Abstract
The concept of “catchment-scale storm velocity” quantifies the rate of storm motion up and down the basin accounting for the interaction between the rainfall space–time variability and the structure of the drainage network. It provides an assessment of the impact of storm motion on flood shape. We evaluate the catchment-scale storm velocity for the 29 August 2003 extreme storm that occurred on the 700 km2-wide Fella River basin in the eastern Italian Alps. The storm was characterized by the high rate of motion of convective cells across the basin. Analysis is carried out for a set of basins that range in area from 8 to 623 km2 to: (a) determine velocity magnitudes for different sub-basins; (b) examine the relationship of velocity with basin scale and (c) assess the impact of storm motion on simulated flood response. Two spatially distributed hydrological models of varying degree of complexity in the representation of the runoff generation processes are used to evaluate the effects of the storm velocity on flood modelling and investigate model dependencies of the results. It is shown that catchment-scale storm velocity has a non-linear dependence on basin scale and generally exhibits rather moderate values, in spite of the strong kinematic characteristics of individual storm elements. Consistently with these observations and for both models, hydrological simulations show that storm motion has an almost negligible effect on the flood response modelling.
Editor Z.W. Kundzewicz; Guest editor R.J. Moore
Citation Nikolopoulos, E.I., Borga, M., Zoccatelli, D., and Anagnostou, E.N., 2014. Catchment-scale storm velocity: quantification, scale dependence and effect on flood response. Hydrological Sciences Journal, 59 (7), 1363–1376. http://dx.doi.org/10.1080/02626667.2014.923889
Résumé
Le concept de « vitesse d’averse à l’échelle du bassin » quantifie le déplacement d’un événement pluvieux à travers un bassin en prenant en compte l’interaction entre la variabilité spatio-temporelle des précipitations et la structure du réseau de drainage. Il fournit une évaluation de l’impact du mouvement de l’événement pluvieux sur la forme de l’hydrogramme de crue. Nous avons évalué la vitesse d’averse à l’échelle du bassin de l’événement extrême du 29 Août 2003 qui a eu lieu sur le bassin versant de la rivière Fella, d’une superficie de 700 km2, dans l’Est des Alpes italiennes. L’évènement a été caractérisé par la grande mobilité des cellules convectives dans le bassin. L’analyse a été effectuée sur un ensemble de bassins dont la superficie va de 8 à 623 km2 afin de: (a) déterminer l’ordre de grandeur de la vitesse pour différents sous-bassins; (b) examiner la relation entre la vitesse et la taille du bassin; et (c) évaluer l’impact du mouvement de l’averse sur la réponse en terme d’hydrogramme de crue simulé. Deux modèles hydrologiques répartis, de différents niveaux de complexité dans la représentation des processus de production d’eaux de ruissellement, ont été utilisés pour évaluer les effets de la vitesse de l’averse sur la modélisation des crues et apprécier la dépendance au modèle des résultats. On a montré que la vitesse de l’averse à l’échelle du bassin versant dépend non linéairement de la taille du bassin et qu’elle présente généralement des valeurs plutôt modérées, en dépit des fortes caractéristiques cinématiques des éléments individuels de l’événement pluvieux. En accord avec ces observations, et pour les deux modèles, les simulations hydrologiques montrent que le mouvement de l’averse a un effet presque négligeable sur la simulation de l’hydrogramme de crue.
1 INTRODUCTION
The temporal and spatial organization of rainfall plays an important role in flood hydrological behaviour. Storm motion and velocity describes a specific feature of space–time rainfall variability. The influence of storm movement on flood hydrographs has been investigated for nearly four decades (Maksimov Citation1964, Niemczynowicz Citation1984, Singh Citation1998, De Lima and Singh Citation2002, Seo et al. Citation2012, among the others). Overall, these investigations have shown that the direction of storm movement might augment or reduce flood peaks and modify the hydrograph shape. A general finding is that, for the same storm duration, the flood peak is greater for storms moving downstream relative to storms moving upstream (Ogden et al. Citation1995, Singh Citation1998, Seo et al. Citation2012). The role of storm movement for short duration, localized floods, like flash floods, is more controversial. Doswell et al. (Citation1996) identified the meteorological root cause of flash flooding as the combination of ample atmospheric moisture and the means of precipitating a large amount of this moisture over a single basin. The means of concentrating this precipitation in a small area include slow cell motion and cell training (Corfidi et al. Citation1996). However, observations from a number of case studies show that flash floods are frequently characterized by near-stationary storm movement, which is expected to have an impact on flood hydrographs. Smith et al. (Citation2000) showed that near-stationary storm motion over a 148-km2 basin for a time period comparable to the maximum travel time for the basin led to an extraordinary flood peak response. Sturdevant-Rees et al. (Citation2001) reported that motion of storm elements played an important role in the magnitude and timing of flood peaks for basin areas between 500 and 1000 km2.
Arguably, accounting for storm movement is important for the prediction of floods (Smith et al. Citation2000, Collier and Fox Citation2003, Moore et al. Citation2006, Collier Citation2007). However, in spite of the long-standing research effort, a methodology for the identification and assessment of the storm motion properties that are important for flood hydrology is still missing. The rainfall properties that need to be considered for a given drainage basin depend on the basin’s drainage network relative to the space–time organization of rainfall. Specifically, for the same storm motion, the impact on the flood hydrograph is expected to depend on the catchment area, on the structure of the drainage system and its directional orientation relative to the main storm movement. For example, the runoff response from a long and narrow basin parallel to the storm motion vector is expected to show more dependence on storm velocity than that from a short and wide basin (Woods and Sivapalan Citation1999, Smith et al. Citation2000).
The aim of this work is to establish the concept of “catchment-scale storm velocity”, which quantifies the rate of storm motion up and down the basin accounting for the interaction between the rainfall space–time variability and the structure of the drainage network. Catchment-scale storm velocity is derived based on the notion of spatial moments of catchment rainfall (Zoccatelli et al. Citation2011). It provides an assessment of the impact of storm motion on flood shape, considering a specific space scale (the catchment size) and time integration scale. Given this background, it is interesting to examine the scale dependency of catchment-scale storm velocity.
Quantification and catchment-scale dependency are examined with reference to an extreme flash flood that occurred over north-eastern Italy in 2003 (Borga et al. Citation2007). Moreover, we introduce a methodology to test the impact of neglecting the storm velocity in flood modelling. To investigate potential model dependency on results, two spatially distributed rainfall–runoff models, of varying complexity in terms of process description and parameter space, are used together with fine-scale rainfall observations to examine the impact of storm motion and velocity on hydrograph simulation at various spatial scales.
The paper is organized as follows: Section 2 provides a description of the study area, data and the flash-flood event under examination. The analytical derivations regarding the definition of catchment-scale storm velocity along with the presentation of the quantification results are presented in Section 3. Section 4 involves the hydrological modelling evaluations used to assess the impact of storm velocity on flood response. Conclusions are summarized and discussed in Section 5.
2 STUDY AREA AND DATA
The basin considered in this study is the Fella River basin, a major left-hand tributary of the Tagliamento River located within the Friuli-Venezia Giulia region of north-eastern Italy (). The Fella River basin at the confluence with the Tagliamento has a drainage area of approximately 700 km2 and a very complex terrain with elevations that range from approximately 300 m a.s.l. close to the outlet to more than 2000 m a.s.l. at the mountain tops. The land cover is dominated by broad-leaf and conifer forests and the area receives on average 1920 mm of precipitation annually (Borga et al. Citation2007). For the purpose of this work, we considered 21 sub-basins of the Fella River system (), with areas ranging between 8 and 623 km2.
Fig. 1 Digital elevation map for the Friuli region showing location of the OSMER radar and outline boundaries of the Fella basin at Moggio.
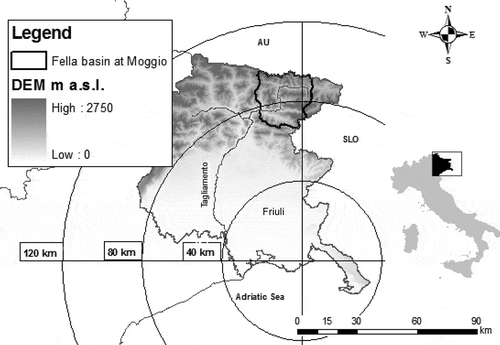
Fig. 2 Left: Map of the Fella basin at Moggio (Basin 11) showing outlet locations of the sub-basins examined in this study. Right: Total rainfall accumulation map over the study area for the 2003 storm event examined.
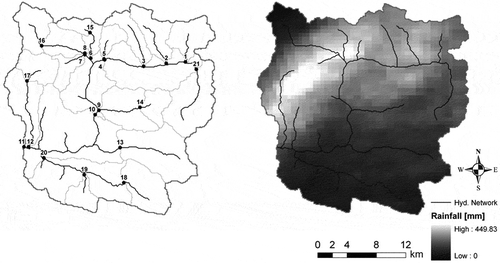
The flash-flood event examined in this study was one of the most devastating floods in north-eastern Italy since the start of systematic observations in the region (Sangati and Borga Citation2009) and resulted in losses of lives and damages close to one billion euro (Tropeano et al. Citation2004) in the area of the Upper Tagliamento River. The flood inducing storm started at 09:00 h coordinated universal time (UTC) during 29 August 2003 and lasted for approximately 12 h. The mesoscale convective system responsible for the flooding exhibited a characteristic persistence of the convective bands over the northern part of the basin that resulted in very large rainfall accumulations and high spatial variability (). In some parts of the basin the 15-min rainfall intensities exceeded 130 mm h-1, while the total rainfall accumulation over the 12-h period exceeded 400 mm. The combined effect of dry initial conditions, due to a prolonged summer drought and the high spatial variability of rainfall caused a highly heterogeneous runoff response with runoff ratios ranging from 0.04 to 0.2 in different parts of the basin (Borga et al. Citation2007). Despite the overall low values of runoff ratio, specific peak discharge exceeded 8 m3 s-1 km-2 in some parts of the basin indicating the severity of flash flooding in those areas. The flood response was flashy, with a lag time (computed as the difference between the time of the centroid of the generating rainfall sequence and the time of the discharge peak) ranging from 1 h for 20 km2 size catchments to 3½ h for the largest basin (623 km2) (Marchi et al. Citation2010).
The precipitation data set utilized in this study includes 15-min rainfall accumulation fields at 0.5-km spatial resolution. Rainfall data were derived from the reflectivity scans of a Doppler, dual-polarized C-band radar (OSMER radar station) located at Fossalon di Grado, approximately 80 km south of the basin (). Radar data were corrected for ground clutter, partial beam blockage, variability of reflectivity along the vertical and attenuation. Partial beam blocking was corrected by using the procedure developed by Pellarin et al. (Citation2002). The methodology described in Borga et al. (Citation2002) was used to adjust for effects of vertical profile of reflectivity, whereas attenuation correction was applied by using the technique reported in Bouilloud et al. (Citation2009) (see Borga et al. Citation2007 for details). Radar rainfall estimates were compared with observations from 15 gauges available within the area (11 of which lie inside the Fella River system). Radar-gauge statistical comparisons of hourly rainfall accumulations showed a generally good agreement, with squared-correlation equal to 0.73 and limited (10%) radar rainfall overestimation (Borga et al. Citation2007). A striking characteristic of the event was its organization in four well-defined banded structures, characterized by lifetimes of 2–3 h. Convective cells, characterized by lifetimes of around 30 min, moved along the convective bands. Storm motion characteristics were analysed based on the temporal sequence of radar images by using two methodologies:
centroid tracking of the storm cells; and
cross-correlation technique over a storm-wide region in two successive radar images.
The two techniques capture the storm at different spatial scales and therefore provide different estimates of storm motion characteristics. The motion of the convective cells through the region was tracked by using the procedure developed by Bacchi et al. (Citation1996). The tracks are reported in for three different periods of the most intense phase of the storm (12:00–18:00 h UTC). The motion vector reported for each cell is obtained from cell locations computed for each volume scan. The velocity magnitude of these storm elements computed by the tracking technique was around 6 m s-1 with peaks of 10 m s-1. The direction of the storm elements motion was generally from southwest to northeast, and was almost parallel to the main drainage line for several sub-basins of the Fella, with a clear up-basin direction. The cross-correlation method (Browning and Collier Citation1989, Wilson et al. Citation1998) was applied over a 40 km by 40 km region centred over the Fella River basin covering the whole storm system. This method provided values of storm velocity which were considerably less intense than those obtained by the tracking technique, with peaks around 3 m s-1, and direction of motion which was almost normal to the vectors obtained by the tracking technique. These results are not unexpected, as several studies investigated the contrasting characteristics of fast-moving convective cells and slow-moving convective systems within the same storm (e.g. Doswell et al. Citation1996, Collier Citation2007, Aylward and Dyer Citation2010). This flash-flood event provides therefore a template for examining how the emerging characteristics of the storm motion transmit to the flood response at various spatial scales.
3 CATCHMENT-SCALE STORM VELOCITY
The term ‘catchment-scale storm velocity’ is based on the concept of ‘spatial moments of catchment rainfall’ presented by Zoccatelli et al. (Citation2011). These statistics, based on the previous work by Viglione et al. (Citation2010) and corresponding in part to the catchment rainfall statistics reported in Smith et al. (Citation2002, Citation2005), provide a metric for the spatial organization of the rainfall in relation to a fundamental descriptor of the structure of the drainage network, i.e. the flow distance. The flow distance is the distance along the runoff flow path from a given point to the outlet. The mathematical formulation of these moments and their relationship to catchment-scale storm velocity is described below.
3.1 Definition
Let the rainfall field value at location x,y over a catchment and at a certain time t, be defined by the function r(x,y,t) [L T-1]. For the flow distance d(x,y) [L] (measured along the flow path) between position x,y and the catchment outlet, the nth spatial moment of catchment rainfall pn [Ln +1 T-1] is defined as:
where A corresponds to catchment area. The 0th-order spatial moment p0(t) is equal to the catchment-averaged rainfall rate at time t. Analogously, the gn [Ln] moments of the flow distance are given by:
and the first-order moment g1 is equal to the catchment-averaged flow distance. By taking the ratio between moments of catchment rainfall (pn) and flow distance (gn), we can obtain a non-dimensional (scaled) form of the spatial moments of catchment rainfall (δn). Equations (3) and (4) provide the formulation of the first two orders:
where δ1 (−) describes the flow distance from the centroid of catchment rainfall with respect to the catchment’s centroid g1; δ2 (−) relates to the dispersion of the rainfall field (relative to its mean position) with respect to the dispersion of the flow distances. For more details on the derivation of δ2 the reader is referred to Zoccatelli et al. (Citation2011). Values of δ1 equal to one mean that the rainfall distribution is either concentrated on the position of the catchment’s centroid or it is uniform over the catchment. Values of δ1 less (greater) than one indicate that rainfall is distributed close to the outlet (the headwater) of the basin. The second scaled moment δ2 (−) relates to the dispersion of the rainfall field (with respect its mean position) relative to the dispersion of the flow distances. Values of δ2 close to 1 reflect a uniform-like rainfall distribution, with values less (greater) than 1 indicating that rainfall is characterized by a unimodal (multimodal) distribution along the flow distance.
Apart from the description of rainfall’s spatial organization at a certain time t, spatial moments of catchment rainfall (pn. and δn) can be extended to reflect the organization of the rainfall over a certain time period (e.g. total storm duration Ts). Equations (5)–(7) present the equivalent form of equations (1), (3) and (4), respectively, when applied over a time period Ts:
Based on the formulation of the spatial moment relationships in equation (3), it is possible to derive an expression for the effective storm velocity as it is filtered by the drainage properties of the catchment. This is obtained as a temporal derivative of the evolution over time of the first-order moment, as shown by Zoccatelli et al. (Citation2011):
Equation (8) shows that the concept of vs takes into account the relative catchment orientation and geometry with respect to storm motion. A positive (negative) value of the catchment-scale storm velocity vs indicates an increase (decrease) over time of the distance from the rainfall centroid to the outlet, and hence up-basin (down-basin) storm movement. In practice, evaluating the effective velocity requires numerical evaluation of the derivative over a specific space and time window. The spatial window is given here by the catchment size, whereas the temporal window is chosen with reference to the problem at hand. In particular, the temporal window should be commensurate with the catchment response time to quantify the catchment-scale storm velocity which may influence the hydrological response (e.g. the hydrograph shape).
Zoccatelli et al. (Citation2011) have shown that the resulting catchment-scale storm velocity needs to consider both the variation over time of the first-order moment and that of the mean areal rainfall. This is obtained by using the rainfall weights w(t), defined as follows:
According to this and based on the definition of the moment δ1, the catchment-scale storm velocity (Vs) is defined as:
where T is time, covt[] denotes the temporal covariance and var[] denotes the variance.
The term var[T] denotes the variance of the uniform variable time T over the duration considered for the computation of the velocity Vs. For a time duration equal to Ts, var[T] is computed as (1/12) Ts2.
Equation (10) shows that the storm velocity is defined as the difference between the slope terms of two linear regressions with time (Zoccatelli et al. Citation2011). The first slope term is estimated based on the space–time regression between weighted scaled first moments and time, and the second term is based on the regression between weights and time. Conceptually, this means that storm motion may produce changes both in the rainfall centroid coordinate and in the mean areal rainfall values. Both are taken into account in the estimation of the catchment-scale storm velocity. For the case of temporally uniform mean areal rainfall, w(t) is constant and the value of Vs depends only on the evolution in time of the position of the rainfall centroid along the flow distance coordinate. In the opposite case, if there is only temporal variation of the mean areal rainfall and no motion (δ1(t) is constant), the two slope terms will be equal in value and opposite in sign, which means that the Vs will be equal to zero. Note that the sign of the velocity is positive (negative) for the case of upstream (downstream) storm motion.
3.2 Quantification of catchment-scale storm velocity and scale dependence
reports the time series of the basin-averaged rainfall, basin coverage with rain >20 mm h-1, coefficient of variation (standard deviation divided by the mean) of rainfall rate (for positive bins) and the spatial moments δ1 and δ2. Time series are shown for three basins of varying size: Basin 9 (46 km2), Basin 10 (329 km2) and Basin 11 (623 km2, corresponding to the largest basin examined in this study). The results in highlight the high variability of the storm properties with time. Flood-producing rainfall for Basins 9 and 10 was concentrated over a period of 7.5 h, from 10:30 to 18:00 h UTC. Four peak values of basin-averaged rainfall can be recognized for Basins 9 and 10, at 11:00, 13:00, 14:30 and 16:00 h. Correspondingly, the peaks of fractional coverage of heavy rainfall (greater than 20 mm h-1) are up to 90% for Basin 9 and up to 75% for Basin 11. The coefficient of variation ranges steadily between 0.7 and 1.8 over the periods of intense rainfall; these values correspond to those reported for other extreme flash-flood-inducing storms observed in northern Italy (Sangati et al. Citation2009).
Fig. 4 Time series (15 min) showing (from top to bottom) the basin-averaged rainfall, the fraction of basin area covered by rain >20 mm h-1, coefficient of variation of non-zero rain-rates, δ1 and δ2. Each column corresponds to a different basin.
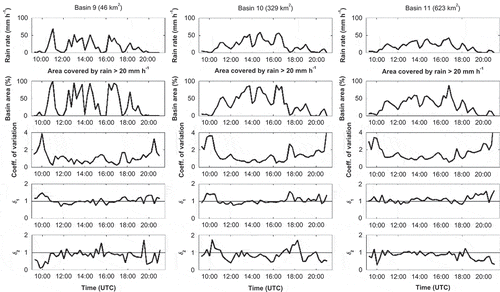
Despite the large rainfall variability, the fluctuations of δ1 remained close to 1 for most of the time, particularly during the period of high rain intensity, suggesting that the rainfall centroid was close to the catchment centroid. The slight fluctuation of δ1 around 1 means that the rainfall centroid was oscillating (up/down) with respect to the catchment centroid thus resulting in fluctuation in the direction of catchment-scale storm velocity (up-basin/down-basin). The temporal variation of rainfall dispersion (δ2) was more distinct with the majority of the values below 1, suggesting spatial concentration over the catchment centroid. Few instances, characterized by low rain rate, show values exceeding 1 (suggesting multimodal distribution).
To examine the characteristics of storm velocity distributions, the analysis was extended to the 21 basins indicated in . We used two time windows to compute the storm velocity:
One hour (the corresponding storm velocity is indicated with V), and
the mean catchment response time (the corresponding storm velocity is indicated with Vs) with values that ranged from 0.75 to 3.5 h for the scales examined.
Note that the 1-h window was chosen as a compromise between a minimal duration which permits the computation of the velocity (given the 15-min resolution of radar data used) and a duration which permits capture of the time variability of the velocity itself. The mean response time for each basin was derived based on the application of the propagation module of the kinematic local excess model (KLEM) model (see Section 4.1 below for details). While we consider Vs as the hydrologically relevant velocity, V corresponds to the storm motion kinematics as filtered by catchment morphological properties. We believe that both velocity values are important and each delivers complementary information: thus we present our subsequent analysis based on both. The temporal distributions of velocity values V and Vs for all basins are presented in and , respectively, as box plots ordered in increasing basin area. One distinct feature derived from the figure is the symmetry in the distribution with respect to the sign of the velocities, which suggests the frequent alternation of the velocity direction (upstream/downstream). Results from show that values for V range from 0 to 5.5 m s-1, with several values exceeding 1 m s-1; moreover, the mean absolute values of V increase with increasing basin size. On the other hand, values for Vs are below 1 m s-1 for almost all cases and no relationship with basin size is apparent. This suggests that, while the rainfall motion effect may be significant over an hourly time window (high V value), this does not hold for generally longer times (most of the 21 basins are associated with a response time greater than 1 h) associated with calculation of Vs.
Fig. 5 Boxplots showing the distribution of catchment-scale storm velocity for each sub-basin vs basin area. Values are: (a) based on using a fixed regression window of 1 h; and (b) calculated using a variable regression window based on the mean response time for each basin. Open circles correspond to values that exceed 1.5 times the interquartile range of the distribution for each case.
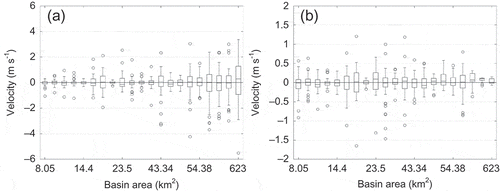
To investigate the features of scale dependency of catchment-scale storm velocity, we analysed the mean absolute values of velocity V and Vs for all 21 basins examined ( and , respectively). As shown in , the magnitude of velocity V exhibits a strong linear dependence (correlation coeff. >0.8) with the logarithm of basin area, suggesting the existence of a logarithmic relationship between velocity and basin scale. This indicates that storm velocity increases non-linearly with basin scale. This dependence is almost completely explained by the structure of the estimator (equation (10)), with a multiplier (g1) which depends on catchment size in accordance with Hack’s law (Rigon et al. Citation1996). The covariance terms, however, show a negligible dependence on catchment size (results not reported here for the sake of brevity). This lack of dependence is discussed in detail by Zoccatelli (Citation2013) in the frame of an investigation of the planar flow owing to storms moving up and down the plane.
Fig. 6 Mean absolute storm velocity vs basin area: (a) average velocity, V, calculated based on a fixed hourly regression window; and (b) averaged Vs values based on a variable time window equal to the basin’s mean response time.
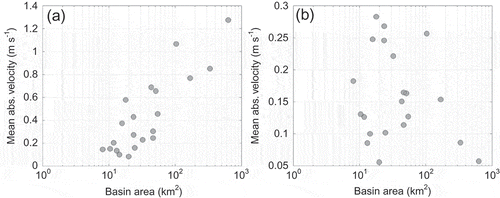
In contrast, the results for velocity Vs () show no clear scale dependence. More specifically, low values of velocity are found for very small catchments and for large basins. The distribution of Vs shows a peak around 0.3 m s-1 in the range 15–100 km2. Hence, the distributions reported in and differ essentially for the case of relatively large basins. This is likely to be due to the high temporal variability of velocity V. When a temporal window >1 h is used for the computation of the catchment-scale velocity Vs, as is the case for medium- and large-sized basins characterized by a longer response time, this results in a smoothing of the velocity magnitudes with a strong reduction of their values. Overall, results from this analysis indicate that the velocity values relevant to hydrological response times are low, suggesting that catchment rainfall storm velocity was not significant during the 2003 flash-flood event. Consequently, it is not expected that it played an important role in shaping the flood hydrograph.
4 HYDROLOGIC SIMULATIONS
Here we introduce a methodology, based on hydrological simulations, to test the impact of neglecting the effect of storm motion and velocity on the flood hydrograph. To investigate potential model dependency on results, two spatially distributed hydrological models, of varying complexity in terms of process description and parameter space, are used together with fine-scale rainfall observations. This is expected to highlight potential model dependencies on the results and thus provide a range of results (in case of differences) or a more robust conclusion (in case of agreement).
4.1 Hydrological models
Two different hydrological models were used in this study. The first is the triangulated irregular network (TIN)-based real-time integrated basin simulator (tRIBS) model (Ivanov et al. Citation2004a, Citation2004b, Vivoni et al. Citation2007). The tRIBS is a distributed physics-based model that explicitly accounts for the spatial variability of land surface descriptors (terrain, soil and vegetation), soil moisture and atmospheric forcing. Infiltration is simulated in a sloped heterogeneous and anisotropic soil based on a kinematic approximation for unsaturated flow (Cabral et al. Citation1992, Garrote and Bras Citation1995). An adaptive multiple resolution approach based on TINs (Vivoni et al. Citation2004) is used to represent the complexity of the simulation domain. Runoff is generated at each computational element of the domain via a variety of mechanisms (infiltration excess, saturation excess, interflow, groundwater exfiltration) depending on the soil saturation state. A detailed description regarding the set-up, calibration and validation of the model for the Fella basin is provided in Nikolopoulos et al. (Citation2011).
The second model used is the KLEM (Zoccatelli et al. Citation2010, Zanon et al. Citation2010). The KLEM model is a simple spatially distributed hydrological model that is based on availability of raster information for the topography and for the soil and vegetation properties. In the model, runoff rate is computed from the rainfall rate using the Green-Ampt infiltration model with moisture redistribution (Ogden and Saghafian Citation1997) and a module for subsurface flow generation. A simple description of the drainage system response (Da Ros and Borga Citation1997, Giannoni et al. Citation2003) is used to represent runoff propagation. This is based on the identification of drainage paths, and requires the characterization of hillslope paths and channelled paths. A channelization support area (As) is used to distinguish hillslope elements from channel elements. Two invariant flow velocities are used along the hillslope and channel portion of the flow drainage structure for runoff propagation. These parameters were constant for each sub-basin but were variable among the various sub-basins to reflect spatial variations in the flood propagation processes. Model parameterization was based on the previous work by Borga et al. (Citation2007) that used a very similar model for the Fella basin, calibrated and validated for the same storm event examined in this study. The model calibration used observed runoff data at the Fella outlet supported by further peak flood observations made for internal sub-basins (Borga et al. Citation2007). In particular, the values of the flow velocities ranged between 2.5 and 5 m s-1 (channel velocity) and between 0.03 and 0.2 m s-1 (hillslope velocity), whereas the channelization support area ranged between 0.01 and 0.01 km2. Post-flood surveys confirmed the accuracy of the calibrated high values of channel velocity (Borga et al. Citation2007).
4.2 Catchment-scale storm velocity effect on flood response
To investigate the effect of storm velocity on flood response, we carried out a series of hydrological simulations for which we used rainfall scenarios with different levels of rainfall space–time variability. More specifically, the hydrological response resulting from the original rainfall field (control simulation) was contrasted with the results obtained from: (a) spatially uniform and (b) constant spatial rainfall pattern cases. In all cases, the basin-averaged rainfall remained constant (i.e. constant rainfall volume applied at each time), while the spatial rainfall pattern was completely removed (in the uniform case), or kept constant and equal to the total rainfall accumulation pattern (constant pattern case). The latter was achieved by scaling the total rainfall pattern with an appropriate factor so that the basin-averaged rainfall remained equal to the original rain. Note that because the overall spatial rainfall organization is preserved in the constant pattern case, the values of Δ1 and Δ2 are the same as for the original rainfall case.
The rationale for developing the three rainfall scenarios is as follows. In our methodology, based on spatial moments, we assume that the shape of the flood hydrograph is controlled by:
the catchment drainage structure,
the temporal pattern of basin-averaged rainfall rates (hyetograph);
the two descriptors of overall rainfall organization at catchment scale Δ1 and Δ2, and
the catchment-scale storm velocity.
The control simulation is the result of the combination of factors (a)–(d), the constant pattern simulation is controlled by factors (a)–(c), whereas the uniform-rainfall simulation is controlled by factors (a) and (b). Comparison of the control simulation with the constant pattern simulation permits isolation of the effect of catchment-scale storm velocity on the flood hydrograph, whereas comparison of the control simulation with the uniform-rainfall simulation affords isolation of the combined effect of Δ1, Δ2 and catchment-scale storm velocity on the flood hydrograph. Note that in order to isolate these effects and be able to perform a consistent analysis, all model simulations were carried out based on the original (control) parameter set and no subsequent recalibration of the models was performed when a new rainfall scenario was considered.
Simulations were carried out for the 21 basins indicated in . Comparisons between uniform and control flood hydrographs and between constant pattern and control flood hydrographs were summarized by using the Nash–Sutcliffe (NS) index. The NS index was selected because it is non-dimensional and permits a quick assessment of the quality of the simulations. The NS indexes are reported in for the two comparisons, for all basins examined and for the two hydrological models. The NS scores are ranked in ascending order for both uniform vs control, and constant pattern vs control cases. Thus, note that the figure presents the distribution of values for each case without providing a one-to-one correspondence between cases or between models. The results for the constant pattern case show that, with the exception of Basin 9 (for tRIBS results), for all other basins NS values are greater than 0.9 for both models. This clearly indicates that the essential elements of spatial rainfall variability which play a role in hydrograph shape are captured by rainfall patterns which preserve the spatial moments Δ1 and Δ2, but neglect storm motion. These results show that storm motion and velocity play a negligible role in controlling the flood response at the various spatial scales examined here. The agreement between the two hydrological models shows that this is a particularly robust outcome of this work.
Fig. 7 Nash–Sutcliffe (NS) scores calculated between the control hydrographs and those corresponding to constant pattern (triangles) and uniform (circles) rainfall, ranked in ascending order, for (a) tRIBS and (b) KLEM. The three selected basins are identified with respect to the constant pattern results. The downward-pointing arrows in the left plot indicate that there are three points at these locations below the NS threshold of 0.5.
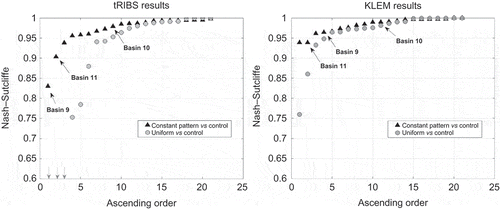
Comparison between the results for the two hydrological models revealed discrepancies for the uniform rainfall case. Specifically, tRIBS simulations exhibit relatively lower NS values than KLEM suggesting higher sensitivity when a spatially uniform forcing is applied. This finding confirms earlier results obtained when considering models of varying complexity and their sensitivity to input errors (Michaud and Sorooshian Citation1994, Andréassian et al. Citation2001, Segond et al. Citation2007, see also references in Moore Citation1999). Comparison of the tRIBS simulations for uniform and original rainfall indicates that the soil buffers a significant amount of rainfall before runoff is generated. This suggests that saturation excess is the dominant mechanism and subsequently runoff generation is essentially a threshold process, controlled by the infiltration module. Negligible runoff is generated when the rainfall is less than the threshold required to saturate the soil column and trigger surface runoff. This clearly enhances the sensitivity to the averaging process which is carried out when the model is forced with spatially uniform rainfall. In the case of KLEM, both surface runoff generated by the infiltration excess mechanism and the subsurface stormflow mechanism were important contributors to the flood volume. Therefore, runoff generation was controlled by both rainfall volume and rainfall intensity, which makes less dramatic the sensitivity to the use of spatially uniform rainfall.
To provide an example of the differences in sensitivity between the two models, shows the simulated hydrographs for the three selected basins: Basin 9 (46 km2), Basin 10 (329 km2) and Basin 11 (623 km2) for tRIBS (, and ) and KLEM (, and ), respectively. reports the three simulations based on the three rainfall scenarios considered in the comparisons. Simulated response based on the original rainfall fields (control) differs for the two models, with relative discrepancies that increase with decreasing catchment size. Comparison of results reported in shows that the relative difference between tRIBS and KLEM simulated flood peaks is around 19% for the two larger basins, and increases to 50% for the smallest basin. It should be noted that discharge values were available for calibration only for the Fella outlet section, the two internal basins representing ungauged catchments. These results point to the large uncertainty in representing the internal hydrological functioning for extreme flash floods, particularly when extremely dry initial conditions are coupled with extreme rain rates and depths.
Fig. 8 Simulated hydrographs based on tRIBS (left) and KLEM (right) models for three selected basins. Results are shown for scenarios of original rainfall forcing (control), uniform rainfall (uniform) and constant rainfall pattern (constant pattern).
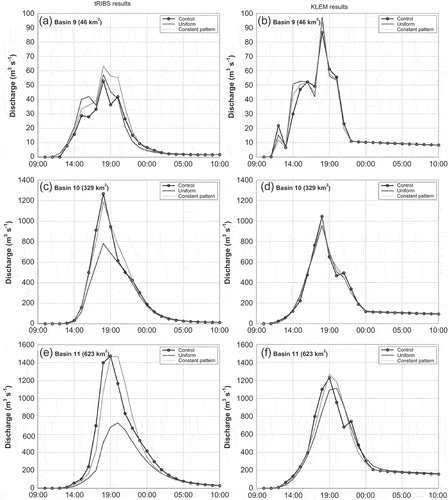
For the case of the constant rainfall pattern scenario, both models show that the discrepancy relative to the control simulation is insignificant. However, for the uniform case the response from the two models is different with tRIBS showing significantly higher sensitivity than KLEM especially for the larger basin scales, as reported above. The simulated hydrographs from tRIBS show a significant decrease in flood response for the larger basins while the corresponding KLEM simulations are affected to a lesser degree by the uniform rainfall forcing. Apparently, KLEM exhibits a less non-linear response than tRIBS, which is attributed to the inherent differences of the dominant runoff generation mechanisms in the two models, as previously explained. These findings indicate (a) the importance of investigating model dependencies and (b) the fact that two different models that do not always agree showed consistent results for the case of constant pattern. The latter indicates that storm velocity did not play an important role in shaping the flood hydrograph during this event. It was mainly the spatial distribution of rainfall volume over the basin, and not its motion, that controlled the flood response.
5 SUMMARY AND DISCUSSION
Catchment-scale storm velocity was quantified for a major flash-flood-triggering storm based on the analyses carried out on 21 sub-basins with areas ranging between 8 and 623 km2. Derivation of velocity was based on two different approaches that involved the calculation of storm velocity over (a) a fixed 1-h time window (V) and (b) a variable time window equal to the basin’s mean response time (Vs). These two different approaches were chosen in order to present velocity values calculated consistently for all basins (case of V) but also to demonstrate the magnitude of the velocity over characteristic time scales which is relevant to the hydrological response of each basin (case of Vs). The results obtained showed that values of V reach up to 5.5 m s-1 and are much higher than values for Vs which did not exceed 1 m s-1 most of the time for all basins examined. Apart from the differences in magnitude there is another distinct difference related to the scale dependence of velocities V and Vs. It was shown that there is a strong non-linear dependence between catchment scale and velocity V, according to which, velocity increases linearly with the logarithm of area. This dependence is almost entirely due to the strong relationship between the parameter g1 and basin size through Hack’s law.
This dependence was masked in the case of Vs because application of a variable regression window had the effect of smoothing the velocity pattern (see Section 3.2). Moreover, the values of Vs were remarkably low with respect to the velocity of the travelling convective cells. It is speculated that this is due to the effect of two different controls emerging at various scales. At basin scales less than 20 km2, the ratio between storm duration and cell travel time is small hence reducing the magnitude of Vs. At basin scales larger than 20 km2, the temporal variability of the hourly catchment-scale storm velocity values is too high, which also limits the magnitude of Vs.
A methodological approach was introduced that allows us to isolate and investigate the effect of catchment-scale storm velocity on the flood hydrograph. Hydrological simulations carried out for a constant rainfall pattern permit the isolation of the effect of velocity on flood response when compared with the original rainfall hydrographs. Results from the hydrological simulations with tRIBS and KLEM showed that simulations based on the original and constant pattern rainfall fields were in good agreement. However, comparison for the uniform case scenario showed significant differences (mainly for the larger scale basins) suggesting a higher sensitivity of tRIBS to the relative distribution of rainfall volume over the basin. Nevertheless, the main conclusion of this analysis, consistent for both models, is that the constant pattern hydrographs were almost identical (NS >0.9) with the original rainfall hydrographs. This implies that the movement of rainfall over the basins had no effect in shaping the flood response. This may suggest that for this storm and flood event, it was the slow-moving convective systems, rather than the fast-moving convective cells, that controlled the space–time distribution of rainfall and the flood response.
The effect of storm velocity on flood response was examined in this study for a single flash-flood-triggering storm case and thus findings cannot be used to derive generalized conclusions. However, the methodology developed in this study may be used to advance the understanding of the effect of storm motion on flood response by considering multiple flood and flash-flood events. Basin scale was hypothesized and proven to have a strong effect on the magnitude of storm velocity, but there are also other factors such as basin shape (e.g. elongation) and orientation (relative to storm movement) that can potentially depict a strong relationship with velocity. These aspects require further research to gain a more complete understanding on the effect of storm motion on flood response.
Funding
This work was financially supported by the STREP Project HYDRATE, Contract (GOCE-037024) and the Research Project GEO-RISKS (University of Padova, STPD08RWBY).
REFERENCES
- Andréassian, V., et al., 2001. Impact of imperfect rainfall knowledge on the efficiency and the parameters of watershed models. Journal of Hydrology, 250, 206–223. doi:10.1016/S0022-1694(01)00437-1.
- Aylward, R.P. and Dyer, J.L., 2010. Synoptic environments associated with the training of convective cells. Weather and Forecasting, 25 (2), 446–464. doi:10.1175/2009WAF2222275.1.
- Bacchi, B., Ranzi, R., and Borga, M., 1996. Statistical characterization of spatial patterns of rainfall cells in extratropical cyclones. Journal of Geophysical Research, 101, 26277–26286. doi:10.1029/96JD01381.
- Borga, M., et al., 2002. Long-term assessment of bias adjustment in radar rainfall estimation. Water Resources Research, 38 (11). doi:10.1029/2001WR000555.
- Borga, M., et al., 2007. Hydrometeorological analysis of the 29 august 2003 flash flood in the eastern italian alps. Journal of Hydrometeorology, 8 (5), 1049–1067. doi:10.1175/JHM593.1.
- Bouilloud, L., et al., 2009. Radar rainfall estimation for the post-event analysis of a Slovenian flash-flood case: application of the mountain reference technique at c-band frequency. Hydrology and Earth System Sciences, 13, 1349–1360. doi:10.5194/hess-13-1349-2009.
- Browning, K.A. and Collier, C.G., 1989. Nowcasting of precipitation systems. Reviews of Geophysics, 27, 345–370. doi:10.1029/RG027i003p00345.
- Cabral, M.C., et al., 1992. A kinematic model of infiltration and runoff generation in layered and sloped soils. Advances in Water Resources, 15, 311–324. doi:10.1016/0309-1708(92)90017-V.
- Collier, C., 2007. Flash flood forecasting: what are the limits of predictability? Quarterly Journal of the Royal Meteorological Society, 133 (622A), 3–23. doi:10.1002/qj.29.
- Collier, C.G. and Fox, N.I., 2003. Assessing the flooding susceptibility of river catchments to extreme rainfall in the United Kingdom. International Journal of River Basin Management, 1, 225–235. doi:10.1080/15715124.2003.9635209.
- Corfidi, S.F., Meritt, J.H., and Fritsch, J.M., 1996. Predicting the movement of mesoscale convective complexes. Weather and Forecasting, 11, 41–46. doi:10.1175/1520-0434(1996)011<0041:PTMOMC>2.0.CO;2.
- Da Ros, D. and Borga, M., 1997. Use of digital elevation model data for the derivation of the geomorphological instantaneous unit hydrograph. Hydrological Processes, 11, 13–33. doi:10.1002/(SICI)1099-1085(199701)11:1<13::AID-HYP400>3.0.CO;2-M.
- De Lima, J.L. and Singh, V.P., 2002. The influence of the pattern of moving rainstorms on overland flow. Advances in Water Resources, 25 (7), 817–828. doi:10.1016/S0309-1708(02)00067-2.
- Doswell, C.A.I.I.I., Brooks, H.E., and Maddox, R.A., 1996. Flash flood forecasting: an ingredients-based methodology. Weather and Forecasting, 11, 560–581. doi:10.1175/1520-0434(1996)011<0560:FFFAIB>2.0.CO;2.
- Garrote, L. and Bras, R.L., 1995. A distributed model for real-time flood forecasting using digital elevation models. Journal of Hydrology, 167, 279–306. doi:10.1016/0022-1694(94)02592-Y.
- Giannoni, F., et al., 2003. Hydrologic modeling of extreme floods using radar rainfall estimates. Advances in Water Resources, 26, 195–203. doi:10.1016/S0309-1708(02)00091-X.
- Ivanov, V.Y., et al., 2004a. Catchment hydrologic response with a fully distributed triangulated irregular network model. Water Resources Research, 40 (11), W11102. doi:10.1029/2004WR003218.
- Ivanov, V.Y., et al., 2004b. Preserving high-resolution surface and rainfall data in operational-scale basin hydrology: a fully-distributed physically-based approach. Journal Of. Hydrology, 298 (1–4), 80–111. doi:10.1016/j.jhydrol.2004.03.041.
- Maksimov, V.A., 1964. Computing runoff produced by a heavy rainstorm with a moving center. Soviet Hydrology, 5, 510–513.
- Marchi, L., et al., 2010. Characterisation of selected extreme flash floods in europe and implications for flood risk management. Journal of Hydrology, 394 (1–2), 118–133. doi:10.1016/j.jhydrol.2010.07.017.
- Michaud, J. and Sorooshian, S., 1994. Comparison of simple versus complex distributed runoff models on a midsized semiarid watershed. Water Resources Research, 30 (3), 593–605. doi:10.1029/93WR03218.
- Moore, R.J., 1999. Real-time flood forecasting systems: perspectives and prospects. In: R. Casale and C. Margottini, eds. Floods and landslides: integrated risk assessment. Berlin: Spinger-Verlag, 147–189.
- Moore, R.J., et al., 2006. Spatio-temporal rainfall datasets and their use in evaluating the extreme event performance of hydrological models. R&D Technical Report FD2208, Report to Defra and the Environment Agency. Wallingford: CEH, 259 p.
- Niemczynowicz, J., 1984. Investigation of the influence of rainfall movement on runoff hydrograph: part I. simulation of conceptual catchment. Nordic Hydrology, 15, 57–70.
- Nikolopoulos, E.I., et al., 2011. Sensitivity of a mountain basin flash flood to initial wetness condition and rainfall variability. Journal of Hydrology, 402 (3–4), 165–178. doi:10.1016/j.jhydrol.2010.12.020.
- Ogden, F.L., Richardson, J.R., and Julien, P.Y., 1995. Similarity in catchment response: 2. moving rainstorms. Water Resources Research, 31 (6), 1543–1547. doi:10.1029/95WR00519.
- Ogden, F.L. and Saghafian, B., 1997. Green and ampt infiltration with redistribution. Journal of Irrigation and Drainage Engineering, 123 (5), 386–393. doi:10.1061/(ASCE)0733-9437(1997)123:5(386).
- Pellarin, T., et al., 2002. Hydrologic visibility of weather radar systems operating in mountainous regions: case study for the Ardèche catchment (France). Journal of Hydrometeorology, 3 (5), 539–555. doi:10.1175/1525-7541(2002)003<0539:HVOWRS>2.0.CO;2.
- Rigon, R., et al., 1996. On Hack’s law. Water Resources Research, 32 (11), 3367–3374. doi:10.1029/96WR02397.
- Sangati, M. and Borga, M., 2009. Influence of rainfall spatial resolution on flash flood modelling. Natural Hazards and Earth System Sciences, 9, 575–584. doi:10.5194/nhess-9-575-2009.
- Sangati, M., et al., 2009. Influence of rainfall and soil properties spatial aggregation on extreme flash flood response modelling: an evaluation based on the Sesia river basin, North Western Italy. Advances in Water Resources, 32 (7), 1090–1106. doi:10.1016/j.advwatres.2008.12.007.
- Segond, M.L., Wheater, H.S., and Onof, C., 2007. The significance of spatial rainfall representation for flood runoff estimation: a numerical evaluation based on the Lee catchment, UK. Journal of Hydrology, 347, 116–131. doi:10.1016/j.jhydrol.2007.09.040.
- Seo, Y., Schmidt, A.R., and Sivapalan, M., 2012. Effect of storm movement on flood peaks: analysis framework based on characteristic timescales. Water Resources Research, 48, W05532. doi:10.1029/2011WR011761.
- Singh, V.P., 1998. Effect of the direction of storm movement on planar flow. Hydrological Processes, 12, 147–170. doi:10.1002/(SICI)1099-1085(199801)12:1<147::AID-HYP568>3.0.CO;2-K.
- Smith, J.A., et al., 2000. Catastrophic rainfall and flooding in texas. Journal of Hydrometeorology, 1, 5–25. doi:10.1175/1525-7541(2000)001<0005:CRAFIT>2.0.CO;2.
- Smith, J.A., et al., 2002. The regional hydrology of extreme floods in an urbanizing drainage basin. Journal of Hydrometeorology, 3 (3), 267–282. doi:10.1175/1525-7541(2002)003<0267:TRHOEF>2.0.CO;2.
- Smith, J.A., et al., 2005. Field studies of the storm event hydrologic response in an urbanizing watershed. Water Resources Research, 41, W10413. doi:10.1029/2004WR003712.
- Sturdevant-Rees, P.L., et al., 2001. Tropical storms and the flood hydrology of the Central Appalachians. Water Resources Research, 37 (8), 2143–2168. doi:10.1029/2000WR900310.
- Tropeano, D., Turconi, L., and Sanna, S., 2004. Debris flow triggered by the 29 August 2003 cloudburst in Val Canale, eastern Italian Alps. In: Proceedings International Symposium INTERPRAEVENT 2004, Riva del Garda, Italy, 121–132.
- Viglione, A., et al., 2010. Generalised synthesis of space-time variability in flood response: an analytical framework. Journal of Hydrology. doi:10.1016/j.jhydrol.2010.05.047.
- Vivoni, E.R., et al., 2004. Generation of triangulated irregular networks based on hydrological similarity. Journal of Hydrologic Engineering, 9, 288–302. doi:10.1061/(ASCE)1084-0699(2004)9:4(288).
- Vivoni, E.R., et al., 2007. Controls on runoff generation and scale-dependence in a distributed hydrologic model. Hydrology and Earth System Sciences, 11 (5), 1683–1701. doi:10.5194/hess-11-1683-2007.
- Wilson, J.W., et al., 1998. Nowcasting thunderstorms: a status report. Bulletin of the American Meteorological Society, 79, 2079–2099. doi:10.1175/1520-0477(1998)079<2079:NTASR>2.0.CO;2.
- Woods, R. and Sivapalan, M., 1999. A synthesis of space–time variability in storm response: rainfall, runoff generation and routing. Water Resources Research, 35 (8), 2469–2485. doi:10.1029/1999WR900014.
- Zanon, F., et al., 2010. Hydrological analysis of a flash flood across a climatic and geologic gradient: the September 18, 2007 event in western Slovenia. Journal of Hydrology, 394 (1–2), 182–197. doi:10.1016/j.jhydrol.2010.08.020.
- Zoccatelli, D., 2013. A framework for the analysis of the influence of rainfall spatial organization and basin morphology on flood response. PhD Dissertation. University of Padova, 145 p.
- Zoccatelli, D., et al., 2010. Which rainfall spatial information for flash flood response modelling? A numerical investigation based on data from the Carpathian range, Romania. Journal of Hydrology, 394 (1–2), 148–161. doi:10.1016/j.jhydrol.2010.07.019.
- Zoccatelli, D., et al., 2011. Spatial moments of catchment rainfall: rainfall spatial organisation, basin morphology, and flood response. Hydrology and Earth System Sciences, 15, 3767–3783. doi:10.5194/hess-15-3767-2011.