Abstract
This study describes a procedure for investigating climate change impacts on reservoir inflows and subsequent hydroelectric power generation (HPG) for cascaded hydropower plants. By integrating a weather generator, an HBV-based hydrological model and an HPG simulation model, both the reservoir inflows and subsequent HPG amounts during the baseline period (1980–1999) and the future period (2020–2039) were simulated and compared. The downscaled rainfalls and temperatures under the A1B and B1 emissions scenarios from seven general circulation models (GCMs) were used to generate the reservoir inflows. The simulated seasonal inflows, averaged by the multi-model ensemble approach for the seven GCMs, tend to decrease in the future. Under the A1B scenario, the percentage change in HPG for the seven GCMs ranges between −14.9% and 4.6% during the May–October wet season and between −33.1% and 0.2% during the November–April dry season. Under the B1 scenario, the corresponding values are −19.6% to 4.1% and −30.6% to −6.4% during the wet and dry seasons, respectively.
Editor Z.W. Kundzewicz; Associate editor Dawen Yang
Citation Yu, P.-S., Yang, T.-C., Kuo, C.-M., Chou, J.-C., and Tseng, H.-W., 2014. Climate change impacts on reservoir inflows and subsequent hydro-electric power generation for cascaded hydropower plants. Hydrological Sciences Journal, 59 (6), 1196–1212. http://dx.doi.org/10.1080/02626667.2014.912035.
Résumé
Cette étude vise à proposer une procédure d’enquête sur les impacts du changement climatique sur l’alimentation d’un réservoir et la production d’énergie hydro-électrique (PEH) correspondante par des centrales hydroélectriques en cascade. En intégrant un générateur de temps, un modèle hydrologique basé sur HBV et un modèle de simulation de PEH, on a pu simuler et comparer l’alimentation d’un réservoir et les niveaux correspondants de PEH au cours d’une période de référence (1980–1999) et d’une période future (2020–2039). Pour générer l’alimentation du réservoir nous avons utilisé des pluies et des températures issues de la désagrégation des résultats de sept modèles de circulation générale (MCG) simulant les scénarios d’émission A1B et B1. Les apports saisonniers simulés, moyennés sur les sept modèles de circulation générale par une approche d’ensemble multi-modèle, ont tendance à diminuer dans le futur. Selon le scénario A1B, la variation en pourcentage de la PEH pour les sept MCG se situe entre –14,9 et 4,6% au cours de la saison des pluies (mai à octobre), et entre –33,1 et 0,2% au cours de la saison sèche (novembre à avril). Dans le cadre du scénario B1, les valeurs correspondantes sont respectivement de ‑30,6 à –6,4% et de –19,6 à 4,1%.
1 INTRODUCTION
Hydroelectric power is the largest provider of renewable energy in the world. Many electric utilities use small reservoirs in mountainous regions to generate hydroelectric power to meet peak energy demands. Water input from a reservoir for hydroelectric power generation (HPG) depends on the water budget of the reservoir catchment. Hence, reservoir performance for HPG depends on climatic factors and is sensitive to climate change (Robinson Citation1997). According to the Fourth Assessment Report (AR4) of the Intergovernmental Panel on Climate Change (IPCC Citation2007), most of the observed temperature increase since the middle of the 20th century was caused by increasing concentrations of greenhouse gases. The global warming will cause sea levels to rise and change the amount and pattern of precipitation, affecting the global water budget. Under the possible threat of climate change, the power authorities need to face the variation of HPG due to the change of water budgets in catchments.
Hydroelectricity’s low cost, near-zero emissions and ability to quickly meet peak loads are particularly valuable. Therefore, close attention is being paid to the issue of the impact of climate change on HPG. Many studies have addressed the effects of climate change on runoff and subsequent HPG in different regions of the world. Recently, for example, Markoff and Cullen (Citation2008) evaluated the impacts of hydrological regime changes on hydropower at the installations of the Pacific Northwest Power and Conservation Council in the USA. The main result of their study is that hydropower would decrease for the majority of climatic projections. Madani and Lund (Citation2010) evaluated the impact of climate warming on California’s high-elevation hydropower systems under different climate warming scenarios. Their results showed that generation decreased by 1.3% and 19.7% under a warming-only scenario and a dry warming scenario, respectively. HP generation under the wet warming scenario increased by 5.8%. Koch et al. (Citation2011) analysed the future development of HPG in detail for the mountainous Upper Danube basin in Central Europe. To cover the range of uncertainty, 16 climate scenarios, based on the A1B scenario and four regional climate trends, were proposed. The results showed a slight to severe decline in HPG. Minville et al. (Citation2009) evaluated the impacts of and adaptation to climate change of the water resource management system of the Peribonka River, a Canadian water resource system exploited for hydropower. The principal conclusion was that the hydropower would increase over the long term for the climate projection used. Schaefli et al. (Citation2007) showed a decline in runoff and subsequent HPG for the time period 2070–2099 in a single, highly glaciated catchment of a reservoir power plant in Switzerland, which was mainly triggered by a decrease in precipitation, glacier retreat and an increase in evapotranspiration. Further, Stanzel and Nachtnebel (Citation2010) estimated the changes of the water balance and attributed HPG for the entire Austrian territory. Due to climate change impacts on seasonal water availability and runoff regimes, an increase in HPG was observed in the winter, whereas a decrease was found in the summer.
The Dajia River basin, located in north-central Taiwan, has rich hydraulic power resources in which the cascaded hydropower plants (including reservoirs and power plants) have been set for HPG. The HPG amount in the basin approximates 41% of total HPG in Taiwan. Therefore, the basin plays a very important role in HPG in Taiwan. This study proposes a procedure for investigating climate change impacts on reservoir inflows and subsequent HPG for cascaded hydropower plants. A case study was performed in the Dajia River basin. The calibration and validation of an HBV-based hydrological model in the reservoir catchment were first conducted. For simulating the future HPG, the operation rules for reservoirs during the future period have to be decided. As at present, the reservoir operation strategy for each hydropower plant in the study area relies on the judgement of the operators’ experience, finding the “representative” operation rules and adopting them to simulate the future HPG are necessary. Therefore, by using observed reservoir inflows and HPG data, the study proposed an optimization approach to find the representative operation rules which can stand for the operators’ experience of reservoir operation during the baseline period. Then, the representative operation rules were adopted for simulating the future HPG under the assumption of no change in operation modes. Further, by integrating the weather generator, the HBV-based hydrological model, and the HPG simulation model, both the reservoir inflows and HPG amounts during the baseline (1980–1999) and the future (2020–2039) periods, were simulated and compared for assessing the climate change impacts on them.
The remainder of this paper is organized as follows. Section 2 provides a summary of the study area and the data set. Section 3 describes all the models used in the study, including the weather generator, HBV-based hydrological model and HPG simulation model. Section 4 proposes the optimization approach for finding the representative operation rules which can stand for the operators’ experience of reservoir operation. Then Section 5 covers the calibration and validation of the HBV-based hydrological model for the reservoir catchment; climate change impacts on rainfall, temperature and reservoir inflow; and climate change impact on HPG. The conclusions also consider the need for future work.
2 STUDY AREA AND DATA SET
Dajia River basin, located in north-central Taiwan, encloses an area of 1235.73 km2. Due to the upstream steep terrain (average gradient 2.6%), the basin is rich in hydraulic power resources. In the basin, the cascaded hydropower plants (including reservoirs and power plants) have been set and operated for water supply and HPG; they include the Deji, Qingshan, Guguan, Tianlun and Ma’an reservoirs and their power plants ().
Daily observed hydrological data (including rainfall, temperature and inflow) recorded continuously from 1975 to 1996 and from 1997 to 2007, respectively, were used as the data set for calibration and validation of the hydrological model in the catchment of Deji Reservoir. shows the locations of raingauges and meteorological stations in the catchment of Deji Reservoir. The daily rainfall data were collected from the nine raingauges of the Taiwan Power Company and used to compute areal precipitation over the reservoir catchment using the Thiessen polygon method. The daily temperature data were collected from the meteorological stations of the Central Weather Bureau.
Fig. 2 The raingauges and meteorological stations in the catchment of Deji Reservoir (•: Taiwan Power Company raingauges; Δ: Central Weather Bureau meteorological stations).
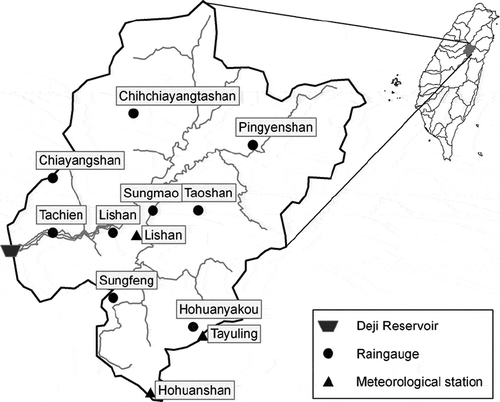
For assessing climate change impacts, the IPCC AR4 (IPCC Citation2007) set a baseline period from 1980 to 1999 as the benchmark. In this study, the same baseline period (1980–1999) is used, and the future period is set to a near-term period from 2020 to 2039. The Taiwan Climate Change Projection and Information Platform Project (TCCIP) (National Science Council of Taiwan Citation2010) provides downscaling projections of monthly rainfall and monthly mean temperature from 24 general circulation models (GCMs) for each node of a 25 km × 25 km grid (covering Taiwan) under A2 (high emissions), A1B (moderate emissions) and B1 (low emissions) scenarios. The downscaling projections for both the baseline and future periods have been bias corrected. Also, for each GCM, each grid node and each month, the change rate (%) of monthly rainfall and the change value (in degree Celsius) of monthly mean temperature from the baseline period to the future period are provided. Seven GCMs were used in this work, and are reported to properly consider the tropical cyclone information and East Asian Monsoon modelling as mentioned in the study of Chu and Yu (Citation2010). The seven GCMs are listed in . The A1B and B1 emissions scenarios were chosen for analysis because the change rates of monthly rainfall and change values of monthly mean temperature are available for all seven GCMs. shows the change rates of monthly rainfall and change values of monthly mean temperature for the seven GCMs on the grid node nearest to the study area and reveals the differences in the results projected by different GCMs. The aforementioned change rates of monthly rainfall and change values of monthly mean temperature from the seven GCMs under A1B and B1 emissions scenarios were used for generating the daily rainfalls and daily mean temperatures with the weather generator for the future period.
Fig. 3 (a) Change rates of monthly rainfall, and (b) change values of monthly mean temperature for the seven GCMs under the A1B and B1 emissions scenarios.
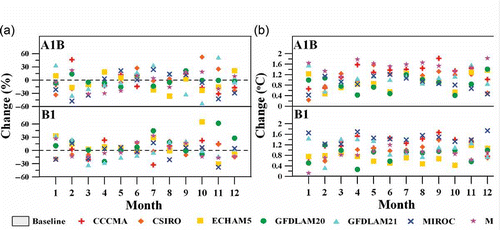
Table 1 GCMs adopted in the study (IPCC Citation2007).
3 METHODOLOGIES
This study investigates the impact of climate change on reservoir inflows and subsequent HPG for the hydropower plants in the study area. By integrating the weather generator, hydrological model and HPG simulation model, both the reservoir inflows and HPG amounts during the baseline and future periods were generated and compared so as to assess the impact of climate change on them. The following sections describe the models used in the study.
3.1 Weather generator
The daily precipitation data generation is based on procedures proposed by Richardson (Citation1981). The generator uses a Markov chain to model the occurrence of wet or dry days, and then uses a probability distribution to generate the precipitation amount conditional on a wet day modelled by the Markov chain. A first-order two-state Markov chain was used in this work. The occurrence of a dry or wet day is modelled by a transition probability matrix consisting of conditional probabilities, given a previous dry or wet day. Many probability distributions have been applied to generate daily precipitation amount, such as the exponential distribution, Weibull distribution, two-parameter gamma distribution and mixed exponential distribution. Among the probability distributions, the Weibull distribution most appropriately approximates the distribution of daily rainfall in Taiwan (Yu et al. Citation2002). Consequently, this work used the Weibull distribution to generate daily rainfalls.
Regarding the daily temperature generation, a first-order autoregressive model was utilized to generate the daily temperature sequences in each month. This daily temperature generation model is expressed as follows:
where T(t,m) is the temperature (°C) on day t in month m; μ(m) is the mean temperature in month m; ρ1(m) is the lag-one autocorrelation coefficient of daily temperature in month m; σ(m) is the standard deviation of daily temperature in month m; and ν is the random standard normal variate. Given the parameters μ(m), σ(m) and ρ1(m), a daily temperature sequence in a month can be generated by this model. For application to the future period, the two parameters in the weather generator (i.e. mean of the Weibull distribution and μ(m) in equation (1)) can be calculated by using the change rates and the change values provided by TCCIP as described in Section 2. Thus the rainfall and temperature series during the future period can be generated.
3.2 Hydrological model
A lumped, continuous hydrological model was used to simulate daily reservoir inflows, after the daily rainfalls and temperatures were generated by the weather generator. This work used a lumped and continuous hydrological model based on the structure of the Hydrologiska Byråns Vattenbalansavdelning (HBV) hydrological model (Bergström Citation1976, Citation1992), which was initially designed for use in Scandinavian catchments by the Swedish Meteorological and Hydrological Institute. Yu and Yang (Citation2000) adapted the HBV hydrological model structure to suit the catchments in Taiwan. The HBV-based hydrological model uses both upper and lower tanks to model the rainfall–runoff behaviour. The model structure has three main parts: (a) soil moisture module, (b) runoff response mechanism and (c) water balance functions. The model calibrated parameters include FC, β, LP/FC, PERC, UZL, K0, K1, K2 and Ce. Detailed description of the HBV-based hydrological model (including model structure and calibrated parameters), as well as its procedures for calibration and validation in this work, can be found in Yu and Yang (Citation2000) and Yu et al. (Citation2002).
In the HBV-based hydrological model, one of the simplest ways to estimate potential evapotranspiration, Hamon’s temperature-dependent equation (Hamon Citation1961), was used to transform the daily temperature series into the daily potential evapotranspiration series. The Hamon equation is simple and does not include the effects of humidity, wind speed and land cover on potential evapotranspiration. Because it only requires inputs of temperature and sunshine duration, it can be widely applied in both time and space. Additionally, although conceptually simple, the Hamon equation has been evaluated and compared with a number of other models and is considered to provide reliable potential evapotranspiration estimates (Federer et al. Citation1996, Vörösmarty et al. Citation1998, Legates and McCabe Citation2005, Lu et al. Citation2005). Hamon’s temperature-dependent equation is:
where Ep(t) is the potential evapotranspiration (mm/d) on day t; H(t) is the sunshine duration (h) on day t; e0(t) is the saturated vapour pressure (millibar) on day t; T(t) is the mean temperature (°C) on day t. The e0(t) can be estimated by the following empirical equation (Bosen Citation1960):
The input variables 5 and 7, of the HBV-based hydrological model, include daily rainfall and daily potential evapotranspiration. Daily potential evapotranspiration can be estimated by using daily temperature and daily sunshine duration as the input to equations (2) and (3).
3.3 Simulation model for HPG
For assessing the climate change impact on HPG in Dajia River basin, the study developed an HPG simulation model for the cascaded reservoirs in the basin and their power plants. The hypothesized conditions for simulation are: (a) the simulation is at daily scale; (b) the water of dead storage for each reservoir is not used for HPG; (c) the initial storage of each reservoir is set to a maximum value (i.e. the reservoir capacity); and (d) the HPG system is assumed to operate normally during the simulation process.
3.3.1 Simulation model of reservoir operation
The daily inflow time series are routed through a reservoir, which can be described by the following continuity equation, equations (4)–(6). The equation considers the inflow, draft, evaporation and storage of the reservoir at each time step:
where V(t + 1) is the storage of reservoir at time t + 1; V(t) is the storage of reservoir at time t; I(t) and e(t), respectively, represent the inflow and the evaporation loss for the reservoir at time t; O(t) is the draft from the reservoir for water uses (including public and agricultural water uses) and HPG at time t; q(t) is the spill at time t; and Vmax is the storage capacity of the reservoir. The evaporation loss from the reservoir is defined by the area of water surface multiplied by the evaporation per unit area of water surface, and the value of evaporation per unit area of water surface can be obtained from evaporation pan observation data at the nearby meteorological station.
For performing the simulation of reservoir operation, the elevation–area–storage relationships for the five reservoirs (Deji, Qingshan, Guguan, Tianlun and Ma’an) and the downstream water demands (including public and agricultural water demands) were collected. The public water demand is around 850 000 m3 d-1 and the agricultural water demand varies from 100 000 to 1 500 000 m3 d-1 in different plant growing seasons.
3.3.2 Calculation of HPG
For calculating the HPG amount, the study collected the energy conversion factor of discharge (ECFOD) provided by Taiwan Power Company for each power plant. The ECFOD is defined as the HPG amount generated by a unit discharge at a given water level. The HPG amount can be easily calculated from the discharge for HPG and the ECFOD as follows:
where E is the HPG amount (megawatt hour, MWh), Qpower is the discharge for HPG (m3 s-1), and R(h) (MWh/( m3 s-1)) denotes the ECFOD for the water level at elevation h (m).
4 RESERVOIR OPERATION STRATEGY
For simulating the future HPG, the operation rules for reservoirs during the future period have to be decided. At the present time, the reservoir operation strategy for each hydropower plant relies on the judgement of the operators’ experience. Therefore, the study used an optimization approach to find the representative operation rules which can stand for the operators’ experience of reservoir operation during the baseline period, and then adopted the representative operation rules for simulating the future HPG under the assumption of no change in operation modes.
Presently, the draft amount of Deji Reservoir for HPG is decided by the Control Center of Taiwan Power Company (TPC). The daily operation rule is based on the fact that the draft is mainly for satisfying the downstream demand if the water level is lower than the operation rule curve (); if the water level is higher than the operation rule curve, the draft is decided partly to satisfy the downstream demand and partly for HPG by the Control Center of TPC referring to the hydro-information (i.e. present water levels and rainfall forecasts by the Central Weather Bureau, Taiwan). When the operators receive the draft instruction for Deji Reservoir from the TPC Control Center, the operators have to monitor and control the water level of the reservoir within the range of the operation water level, and allocate the total required HPG amount to each of the five hydropower plants. Therefore, the reservoir operation strategy for each hydropower plant presently relies on the judgement of the experienced operators. Moreover, among the five reservoirs in Dajia River Basin, only a simple operation rule curve can be found in the literature for Deji Reservoir (as illustrated in ), and the other four reservoirs do not have any operation rule curves. To assess the impact of climate change on HPG, the representative operation rules for each reservoir in Dajia River basin are required and have to be decided. Therefore, the historical inflows of each reservoir were used to calibrate the representative operation rules for each reservoir.
Since the HPG in Dajia River basin is mainly dominated by the operation of Deji Reservoir, the study first tried to decide the representative operation rules of Deji Reservoir according to the optimization approach in Section 4.1. Based on the optimized operation rules for Deji Reservoir, the operation rules for the other reservoirs were further calibrated in Section 4.2.
4.1 Operation strategy of Deji Reservoir
The operation strategy of Deji Reservoir is based on the following principles: If the water level is higher than the operation rule curve, the draft is decided partly for satisfying the downstream demand and partly for HPG; if the water level is lower than the operation rule curve, the draft is mainly for meeting the downstream demand. Based on these principles, some assumptions are made for constructing the operation strategies. The observed inflows of Deji Reservoir during the period 1975–2007 were used to calibrate its operation rules. The simulated mean monthly HPG for each month was calculated and compared with the observed value by using the performance indices, i.e. mean absolute percentage error (MAPE) and root mean squared error (RMSE). Based on the performance indices, different cases of the operation strategy were compared to find the optimal one. The MAPE and RMSE are calculated as follows:
where Eobs(m) is the observed mean monthly HPG amount (MWh) in month m, and Esim(m) is the simulated mean monthly HPG amount (MWh) in month m.
The operation strategy based on the operation rule curve of Deji Reservoir () was proposed first, called the “Case I operation strategy”, it has three rules:
Rule 1 The maximum operating water level (elevation) of the reservoir is 1408 m.
Rule 2 If the water level is higher than the operation rule curve, the draft is decided to satisfy the downstream demand and the electricity amount generated under the maximum flow rate for 6-h operation.
Rule 3 If the water level is lower than the operation rule curve, the minimum amount between the downstream water demand and the reservoir inflow is selected as the draft. This strategy is based on minimizing the draft for recovering the water level to the operation rule curve.
The study assumes that the reservoir operators obey the Case I operation strategy for HPG. shows the observed and simulated mean monthly HPG amounts for the Case I operation strategy; the simulations for January, February, November and December are close to the observed values. The simulations are overestimated from March to July and underestimated from August to October. In August, there is an especially large bias. The value of MAPE is 13.63%, and the value of RMSE is 5.04 GWh/month (GWh is the abbreviation of Gigawatt hour).
Fig. 5 Observed and simulated mean monthly HPG amounts for: (a) Case I, (b) Case II, and (c) Case III operation strategies.
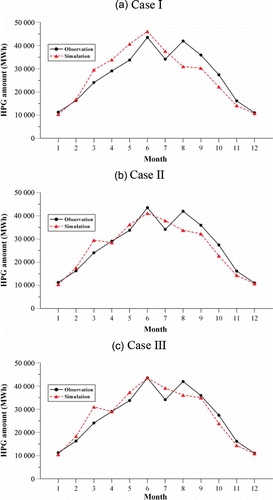
The observed and simulated average monthly mean water levels are shown in to find a way to improve the Case I operation strategy. In , the simulated mean water levels are higher than the operation rule curve during March–July, which makes the draft satisfy the downstream demand and the electricity amount generated under the maximum flow rate for 6-h operation (Rule 2); during August–February, the simulated mean water levels are lower than the operation rule curve, which requires a comparison between the downstream water demand and the reservoir inflow to select the smaller one as the draft (Rule 3). During April–August, and especially in August, the simulated water levels are lower than the operation rule curve, while the observed water levels are higher than the rule curve and remain at a high level of around 1393 m, causing a significant bias between the observed and simulated amounts of HPG shown in . These findings imply that the Case I operation strategy has not been totally obeyed by the operators for HPG.
Fig. 6 Observed and simulated average monthly mean water levels using the Case I operation strategy.
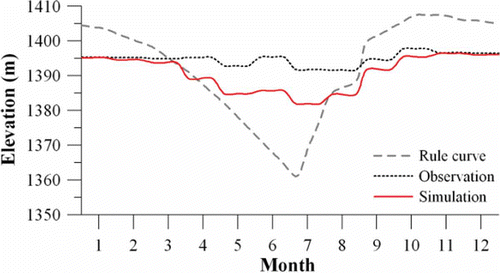
Using the Case I operation strategy for the period October–February, when water levels are lower than the operation rule curve, the simulated water levels are close to observed water levels, which means the operation rule curve is suitable for this period. However, during March–September, when the water levels are higher than the operation rule curve, there are biases between the simulated and observed water levels, meaning the operation rule curve is not suitable for this period and should be modified for improvement.
To improve the Case I operation strategy, the study combined the original operation rule curve and observed mean water levels to form a new operation strategy, the Case II operation strategy, which is shown in . It uses the observed mean water levels as the threshold of operation during March–September and the original operation rule curve as the threshold of operation during the period October–February. The Case II operation strategy has three rules:
Rule 1 The maximum operating water level (elevation) of the reservoir is 1408 m.
Rule 2 If the water level is higher than the threshold of operation, the draft is decided for satisfying the downstream demand and the electricity amount generated under maximum flow rate for 6-h operation.
Rule 3 If the water level is lower than the threshold of operation, the minimum amount between the downstream water demand and the reservoir inflow is selected as the draft. This strategy is based on minimizing the draft to allow recovery of the water level to the threshold of operation.
The study assumes that the reservoir operators obey the Case II operation strategy for HPG; shows the observed and simulated mean monthly HPG. The resulting MAPE is 10.49%, and the RMSE is 3.71 GWh/month, values which are smaller than those obtained using the Case I operation strategy. Hence, the modified operation strategy, Case II, performs better than the Case I operation strategy. The overestimation and underestimation during April–June and August–September, respectively, by the Case I operation strategy have been improved by the Case II operation strategy. The observed and simulated average monthly mean water levels are shown in ; the Case II simulated mean water levels are closer to the observed levels than those derived using the Case I operation strategy.
Fig. 8 Observed and simulated average monthly mean water levels using the Case II operation strategy.
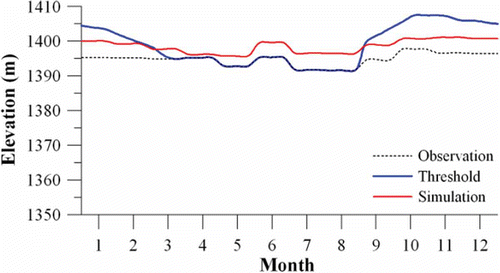
In , during the wet season (June–October), the simulations in June by Case I and by Case II are larger and smaller, respectively, than the observations. In August, the simulation by Case II is closer to the observed values than those by Case I, but the difference between the simulation and the observations is still large and around −19% of the observations. There is need for improvement of Case II during the wet season. The study found that during the wet season the large amount of inflow caused by heavy rainfalls cannot be totally reserved and is spilled from the reservoir, and the Case I and II operation strategies set the spill directly to the downstream, not for HPG. Therefore, the Case III operation strategy considers an additional operation rule of using the spill for HPG. The Case III operation strategy thus has four rules:
Rules 1–3 The same as the Rules 1–3 of Case II.
Rule 4 If spill from the reservoir occurs, the spill amount is used for HPG under maximum flow rate for 2-h operation at most.
The study assumes that the reservoir operators obey the Case III operation strategy for HPG, and shows the observed and simulated mean monthly HPG amounts. The value of MAPE is 9.68%, and the value of RMSE is 3.43 GWh/month; both are smaller than the values using Cases I and II (). From , it is evident that the simulation for each month by Case III is closer to the observation than by Cases I and II. Therefore, the Case III operation strategy is the most representative operation strategy and adopted for the following analysis.
Table 2 Performance criteria (MAPE and RMSE) of different cases of operation strategy for Deji Reservoir.
4.2 Operation strategies for reservoirs downstream of Deji Reservoir
The four reservoirs downstream of Deji Reservoir (Qingshan, Guguan, Tianlun and Ma’an reservoirs) do not have the operation rule curves and complex operation strategies. The actual operation strategy for each one is to maintain the water level close to the operation water level. Therefore, the operation strategy for each reservoir downstream of Deji Reservoir is based on the available storage, Va(t), and the reservoir inflow, I(t), at time t. The available storage at time t is defined as the volume between the initial water level at time t and the minimum operation water level. To make good use of the water in the reservoir for HPG, the following three rules are proposed:
Rule 1 If the available storage, Va(t), plus the reservoir inflow, I(t), at time t is greater than or equal to the water amount of maximum HPG for 6 h (WA6), the draft of the reservoir is the larger value out of the draft of Deji Reservoir (DraftDeji(t)) and WA6:
(10)
Rule 2 If the available storage, Va(t), plus the reservoir inflow, I(t), at time t is less than WA6, the draft of the reservoir is equal to the reservoir inflow, I(t):
(11)
In addition to these two rules, Rule 4 of the Case III operation strategy for Deji Reservoir is adopted here as Rule 3 for the reservoirs downstream of Deji Reservoir.
Rule 3 If the spill of reservoir occurs, the spill amount is used for HPG under maximum flow rate for 2-h operation at most.
–(d) shows the observed and simulated mean monthly HPG amounts for the four reservoirs. The values of MAPE range from 8% to 12%. In , the simulated mean monthly HPG amounts for each month are close to the observations. Therefore, the operation strategy for each of the reservoirs proposed here is representative and can be adopted for the following analysis.
5 IMPACT ASSESSMENT OF CLIMATE CHANGE
This section describes the assessment results of climate change impacts on rainfall, temperature, reservoir inflow and HPG. The calibration and validation results of HBV-based hydrological model in the catchment of Deji Reservoir are first described.
5.1 Calibration and validation of HBV-based hydrological model
The HBV-based hydrological model was applied in the catchment of Deji Reservoir for inflow simulation. The fuzzy multiple objective functions, proposed by Yu and Yang (Citation2000), and the shuffled complex evolution optimization method (Duana et al. Citation1994) were adopted in the study. Thirty-three years of historical daily rainfall, temperature and inflow data were used for model calibration and validation. Data for 1975–1996 were used for model calibration, and the optimal model parameters are listed in . The calibrated HBV-based hydrological model was further verified by the historical data for 1997–2007. To assess the model performance, three criteria, the flow ratio (Rflow, defined as the volume of simulated inflows divided by the volume of observed inflows), RMSE and the coefficient of correlation (CC) between the simulated and observed daily inflows, were calculated for the calibration and validation periods, respectively (). For the calibration period, the values of Rflow, RMSE and CC are 1.10, 3.76 mm d-1 and 0.81, respectively. For the validation period, the corresponding values are 0.84, 8.28 mm d-1 and 0.73, respectively. and (b) illustrates the calibration and verification results for 1996 and 2006. The above results show that the HBV-based hydrological model is able to simulate the rainfall–runoff behaviour over the study area.
Fig. 10 Calibration and verification results of the HBV-based hydrological model: (a) calibration in 1996 and (b) verification in 2006.
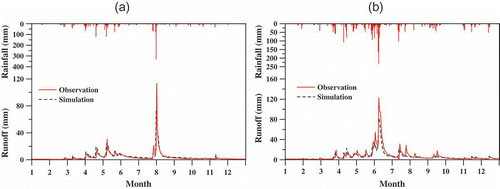
Table 3 Calibrated model parameters of the HBV-based hydrological model for the Deji Reservoir catchment.
Table 4 Performance criteria (Rflow, RMSE and CC) of simulated daily inflows during the calibration and verification periods, respectively, for Deji Reservoir.
5.2 Impacts on rainfall, temperature and inflow
In the study, the baseline period was set to 1980–1999 and the future period to 2020–2039. The observed daily rainfall and temperature data for 1980–1999 were used to calculate the mean monthly rainfall, the average monthly mean temperature and other parameters (e.g. transition probability of daily rainfall and autocorrelation coefficient of daily temperature) for each month. The weather generator adopted these parameters to generate 30 sets of 20-year daily rainfall and temperature series as the data set of baseline generation. Then, the monthly rainfall change rate for each month provided by TCCIP was used to calculate the parameter of the weather generator, the mean of the Weibull distribution, for the future period. For example, in a given month, the mean monthly rainfall during the baseline period multiplied by the change rate is the mean monthly rainfall during the future period which is taken as the parameter, mean of Weibull distribution, for the future period. The change value of monthly mean temperature for each month provided by TCCIP was used to calculate the weather generator parameter μ(m) in equation (1) for the future period. For example, in a given month, the average monthly mean temperature during the baseline period plus the change value is the average monthly mean temperature which is taken as parameter μ(m) in equation (1) for the future period. After calculating these two parameters for each month during the future period under the A1B and B1 emissions scenarios, 30 sets of 20-year daily rainfall and temperature series under the two emissions scenarios were generated as the data set for scenario generation. Using the above simulated rainfalls and temperatures as input, the HBV-based hydrological model was run to generate the reservoir inflows of Deji Reservoir.
The simulated average seasonal mean temperature, mean seasonal rainfall and seasonal mean runoff values for the baseline period and the future period for the seven GCMs under the A1B and B1 emissions scenarios are shown in –(c), respectively. shows that the average seasonal mean temperatures simulated by different GCMs under the A1B emissions scenario are close to the values simulated under the B1 emissions scenarios for the May–October wet season and the November–April dry season. indicates that the simulated average seasonal rainfalls by different GCMs under the A1B and B1 emissions scenarios are close to each other for the two seasons, though for the dry season, the values under B1 are slightly less. In , the simulated average seasonal mean runoffs from different GCMs under the A1B emissions scenario are close to the simulated values under the B1 emissions scenarios for the two seasons. The simulated seasonal inflows, averaged by the approach of multi-model ensemble (MME), tend to decrease in the future. The MME approach is to average the projected results from the seven GCMs. For the dry season, the values under B1 emissions scenarios are slightly smaller than those under the A1B emissions scenario. Since the differences of seasonal impacts between the two emissions scenarios are not obvious, only the A1B emissions scenario was chosen for presenting the monthly impacts for different GCMs.
Fig. 11 Simulated: (a) average seasonal mean temperature, (b) mean seasonal rainfall, (c) average seasonal mean runoff and (d) mean seasonal HPG amount, for the baseline period and the future period using the seven GCMs under A1B and B1 emissions scenarios.
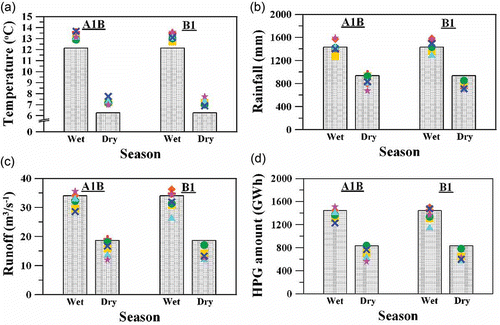
The average monthly mean temperatures for the baseline and future periods simulated by different GCMs with the A1B emissions scenario, and the MME results are shown in . This shows that the increases of simulated average monthly mean temperatures by different GCMs are close and range from 0°C to 2°C. The simulated mean monthly rainfalls for the baseline period and the future period by different GCMs under the A1B emission scenario are shown in . The MME results show that the mean monthly rainfalls tend to decrease from the baseline period to the future period for most of months of the year except June and September. The change rates of monthly rainfall and the change values of monthly mean temperature calculated from the generated data have similar values to those provided by TCCIP, which means the data generated by the weather generator can reasonably reflect the change in rainfall and temperature.
Fig. 12 Simulated average monthly mean temperatures for the baseline and future periods by different GCMs and MME under the A1B emissions scenario.
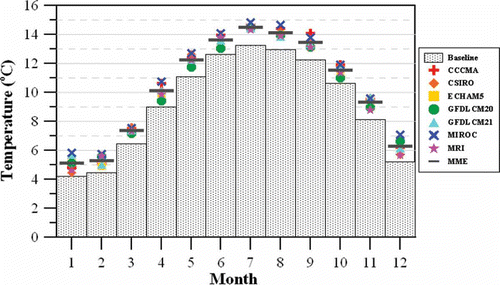
Fig. 13 Simulated mean monthly rainfalls for the baseline and future periods by different GCMs and MME under the A1B emissions scenario.
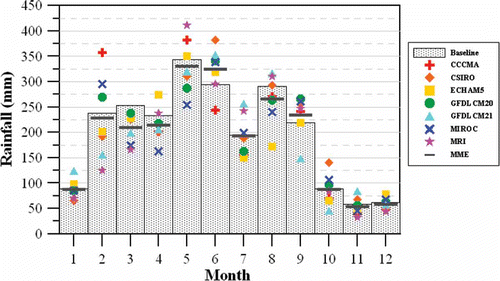
Fig. 14 Simulated average monthly mean inflows for the baseline and future periods by different GCMs and MME under the A1B emissions scenario.
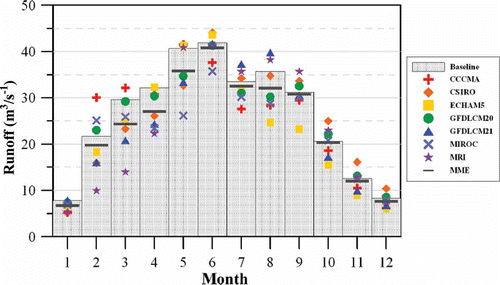
shows the average monthly mean inflows for the baseline and future periods. The results show that the average monthly mean inflows by the seven GCMs have an uncertain trend, which may increase or decrease from the baseline period to the future period. The MME results show that the average monthly mean inflows tend to decrease from the baseline period to the future period; obvious decreases can be found in March, April, May and August.
5.3 Impact on HPG
For assessing the climate change impact on HPG in the Dajia basin, the study used the above generated inflows for the baseline and future periods as the input to the HPG simulation model for calculating the HPG amounts during the baseline and future periods.
The simulated mean seasonal HPG amounts for the baseline period and the future period for the seven GCMs under the A1B and B1 emissions scenarios are shown in . Under A1B, the percentage change in HPG amount for the seven GCMs range between −14.9% and 4.6% (mean: −5.0%) for the wet season and between –33.1% and 0.2% (mean: −12.6%) for the dry season. Under the B1 emissions scenario, the ranges of percentage change in HPG amount for the seven GCMs are −19.6% to 4.1% (mean: −5.1%) for the wet season and −30.6% to −6.4% (mean: −22.8%) for the dry season. For the wet season, the mean reductions for the seven GCMs under both emissions scenarios (i.e. −5.0% and −5.1%) are very close. The differences of seasonal impacts on HPG between the two emissions scenarios are insignificant for the wet season. For the dry season, the mean reduction (–22.8%) under the B1 emissions scenario is greater than that (–12.6%) under the B1 emissions scenario.
The A1B emissions scenario was chosen for further presenting the monthly impacts on HPG for different GCMs and analysing the uncertainty of HPG caused by the weather-generated data. shows the percentage changes of mean monthly HPG amount for different reservoirs by different GCMs. In , different GCMs have different temporal distributions of percentage change. However, the five reservoirs have similar temporal distributions of percentage change for a given GCM. The study considered the total HPG amount (sum of the amounts of the five reservoirs) for assessing the climate change impact on HPG in the study area. Also, the uncertainties of HPG caused by the weather-generated data for both the baseline and future periods were preliminarily analysed with the 30 sets of 20 years of generated data. For each set, the mean monthly HPG amount was estimated in each month. The 30 sets of data provided 30 values of mean monthly HPG amount, which were used to calculate the mean and standard deviation for the confidence interval estimation in each month. displays the uncertainty intervals of mean monthly HPG amounts for the baseline period and the future period by the seven GCMs for each month. In Fig. 16, the thin and thick lines represent the mean value for the baseline period and the future period, respectively; and the thin dashed and thick dashed lines denote the 90% confidence intervals for the baseline and future periods, respectively. For visual identification, the 90% confidence interval for the baseline period is shaded in grey. From , it is clear that the uncertainty interval for the wet season is larger than that for the dry season for each GCM.
Fig. 15 Percentage change of mean monthly HPG amount projected for different reservoirs by different GCMs under the A1B emissions scenario.
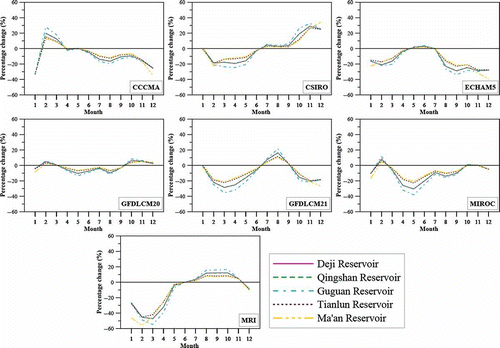
Fig. 16 Uncertainty intervals of mean monthly HPG amounts for the baseline and future periods by different GCMs under the A1B emissions scenario.
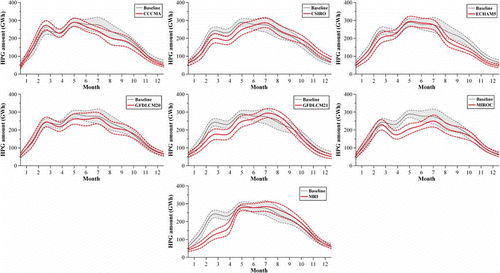
The study adopted hypothesis testing to determine if there is a significant increase/decrease of mean monthly HPG amount from the baseline period to the future period for each month. The null hypothesis (H0) is that the mean monthly HPG amount for the baseline period equals the mean monthly HPG amount for the future period; the alternative hypothesis (Ha) is that the mean monthly HPG amount for the baseline period is not equal to the mean monthly HPG amount for the future period. The z value was used as the test statistic, and the significance level (α) was 0.1. shows the test results, “+” denoting that a significant increase exists, and “–” indicating a significant decrease exists. Significant differences are simulated for most months (at least 10 months) by the six GCMs, except GFDLCM20.
Table 5 Results of hypothesis testing for each month by different GCMs under the A1B emissions scenario.
lists the change rate of mean monthly HPG amount from the baseline period to the future period for each month and each GCM; numbers in grey mean the difference is not significant. The study excluded the insignificant differences in and transformed the table into for visual identification. In , the mean monthly HPG amount tends to decrease from the baseline period to the future period for most months with the seven GCMs. ECHAM5 and MIROC show only a significant increase in one month but significant decreases in most months. The smallest variation of monthly differences through the year is found with GFDLCM20. Because the period of peak load is between June and September, the results of three GCMs (CCCMA, ECHAM5 and MIROC) should be given more attention due to their reductions of HPG amount (by 2–28%) during June–September in the future. The above results suggest that in the future the export of available electricity during the period of peak load may face a big challenge while the HPG amount is reduced.
Fig. 17 Percentage changes of mean monthly HPG amount projected by different GCMs under the A1B emissions scenario.
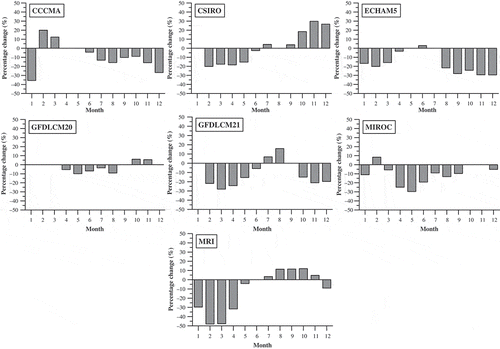
Table 6 Percentage changes (%) of mean monthly HPG amount from the baseline period to the future period for each month by different GCMs under the A1B emissions scenario.
6 CONCLUSIONS
This study proposed a procedure for investigating climate change impacts on reservoir inflows and subsequent HPG for cascaded hydropower plants. A case study was performed in the Dajia River basin, north-central Taiwan. By integrating the weather generator, the HBV-based hydrological model and the HPG simulation model with representative operation rules, the reservoir inflows and subsequent HPG amounts for the baseline period (1980–1999) and the future period (2020–2039) were generated. By comparing the results during the baseline and future periods, the climate change impacts on the reservoir inflows and subsequent HPG amounts were assessed.
The downscaling results under the A1B and B1 emissions scenarios from the seven GCMs that consider tropical cyclone information and East Asian monsoon modelling were used in this work. Thirty sets of 20-year daily rainfall and temperature series during the future period for each GCM and during the baseline period, respectively, were generated and input to the HBV-based hydrological model to generate daily reservoir inflows. The differences of seasonal impacts on reservoir inflow between the two emissions scenarios are not obvious. Under the A1B emissions scenarios, the reservoir inflow simulated by the MME approach shows that average monthly mean inflows tend to decrease from the baseline period to the future period. The most obvious decreases occur in March, April, May and August.
For simulating the future HPG, the operation rules for reservoirs in the future period have to be decided. Therefore, the study proposed an optimization approach to find the representative operation rules which can stand for the operators’ experience of reservoir operation during the baseline period, and adopted the representative operation rules for simulating the future HPG under the assumption of no change in operation modes. Through the HPG simulation model, the daily HPG amounts for the baseline and future periods were generated.
The results of seasonal and annual impacts on HPG show reductions of HPG in the future. Under the A1B emissions scenario, the ranges of percentage change in HPG for the seven GCMs are −14.9% to 4.6% for the wet season and −33.1% to 0.2% for the dry season. Under the B1 emissions scenario, the corresponding percentages are −19.6% to 4.1% and −30.6% to −6.4% for the wet and dry seasons, respectively. For the future period, the annual decreases of HPG amount for the seven GCMs are around 284–65 GWh and 539–156 GWh, respectively, under the A1B and B1 emissions scenarios.
The study adopted hypothesis testing to establish if there is a significant increase/decrease of mean monthly HPG amount from the baseline period to the future period for each month under the A1B emissions scenario. It was found that the mean monthly HPG tends to decrease from the baseline period to the future period for most of months with all seven GCMs. Since the period of peak load is during June to September, the results of the three GCMs (CCCMA, ECHAM5 and MIROC) should be given more attention, as their reductions of HPG are about 2–28% during June–September in the future. The export of available electricity during the period of peak load in the future may face a big challenge.
Because HPG is responsible for providing electricity to satisfy the peak load in a power supply system, if the HPG amount reduces in the future, the peak load deficit cannot be instantly compensated, which may make the power supply system unstable. Especially under climate change with increasing temperature, which may result in the increasing consumption of electricity in summer, the decreasing HPG amount will influence the stability of the power supply system. How to reduce this impact (i.e. the possible reduction of HPG) is an essential question for the power authority in Taiwan. Proposing adaptation strategies for reducing this impact will be an important task for future work. Because this study aimed to propose a procedure for investigating climate change impacts on reservoir inflows and subsequent HPG for cascaded hydropower plants, only the A1B and B1 emissions scenarios during the near future (2020–2039) were used as a case study. Further work may consider more emissions scenarios during the mid- to long-term future periods and compare their results for detecting the climate change impacts on reservoir inflow and subsequently under different emissions scenarios during different future periods.
Acknowledgement
The authors would like to thank the Taiwan Climate Change Projection and Information Platform Project (TCCIP) of the National Science Council for offering future precipitation and temperature projections.
Funding
The National Science Council of the Republic of China (Taiwan) financially supported this research under Contract no. NSC 97-2221-E-006-150-MY3.
REFERENCES
- Bergström, S., 1992. The HBV model – its structure and applications. Norrköping: SMHI, Reports RHO 4.
- Bergstrom, S., 1976. Development and application of a conceptual runoff model for Scandinavian Catchments. Norrköping: SMHI, Reports RHO 7.
- Bosen, J.F., 1960. A formula for approximation of the saturation vapor pressure over water. Monthly Weather Review, 88 (8), 275–276. doi:10.1175/1520-0493(1960)088<0275:AFFAOT>2.0.CO;2.
- Chu, J.-L. and Yu, P.-S., 2010. A study of the impact of climate change on local precipitation using statistical downscaling. Journal of Geophysical Research, 115, D10105. doi:10.1029/2009JD012357.
- Duana, Q., Sorooshian, S., and Gupta, V.K., 1994. Optimal use of the SCE-UA global optimization method for calibrating watershed models. Journal of Hydrology, 158 (3–4), 265–284. doi:10.1016/0022-1694(94)90057-4.
- Federer, C.A., Vörösmarty, C., and Fekete, B., 1996. Intercomparison of methods for calculating potential evaporation in regional and global water balance models. Water Resources Research, 32, 2315–2321. doi:10.1029/96WR00801.
- Hamon, W.R., 1961. Estimating potential evapotranspiration. Journal of Hydraulics Division, Proceedings of the American Society of Civil Engineers, 87, 107–120.
- IPCC (Intergovernmental Panel on Climate Change), 2007. Climate change 2007: the physical science basis. Fourth Assessment Report of the Intergovernmental Panel on Climate Change. Geneva: IPCC Secretariat.
- Koch, F., et al., 2011. How will hydro-electric power generation develop under climate change scenarios? A case study in the Upper Danube Basin. Energies, 4, 1508–1541. doi:10.3390/en4101508.
- Legates, D.R. and McCabe, G.J., 2005. A re-evaluation of the average annual global water balance. Physical Geography, 26, 467–479. doi:10.2747/0272-3646.26.6.467.
- Lu, J., et al., 2005. A comparison of six potential evapotranspiration methods for regional use in the southeastern United States. Journal of the American Water Resources Association, 41, 621–633. doi:10.1111/j.1752-1688.2005.tb03759.x.
- Madani, K. and Lund, J.R., 2010. Estimated impacts of climate warming on California’s high-elevation hydropower. Climatic Change, 102 (3–4), 521–538. doi:10.1007/s10584-009-9750-8.
- Markoff, M.S. and Cullen, A.C., 2008. Impact of climate change on Pacific Northwest hydropower. Climatic Change, 87 (3–4), 451–469. doi:10.1007/s10584-007-9306-8.
- Minville, M., et al., 2009. Adaptation to climate change in the management of a Canadian water-resources system exploited for hydropower. Water Resources Management, 23, 2965–2986. doi:10.1007/s11269-009-9418-1.
- National Science Council of Taiwan, 2010. Taiwan Climate Change Projection and Information Platform Project (TCCIP) [online]. Available from: http://tccip.ncdr.nat.gov.tw/NCDR/main/index.aspx [Accessed 1 January 2013].
- Richardson, C.W., 1981. Stochastic simulation of daily precipitation, temperature, and solar-radiation. Water Resources Research, 17 (1), 182–190. doi:10.1029/WR017i001p00182.
- Robinson, P.J., 1997. Climate change and hydropower generation. International Journal of Climatology, 17, 983–996. doi:10.1002/(SICI)1097-0088(199707)17:9<983::AID-JOC174>3.0.CO;2-I.
- Schaefli, B., Hingray, B., and Musy, A., 2007. Climate change and hydropower production in the Swiss Alps: quantification of potential impacts and related mo delling uncertainties. Hydrology and Earth System Sciences, 11 (3), 1191–1205. doi:10.5194/hess-11-1191-2007.
- Stanzel, P. and Nachtnebel, H.P., 2010. Mögliche Auswirkungen des Klimawandels auf den Wasserhaushalt und die Wasserkraftnutzung in Österreich. Österreichische Wasser- und Abfallwirtschaft, 62 (9–10), 180–187. doi:10.1007/s00506-010-0234-x.
- Vörösmarty, C.J., Federer, C.A., and Schloss, A.L., 1998. Potential evaporation functions compared on US watersheds: possible implications for global-scale water balance and terrestrial ecosystem modeling. Journal of Hydrology, 207, 147–169. doi:10.1016/S0022-1694(98)00109-7.
- Yu, P.-S. and Yang, T.-C., 2000. Fuzzy multi-objective function for rainfall-runoff model calibration. Journal of Hydrology, 238 (1–2), 1–14. doi:10.1016/S0022-1694(00)00317-6.
- Yu, P.-S., Yang, T.-C., and Wu, C.-K., 2002. Impact of climate change on water resources in southern Taiwan. Journal of Hydrology, 260 (1–4), 161–175. doi:10.1016/S0022-1694(01)00614-X.