Abstract
A study of rainfall trends and temporal variations within seven sub-basins of Uganda spanning from 1940 to 2009 has been made. Rainfall climatologies are constructed from observational data, using 36 station records which reflect hydroclimatic conditions. Long-term changes in rainfall characteristics were determined by non-parametric tests (Mann-Kendall and Sen’s T tests), coefficient of variation (CV), precipitation concentration index and drought severity index. Magnitude of change was estimated by applying Sen’s estimator of slope. Decadal variability of rainfall with marked seasonal cycles is evident. Temporal variability of drought patterns is detected. Variations in annual rainfall are low with no significant trends observed in the main drainage sub-basins. Significant trends occur in October, November, December and January. A noticeable decrease in the annual total rainfall was observed mostly in northwestern and southwestern sub-basins. Rainfall trend in the second normal of June–July–August (JJA) was decreasing in all the main drainage sub-basins.
Editor Z.W. Kundzewicz; Associate editor S. Yue
Citation Nsubuga, F.W.N., Botai, O.J., Olwoch, J.M., Rautenbach, C.J.deW., Bevis, Y., and Adetunji, A.O., 2014. The nature of rainfall in the main drainage sub-basins of Uganda. Hydrological Sciences Journal, 59 (2), 278–299.
Résumé
Une étude des tendances et des variations temporelles des précipitations dans sept sous-bassins de l’Ouganda à été réalisée sur lapériode allant de 1940 à 2009. La climatologie des précipitations a été construite à partir des données observées dans 36 stations représentatives des conditions hydroclimatiques. Les changements à long terme des caractéristiques des précipitations, coefficient de variation, indice de concentration des précipitations et indice de gravité de la sécheresse, ont été déterminés par des tests non paramétriques (test de Mann-Kendall et test T de Sen). L’amplitude du changement a été estimée en appliquant l’estimateur de pente de Sen. La variabilité décennale des précipitations aux cycles saisonniers marqués est évidente. La variabilité temporelle des phénomènes de sécheresse a été détectée. Les variations des précipitations annuelles sont faibles sans tendances significatives observées dans les principaux sous-bassins. Des tendances significatives ont été observées en octobre, novembre, décembre et janvier. Une diminution notable des précipitations totales annuelles a été observée principalement dans les sous bassins du Nord-ouest et du Sud-ouest. Une tendance à la décroissance des précipitations en juin–juillet–août (JJA) a été observée dans tous les sous-bassins principaux.
INTRODUCTION
Rainfall, which is the main input to the global hydrological cycle, is one of the most important natural resources that has recently shown significant variations both regionally and globally. Such variation can have profound effects on the availability of water resources, especially those which derive significant recharge from rainfall (Ngongondo Citation2006). Thus, it is important to monitor closely the rainfall variation on monthly, seasonal and annual time scales in order to manage water resources well. For example, 80% of the total catchments of Lake Victoria in East Africa rely on direct rainfall, while the remaining 20% comes from river and underground discharges (Awange Citation2008). Rainfall is also very important for the economic development, disaster management and hydrological planning of a country. This natural resource and the associated variables, such as temperature, runoff and groundwater, are commonly used to characterize the climatic state of a region (Kothyari and Singh Citation1996). Careful assessment of the magnitude and extent of these variables is done, using them as indicators of climatic change.
As a region, Uganda experiences varied rainfall whose effects are especially noticeable in the eastern part, where drought has been reported to be frequent in the last decades and has drastically altered the landscape. Some areas in Uganda receive rains that have resulted in floods every year, destroying property worth millions of Uganda shillings. This kind of weather has evoked considerable speculation that the country’s rainfall characteristics are changing. Detection of rainfall changes over recent decades is needed, together with an appreciation of those characteristics of rainfall supply that are most essential for its effective utilization as a resource and those which are most sensitive to change (Hulme Citation1992). Uganda’s economy is still dependant on agriculture, so extreme events, such as floods, famine, and drought or crop failure, become very critical to the country. Climate change and variability exacerbate the socio-economic woes of Uganda, especially in the light of pressures of trying to achieve food security and alleviate poverty. Furthermore, Uganda has just come out of civil war, but still experiences political volatility, worsening terms of international trade, rapid population growth and HIV infection.
Variability and trends in rainfall are among the important aspects of climate change studies (Patra et al. Citation2011), and there is a lot of literature worldwide on both spatial and temporal variations in rainfall. For example, Ruiz Sinoga (Citation2011) points out studies of temporal variability and trends in precipitation at both global and regional scales in the last 25 years. Very large spatial and seasonal variability, with implications for water management and risk assessment in the northeastern Iberian Peninsula were revealed by (Lopez-Moreno et al. Citation2009). Zhao (Citation2011) used the entropy method to detect the spatial variability of precipitation over temporal scales in Xinjiang, China, which showed that precipitation was increasing especially in mountainous regions. Rai et al. (Citation2010) observed falling trends in temporal scales, including aridity index, over the Yamuna River basin in India. Moderate to high variations in inter- and intra-annual rainfall have been identified by Shahid and Khairulmaini (Citation2009) in Bangladesh. Meanwhile, Ngongondo (Citation2006) found that annual rainfall and groundwater in the Mulunguzi River catchment area (Malawi) had declined by up to 15% and 50%, respectively, since 1954.
Recent studies of rainfall fluctuations in Uganda (e.g. Nsubuga et al. Citation2011) have demonstrated that total rainfall during the March–May season and the number of wet days (at Namulonge) were decreasing. Kizza (Citation2009) found positive trends at most stations located in the northern part of the Lake Victoria basin, especially in the short rains compared to the long rains. Nicholson (Citation1996) also revealed that inter-annual variability of rainfall is remarkably coherent throughout most of eastern Africa, especially during the October–December season, with a dominant time scale of variability of 5–6 years. Phillips and Mcintyre (Citation2000) investigated Pacific El Niño-Southern Oscillation (ENSO) and inter-annual rainfall variability in relation to agricultural management in Uganda. They discovered that Pacific Ocean NINO3 region sea-surface temperatures (SSTs) from July to September are significantly correlated with both August–September and November–December rainfall, but with opposite signs. In another related study on the effects of climate change on the groundwater system of the Upper Ssezibwa catchment, Uganda, Nyenje and Batelaan (Citation2009) found evidence of increasing temperature trends and decreasing trends in river discharge, especially since 1990. No significant trends were observed in precipitation, though it is projected to increase by over 30% and 100%, especially in short and long rain seasons, in the 2020s and 2080s, respectively. The point of departure in this study in relation to previous work is: (a) the wider scope of investigation using stations spread across the country in seven sub-basins; and (b) the use of two non-parametric techniques in conjunction with other indices to establish trends and characterize rainfall in the different drainage sub-basins. The debate about the different results that are sometimes reported (e.g. GOU Citation2007) triggered the study presented herein. The main contribution of the present work is to build on previous studies by utilizing data spanning seven decades, and the results are expected to support policy with regard to water resource management under changing climate across Ugandan sub-basins. In this regard, the study aimed to analyse the evolution of precipitation values at different time scales (monthly, seasonal, intra-annual and annual), as well as the spatial patterns of rainfall trends in the main drainage sub-basins of Uganda.
DATA AND ANALYSIS METHODS
Study area
Uganda is situated in the eastern part of Africa. It covers an area of nearly 241 548 km2, comprising 0.8% of the total geographical area of the continent. Its exact geographical location lies between 1°30′S–4°N latitude and 29°30′E–34°E longitude. The country is landlocked and shares a total length of 2698 km of international borders with its neighbours (MWE Citation2005). Its climate is much influenced by latitude, orographic aspects and the proximity to water bodies. The country has a modified climate characterized by high temperature, high humidity and high rainfall. The annual rainfall varies from 500 to 2800 mm, with an average of 1180 mm (NEMA Citation2008). The spatial pattern of rainfall in Uganda is complex and arises from the interaction of a number of different forcing factors, including large-scale patterns which are dominated by convective rainfall from the seasonal migration of the Inter-tropical Convergence Zone (ITCZ) as it follows the path of the overhead sun, modulated by the complex topography and the presence of lakes. This leads to two main rainfall seasons: the long rains in March, April and May (MAM) and the short rains in September, October and November (SON).
Generally, the seasonal pattern follows this bimodal system near the Equator and tends to a unimodal system away from the Equator (Conway Citation2005, Asadullah et al. Citation2008). Inter-annual variability of rainfall correlates with SSTs in the Pacific through atmospheric teleconnections: the ENSO phenomenon. Highest totals are generally observed in the mountainous regions in the southwest and east, and in the vicinity of Lake Victoria. The lowest rainfall totals are found in the northeast of the country on the border with Kenya and Sudan, where drought is a common occurrence (NARO Citation2001). The country is pleasantly cool with a long-term mean temperature of 21°C. Annual temperature ranges from a minimum of 15°C in July to a maximum of 30°C in February. The economic activities on which the country depends include, among others, agriculture, tourism, industry and services. These are key sectors of the economy that are controlled by climate. In particular, the percentage share of some of these sectors to GDP in 2011, according to the Uganda Bureau of Statistics (UBOS), was 24.1% for agriculture, 26.9% for industry and 49.0% for services (MOFPED Citation2011). Agriculture continues to determine Uganda’s success in terms of economic growth and poverty reduction, because it absorbs the largest share (73%) of the labour force (NEMA Citation2008). Uganda may be divided into eight drainage sub-basins, which drain into the Nile, as shown in . Ranked by area from the largest to the smallest, they are Lake Victoria, Lake Kyoga, Victoria Nile, River Aswa, Albert Nile, Lakes Edward, Lake Albert and Kidepo Valley (MWE Citation2005).
Fig. 1 Map of Uganda showing the distribution of observatories and the size of the main drainage sub-basins. Source of original map: MWE (Citation2005).
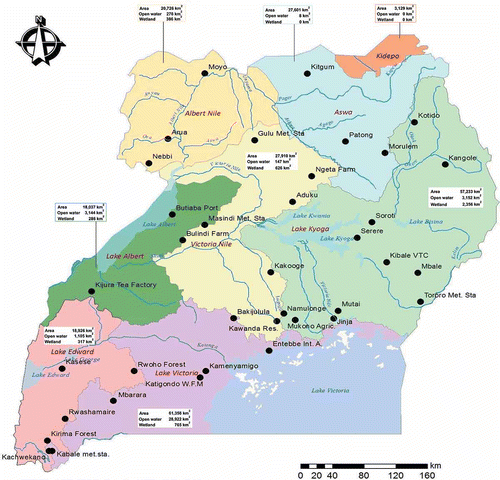
Data preprocessing
Monthly rainfall data (mm) over the study area were obtained from Uganda Department of Meteorology (UDM), as well as from the UBOS. The raw data sets from UDM are archived as monthly values using climate-computing format. A basic data quality check, such as for abnormal values in the data sets, was assumed in the current study as this was confirmed by UDM staff. Stations considered in the study were selected based on the length of time and record of areal coverage. In total, 36 Ugandan rainfall stations, depicted in , were considered. Some station records commence in the 1920s or 1930s and nearly all cover at least the period 1940–1999. Stations in the seven selected sub-basins, namely, Albert Nile, Lake Albert, River Aswa, Lake Edward, Lake Kyoga, Lake Victoria and Victoria Nile (see basin characteristics in ) were used for the study. Kidepo sub-basin was dropped because much of its water drains into the neighbouring countries to the north.
One of the limitations of raw data sets is the occurrence of missing values. Thus, gaps were filled using long-term monthly mean values (i.e. monthly climatology) of a particular month for that station because, in some instances, stations had consecutive missing values (up to a whole year). The authors felt that using interpolation to fill in the missing data gaps could not be robust. Furthermore, records of seasonal and annual totals for each station were derived from the monthly data records. The series were then normalized to minimize any resultant inhomogeneities in the regional series that may have been brought about by varied record length and missing data (Nicholson Citation1986).
For this study, the original monthly precipitation series were normalized into monthly series (Msy) by subtracting the long-term mean, , from the original monthly values, Rsy, and then dividing by the standard deviation (SD), σs, at each station. This process minimizes the problem of highly diverse means and variability, and the randomness of the convective process reflected in the individual station totals (Nicholson Citation1986). The normalized monthly rainfall anomaly for a given station is given by:
Methodology
Rainfall patterns across the different sub-basins were characterized using statistics such as mean, SD and CV, and the percentage contribution to annual was computed for monthly and seasonal periods, i.e. December–January–February (DJF), MAM, June–July–August (JJA) and SON. Furthermore, the precipitation concentration index (PCI) proposed by Oliver (1980, in Shahid and Khairulmaini Citation2009) was used to analyse the annual rainfall distribution. Here, PCI is expressed as a percentage, according to De Luis et al. (Citation2000) and Ceballos et al. (Citation2004):
Using rainfall data computed from equations put forward by Awange et al. (Citation2008), seasonal means, , were obtained by averaging the monthly totals, Mt, for three months of a particular season, i.e.:
Wet: >75%
Near normal: 25–75%
Drought: <25%
In order to investigate for the presence of trends in the data sets, the Mann-Kendall (MK) test was used. This test, which is also known as Kendall’s t-statistic, is a non-parametric method that has been widely used to test for randomness against trend in hydrology and climatology. It is a rank-based procedure, which is robust to influence of extremes and good for use with skewed variables (De Luis et al. Citation2000, Yue Citation2002, CRCCH Citation2005, Partal and Kahya Citation2006, Nsubuga et al. Citation2011). The null hypothesis H0 of no trend (i.e. the observations are randomly ordered in time) is tested against the alternative hypothesis, H1, associated with increasing or decreasing monotonic trends (de Lima Citation2010). In this work, the null hypothesis is tested at 5% significance level, taken in its two-sided form. This method accounts for missing values, serial correlation and numbers below a detection limit that commonly confound trend detection procedures in time series analysis (Hirsch and Slack Citation1984). The MK statistic has the advantage of making no assumptions about the statistical distribution of variables (Kampata et al. Citation2008). Furthermore, Sen’s T test was also used for trend analysis. Sen’s T test is an aligned rank method having procedures that first remove the block (season) effect from each datum, then sum the data over blocks, and finally produce a statistic from these sums. The aligned rank test is more powerful, its distribution free and not affected by seasonal fluctuations (Partal and Kahya Citation2006 and references therein). The procedure used in the present analysis was as outlined by Partal and Kahya (Citation2006), and takes the following steps:
Compute xj and xi for averages of the jth month and ith year by:
(10)
Subtract the monthly average from each of the corresponding months in the n years of data to remove monthly or seasonal effects.
Replace all differences obtained in Step 2 by ranks, resulting in a similar table with the notation of R instead of X. It is important to note that the integer 1 would be assigned to the smallest difference, whereas the integer nm would correspond to the rank of the highest difference. Hence, Rj refers to the rank (xij – xj), where i = 1, …, n and j = 1, …, m. For each of the t tied values, the average of the next t ranks is assigned.
Calculate the test statistic:
(11)
The non-parametric method proposed both by Theil (1950) and Sen (1968) was used to estimate the magnitude of trends. This approach uses Sen’s slope estimation method by assuming the trend to be linear, such that f(t) = Q(t) + B, where Q is the gradient of the linear trend, and B is a constant. If a linear trend is present in a time series, then the true slope (change per unit time) can be estimated by using a simple non-parametric procedure developed by Sen (1968b in Partal and Kahya Citation2006). The slope estimates of N pairs of data are first computed by:
RESULTS AND DISCUSSION
A subset of 36 stations was used to derive areally averaged departure series for the seven sub-basins in this study (locations shown in ). The highest and lowest values of annual total rainfall, with years of occurrence, for each station in the respective drainage sub-basin are shown in .
Table 1 Basic statistical characteristics of annual rainfall data for the 35 stations in the seven drainage sub-basins in the period 1940–2009.
Serial correlation effect
Bold figures in indicate series, which are serially correlated at 95% level of significance. Annual series of 18 stations show that the data do not come from the same random process. The results reveal a significant serial correlation value in 51% of the 36 stations (). The locations of the stations with a significant serial correlation were, for the most part, in central Uganda, especially in the Lake Victoria sub-basin.
Rainfall characteristics
Mean rainfall for the 70-year period was 43 842 mm; maximum and minimum rainfall was recorded in 1961 and 1943, respectively, with a CV of 7.23%. Cycles are observed in rainfall patterns in Uganda for the period 1940–2009. Data show decades of normal and beyond-normal rainfall () during the period of analysis. Three long spans of below-normal rain are evident in the 1940s, 1960s/70s and 1980s. Other particularly wet periods were experienced in the early 1950s, 1960s, the late 1970s and the 1990s (). The contributing factors to the below-average annual total rainfall for Uganda have not been established in this study, despite the 1980s being drought years which had consequences on food security for the continent (Hulme Citation1992). shows that very high rainfall totals in the early 1960s and late 1970s were preceded by relatively low rainfall in Uganda.
Average seasonal cycles in precipitation rate from station data for 1940–2009 over the seven sub-basins in Uganda are shown in . It can be seen that there is a marked contrast in seasonal cycle between sub-basins that lie over and below latitude 2°N (i.e. northern and southern sub-basins). According to Black et al. (Citation2003), this contrast is evidence that reflects a strong influence of the southwest monsoon circulation on rainfall in East Africa. The other basins depict low amounts of rain during the southwest monsoon and show a semi-annual distribution of rainfall, which is characteristic of the equatorial zone.
Mean rainfall
Calculations for station means reveal that Entebbe International Airport recorded the highest mean annual rainfall (1617 mm), followed by Gulu meteorological station (1559 mm), during the time of analysis. In contrast, Kangole station shows the lowest mean rainfall (660 mm) followed by Kotido (677 mm) (). These two stations with low rainfall lie within the Lake Kyoga sub-basin in the far northeast of Uganda ().
It is also revealed that eight stations (Morulem, Kawanda, Kijura, Nebbi, Tororo, Mutai, Kangole and Kibale) had their highest rains recorded in 1961. Six of these stations are within the Kyoga sub-basin. Reports in the literature have indicated that the 1960s were times of high rains (e.g. Nicholson Citation1996, Kizza et al. Citation2009). Overall 12 stations recorded very high rainfall in the 1960s (). Six stations (Rwashamaire, Mbarara, Kamenyamigo, Rwoho Forest station, Moyo and Mbale) had their highest rains in 1951 (). Four of these stations are located in the Lake Victoria sub-basin and, specifically, lie within what is often referred to as “the dry corridor of Uganda”. This area lies within the rain shadow of the western highlands, which shield it from the winds coming from the Congo basin, and is also far from the reach of the southeast monsoon winds. These wind types have been identified to be influential in the distribution of rainfall in Uganda (Basalirwa Citation1995). The mean annual rainfall for these stations ranges between 985 mm at Rwoho and 1025 mm at Rwashamaire (CV between 17% and 22%). Mean annual rainfall for most of the stations under analysis exceeds 1200 mm. Unlike that reported by Nicholson (Citation1996), the highest mean rainfall during the period of analysis is recorded around the lakes.
Coefficient of variation
Inter-annual variability, according to Hulme (Citation1992), is an important indicator of the reliability of the rainfall resource in Africa. The CVs for 36 stations were computed in order to investigate the spatial pattern of inter-annual variability of rainfall totals over Uganda.
The CV is defined as:
Variations in sub-basin normalized rainfall series
Before using the sub-basin normalized series for further analysis, the variance analysis was applied to regional data in order to assess whether the series adequately represent the region as a whole. Using the ratios of the variance estimates in time to the variance estimates in the area, it is apparent that all area-averaged rainfall anomalies have coherence in rainfall fluctuations (results not shown). An F-test helps to assess the relative importance of the two components of variance (Nicholson Citation1986). In most cases, according to Nicholson (Citation1986), the limiting value for the 0.01 probability level is about 1.50 or lower. Results from the analysis show that the majority of the series had values exceeding 1.50, meaning that there is a 1% probability that most of the temporal variance in the sub-basin can be accounted for by random fluctuations at a few stations.
Characterizing monthly rainfall
The concentration of the rainfall in a year is an important aspect of climate. Simply, this is because when rainfall distribution is not balanced, it makes water resources planning, plant and crop growth difficult. It is therefore important to investigate rainfall concentration needs using a statistically derived index (PCI) that quantifies the relative distribution of the rainfall patterns. Based on the approach described by Michiels et al. (Citation1992), two different calculation procedures were used for deriving the PCI. In the first procedure, the mean monthly rainfall data set was estimated by averaging the monthly rainfall data over a number of years; afterwards the PCI was calculated (here referred to as seasonal concentration). In the second procedure, the PCI was calculated from rainfall totals of individual years (here referred to as temporal concentration). The PCI permits grouping of data sets according to the derived value: PCI < 10 suggests a uniform distribution, a value from 11 to 15 denotes a moderate seasonal distribution and a value from 16 to 20 denotes a seasonal distribution (Michiels et al. Citation1992). A PCI > 20 represents strong seasonal effects, with increasing values indicating increasing monthly rainfall concentration.
The application of the first procedure based on the mean monthly rainfall data sets of the seven sub-basins () results in PCI > 10 for Albert Nile and Aswa, suggesting a uniform to moderate seasonal distribution of rainfall in the northern sub-basins of Uganda. The other five sub-basins reveal a PCI < 10, suggesting uniform seasonal rainfall. Temporal analysis for all stations results in PCI values below 10, which indicates a uniform concentration of rainfall for the years under analysis. The annual PCIs calculated for individual years are small compared to the seasonal concentration index, because the monthly rainfall of individual years deviates from the long-term mean monthly rainfall. Higher values for temporal concentration of rainfall for individual years can also be due to seasonal concentration of the rainfall, or to a random deviation from the long-term mean monthly rainfall (Michiels et al. Citation1992). Further analysis at 30-year normals (1940–1969 and 1970–1999) also reveals the same pattern, which further implies that the distribution of rainfall in the sub-basins has not changed with time ().
Table 2 Results from PCI calculations using temporal and seasonal concentration methods.
Since the PCI, like other statistical indices, does not reveal the actual month or season of maximum or minimum rainfall, examination of the sub-basin data sets is necessary to identify the month (or season) with maximum (or minimum) rainfall, if there is any.
demonstrates that there is not a single month without rainfall, which is consistent with the uniform to moderate seasonal distribution derived from the PCI. One can conclude from the shape of the mean monthly rainfall plots that rainfall distribution is bi-modal in four of the sub-basins (Lake Albert, Lake Edward, Lake Victoria, Victoria Nile) located in the west and central parts of Uganda and unimodal in the two sub-basins (River Aswa, Albert Nile) located in the northern part. Lake Kyoga sub-basin in the central part and extending to the east of Uganda, gives a different picture of a well-spread distribution of rainfall for a period of 9 months (March–November). The plot indicates two peak periods of rainfall in April/May and August/September. This is evidence of a transition zone between the northern and southern sub-basins. Such a trend could also be attributed to the merging of stations to derive an area-averaged series.
Maximum rainfall in the four sub-basins which experience a bi-modal distribution pattern is recorded in the MAM and SON seasons. In July and August, the four sub-basins recorded low rainfall. Lake Kyoga sub-basin, however, indicates low rainfall during DJF. Peak rainfall for the northern sub-basins (River Aswa and Albert Nile) is in August (). This kind of rainfall pattern over Uganda is related to the movement of the ITCZ, which brings rainfall approximately 1 month after the sun’s migration north or south resulting in a bi-modal rainfall pattern, with the primary rain season occurring in MAM, and a shorter secondary rainy season occurring in October–November–December (OND) (Nicholson Citation1996, Kizza et al. Citation2009). Phillips and Mcintyre (Citation2000) attribute moisture during OND to the northeasterly winds originating in the Indian Ocean. However, the northern sub-basins, which have peaks in August, receive moisture from the Congo/Zaire basin to the southwest.
Fig. 6 Distribution of long-term mean monthly rainfall (mm) in the sub-basins of Uganda from 1940 to 2009.
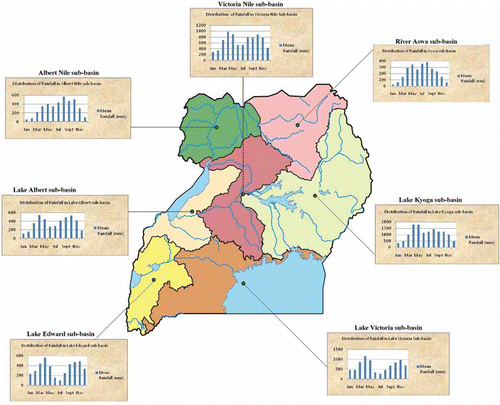
Visual inspection of plots in led us to examine the contribution of each month to the annual total rainfall in the seven sub-basins (expressed as a percentage). Five of the sub-basins show that April contributes the highest percentage of rainfall to the annual total received, while two basins reveal that the highest percentage of rain is received in August. The analysis also reaffirms that central and southern sub-basins receive a high percentage of rains in the MAM and SON seasons. The Albert Nile and River Aswa sub-basins have a longer wet season (April–October) compared to the rest of the sub-basins. Further explanation underlying the general atmospheric circulation that accounts for the above rainfall patterns can be found in the literature (e.g. Ogallo Citation1993, Basalirwa Citation1995, Nicholson Citation1996).
Drought years from rainfall anomalies
Drought may be defined as “a recurring extreme climate event over land characterized by below-normal precipitation over a period of several months to several years, or even a few decades” (Dai Citation2011, p. 1). Drought years from annual and seasonal anomalies of the sub-basins are identified in two climatic normals, i.e., 1940–1969 and 1970–1999. Droughts and floods may be linked to the ENSO, Indian Dipole and other large-scale climate system (e.g. Ogallo Citation1988, Nicholson Citation1996). Our study indicates that there were anomalous years that received below- and above-normal rainfall. The tendency for rainfall to be above average in most of the sub-basins during ENSO years and for drought to occur during the following year ((a)–(g)) is evident. We also investigated what Nicholson (Citation1996, p. 49) referred to as “seasonal preference” for the ENSO-related rainfall anomalies. She noted that, in East Africa, “positive anomaly occurs during the short rains of an ENSO year and the drought during the long rains of the following year”. Our analysis reveals that the seven sub-basins experienced drought in the periods 1945/46, 1952–1954 and 1980–1984 (not shown here). Using the argument of Nicholson (Citation1996), the years 1942, 1952, 1958, 1964, 1969, 1973, 1979 and 1983 should have been drought years. Using the percentage of normal from equation (9), the DSI, captures well the predicted years among others (results not shown). Some of the years revealed by the analysis were identified as La Niña years by Phillips and Mcintyre (Citation2000).
For the 30-year period (hereafter referred to as the climatic normal) and for each season, the occurrences of drought across the respective sub-basins were also investigated. For example, 1943 and1949 appear as drought years for six sub-basins, while 1952/53 appears as drought in five sub-basins. Further, Albert Nile, Victoria Nile and Lake Victoria had similar drought years in the first climate normal (1940–1969), which is also reflected in the MAM rainfall seasons of Albert Nile and Victoria Nile sub-basins. The three sub-basins have 62.4% of the country’s water resources when combined. Thus, drought occurrence in these sub-basins in the future may have adverse effects on the water distribution with in the country.
MK test
Seventeen precipitation variables (i.e. 12 monthly totals, annual and seasonal series) were subjected to the MK test at each sub-basin (). Significant increasing trends at the 0.05 significance level were revealed for January (14%), October (57%), November (43%) and December (14%). The other months showed increasing and decreasing trends which were not statistically significant, but the SON season had 43% increasing trends. The number of negative trends exceeded that of positive trends during April, May and August, especially in the sub-basins that lie on or close to the Equator. This means that the sub-basins are experiencing a reduction in rainfall in the months that contribute the greatest percentage of rainfall. The continued reduction may affect the timing of agricultural activities in the sub-basins in the future.
Table 3 Temporal rainfall characteristics in the main drainage sub-basins of Uganda.
Monthly total precipitation
During January, only positive trends are detected in all sub-basins. During February, four sub-basins to the north and west of Uganda showed positive trend. Negative trends are observed in sub-basins in central Uganda around Lake Victoria and the Victoria Nile. During March, the sense of trend is positive in the central, east and west and becomes negative for the northern sub-basins (Albert Nile and River Aswa). When it comes to April, the trend behaviour is mixed in such a way that decreasing trends are dominant, with occurrences around the northwestern, southwestern and central parts of Uganda. May has more occurrences of decreasing rainfall, with one sub-basin (Lake Edward) showing no trend. June shows mixed occurrences of trend where four sub-basins have positive trends, and the trends in the three sub-basins that form the Albert water management zone are decreasing. During July and August, five sub-basins demonstrate increase and decrease in rainfall, respectively. Kyoga and Victoria Nile are sub-basins that have shown a non-significant decrease in both months. September is one of those months whose results give mixed trend characteristics. Three of the sub-basins detected experienced a decreasing trend from May to September when they hit a turning point and started to increase. During OND, homogeneity of direction across the country is detected, similar to what Nicholson (Citation1996) found for most of East Africa: in October four sub-basins (River Aswa, Lake Albert, Lake Victoria and Victoria Nile), in November three sub-basins (River Aswa, Lake Albert and Lake Kyoga) and in December one sub-basin (Albert Nile) demonstrated significant trends at the 0.05 level. Kizza et al. (Citation2009) also found positive trends in the short rains at most stations located in the northern part of the Victoria basin.
Climatic normal
To ascertain the changed behaviour of annual, seasonal and monthly rainfall, we performed a trend analysis within a climatic normal, and the results are given in . The analysis shows more positive trends in monthly rainfall totals, especially in the second normal. Positive trends were dominant during OND, and these were equally distributed in the first and second normals. Negative trends are prominent in January and August during the first normal (1940–1969). The rest of the months show mixed results of increasing and decreasing trends during the period of analysis. Significant trends for the first normal were evident in Victoria Nile during March, in Lake Edward during July, Lake Kyoga and Victoria Nile during August and Lake Albert sub-basin during November (). Six of the sub-basins experienced significant trends in October, except Albert Nile sub-basin. However, the second normal showed significant negative trends in May and September for River Aswa, October trends were positive for Lake Albert and Lake Edward, and in December positive significant trends were evident for the Albert Nile, Lake Albert and Lake Kyoga sub-basins ().
Annual total precipitation
shows the spatial distribution of negative and positive trends for annual total rainfall. The majority of the sub-basins have positive trends which are non-significant at 5%. Sub-basins showing negative trends are Albert Nile and Lake Edward. From a regional perspective, the decreasing trends are observed in western and northwestern Uganda. The rest of the regions show an increase in annual total rainfall.
Fig. 7 Spatial distribution of negative (–) and positive (+) trends for annual total rainfall in the main drainage sub-basins in Uganda.
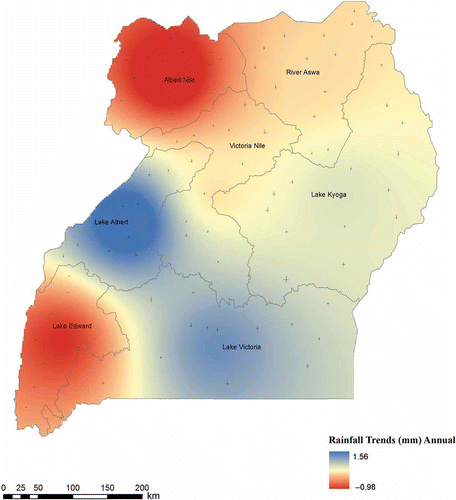
The time-series plots for Albert Nile sub-basin indicate a slight decrease in annual total rainfall with a low-frequency fluctuation ((a)). The normalized rainfall anomalies for Albert Nile sub-basin are characterized by four major wet periods, during the years 1954–1958, 1961–1964, 1976–1979 and 1997–1998, and three major dry periods, during the years 1942–1944, 1966–1974 and 1980–1994 ((a)). The maximum and minimum rainfall was recorded in 1961 and 1971, respectively ().
Fig. 8 Variations and trends in normalized annual rainfall anomalies series with nine-point Gaussian filter, over the main drainage sub-basins in Uganda.
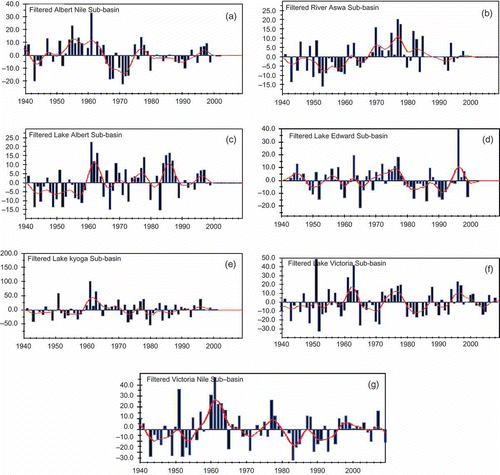
The time-series plot of River Aswa sub-basin ((b)) shows a slightly decreasing trend in annual rainfall with high inter-annual variability. Three notable periods, 1942–1945, 1952–1960 and 1964–1968, were dry. Almost all years within the period 1952–1960 are characterized with negative rainfall anomalies. The wet periods were revealed in the years 1946–1951, 1961–1963, 1969–1972, 1976–1978 and 1982–1984, with some dry breaks in between of one or two years. The maximum and minimum rainfall values were recorded in 1977 and 1953, respectively ().
The time-series plot of Lake Albert sub-basin ((c)) exhibits high-frequency fluctuation. There was a major dry spell during the period 1942–1960. However, the situation reverses to shorter dry years than wet years during the period after 1960. The wettest and driest years were 1961 and 1983, respectively ().
The normalized rainfall anomalies of Lake Edward sub-basin show a high frequency about a decreasing long-term mean ((d)). In spite of a spell of wet years during the periods 1943–1945, 1974–1978 and 1996–1998, one can also detect wet anomalies interspersed with short dry spells of 1 or 2 years in the period 1954–1974. The sub-basin experienced two long dry periods between 1979–1986 and 1990–1995. Highest rainfall was recorded in 1996, while the lowest was in 1965 ().
The time-series plots of Lake Kyoga sub-basin show a slightly downward trend in annual rainfall with a weak frequency fluctuation ((e)). The sub-basin is characterized by three major dry periods, during the years 1942–1949, 1952–1959 and 1979–1981 and a wet period in 1961–1970, with the exception of two, one-year dry breaks in 1965 and 1971 and a two-year dry break in 1975–1976. After the 1997/98 wet spell (El-Niño years in Uganda), annual totals have continued to decline. Maximum rainfall was recorded in 1961 and minimum rainfall in 1980 ().
A snapshot inspection of the time-series plot of Lake Victoria sub-basin would give an impression that the normalized rainfall series have equal variation of wet and dry years ((f)). The frequency is apparently low, which tends towards a reduction in annual total rainfall. Sub-basin total rainfall anomalies experienced four major wet conditions during the periods 1961–1964, 1972–1978, 1987/88 and 1996/97, along with seven dry conditions during the periods 1940–1950, 1951–1954, 1958–1961, 1965–1971, 1980–1986, 1989–1993 and 2002–2005. The year 1951 is the wettest on the sub-basin record, with a positive anomaly of +48.4, while 1952 was the driest with a negative anomaly of –33.3.
The time-series plots of the Victoria Nile sub-basin depict high frequency of variability ((g)). This is the only sub-basin that has equal periods of wet and dry years. Wet conditions dominate during the periods 1940–1942, 1951, 1960–1964, 1977–1979, 1987/88, 1996–1999 and 2006/07, with the highest wet anomaly in 1961, whereas dry conditions occur during the periods 1943–1950, 1952–1954, 1968–1972, 1980–1986, 1989–1995, 2004/05 and 2008. The year 1983 is the driest of the sub-basin annual series, with a high negative anomaly of –28.5.
A closer inspection of the above plots () reveals consistence in what is found in the literature. For example, the annual series plots above indicate dry conditions in the 1950s and wet conditions in the 1960s. Analysis also shows that sub-basins such as Albert Nile, River Aswa, Lake Albert, Lake Edward & George, Lake Kyoga and Kafu-Victoria Nile recorded their highest rainfall in 1961 and 1977. These sub-basins were affected by the incursion of humid Congo air, which played a role in the 1961/62 floods (Nicholson Citation1996). This study has found that dry conditions existed in the early 1980s and 1990s, and relatively wet conditions were experienced in the late 1990s ((a)–(g)).
Seasonal trends
Inspection of the map patterns in ((a)–(d)) and reveals an interesting pattern of trends in the seasonal distribution of rainfall. For instance, the DJF season has positive trends in all sub-basins, although none of the trends is statistically significant at the 0.05 level ((a)). The respective slopes of change are also negligible, where the highest is 0.11 in Victoria Nile sub-basin (). The MAM season, a period which contributes a high percentage of rainfall ((b)), revealed mixed trends of decrease and increase that are not statistically significant at the 0.05 level. Four of the sub-basins exhibit positive trends, and the three show negative trends. These results are consistent with what Nyenje and Batelaan (Citation2009) found. They predict a general increase in rainfall, with the greatest increase expected in the wet seasons (MAM and OND). Unlike the rest of the seasons, JJA has more negative trends than positives, with slope changes that are negligible (). A positive trend was recorded in the River Aswa sub-basin for the JJA season ((c)). Maximum and minimum rainfall at this basin was recorded in 1946 and 1959, respectively.
Fig. 9 Spatial distribution of negative (–) and positive (+) trends for seasonal distribution of rainfall for the period 1940–2009 in the main drainage sub-basins of Uganda. Magnitude is in mm/season (not indicated on map).
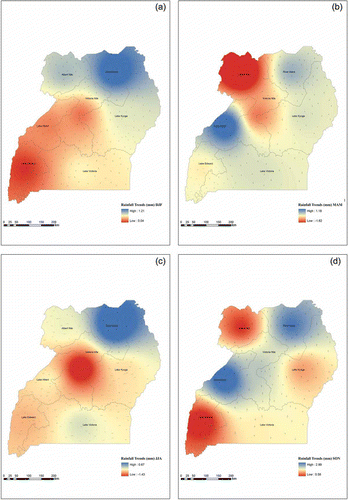
Rainfall trends in the SON season are positive for all sub-basins ((d)), with slope changes that are negligible. This is the only season where positive and statistically significant trends (at the 0.05 significance level) have been revealed at Lake Albert, Lake Victoria and Victoria Nile sub-basins (). Most of the high rains during the period of analysis were recorded in 1961 for five of the sub-basins, with the exception of River Aswa for 1983 and Lake Edward and George for 1996. Both the literature and our analysis identify SON as the second wettest season that contributes greatly to the total annual rainfall in the seven sub-basins. Downscaled data analysed for the SON season by Nyenje and Batelaan (Citation2009) project that rainfall will increase in this season by more than 30% in the 2020s, >80% in the 2050s and >100% in the 2080s. Three years, 1941, 1951 and 1957, which are also mentioned by Nicholson (Citation1996) and Phillips and Mcintyre (Citation2000), have been detected as wet years in the analysis of the sub-basin series in this study.
Trend analysis has shown that the only months in which significant rainfall occurs throughout the analysis period are OND. It is also identified that the climatological seasons are DJF, MAM, JJA and SON. The means of these periods are depicted in . Coefficients of correlation between annual and seasonal rainfall departure series for individual sub-basins are presented in . Correlation coefficients between annual totals and MAM exceed 0.5 for nearly all sub-basins, except for Lake Albert sub-basin, whose correlation coefficient is 0.2. Correlation coefficients for SON are above 0.4 and the highest, for Lake Edward basin, correlation coefficient is 0.7. The correlation coefficients are somewhat higher for four sub-basins that cover an extensive area in Uganda during the DJF season. The Albert Nile, River Aswa and Lake Edward sub-basins depict correlation coefficients above 0.5, while the other four have low values during the JJA season (). Since the three sub-basins have peak rains in August relative to others ((a), (b) and (e)), this is evidence to support the argument that some parts of the country receive moisture from the Congo/Zaire basin to the west (Ogallo Citation1988, Phillips and Mcintyre Citation2000).
Table 4 Coefficients of correlation between annual and seasonal rainfall departure series for main drainage sub-basins in Uganda.
Sen’s T test
The Sen’s T test results indicate negative trend in six of the sub-basins. The sub-basins for which negative trends were detected are Albert Nile, River Aswa, Lake Albert, Lake Edward, Lake Kyoga and Lake Victoria. Significant decreasing trends were observed in River Aswa and Lake Albert sub-basins. The results for Victoria Nile sub-basin show a positive trend, which is not statistically significant at the 0.05 level. The results are summarized in .
Sen’s estimator
The Sen’s slopes (Qi) of all trends in rainfall for the sub-basins are presented in for three periods: 1940–2009 and the two normals, 1940–1969 and 1970–1999. The results indicate change per unit time of the trends detected monthly, annually and seasonally, but do not reveal any slope that is statistically significant. It has also been presented that slopes for DJF are positive for all sub-basins, while those of JJA are negative, with the exception of Albert Nile and River Aswa. The rest of the seasons across all sub-basins show slopes with mixed signs. The positive change in DJF can be attributed to the increase in rainfall in December and January. The other months that showed positivity are November and December, with slopes that were higher in the second climatic normal. However, all these magnitudes of change in all sub-basins are not statistically significant at the 0.05 level. Seasonal slopes for the sub-basins indicate that the magnitude of change was higher in the first normal (1940–1969) than those calculated for the second normal, except for the JJA season in Victoria sub-basin.
DISCUSSION AND SUMMARY
The conclusions drawn from understanding the nature of rainfall can be applied to various social and economic spheres to support decision-making related to water resources for urban and industrial use, energy planning, tourism, commercial activities, protection of the environment and agricultural production (Sinoga et al. Citation2011). These aspects of local environmental conditions, natural resources and social–economic activities are closely linked. For example, when rains were late and early rains were poor in the East African region in 2010, it created a situation of food insecurity in the region (MOFPED Citation2011), resulting in hyperinflation, among other factors. However literature on Uganda’s rainfall is scarce and, where it is available, it is on a small-scale analysis or in less detail within the East African context. As a starting point to help in the decision-making process on water resources availability, the increasing need to know the precipitation behaviour and trends justifies the present work, in a water resource-rich country like Uganda.
A study by Guijarro (2002 in Sinoga et al. Citation2011) warns of the tendency of increased spatial–temporal variability in seasonal precipitation and the low statistical significance occasionally found (as a result of the high variability in rainfall) that can lead to underestimating the importance of these precipitation trends. If such trends continue in the sub-basins studied, there will be major changes in distribution of water resources, which are critical for economic activities like tourism, energy, industry and agriculture.
Starting out from a wide base of 36 observatories, with homogeneous data and contrasting quality, the precipitation trends and the spatial patterns of precipitation evolution in time have been analysed for the period 1940–2009. The analysis shows decades of normal and beyond normal rainfall, with a marked contrast in seasonal cycles within sub-basins. Mean annual rainfall is seasonal, with much of the rainfall measured during March–May, and, although no significant change in annual rainfall can be discerned in Uganda, an increase in the variability in the distribution of monthly rainfall can be seen. Variations in annual rainfall are relatively low which implies a dependable rainfall distribution. Results also indicate that no single month in a year of studied sub-basins for Uganda goes without rainfall. Sub-basins in the north have a unimodal type of rainfall distribution, while those in the south have a bimodal distribution with maxima in July–August and March–May, respectively. The DSI used in the study informs us of the drought years during the analysis period, which was consistent with previously published results.
From the monthly rainfall variables, October, November, December and January were found to have significant increasing trends, as opposed to other months showing positive or negative trend with in the main drainage sub-basins in Uganda. The result of trend analysis of monthly total rainfall, especially in October and November, is reflected in the SON season, but not in the annual series. There were downward trends in the annual rainfall series, predominantly in northwestern and southwestern parts of Uganda, while the rest of the sub-basins had upward trends.
The results could have considerable implications for society and the economy. A decrease in inland precipitation will cause problems. This is because the reservoirs controlling and storing water resources are located in the northeastern (Aswa/Kyoga) part of the study region, and a decrease in precipitation in this area could reduce water reserves. These reservoirs (valley dams) supply water to the pastoralists in times of drought. Studies of trends in rainfall and its evolution provide useful tools for managers and administrators of water resources, especially in areas where periods of drought can result in major economic losses, and social and political conflict.
Water is probably the country’s most abundant resource, and therefore it seems to attract less attention, but if water demand increases and pessimistic predictions about climate change come true, then adverse effects have to be faced. These will require efficient water policy actions with a high degree of hydrological planning that demands appropriate infrastructures and a rational use of the resource.
It is worthwhile emphasizing that the trend results presented in this study were not sufficient to suggest climatic change in the main drainage sub-basins of Uganda. Future studies are needed to address the issue of trend attribution and to attempt to establish a linkage between climatic change and the observed hydrological trends.
REFERENCES
- Asadullah, A., Mcintyre, N., and Kigobe, M., 2008. Evaluation of five satellite products for estimation of rainfall over Uganda/Evaluation de cinq produits satellitaires pour l’estimation des précipitations en Ouganda. Hydrological Sciences Journal, 53 (6), 1137–1150. doi:10.1623/hysj.53.6.1137
- Awange, J.P., et al., 2008. Falling Lake Victoria water levels: is climate a contributing factor? Climatic Change, 89, 281–297. doi:10.1007/s10584-008-9409-x
- Basalirwa, C.P.K., 1995. Delineation of Uganda into climatological rainfall zones using the method of principal component analysis. International Journal of Climatology, 15, 1161–1177. doi:10.1002/joc.3370151008
- Black, E. and Slingo, J., 2003. An observational study of the relationship between excessively strong short rains in coastal East Africa and Indian ocean SST. Monthly Weather Review, 131, 74–94. doi:10.1175/1520-0493(2003)131<0074:AOSOTR>2.0.CO;2
- Ceballos, A., Martı́nez-Fernández, J., and Luengo-Ugidos, M.A., 2004. Analysis of rainfall trends and dry periods on a pluviometric gradient representative of Mediterranean climate in the Duero basin, Spain. Journal of Arid Environments, 58, 215–233. doi:10.1016/j.jaridenv.2003.07.002
- Conway, D., 2005. From headwater tributaries to international river: Observing and adapting to climate variability and change in the Nile basin. Global Environmental Change, 15, 99–114. doi:10.1016/j.gloenvcha.2005.01.003
- CRCCH. Co-operative Research Centre for Catchment Hydrology, 2005. TREND user guide, p. 17.
- Dai, A., 2011. Characteristics and trends in various forms of the Palmer Drought Severity Index during 1900–2008. Journal of Geophysical Research, 116, D12115. doi:10.1029/2010JD015541
- de Lima, M.I.P., et al., 2010. Trends in precipitation: analysis of long annual and monthly time series from mainland Portugal. Advances in Geosciences, 25, 155–160. doi:10.5194/adgeo-25-155-2010
- De Luis, M., et al., 2000. Spatial analysis of rainfall trends in the region of Valencia (East Spain). International Journal of Climatology, 20, 1451–1469. doi:10.1002/1097-0088(200010)20:12<1451::AID-JOC547>3.0.CO;2-0
- GOU, 2007. Climatic change: national adaptation plan action. Kampala: Ministry of Water and Environment.
- Hirsch, R.M. and Slack, J.R., 1984. A nonparametric trend test for seasonal data with serial dependence. Water Resources Research, 20 (6), 727–732. doi:10.1029/WR020i006p00727
- Hulme, M., 1992. Rainfall changes in Africa: 1931–1960 to 1961–1990. International Journal of Climatology, 12, 685–699. doi:10.1002/joc.3370120703
- Kampata, J.M., Parida, B.P., and Moalafhi, D.B., 2008. Trend analysis of rainfall in the headstreams of the Zambezi River Basin in Zambia. Physics and Chemistry of the Earth, Parts A/B/C, 33, 621–625. doi:10.1016/j.pce.2008.06.012
- Kizza, M., et al., 2009. Temporal rainfall variability in the Lake Victoria Basin in East Africa during the twentieth century. Theoretical and Applied Climatology, 98, 119–135. doi:10.1007/s00704-008-0093-6
- Kothyari, U.C. and Singh, V.P., 1996. Rainfall and temperature trends in India. Hydrological Processes, 10, 357–372. doi:10.1002/(SICI)1099-1085(199603)10:3<357::AID-HYP305>3.0.CO;2-Y
- Kraus, E.B., 1977. Subtropical droughts and Cross-Equatorial energy transports. Monthly Weather Review, 105, 1009–1018. doi:10.1175/1520-0493(1977)105<1009:SDACEE>2.0.CO;2
- Lopez-Moreno, J.I., et al., 2009. Trends in daily precipitation on the northeastern Iberian peninsula 1955–2006. International Journal of Climatology, 30, 1026–1041.
- Michiels, P., Gabriels, D., and Hartmann, R., 1992. Using the seasonal and temporal precipitation concentration index for characterizing the monthly rainfall distribution in Spain. Catena, 19, 43–58. doi:10.1016/0341-8162(92)90016-5
- MOFPED (Ministry of Finance, Planning and Economic Development), 2011. Annual economic performance report 2010/11. Kampala: Directorate of Economic Affairs, Ministry of Finance, Planning and Economic Development, December 2011.
- MWE (Ministry of Water and Environment), 2005. Uganda National Water Development Report 2005. Kampala: Ministry of Water and Environment.
- NARO (National Agriculture Research Organisation), 2001. Agriculture in Uganda. Vol. 1. Kampala: Fountain Publishers Ltd. General Information. ISBN 9789970022434.
- NEMA (National Environment Management Authority), 2008. State of Environment Report for Uganda, 2008. Kampala: NEMA.
- Ngongondo, C.S., 2006. An analysis of long-term rainfall variability, trends and ground water availability in the Mulunguzi river catchment area, Zomba mountain, southern Malawi. Quaternary International, 148, 45–50. doi:10.1016/j.quaint.2005.11.006
- Nicholson, S.E., 1986. The nature of rainfall variability in Africa south of the equator. International Journal of Climatology, 6, 515–530. doi:10.1002/joc.3370060506
- Nicholson, S.E., 1996. A review of climate dynamics and climate variability in Eastern Africa. In: T.C. Johnson and E. Odada, eds. The limnology, climatology and paleoclimatology of East African lakes. Gordon and Breach: Amsterdam, 25–56.
- Nsubuga, F.W.N., Olwoch, J.M., and Rautenbach, C.J., 2011. Climatic trends at Namulonge in Uganda: 1947–2009. Journal of Geography and Geology, 3, 119–131. doi:10.5539/jgg.v3n1p119
- Nyenje, P.M. and Batelaan, O., 2009. Estimating the effects of climate change on groundwater recharge and baseflow in the upper Ssezibwa catchment, Uganda. Hydrological Sciences-Journal, 54 (4), 713–726. doi:10.1623/hysj.54.4.713
- Ogallo, L.A., 1993. Dynamics of the East African climate. Proceedings of Indian Academy of Science (Earth Planetary Sciences), 102 (1), 203–217.
- Ogallo, L.J., 1988. Relationships between seasonal rainfall in East Africa and the southern oscillation. International Journal of Climatology, 8, 31–43. doi:10.1002/joc.3370080104
- Partal, T. and Kahya, E., 2006. Trend analysis in Turkish precipitation data. Hydrological Processes, 20, 2011–2026. doi:10.1002/hyp.5993
- Patra, J.P., et al., 2011. Detecting rainfall trends in twentieth century (1871–2006) over Orissa State, India. Climatic Change. doi:10.1007/s10584-011-0215-5
- Phillips, J. and Mcintyre, B., 2000. Enso and inter-annual rainfall variability in Uganda: implications for agricultural management. International Journal of Climatology, 20, 171–182. doi:10.1002/(SICI)1097-0088(200002)20:2<171::AID-JOC471>3.0.CO;2-O
- Rai, R.K., Upadhyay, A., and Ojha, C.S.P., 2010. Temporal variability of climatic parameters of Yamuna river basin: spatial analysis of persistence, trend and periodicity. The Open Hydrology Journal, 4, 184–210. doi:10.2174/1874378101004010184
- Shahid, S. and Khairulmaini, O.S., 2009. Spatio-temporal variability of rainfall over Bangladesh during the time period 1969–2003. Asia-Pacific of Atmospheric Sciences, 45 (3), 375–389.
- Sinoga, J.D.R., et al., 2011. Precipitation dynamics in southern Spain: trends and cycles. International Journal of Climatology, 31, 2281–2289. doi:10.1002/joc.2235
- Türkeş, M., 1996. Spatial and temporal analysis of annual rainfall variations in Turkey. International Journal of Climatology, 16, 1057–1076. doi:10.1002/(SICI)1097-0088(199609)16:9<1057::AID-JOC75>3.0.CO;2-D
- Yue, S., et al., 2002. The influence of autocorrelation on the ability to detect trend in hydrological series. Hydrological Processes, 16, 1807–1829. doi:10.1002/hyp.1095
- Yue, S. and Hashino, M., 2003. Long-term trends of annual and monthly precipitation in Japan. Journal of the American Water Resources Association (JAWRA), 39 (3), 587–596. doi:10.1111/j.1752-1688.2003.tb03677.x
- Zhang, X., et al., 2000. Temperature and precipitation trends in Canada during the 20th century. Atmosphere-Ocean, 38, 395–429. doi:10.1080/07055900.2000.9649654
- Zhao, C., et al., 2011. An analyses of long-term precipitation variability based on entropy over Xinjiang, north western China. Hydrology and Earth System Sciences Discussions, 8, 2975–2999. doi:10.5194/hessd-8-2975-2011