125 mm) was assumed to reflect a representative capacity. For the groundwater body, an intermediate responsiveness of j = 100 d was also chosen to be representative. Representative properties were selected instead of real soil and groundwater properties for the three study areas to enable inter-comparison of the impact of climate alone on the relationship between meteorological and hydrological droughts.
Based on this model, different numbers of meteorological and hydrological droughts were identified for each area. and display the basic statistics of the meteorological and hydrological drought durations and deficit volumes, respectively, identified for different thresholds, for the Nedožery, Metuje and Guadiana areas over the period 1958–2001.
Table 1 Basic statistics of hydrological and meteorological drought durations for different thresholds in all three study areas
Table 2 Basic statistics of hydrological and meteorological drought deficit volumes for different thresholds in all three study areas
In general, a larger number of meteorological droughts than hydrological droughts was identified for all areas, mainly due to the fact that not all meteorological droughts, which result from a shortage of precipitation, will eventually lead to a hydrological drought (). It is obvious that, as the drought threshold increases, the number of droughts identified decreases. Overall, the mean hydrological drought duration is longer than that of the meteorological drought across all thresholds, for all three areas. This is also noticeable for Nedožery and Metuje, when comparing the mean drought deficit volumes for both meteorological and hydrological droughts, where the hydrological droughts are often more severe ().
The analysis of the relationship between both drought types followed the basic principle that a preceding meteorological drought will, to some extent, explain the following hydrological drought. Theoretically, meteorological drought precedes or partly overlaps the hydrological drought. By identifying the appropriate prior meteorological drought, a similar length of data for each catchment can be guaranteed. In the next section we introduce two different approaches of clustering meteorological droughts that precede or overlap the hydrological drought.
CORRELATION ANALYSIS
Methodology
Two approaches of identifying prior meteorological droughts from hydrological droughts are considered: (a) a single event, and (b) a multiple-event approach with two different time windows (1 and 2 years).
The first method, referred to herein as the single-event approach, is straightforward and simply uses the characteristics of the immediately preceding meteorological drought to predict the characteristics of the subsequent hydrological drought.
The second method is the multiple-event approach, and is based on the theory (Peters et al. Citation2003, Citation2006, van Lanen et al. Citation2012) that a hydrological drought is dependent on the possibly long-term memory properties of an area that are associated with stores (e.g. aquifers, lakes) that can occur. Let m denote the current meteorological drought, and h the current hydrological drought of a specific area (), where Sm and Sh refer to any meteorological or hydrological drought characteristic, respectively. Then the previous meteorological droughts are denoted m, –1 and m, –2, with the characteristics Sm ,-1 and Sm ,-2, respectively (). For example, the duration of the hydrological drought h may be defined as Dh = t 11 – t 9 , while that of the meteorological drought is Dm = t 10 – t 8 ().
Based on hydrological assumptions, the hydrological drought h with duration Dh
is caused by a series of preceding meteorological droughts (m, 0), (m, –1) and (m, –2) … (m, –n) with durations Dm
(0), Dm
(–1), Dm
(–2), … , Dm
(–n), respectively. To take into account the influence of preceding meteorological droughts, as well as their respective recovery period (that is, the end of a meteorological drought and the start of a hydrological drought), weights (wi
) for each of these events are required for the calculation of the associated weighted meteorological drought duration ():
– Preceding droughts
– Partly overlapping droughts
Conceptually, the weights are the ratios of the meteorological drought durations to the recovery periods. For preceding droughts, the weights are kept at a maximum of 1 to prevent preceding droughts with short recovery periods having a larger influence than partly overlapping droughts.
Observe that from Equationequation (1)(1), as inter-drought duration increases, there is more time for recovery and, hence, less weight is being placed on preceding droughts. The associated weighted meteorological drought duration
is then defined as:
It also follows that the deficit volume of the current hydrological drought, is similarly dependent on the deficit volumes of preceding meteorological droughts, V
m
, Vm
(–1), Vm
(–2), … , Vm
(–n) . The associated weighted meteorological drought deficit volume
is calculated as:
ANALYSIS OF DATA FROM THE STUDY AREAS
Both approaches were compared for all three study areas for different thresholds. and show the correlations between the meteorological and hydrological drought durations and deficit volumes, respectively, for Nedožery, Metuje and Guadiana catchments, along with their associated p values.
Table 3 Correlations between meteorological and hydrological drought duration using both approaches for different thresholds (p values of the correlation are in brackets; correlation values significant at the 95% confidence level are highlighted in bold)
Table 4 Correlations between meteorological and hydrological drought deficit volume using both approaches for different thresholds (p values of the correlation are in brackets; correlation values significant at the 95% confidence level are highlighted in bold)
Single-event approach
For all three study areas, employing a different drought threshold results in a change in the correlations between the meteorological and hydrological drought characteristics, as well as the associated p values, due to different sample sizes in each case. In general, increasing the meteorological and/or hydrological drought thresholds for both single-event and multiple-event approaches led to a reduced data set, which may result in relatively high correlations not being statistically significant, due to a corresponding increase in uncertainty. This is particularly evident in the Guadiana catchment, where there is a stark contrast in the correlation of –0.07 with a p value of 0.72, and the much more significant correlation of 0.63, just by increasing the hydrological drought threshold (). This is also observed for the other meteorological drought thresholds in Guadiana, where keeping the meteorological drought threshold constant and increasing the hydrological drought threshold led to a less significant correlation. Correlations between meteorological and hydrological drought deficit volume () display similar behaviour. However, this pattern was not observed for the other study areas in the single-event approach.
Multiple-event approach
The multiple-event approach was applied to the study areas with moving windows of both 1 year and 2 years. Correlations from the multiple-event approach were also calculated for a moving time window of 2 years, as a comparison. The correlations of the drought characteristics for the multiple-event approach were evaluated for pairs of hydrological droughts and the associated weighted meteorological droughts as calculated using Equationequations (3)(3) and (4). The results are also shown in and .
As an example of the multiple event approach, correlations between meteorological and hydrological drought duration from Metuje study area () are analysed. For both 70% and 80% meteorological drought thresholds, the correlations become less significant as the hydrological drought threshold increases for the 1-year time window. However, there is no clear pattern for the 90% meteorological drought threshold in either the 1-year or the 2-year time window. Similarly, the significance of correlations for the other drought thresholds does not display any pattern when varying either the meteorological or the hydrological threshold.
Analysis of results
In general, different thresholds which define both meteorological and hydrological droughts have an influence on the correlations between the drought characteristics and their associated p values. Overall, for both single-event and multiple-event approaches, there is a good correspondence for the correlations of both drought durations and deficit volumes. That is, by comparing and , it is observed that, when the correlation between the meteorological and hydrological drought durations is high and statistically significant, the same is observed for the correlation between the corresponding meteorological and hydrological drought deficits. Their associated p values also match pretty well. Furthermore, the climate of each study area clearly defines and affects these correlations, as confirmed by van Lanen et al. (Citation2012), who showed that climate influences the 90% probability kernel density fields.
The single-event approach produces higher correlations between meteorological and hydrological characteristics for Nedožery and Guadiana areas, while stronger correlations are observed for Metuje when the multiple-event approach is applied. Increasing the length of the time window in the multiple-event approach increases the correlations between the characteristic and its statistical significance, particularly for Metuje. This is strong evidence that the catchment properties of Metuje catchment favour a longer memory response for hydrological drought. The opposite is observed for the Nedožery catchment, where the recent prior meteorological drought is sufficient to explain the current hydrological drought, using the single-event approach. In fact, the multiple-event approach did not improve the correlations for Nedožery.
Overall, stronger yet relatively low correlations for each study area are observed using the multiple-event approach, when the percentile threshold for meteorological drought is lower than or equal to the hydrological drought threshold. This indicates that there is no clear evident relationship between meteorological and hydrological drought events for all areas, and any statistic of a hydrological drought is not a straightforward function of a preceding meteorological drought, which, in turn, indicates that different drought percentile thresholds are needed for both types of drought. Moreover, these low correlations would result in poor linear regression predictions of hydrological drought, based on the preceding meteorological drought.
Based on the above observations, the strongest correlation between both meteorological and hydrological drought duration and deficit volumes was chosen to demonstrate the application of a copula. Here, the correlation coefficient has been used as a guide to the strength of the dependence between meteorological and hydrological drought characteristics. Since the data may not have a Gaussian distribution, this correlation coefficient may not fully describe the dependence structure. However, it is a straightforward means of selecting whether a single-event or multiple-event analysis is suitable for a particular catchment. Other dependency methods, such as conditional probability or mutual information criteria, would be equally valid for this purpose. In the following section, we give a brief description of the copula concept and illustrate its application.
FURTHER CORRELATION ANALYSIS USING COPULAS
Having observed that linear correlation techniques are not effective in measuring the dependency between meteorological and hydrological drought characteristics, we introduce copulas as an explorative tool for modelling a variety of dependence structures.
Copulas provide a general form for multivariate distributions, allowing arbitrary marginal distributions and a wide variety of correlation structures. Copulas were first introduced in 1959 by Sklar and have been used extensively in the area of finance and econometrics (Embrechets et al. Citation2003, Cherubini et al. Citation2004), while applications in hydrology are more recent (De Michele and Salvadori Citation2003, Favre et al. Citation2004, Salvadori and De Michele Citation2004a, 2004b, 2006, 2007, De Michele et al. Citation2005).
A 2-copula is the cumulative density function (cdf) of a bivariate distribution function with all the univariate marginal distributions being uniform on the interval [0,1], written U(0,1). It follows that a copula C is a mapping C: [0,1]2 → [0,1]. Sklar's Theorem (Sklar Citation1959, Nelsen Citation2006) states that, for a 2-dimensional cdf H with univariate margins F 1 and F 2, there exists a 2-copula, C, such that:
Gumbel-Hougaard copula
The Gumbel-Hougaard (GH) copula belongs to the Archimedean copula family. This family of copulas is a common choice for hydrological applications, because it includes multivariate extreme distributions which exhibit tail dependence, and has been found to give a reasonable empirical fit to field data (see, for example, Serinaldi and Grimaldi Citation2007). The Archimedean symmetric (one-parameter) copula is of the form:
The GH copula is uniquely defined by its generator φ i (t) = (–lnt)θi with θ i ≥ 1, and the bivariate version is:
The parameter θ i is estimated using the inference function for margins (IFM) method (Joe Citation1997). In the first of this two-step approach, maximum likelihood estimation (MLE) is applied to the univariate marginal distributions to obtain estimates of the parameters used for the chosen marginal distributions. The second step involves the estimation of θ i using MLE.
Marginal distributions of drought characteristic data
For illustration purposes, drought characteristic data derived from the single-event approach for Nedožery, along with the data obtained from the multiple-event approach with a 2-year time window for Metuje, were chosen. The scatter plots between the meteorological and hydrological characteristics are shown in (a) and (b) for Nedožery and (c) and (d) for Metuje. Their corresponding correlations are given in .
Table 5 Correlations between meteorological and hydrological drought characteristics
Fig. 4 Scatter plots of meteorological and hydrological drought durations and deficit volumes: (a) and (b) for Nedožery, and (c) and (d) for Metuje.
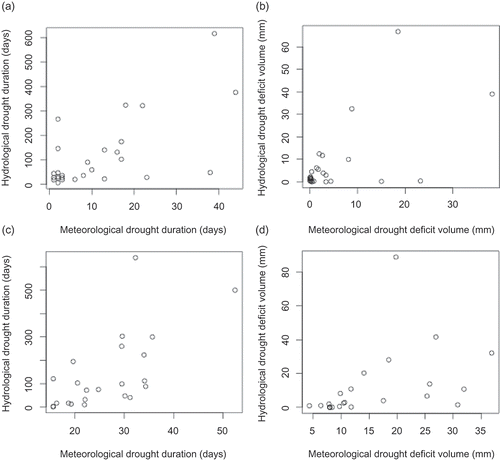
The advantage of using the copula approach is the ability to model nonlinear dependence structures, since this approach allows marginal distributions and the joint behaviour of variables to be modelled separately. For this reason, appropriate marginal distributions, such as normal, lognormal, Weibull and gamma distributions, were fitted and compared. These marginal distributions differ in the number of parameters and shape of the distribution. Hence, the Akaike information criterion (AIC) was used to assess the goodness of fit of the chosen distribution to the data set. The AIC is defined as AIC = 2k – 2ln(L), where k represents the number of parameters in the statistical model and L is the maximized value of the likelihood function for the estimated model. and show the fitting of the appropriate marginal distributions for each variable for Nedožery and Metuje, respectively, and the best-fit marginal distributions are given in .
Table 6 Marginal distributions fitted to each variable
Fig. 5 Nedožery area: appropriate marginal distributions fitted to (a) meteorological drought duration and (b) deficit volume; and (c) hydrological drought duration and (d) deficit volume.
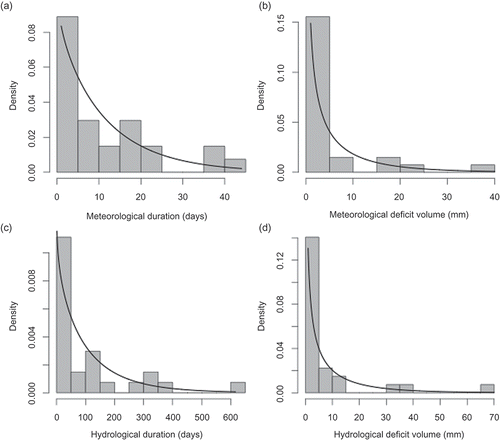
Fig. 6 Metuje area: appropriate marginal distributions fitted to (a) meteorological duration, and (b) deficit volume; and (c) hydrological drought duration, and (d) deficit volume.
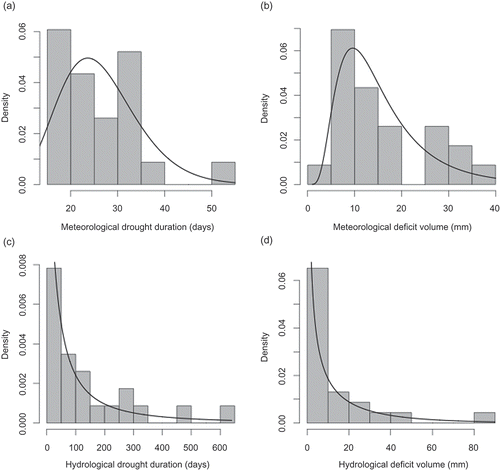
Following the fitting of respective marginal distributions, the drought variables were transformed to uniform variables ui = F(xi ), where i refers to the drought variable. The correlations between these transformed uniform variables are given in . The transformation to uniform variables has different effects in the two study areas. There is a slight decrease in correlations between meteorological and hydrological durations for Nedožery and a slight increase for Metuje. The difference between the correlations calculated for the uniform meteorological and hydrological deficit volumes are comparatively larger.
Table 7 Correlations of meteorological and hydrological drought characteristics after fitting respective marginal distributions
Fitting the bivariate Gumbel-Hougaard copula
The crucial step is to estimate the copula parameter θ
i
of the Gumbel-Hougaard copula (Equationequation (7)(7)) using MLE, which requires the cdf of the copula. This can be achieved by differentiating Equationequation (7)
(7) using a symbolic algebraic package. The copula parameter was estimated for each pair of meteorological and hydrological characteristics in the two study areas, and the results are given in . The estimated copula parameters between meteorological and hydrological drought durations and deficit volumes are denoted by θ
D
and θ
V
, respectively.
Table 8 Estimated copula parameters
and
between meteorological and hydrological drought durations and deficit volumes, respectively, for two study areas
The contour plots of the marginal bivariate copula density functions between pairs of meteorological and hydrological drought durations and deficit volumes for the Nedožery and Metuje areas are shown in The drought characteristics data derived from the synthetic model for both areas are superimposed on the contour plots as circles.
Fig. 7 Bivariate pdf contours constructed from the copula and observation points (circles) for: (a) and (b) Nedožery, and (c) and (d) Metuje. Contour lines represent 0.05 probability density increments, where the outermost line is 0.05 and dotted lines represent 0.001.
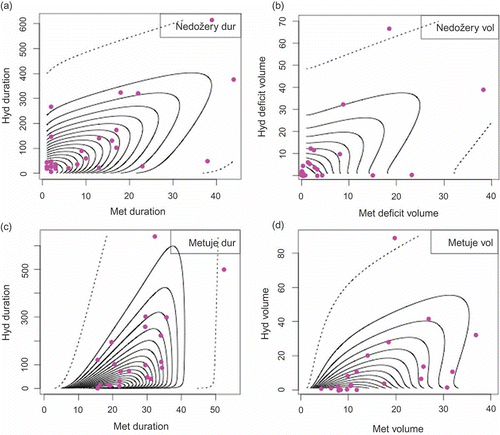
Overall, the copula model is able to capture most of the data derived from the synthetic model, though a few of the extreme points lie outside the probability line of 0.001. The effect of the estimated copula parameters and
on the shape of the contours is observed for both areas. The difference in contour shape can be attributed to the climate properties, which gives rise to the different relationship between meteorological and hydrological characteristics in each catchment.
DISCUSSION
Investigating cross-dependencies between meteorological and hydrological drought characteristics is essential for managing water resources under drought (Wilhite Citation2000, Citation2005, Tallaksen and van Lanen Citation2004, Iglesias et al. Citation2009) and provides valuable information on hydrological drought characteristics given prior meteorological drought characteristics. The proposed method to investigate these cross-dependencies differs from the few other attempts to connect these characteristics (e.g. Tabrizi et al. Citation2010) by using precipitation deficit volume instead of SPI. The SPI has a valuable alert function, but because of the normalization, the index does not directly provide a measure of depth, which after the alert, is essential for water management.
It is evident that the bivariate pdf () does not allow a reliable nonlinear forecast of the hydrological drought to be made. Nevertheless, shows that there is valuable information in the meteorological drought that allows probability statements about the subsequent hydrological drought to be made in terms of conditional pdf. (a) and (b) shows the corresponding conditional pdf of hydrological drought deficit volume for two different values of meteorological drought deficit volume.
Fig. 8 Conditional pdf of hydrological drought deficit volume given a meteorological drought deficit volume of 10 mm (–––) and 20 mm (- - - -) for: (a) Nedožery and (b) Metuje catchments.
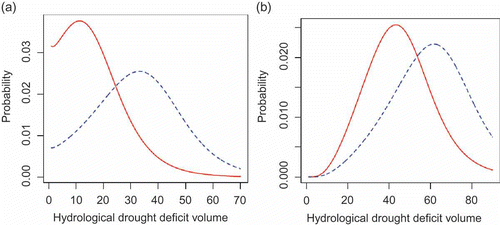
From , it is expected that, for a larger meteorological drought deficit volume, a larger hydrological drought deficit volume will be observed. Both parts of show a shift in the mode and a change in the spread of these distributions. Different conditional pdf behaviours are observed for both catchments and it is evident that the difference between the means (peaks) for Nedožery is larger than that for Metuje. This study has shown that, for different study catchments, there is a difference in the behaviour of the linkage between meteorological and hydrological drought characteristics, based on their distributions, as seen in (a) and (b). In order for appropriate analysis of hydrological drought characteristics to be made, it is essential that this relationship to meteorological drought characteristics is considered. This information will be important for future mitigation planning of hydrological drought.
(a) and (b) shows a comparison between the marginal distribution of hydrological drought deficit volume and the conditional distribution of a hydrological drought deficit volume for a meteorological drought deficit volume of 20 mm, for Nedožery and Metuje, respectively. The figure clearly shows an obvious difference in the probability distribution of the hydrological drought deficit volumes for each study catchment. Given the occurrence of an extreme meteorological drought event, the characteristics of the following hydrological drought are also likely to be extreme. There is a risk of underestimation of the associated hydrological drought characteristics if the marginal distributions are used instead of the conditional distribution in the case of an extreme meteorological drought event. This effect can be observed in both catchments, despite the correlations being 0.61 and 0.43 for Nedožery and Metuje catchments, respectively (). Even in cases where the joint distributions yield average correlations of 0.43, this conditional distribution is very different from considering solely the marginal distributions and hence should be considered.
Fig. 9 Comparison of marginal distribution of hydrological drought deficit volume (–––) and conditional distribution of hydrological drought deficit volume given a meteorological drought deficit volume of 20 mm (- - - -) for: (a) Nedožery and (b) Metuje catchments.
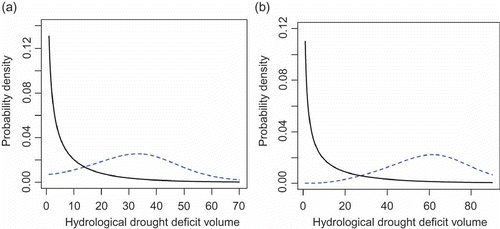
The proposed method was demonstrated for two Central European study areas. It could be further elaborated for other regions with different drought typology as recently proposed by van Loon and van Lanen (Citation2011). Sets of curves with conditional pdf of hydrological drought deficit volume given a certain meteorological drought deficit volume (cf. ) could be constructed for these regions and could form the basis of information provided to water resources management on what to expect in terms of the duration and deficit volume of an upcoming hydrological drought given the characteristics of the pre-existing meteorological drought. In addition to this forecasting purpose, the sets of curves, which basically reflect drought propagation, can also be useful for implementing more structural measures to reduce drought impacts.
CONCLUSIONS
Drought is a critical component in water resources planning and management, and understanding drought characteristics is an essential step to developing efficient drought adaptation policies and drought management plans. However, complex relationships between meteorological and hydrological drought characteristics have hindered the understanding of overall droughts in the past. This study examines these cross-dependencies between meteorological and hydrological drought characteristics and provides explorative statistics, which seek to improve and advance our understanding of this complicated relationship.
Two approaches (i.e. single-event and multi-event) were introduced to identify the prior meteorological drought associated with each hydrological drought, for the purpose of investigating the dependence structure between their corresponding characteristics. These approaches were applied to three study areas with varying drought thresholds. The results show that different thresholds affect the correlations between drought characteristics. Areas which have a slower responding subsurface system tend to favour the multiple-event approach, and give rise to stronger correlations between meteorological and hydrological characteristics. However, the linear correlation and predictions produced for both approaches were not promising. Ultimately, no combination of joint threshold proved to be highly correlated for all catchments. This may mean that there cannot be a general definition of joint threshold that will be applicable to all catchments, and that drought thresholds may need to be defined on a catchment-by-catchment basis.
The more sophisticated approach of copulas gave more favourable results, where it was shown to include probability information in the meteorological drought about the following hydrological drought. The contour plots in indicate that the fitted copulas provide a reasonable fit to the observed data with only one or two points lying beyond the 0.001 probability contour. Copulas can play an important role in providing an insight into the expected probability distribution of a hydrological drought characteristic, given that information of the prior meteorological drought characteristic is known.
While this study has demonstrated that the concept of copulas is a promising method in analysing multi-dimensional stochastic relationships between meteorological and hydrological characteristics, and that this concept provides some information, the relationship between these variables remains complicated and simple correlation methods are insufficient to describe the correlation structure and provide an efficient forecast. A possible solution to analysing the complicated drought propagation would be a hybrid approach combining deterministic models with sophisticated statistical techniques. Further work is required to improve our understanding of these statistical relationships together with deterministic approaches (van Loon and van Lanen Citation2011) within the whole drought framework.
Acknowledgements
This research was funded by the XEROCHORE (An Exercise to Assess Research Needs and Policy Choices in Areas of Drought, Grant No. 211837) project, under the European Union Seventh Framework Programme (EU-FP7). The study also contributes to the EU-FP7 Project DROUGHT-R&SPI (Fostering European Drought Research and Science-Policy Making, 282769) and the research programmes of the UNESCO-FRIEND-Water project group on Low Flows and Drought and the Wageningen Institute for Environment and Climate Research (WIMEK-SENSE). The authors would like to thank Niko Wanders and Anne van Loon from the Centre for Water and Climate, Wageningen University, for providing us with synthetic model simulations.
REFERENCES
- Andreadis , K.M. 2005 . Twentieth-century drought in the conterminous United States . Journal of Hydrometeorology , 6 : 985 – 1001 .
- Bates , B.C. 2008 . Climate change and water , Geneva : IPCC Secretariat, Technical Paper of the Intergovernmental Panel on Climate Change .
- Cancelliere , A. and Salas , J.D. 2010 . Drought probabilities and return periods for annual streamflow series . Journal of Hydrology , 391 : 77 – 89 .
- Cherubini , U. , Luciano , E. and Vecchiato , W. 2004 . Copula methods in finance , Chichester : John Wiley .
- Conan , C . 2003 . A long-term hydrological modelling of the Upper Guadiana river basin, Spain . Physics and Chemistry of the Earth , 28 : 193 – 200 .
- De Michele , C. and Salvadori , G. 2003 . A generalized Pareto intensity–duration model of storm rainfall exploiting 2-copulas . Journal of Geophysics Research , 108 ( D2 ) : 4067
- De Michele , C. 2005 . Bivariate statistical approach to check adequacy of dam spillway . Journal of Hydrologic Engineering , 10 ( 1 ) : 50 – 57 .
- EC (European Commission) . 2007 . Addressing the challenge of water scarcity and droughts in the European Union , Brussels : European Commission .
- EEA (European Environmental Agency) . 2010 . Mapping the impact of recent natural and technological disasters in Europe. An overview of the last decade , Copenhagen : EEA .
- Embrechets , P. , Lindskog , F. and McNeil , A.J. 2003 . “ Modelling dependence with copulas and applications to risk management. In ” . In Handbook of heavy tailed distributions in finance , Edited by: Rachev , S.T. 329 – 384 . 1st ed. Karlsruhe , , Germany : Elsevier .
- Favre , A.-C. 2004 . Multivariate hydrological frequency analysis using copulas . Water Resources Research , 40 : W01101
- Fleig , A.K. 2006 . A global evaluation of streamflow drought characteristics . Hydrology and Earth System Sciences , 10 : 535 – 552 .
- Fleig , A.K. 2011 . Regional hydrological drought in north-western Europe: linking a new Regional Drought Area Index with weather types . Hydrological Processes , 25 : 1163 – 1179 .
- Hisdal , H. 2009 . Streamflow deficit . World Meteorological Organisation Operational Hydrology Report 50 , 1029 : 71 – 76 .
- Hisdal , H. 2001 . Have droughts in Europe become more severe or frequent? . International Journal of Climatology , 21 : 317 – 333 .
- Hisdal , H. 2004 . “ Hydrological drought characteristics. In ” . In Hydrological drought processes and estimation methods for streamflow and groundwater , Edited by: Tallaksen , L.M. and van Lanen , H.A.J. 139 – 198 . Amsterdam : Elsevier Science BV .
- Iglesias , A. , Garrote , L. and Cancelliere , A. 2009 . Coping with drought risk in agriculture and water supply: drought management guidelines for the Mediterranean , 1st , Berlin : Springer-Verlag .
- ISDR (International Strategy for Disaster Reduction) . 2009 . Global assessment report on disaster risk reduction , Geneva : United Nations .
- Joe , H. 1997 . Multivariate models and dependence concepts , New York : Chapman and Hall .
- Kim , T , Valdes , J.B. and Yoo , C. 2003 . Nonparametric approach for estimating return periods of droughts in arid regions . Journal of Hydrologic Engineering , 8 : 237 – 246 .
- Lloyd-Hughes , B. and Saunders , M.A. 2002 . A drought climatology for Europe . International Journal of Climatology , 22 : 1571 – 1592 .
- Mishra , A.K. , Singh , V.P. and Desai , V.R. 2009 . Drought characterization: a probabilistic approach . Stochastic Environmental Research and Risk Assessment , 23 ( 1 ) : 41 – 55 .
- Nelsen , R. 2006 . An introduction to copulas , 2nd , New York : Springer-Verlag .
- Peters , E. 2006 . Propagation and spatial distribution of drought in a groundwater catchment . Journal of Hydrology , 321 : 257 – 275 .
- Peters , E. 2003 . Propagation of drought through groundwater—a new approach using linear reservoir theory . Hydrological Processes , 17 : 3023 – 3040 .
- Rees , G. 2004 . “ Hydrological data. In ” . In Hydrological drought processes and estimation methods for streamflow and groundwater , Edited by: Tallaksen , L.M. and van Lanen , H.A.J. 99 – 138 . Amsterdam : Elsevier Science BV .
- Salas , J.D. 2005 . Characterizing the severity and risk of drought in the Poudre River, Colorado . Journal of Water Resources Planning and Management , 131 ( 5 ) : 383 – 393 .
- Salvadori , G. and De Michele , C. 2004a . Analytical calculation of storm volume statistics with Pareto-like intensity–duration marginals . Geophysical Research Letters , 31 : L04502
- Salvadori , G. and De Michele , C. 2004b . Frequency analysis via copulas: theoretical aspects and applications to hydrological events . Water Resources Research, 40, W , 112511 : 1 – 17 .
- Salvadori , G. and De Michele , C. 2006 . Statistical characterization of temporal structure of storms . Advances in Water Resources , 29 ( 6 ) : 827 – 842 .
- Salvadori , G. and De Michele , C. 2007 . On the use of copulas in hydrology: theory and practice . Journal of Hydrologic Engineering , 12 ( 4 ) : 369 – 380 .
- Santos , M.J.J. , Verissimo , R. and Rodrigues , R. 2001 . Hydrological drought computation and its comparison with meteorological drought , Freiburg , , Germany : Institute of Hydrology, Final report EU contract ENV4-CT-97-0553 .
- Schaake , J. 2006 . The Hydrologic Ensemble Prediction EXperiment (HEPEX) . Hydrology and Earth System Sciences , 3 : 3321 – 3322 .
- Schaake , J.C. 2007 . HEPEX, the Hydrological Ensemble Prediction Experiment . Bulletin of the American Meteorological Society , 88 ( 10 ) : 1541 – 1547 .
- Serinaldi , F. 2009 . Probabilistic characterization of drought properties through copulas . Physics and Chemistry of the Earth , 34 ( 10–12 ) : 596 – 605 .
- Serinaldi , F. and Grimaldi , S. 2007 . Fully nested 3-copula: procedure and application on hydrological data . Journal of Hydrologic Engineering , 12 ( 4 ) : 420 – 430 .
- Sheffield , J. 2009 . Global and continental drought in the second half of the twentieth century: severity–area–duration analysis and temporal variability of large-scale events . Journal of Climate , 22 : 1962 – 1981 .
- Shiau , J.T. 2006 . Fitting drought duration and severity with two-dimensional copulas . Water Resources Management , 20 : 795 – 815 .
- Shiau , J.T. , Feng , S. and Nadarajah , S. 2007 . Assessment of hydrological droughts for the Yellow River, China using copulas . Hydrological Processes , 21 : 2157 – 2163 .
- Shiau , J.T. and Shen , H. W. 2001 . Recurrence analysis of hydrologic droughts of differing severity . Journal of Water Resources Planning and Management , 127 : 30 – 40 .
- Sklar , A. 1959 . Fonctions de répartitions à n dimensions et leurs marges . Publications de l'Institut de Statistique de l'Université de Paris , 8 : 229 – 231 .
- Song , S.B. and Singh , V.P. 2010a . Frequency analysis of droughts using the Plackett copula and parameter estimation by genetic algorithm . Stochastic Environmental Research and Risk Assessment , 24 : 783 – 805 .
- Song , S.B. and Singh , V.P. 2010b . Meta-elliptical copulas for drought frequency analysis of periodic hydrologic data . Stochastic Environmental Research and Risk Assessment , 24 : 425 – 444 .
- Soule , P.T. 1992 . Spatial patterns of drought frequency and duration in the contiguous USA based on multiple drought event definitions . International Journal of Climatology , 12 : 11 – 24 .
- Stahl , K. 2010 . Streamflow trends in Europe: evidence from a dataset of near-natural catchments . Hydrology and Earth System Sciences , 14 : 2376 – 2382 .
- Tabrizi , A.A. 2010 . Utilization of time-based meteorological droughts to investigate occurrence of streamflow droughts . Water Resources Management , 24 : 4287 – 4306 .
- Tallaksen , L.M. , Madsen , H. and Hisdal , H. 2004 . “ Frequency analysis. In ” . In Hydrological drought processes and estimation methods for streamflow and groundwater , Edited by: Tallaksen , L.M. and van Lanen , H.A.J. 199 – 271 . Amsterdam : Elsevier Science .
- Tallaksen , L.M. and van Lanen , H.A.J. 2004 . Hydrological drought: processes and estimation methods for streamflow and groundwater , Amsterdam : Elsevier Science .
- UNESCO (United Nations Educational, Scientific and Cultural Organization), 2009. The United Nations world water development report 3: water in a changing world [online]. http://www.unesco.org/new/en/natural-sciences/environment/water/wwap/wwdr/wwdr3-2009/ (http://www.unesco.org/new/en/natural-sciences/environment/water/wwap/wwdr/wwdr3-2009/) (Accessed: 9 November 2010 ).
- Van den Hurk , B. and Jacobs , D. 2009 . “ The art of predicting climate variability and change ” . In F. Ludwig, et al., eds. Climate change adaptation in the water sector , London : Earthscan .
- van Huijgevoort , M.H.J. 2010 . “ Drought assessment using local and large-scale forcing data in small catchements. In: E. Servat, et al ” . In Global change: facing risks and threats to water resources , Vol. 340 , 77 – 85 . Wallingford , , UK : IAHS Press, IAHS Publ .
- van Lanen , H.A.J. and Tallaksen , L.M. 2008 . “ Drought in Europe. In ” . In Proceedings of water down under 2008 98 – 108 . 14--17 April 2008, Adelaide , , Australia
- van Lanen , H.A.J. 2012 . Hydrological drought across the world: impact of hydroclimatology and physical catchment structure . International Journal of Climatology , submitted
- van Loon , A.F. and van Lanen , H.A.J. 2011 . A process-based typology of hydrological drought . Hydrology and Earth System Sciences Discussion , 8 : 11413 – 11483 .
- van Loon , A.F. 2010 . “ Understanding hydrological winter drought in Europe ” . In Global change: facing risks and threats to water resources , Edited by: Servat , E. Vol. 340 , 189 – 197 . Wallingford , , UK : IAHS Press, IAHS Publ .
- Wanders, N., van Lanen, H.A.J., and van Loon, A.F., 2010. Indicators for drought characterization on a global scale [online]. WATCH. www.eu-watch.org/publications/technical-reports/3 (http://www.eu-watch.org/publications/technical-reports/3) (Accessed: 11 June 2012 ).
- Weedon , G.P. 2011 . Creation of the WATCH Forcing Data and its use to assess global and regional reference crop evaporation over land during the twentieth century . Journal of Hydrometeorology , 12 : 823 – 848 .
- Wilhite , D.A. 2000 . Drought—a global assessment , London : Routledge .
- Wilhite , D.A. 2005 . Drought and water crises, science, technology and management issues , Boca Raton , FL : CRC Taylor and Francis .
- Wong , G. 2010 . Drought analysis using trivariate copulas conditional on climatic states . Journal of Hydrologic Engineering , 15 ( 2 ) : 129 – 141 .
- Yevjevich , V. 1967 . On objective approach to definitions and investigations of continental hydrologic droughts . Hydrology Papers , 23 : 4 – 18 .
- Zhang , L. and Singh , V.P. 2006 . Bivariate flood frequency using the copula method . Journal of Hydrologic Engineering , 11 ( 2 ) : 150 – 164 .
- Zhang , L. and Singh , V.P. 2007 . Gumbel-Hougaard copula for trivariate frequency analysis . Journal of Hydrologic Engineering , 12 ( 4 ) : 409 – 419 .