Abstract
Coastal areas will be exposed to increasing risks over coming decades due to climate change and sea-level rise. Our study focuses on the Vistula Lagoon located in the south Baltic, whose water regime and ecological status are highly dependent on the river inflow coming from its catchment. Changes in climate may cause variations in water quantity and quality in the rivers and affect the ecological and socio-economic potential of the lagoon. The climate impact study in this catchment containing more than 20 rivers is challenging as only a few of them are gauged. The impacts were modelled, using the ecohydrological model SWIM, and applying a set of 15 climate scenarios from the ENSEMBLES project. Scenario analysis revealed a projected overall increase in precipitation, as well as in temperature, for the Vistula Lagoon catchment, which cause diverse changes in discharge, water temperature, dissolved nutrient concentrations and loads coming from the rivers.
Editor D. Koutsoyiannis; Guest editor M. White
Résumé
En raison du changement climatique et de la hausse du niveau de la mer, les zones côtières vont être exposées à des risques croissants dans les prochaines décennies. Notre étude porte sur le delta de la Vistule, localisé au Sud de la mer Baltique, dont le régime hydrologique et l’état écologique dépendent directement de ses affluents. Les changements climatiques risquent de modifier les débits et les apports de ces cours d’eau, entraînant des modifications du potentiel écologique et socio-économique du delta C’est une gageure que de vouloir faire cette étude pour un bassin versant comprenant plus de 20 affluents, la plupart non jaugés. Les impacts ont été modélisés à partir le modèle éco-hydrologique SWIM, en prenant en compte 15 scénarios climatiques établis par le projet ENSEMBLES. L’analyse des divers scénarios montre une augmentation généralisée aussi bien des températures que des précipitations dans le bassin d’alimentation du delta de la Vistule, ce qui entraîne la modification des débits, de la température, de la charge solide et de la concentration en éléments nutritifs dissous des divers affluents.
1 INTRODUCTION
According to the Intergovernmental Panel on Climate Change (IPCC Citation2007), coastal areas will be exposed to increasing risks over coming decades due to climate change, land-use change and sea-level rise. The anticipated changes include a rise in air and sea-surface temperatures, an accelerated rise in sea level, altered precipitation and runoff from rivers, and larger extreme waves and storm surges (IPCC Citation2007). The potential impacts will differ considerably at regional and local scales, and have to be studied in order to improve the preparedness of people and adaptive capacity.
Climate impact studies for lagoons and coastal areas worldwide often deal with direct impacts of climate change on their water balances and ecosystems (e.g. Simas et al. Citation2001, Scavia et al. Citation2002, Lloret et al. Citation2008, Jakimavicius and Kriauciuniene Citation2013), and a consensus exists regarding the importance of developing adequate lagoon management plans and of implementing proactive measures (Anthony et al. Citation2009, Chapman Citation2012).
Besides water exchange with the connected sea or ocean, the status of a lagoon largely depends on the ecological situation within its catchment and on the water quality of all inflowing rivers. Changes in climate conditions, which can be expected worldwide and are already visible in overall global and regional trends (IPCC Citation2007, Dailidienė et al. Citation2011, Lehmann et al. Citation2011), may cause variations in water quantity (Reihan et al. Citation2007, Hirabayashi et al. Citation2008) and quality (Whitehead et al. Citation2009) coming from the drainage area, and may affect the lagoon’s ecological and socio-economic potential. An impact assessment of the lagoon catchment using modelling will support stakeholders and water managers in choosing appropriate adaptation measures (Nicholls et al. Citation2007, NRC Citation2010) to help protect its ecosystem.
The simulation of river runoff provided directly by climate models is not reliable, as shown by comparisons between observed discharge and model outputs for the 20th century (IPCC Citation2007, Graham et al. Citation2007). A commonly-used technique for conducting hydrological impact studies at the catchment scale is to use climate parameters (e.g. precipitation and temperature) provided by climate models as input for hydrological models (Teutschbein and Seibert Citation2010). As global climate models have too coarse resolution for regional ecohydrological studies, some downscaling is needed to get reliable input data for the hydrological models. It is usually provided either by regional climate models (RCMs) driven by general circulation models (GCMs), or by using statistical downscaling methods. Using several driving RCMs (multi-model approach) to investigate the range of uncertainty of impact projections is preferable to applying just one climate model as a driver (Tebaldi and Knutti Citation2007).
The Vistula Lagoon, located in the south of the Baltic Sea and shared by Poland and Russia, is one of the largest inner-marine water bodies in Europe. More than 20 rivers flow into the Vistula Lagoon and drain an area of about 21 000 km2. The catchment is mainly used for agriculture (67%), which causes diffuse nutrient inputs to the marine water body accompanied by emissions from point sources. According to Chubarenko et al. (Citation2012), climate parameters observed in the Russian part of the Vistula Lagoon catchment show statistically significant positive trends in both air temperature and precipitation for the period 1981–2010. Trends to a warmer climate were also observed in northern Poland (Marszelewski and Skowron Citation2006, Wibig and Jakusik Citation2012), and this may affect the Vistula Lagoon and its catchment.
The objective of this study was to simulate climate change impacts on runoff and nutrient flows in the catchment of the Vistula Lagoon until the end of 21st century. It uses a set of available climate scenarios to provide boundary conditions to lagoon modellers for a subsequent separate climate impact assessment on the marine ecosystem of the Vistula Lagoon. An ecohydrological model, Soil and Water Integrated Model (SWIM; Krysanova et al. Citation2000) was used as modelling tool, and climate scenarios from the ENSEMBLES project (see Section 2.2) served as drivers.
The climate impact study in this catchment of more than 20 rivers is challenging, because (a) only a few of the rivers are gauged hydrologically—the model can therefore be properly calibrated/validated only on part of the study area, and then applied to the whole drainage area of the lagoon; and (b) in addition to water discharge, nutrient concentrations and loads also have to be modelled, although observed data on water quality and data on point-source emissions are even more scarce. Therefore, an approach is necessary for estimating input data using all available data and empirical methods, and also for an application of the modelling tool partly in ungauged mode.
The results will enable an understanding of future developments in the Vistula Lagoon catchment in view of climate change, and contribute to developing an appropriate adaptation strategy for the region.
2 MATERIAL AND METHODS
2.1 The Soil and Water Integrated Model (SWIM)
The ecohydrological SWIM model (Krysanova et al. Citation2000) was developed based on two models: SWAT (Arnold et al. Citation1993) and MATSALU (Krysanova et al. Citation1989). SWIM is a process-based semi-distributed model for river basin and regional scales. The model simulates all processes with a daily time step and uses a spatial disaggregation scheme for catchments into sub-basins and hydrotopes. The latter, defined by overlaying sub-basin, land-use and soil maps, are used to simulate all water flows and nutrient cycling in soil as well as vegetation growth, as it is assumed that units that have the same land-use and soil types within one sub-basin behave similarly.
Climate as well as land-use, water management and nutrient input to surface waters from point sources are important external drivers for processes simulated in the model. Climate parameters are assumed to be homogeneous at the sub-basin level. Measured or projected climate data are interpolated to the sub-basin centroids by using an inverse-weighted distance method.
Hydrological processes in SWIM are based on the water balance equation and take into account surface, subsurface and groundwater flows, as well as percolation and recharge of the aquifers. Main external drivers are precipitation and snow amounts, together with temperature and solar radiation influencing snowmelt processes and evapotranspiration potential.
The crop and vegetation module represents an important interface between hydrological processes and nutrients. Vegetation growth, and its decline and harvesting, influence evapotranspiration processes as well as nutrient availability in the soils. Plant growth in the model can be influenced by four potential stress factors: temperature, soil moisture, nitrogen and phosphorus content in soil.
The nitrogen module for the soil layers includes four pools: nitrate nitrogen, active and stable organic nitrogen, and organic nitrogen in plant residues, as well as flows: fertilization, input with precipitation, plant uptake, mineralization, denitrification, wash-off, leaching and erosion. An ammonium nitrogen pool was added to the nitrogen cycle (Hesse et al. Citation2012) taking into account decomposition, mineralization, nitrification, volatilization, leaching, erosion and plant uptake processes.
The following pools represent soil phosphorus in SWIM: labile phosphorus, active and stable mineral phosphorus, organic phosphorus and phosphorus in the plant residue, and the flows: fertilization, sorption and desorption, mineralization, plant uptake, erosion and wash-off. In the applied SWIM version, soluble phosphorus is also allowed to leach vertically through the soil profile (Hesse et al. Citation2008).
While passing the soil layers nitrogen and phosphorus are added to the corresponding water flows (interflow and baseflow). Then nutrients are transported with surface, subsurface and groundwater flows to the river network, and afterwards routed to the river mouth and corresponding water body (lagoon in this case study).
Water temperature was calculated in the model according to Stefan and Preud'homme (Citation1993), by using the average daily air temperature of the sub-basin. Dissolved oxygen concentration in the rivers is the difference between the saturated oxygen concentration and oxygen consumption. It is calculated as a function of oxygen re-aeration processes, oxygen demand by ammonium nitrogen, as well as oxygen demand of the river sediments.
SWIM has been successfully applied to several catchments of different sizes in Germany, and later in other river basins in Europe, Africa, Asia and South America. It is still being developed further in accordance with particular research needs or specific case characteristics (see overview in Krysanova et al. Citation2015).
2.2 ENSEMBLES climate scenarios
A set of climate scenario data provided for climate impact studies in Europe by the ENSEMBLES project (van der Linden and Mitchell Citation2009) was used in our study. In this project a set of regional climate models (RCMs) was run using boundary conditions produced by different GCMs driven by the A1B emissions scenario. Such a multi-model approach delivers several projections for the future European climate, whose average has a higher reliability than each single scenario with its general uncertainty. The spatial resolution of the scenarios is 25 or 50 km, and the simulated period is either 1951–2050 or 1951–2100. For our study only the scenarios with 25 km resolution, which fits better to the scale of the catchment area, and the period until 2100 (in some cases 2098) were selected. Altogether, 15 climate scenarios produced by nine European institutes were chosen (see S1—S15 in ). A usual climatological baseline period is a 30-year normal period, as defined by the World Meteorological Organization (IPCC-TGCIA Citation1999). For the impact assessment, a reference period 1971–2000 (p0) and three scenario periods: near future 2011–2040 (p1), intermediate future 2041–2070 (p2), and far future 2071–2098 (p3) were chosen.
Table 1 GCM/RCM combination matrix of the climate scenarios used in this study for climate impact assessment.
Before we applied the ENSEMBLES scenarios for impact assessment, they were analysed by comparing the long-term means of climate parameters: temperature and precipitation of the reference period p0 and the future scenario periods, p1, p2 and p3.
After hydrological and water quality calibration, all 15 chosen climate change scenarios from the ENSEMBLES project were applied to run the calibrated SWIM model for the Vistula Lagoon catchment. The land-use and management input data of the reference period remained unchanged for the future periods in order to evaluate the impact of climate change alone. The climate impacts on water discharge and water quality variables were analysed, considering an average of all 15 model runs on a long-term average daily, seasonal and annual basis. To get an impression of the ranges of uncertainty of future projections, different percentiles of the scenario results, as well as minimum and maximum values, were also analysed. The three future scenario periods were always analysed in comparison to the reference conditions simulated by SWIM driven by the same climate model set.
2.3 Evaluation of the model results
Performance of the SWIM model for simulating water discharge in the rivers of the Vistula Lagoon catchment was analysed using two common criteria of fit: (a) the Nash and Sutcliffe efficiency criterion (NSE; Nash and Sutcliffe Citation1970) and (b) the deviation in balance (DB). The equations to calculate NSE and DB can be found in Hesse et al. (Citation2008).
The non-dimensional NSE criterion describes the squared differences between the observed and simulated values, and can be used to prove the quality of simulated hydrological results. Its value is based on the dispersion of values around the line of equal values. The NSE is very sensitive to extreme values due to the squared differences; it can vary from –∞ to 1 and should be as near as possible to 1. In hydrological modelling, NSE values above 0.5 are considered to correspond to ‘satisfactory’, and above 0.65 to ‘good’ modelling results for a monthly time step (Moriasi et al. Citation2007).
The DB is the relative difference in balance, which shows the long-term differences of the observed values against the simulated ones for the whole modelling period. It corresponds to the percent bias (PBIAS), but with the opposite algebraic sign. For best model results the deviation in balance should be as near as possible to 0. Usually, DB/PBIAS below ±25% is considered as ‘satisfactory’ in hydrological modelling with monthly time steps, and DB/PBIAS lower than ±10% shows ‘very good’ model results (Moriasi et al. Citation2007).
In this study, model performances were mainly calculated with daily values, where good performance rates are generally more difficult to obtain than for simulations with monthly time steps.
3 STUDY AREA, DATA PREPARATION AND MODEL SET-UP
3.1 Description of the case study area
The Vistula Lagoon in the south Baltic Sea is one of the largest inner-marine water bodies in Europe (area: 838 km2), with a drainage area of about 20 730 km2, shared by two countries, Poland (approx. 60%) and Russia (approx. 40%) (). The average annual precipitation in the catchment is about 750 mm with summer maxima, while the average annual temperature is about 7.7°C.
Fig. 1 Overview map of the Vistula Lagoon catchment showing the digital elevation model (DEM), national borders, major sub-catchments (numbered), main river courses, discharge gauges with available data, as well as the grid points of WATCH climate data.
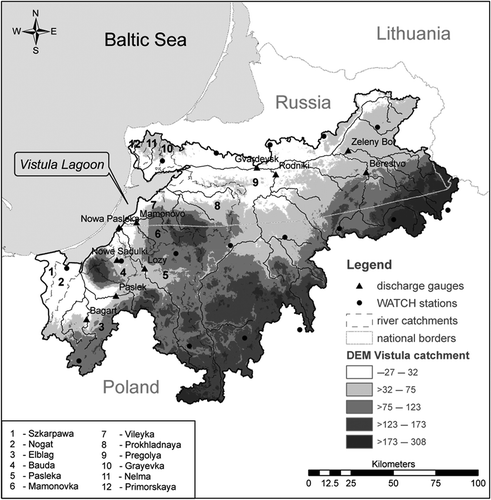
According to Shuttle Radar Topography Mission (SRTM) data, altitude in the catchment ranges from −27 to 308 m a.s.l. Watershed delineation using a digital elevation model (DEM) divided the catchment into 442 sub-basins, which were used for further model set-up.
More than 20 rivers drain to the Vistula Lagoon (3.49 km3 water inflow per year on average), of which the Pregolya River is the largest and most important, delivering more than 40% of the total incoming water discharge and accounting for almost 60% of its total catchment area. The average annual discharge to the lagoon delivered by the Pregolya River is 86 m3 s-1. This does not represent the full volume of water originating in the basin, as 40% of its discharge is transferred to the Curonian Lagoon via the Deyma branch near Gvardeysk. The second most significant river flowing to the Vistula Lagoon is the Pasleka River, with a catchment area of about 2250 km, located in Polish territory, and delivering, on average, a discharge of 17 m3 s-1. Discharge regimes of all rivers are characterized by higher water amounts in winter and lower in summer, and a discharge peak in March/April due to snowmelt.
3.2 Data preparation and model set-up
To set up the SWIM model, two main types of data are necessary: (a) maps depicting spatial data on topography, soil types, land-use classes and location of sub-basins; and (b) temporal data and time series on climate parameters and measured discharges. Additionally, information on point and diffuse nutrient sources or water management measures is useful for modelling a human-influenced region.
The density of climate stations with available measured data, especially the continuity of their time series, is quite poor. Therefore it was decided to use the GPCC-corrected WATCH climate forcing dataset (WFDEI) for model set-up and calibration (Weedon et al. Citation2011, Schneider et al. Citation2013). The WATCH climate data used for this study cover the period 1979–2009 to enable model warm-up, and include sub-periods with available observed discharge and water quality data of different rivers in the study area. The WATCH, as well as the ENSEMBLES scenarios daily data on minimum, maximum and average temperatures (°C), precipitation (mm), solar radiation (J cm-2) and relative air humidity (%) were interpolated to the centroids of all sub-basins using an inverse distance method.
Due to the transboundary location of the Vistula Lagoon catchment, with not only a border between two countries, but also between EU and non-EU areas, it was not possible to use a single source for spatial data on land use and soil type distribution. In both cases two different sources had to be combined.
The land use map for the whole catchment was created by using (i) CORINE Land Cover 2000 (CLC2000) for the Polish part, and (ii) the territorial planning scheme of Kaliningrad oblast for the Russian part. Their land-use classes were reclassified to the 15 SWIM land-use classes, which are required by the model. The main land-use category within the Vistula Lagoon catchment is cropland, covering 67% of the area, followed by forested areas (25%). Grasslands as well as settlements each cover about 3% of the catchment.
The soil map used for setting up the SWIM model was derived in a similar way by combining two different data sources: (i) the Harmonized World Soil Database (HWSD), and (ii) the Soil Geographical Database of the European Community (SGDB). Names were assigned according to the FAO-90 soil unit symbols. The main soil types appearing in the study area are variants of Cambisol (37%), followed by Podzoluvisols (18%) and Arenosols (12%).
Alongside these two soil maps, information was provided about horizon nomenclature, depth of upper and lower soil layers, particle size fractions (clay, silt and sand percentages), organic carbon content and bulk density, that were used for the model set-up. As these soil parameters do not cover all the information needed for soil parameterization in SWIM, missing parameters (e.g. saturated conductivity, field capacity and porosity) were derived using a German manual of soil mapping guidelines (AG Boden Citation2005), in which typical relationships between different soil parameters are described. This enabled estimation of all soil parameters required for modelling.
By overlaying sub-basin, soil and land-use maps during the SWIM model set-up, a hydrotope map for the whole Vistula Lagoon catchment was created, containing 4469 hydrotopes.
According to available data, three different methods were used to calculate nutrient inputs to the rivers by point sources in the Vistula Lagoon catchment. The most detailed information was available for the Pasleka basin, with lists of outputs of single wastewater treatment plants (Mantra-East Citation2004). Rautio et al. (Citation2006) noted that 40–50% of the inhabitants of the Pasleka basin are not connected to any sewage system. Nutrient loads originating from this part of the population were estimated per capita (5.5 g N d-1, 1.2 g P d-1) and added to the point-source emissions. For the Mamonovka basin, the point-source emissions per month, listed in HELCOM (Citation2012), were converted to kg d-1 and then implemented in the model. Nutrient inputs from point sources of the remaining part of the Vistula Lagoon catchment (including the largest city of Kaliningrad) were analogously derived using number of inhabitants and assuming that 60% of the population is connected to sewage water treatment plants.
The resulting calculated point-source amounts in kg d-1 (see ) were introduced to the model as constant values over the whole period. During the modelling the estimated total nitrogen (TN) and total phosphorus (TP) values were equally divided between the nutrient forms.
Table 2 Total amounts of point-source nutrient inputs and fertilization rates implemented in the model.
also lists fertilizer amounts used in the model on all agricultural areas assuming winter wheat on all fields, as wheat was the main crop sown in the Polish Odra and Vistula basins in the reference year 2000 (Eriksson et al. Citation2007). The values were estimated based on different sources on fertilization practices in Poland, Warmia-Masuria and the Vistula Lagoon catchment (FAO Citation2003, Mantra-East Citation2004, Burakowska et al. Citation2005).
Regarding water management measures, the two most important water transfer points were implemented in the SWIM model. First, water (and nutrient) outflow via the Deyma River was implemented by assuming that 60% of discharge (and loads) remains in the Pregolya River, whereas 40% flows out of the catchment (Markova and Nechay Citation1960). Second, water inflow to the study catchment was implemented according to Robakiewicz (Citation2010), who noted that 1–3% of the Vistula River discharge still flows to the Vistula Lagoon, although the major part of the discharge is directly channelized to the Baltic Sea. To take this additional water into account during SWIM modelling, it was assumed that water is flowing via the Szkarpawa (0.25%) and Nogat (0.75%) rivers to the lagoon. Water inflow was calculated on a monthly basis using the long-term (1900–1987) averaged water discharge data of the Vistula River (source: http://www.sage.wisc.edu). The connected nutrient loads in the inflowing water were estimated using information from Dojlido et al. (Citation1994) on the average nutrient concentrations in the Vistula River (1989–1991). Assuming an improvement of water treatment facilities, as well as closure of many industrial units in the Vistula basin during the last two decades, the average nutrient concentrations from Dojlido et al. (Citation1994) were halved.
Data on observed discharges and nutrient concentrations of different rivers within the Vistula Lagoon catchment originated from the Institute of Meteorology and Water Management—National Research Institute (IMGW-PIB) and the State Environmental Monitoring (PMŚ), both in Poland, the Kaliningrad Regional Center for Hydrometeorology and Environmental Monitoring (HYDROMET) in Russia, as well as from the Mantra-East project.
3.3 Calibration strategy
Hydrological calibration and validation for the Vistula Lagoon catchment was a challenging task. In addition to the high heterogeneity of spatial input data, and inconsistent time series of water discharge and water quality data with many gaps, a non-trivial SWIM modelling strategy was required, as more than 20 river catchments had to be combined within one SWIM modelling project. Some sub-catchments of smaller rivers flowing to the lagoon had to be modelled without any information on discharge and/or water quality, and could not be calibrated. Therefore, it was decided to carefully calibrate and validate the model for the two largest sub-catchments Pregolya and Pasleka (by dividing the total period with available observed data into two half sub-periods), and then to use the calibrated parameter set for the whole catchment. This approach was reasonable, because (a) the Pregolya and Pasleka sub-catchments cover 82% of the total Vistula Lagoon drainage area, (b) these two rivers have the longest available time series with measured discharge data, and (c) the other smaller catchments most likely have similar geophysical and hydrological conditions (assumed due to their location close to the calibrated catchments in the same climatic and geographic region).
The dynamics of water quality variables—nitrate nitrogen (NO3-N), ammonium nitrogen (NH4-N), phosphate phosphorus (PO4-P), dissolved oxygen (DO)—and water temperature were properly calibrated and validated in detail only for the Pasleka River, due to availability of measured data. Unfortunately, it was not possible to get time series on measured water quality parameters for the largest river, Pregolya, and it could not be included in the calibration. There were only rare measurements on water quality available (mainly for the short period 1998–2000), which were partly real observed values and partly estimated ones. They could be used only as average values for the spatial model verification.
Calibration of water discharges and nutrient loads was performed by changing the model calibration parameters listed in the SWIM manual (Krysanova et al. Citation2000), and identified in former SWIM model applications (e.g. Hattermann et al. Citation2005, Hesse et al. Citation2008, Citation2012, Huang et al. Citation2009). The most sensitive and important calibration parameters for the water flows of the Vistula Lagoon catchment were: the correction factor for solar radiation (used to describe the intensity of evapotranspiration); the correction factor for saturated conductivity in soils (used to adjust water movement through the soil profile); the baseflow factor (used to calculate return flow travel time); and routing coefficients (used to correct the storage time constants for the reaches). A slight correction of temperature thresholds, defining when snowfall or snowmelt are starting, was important to catch the exact timing of snowmelt peaks in spring. The most important parameters for water quality calibration were the retention times and degradation rates of nutrients in soils, the soil water content threshold for denitrification of nitrate nitrogen, as well as parameters describing the ratios of adsorbed ammonium nitrogen and phosphate phosphorus in the soil particles to that in soil water.
4 RESULTS AND DISCUSSION
4.1 Model calibration
4.1.1 Hydrological calibration and validation
illustrates the results of model calibration and validation achieved for the two rivers: Pasleka at the Lozy gauge for the period 2005–2010 and Pregolya at Gvardeysk gauge for the period 1986–1996. The simulation results for both rivers show satisfactory to good model performance for the total calibration/validation period (NSE > 0.6), with slightly better results for the Pregolya River than the Pasleka. Total deviations in water balance are within ±5%. The long-term average daily discharges from the observed and simulated time series indicate a good agreement (, right). The discharge peaks in spring, resulting from snowmelt, as well as the level of low flow in summer, were reproduced quite well with the SWIM model.
Fig. 2 Performance of SWIM calculated for the total period and distinguished into calibration/validation periods for the two main rivers flowing to the Vistula Lagoon: Comparison of simulated and observed discharges as daily (left) and long-term average daily (right) dynamics for the Pasleka and Pregolya rivers.
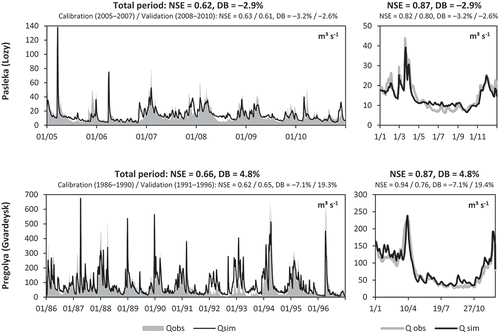
After hydrological calibration and validation for the two main rivers flowing to the Vistula Lagoon, the SWIM model was set up for spatial validation of the entire lagoon drainage area with a common calibration parameter set. The modelling results using WATCH forcing climate data for the period 1979–2009 were compared with all available measured discharge data. Altogether, 10 discharge gauges were taken into account (for location see ). The NSE and DB criteria are listed in , and show a satisfactory model performance in most cases. The best results in terms of NSE are achieved for the main gauges on Pasleka and Pregolya rivers and gauges located on their tributaries. This is understandable, as the parameter set was chosen after calibration for these sub-catchments. However, the discharge in smaller sub-catchments with short periods of measured data could also be reproduced quite well.
Table 3 Model performances at 10 discharge gauges located within the Vistula Lagoon catchment achieved by using the SWIM model covering the entire drainage area with a common parameter set.
As the last step in hydrological spatial validation, data found in the literature (Silicz Citation1975, Kruk et al. Citation2011, http://www.ebrd.com/pages/project/eia/balkalen.pdf) on long-term mean inflows to the lagoon coming from the 12 most important rivers were compared with the simulated average discharges for these rivers for the period 1980–2009 (). Though some minor discrepancies can be seen, in general the results show a good agreement, especially for the total water inflows to the Vistula Lagoon. The sum of water discharges for all simulated water courses flowing to the Vistula Lagoon almost perfectly fits the literature value. Differences are most obvious for the Elblag River, located in the lowest part of the catchment area and characterized by intensive water management measures and channelizing that were not represented by the model. This lowland region was formed by the former delta of the large Vistula River, which is now cut off from the Vistula Lagoon but still has some minor inflow controlled by water management measures.
4.1.2 Calibration and validation of water quality
illustrates results of water quality calibration and validation for the Pasleka River. The monthly observed data and seasonal dynamics derived from bi-weekly measurements for the period 2005–2009 are compared with the monthly mean values and seasonal dynamics derived from the simulated daily outputs for the same period. In general, the best results are achieved for water temperature and DO concentrations, mainly due to the simple physical relationships between these two variables and air temperature.
Fig. 4 Observed (obs) and simulated (sim) water quality variables for the Pasleka River (gauge Nowa Pasleka): monthly averages (left) and long-term average seasonal dynamics (right) of nitrate nitrogen (NO3-N), ammonium nitrogen (NH4-N), phosphate phosphorus (PO4-P), and dissolved oxygen (DO) concentrations, as well as water temperature for the period 2005–2009; the long-term average seasonal nutrient dynamics could be also compared with the SWIM simulated average monthly discharge (Qsim) at the same gauge.
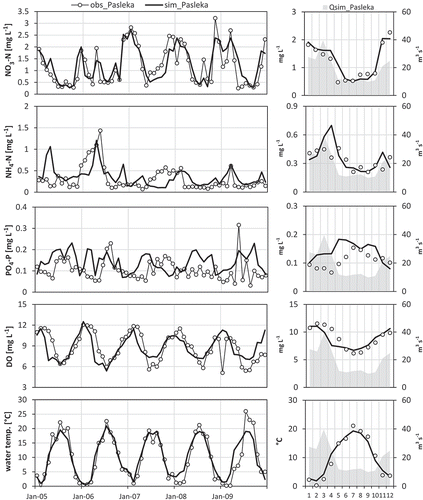
However, it is more complicated to simulate nutrient behaviour in the catchment, as these compounds are not simple conservative substances, but are subject to many transformation processes. They may be taken up by plants, bounded to soil particles (especially NH4-N and PO4-P) or degraded by chemical or biological decomposition processes (e.g. denitrification) in the catchment. Besides, the non-conservative transport processes cause retention or loss of nutrients in the catchment, which also influence simulation of water quality outputs.
Still, NO3-N concentrations are simulated quite well and better than other nutrients in the Pasleka basin. The general dynamic pattern, with high concentrations in winter and low concentrations in the low-flow summer months, was well reproduced. When comparing the seasonal dynamics of nitrate concentrations and average discharge (a high correlation between them is visible in ), it can be reasoned that NO3-N in the river originates mainly from diffuse sources.
A similar, but not so explicit, pattern can be seen for NH4-N (). Concentrations are higher in winter but lower in summer, so that in this case a prevailing diffuse-source pollution can be confirmed for the basin. The level of observed values was matched in the majority of cases, with some slight discrepancies during the year. Due to a high adsorption potential of NH4-N to soil particles, the transport with water flows through the catchment is less distinct than for NO3-N.
Phosphate phosphorus (PO4-P) shows an opposite seasonal dynamic behaviour (). Looking at long-term monthly averages, the conclusion can be drawn that this substance is coming to the river mainly from steady (over the year) point source emissions, leading to higher phosphate concentrations in summer with lower discharges (due to less dilution) than in winter with higher discharges. The monthly averages of the SWIM-simulated outputs for PO4-P are less good compared with the observations, but the average level and minimum/maximum values are captured.
lists the results of spatial model validation for water quality, using short periods of available data from 1980 to 2009, originating from the Mantra-East project (Margoński et al., 2003). The average nutrient and DO concentrations for the main rivers flowing to the Vistula Lagoon are compared with model outputs. As one can see (), in most cases, the observed levels are reached, although some discrepancies can be detected as well. The overestimation for the small river Vileyka (0.9% of the catchment area), bringing negligibly small loads (compare in ), should not influence the total load to the lagoon to any great extent. Larger problems can be seen for the Elblag and Pregolya rivers, where concentrations are mostly overestimated. Regarding the Elblag, the discrepancies can most probably be explained by discrepancies in the modelled discharge (see discussion above). Regarding the Pregolya, the discrepancies most probably occur due to missing information on pollution sources and non-calibrated nutrient processes for this river, for which only a few estimates on nutrient concentration were available.
Table 4 Spatial validation of water quality variables for nine rivers flowing into the Vistula Lagoon: comparison of the average of all available observed nutrient and dissolved oxygen (DO) concentrations with the average of the corresponding simulated values for the period 1998–2000 and some additional sub-periods in 1980–2009.
Table 5 Average daily nutrient loads of the 12 largest rivers entering Vistula Lagoon, as simulated with SWIM for the calibration/validation period 1980–2009.
lists simulated daily nutrient loads of 12 main rivers entering the Vistula Lagoon averaged for the period 1980–2009. In general, river loads increase with increasing catchment size (see ), with the sum of loads of all Russian rivers higher than the sum of loads from the Polish rivers. The Russian rivers discharge 60–75% of all nutrient loads to the lagoon (the share is smallest for NO3-N, and largest for NH4-N). However, not all loads entering the lagoon in the Russian part originate in this country, as large areas, especially of the Pregolya catchment, are located in Polish territory. Therefore, it seems that the catchment size and population amounts have higher influences on nutrient pollution than other potential factors.
As a result of quite poor data availability, some uncertainty still remains regarding nutrient processes in the drainage area under study, and the water quality results have to be treated with caution, especially for the non-calibrated parts. However, the results achieved on water quality in the entire catchment still provide a sound basis for climate impact assessment in the case study area.
4.2 Climate impact assessment
4.2.1 Climate change signals
The climate change signals, i.e. changes in long-term average temperature and precipitation between the reference and scenario periods, averaged over the whole Vistula Lagoon catchment and over all 15 scenarios, are listed in . The climate change signals for temperature show a continuous increase from period p1 to period p3. The increase amounts to approximately 1°C per 30-year-period. An increasing trend in precipitation of about 10% until the end of the 21st century is also projected for the Vistula Lagoon.
However, the values in are averaged over 15 climate scenario results. If single scenarios are analysed in the same way, differences between the scenarios are notable (). While most of the scenarios simulate an increase in precipitation, though with different amplitudes, a few of them project a decreasing trend. But, in general, it is obvious that the increasing precipitation trend is most likely. Regarding future temperatures, all scenarios agree on an increasing trend, but the increase for period p3 is variably projected to be in the range 2–5°C depending on the scenario.
Table 6 Annual climate change signals for the Vistula Lagoon catchment as the average of all 15 ENSEMBLES scenarios.
4.2.2 Climate impact on water quantity and quality
shows climate impacts on the total water discharge to the Vistula Lagoon, with the average daily dynamics of total water discharge in three scenario periods depicted on the left, and differences in total water discharge between these three periods and the reference period depicted as mean monthly values on the right. The average values from all simulations driven by 15 climate scenarios are shown for three future scenario periods as black lines, and can be compared with the average simulated values driven by simulated climate for the reference period 1971–2000 (dotted lines). The range of uncertainty is shown by degrees of grey shading. The outer uncertainty band (light grey) is defined by the maximum and minimum values of all the results driven by 15 climate scenarios, the medium range (darker grey) includes 10/90 percentiles, and the inner range (dark grey) is defined by the 25th and 75th percentiles of all the results and includes 50% of all simulation results around the average. The dark grey 25/75-percentile band indicates the range in which water discharge can be expected with a high probability.
Fig. 6 Dynamics of total water inflow to the Vistula Lagoon averaged for simulations driven by 15 ENSEMBLES climate scenarios: (left) long-term average daily discharges with percentile bands simulated in future periods compared to the long-term average daily discharge simulated in the reference period driven by simulated climate; and (right) absolute difference in monthly average discharges of three future periods compared to those simulated in the reference period 1971–2000. See text for explanation.
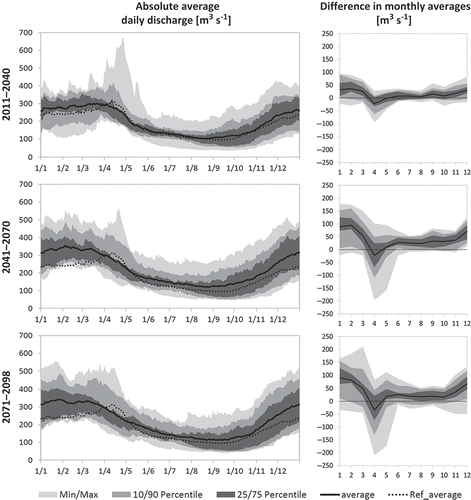
Due to a clear increasing trend in precipitation in this region, which was already detected during the climate scenario evaluation, an overall increase in river discharge to the Vistula Lagoon can be expected in all three scenario periods. The positive absolute changes in river discharge are largest in the winter months and lowest (but still positive) during the low-flow period in summer. The probability of increasing river discharge in winter is higher than 90% for all three periods.
The only negative change, consistent in all three scenario-periods, can be seen in April. This negative change is probably due to continuously increasing future average temperatures, as expected in all future climate scenarios. Higher temperatures have an impact on snowfall and snowmelt processes within the catchment. Snowmelt peaks will be lowered in the future, or may even disappear, as a result of less precipitation falling as snow in winter. This development is accompanied by higher winter discharge than simulated under reference climate conditions due to higher precipitation (mostly as rain) in the warmer winter months in future.
In general, differences in average monthly discharge compared to the reference period are lowest in the near future (2011–2040) and much higher towards the middle and end of the century, with increasing min/max-uncertainty bands, but with quite narrow 25/75-percentile bands. Therefore, an overall increase in water discharge in the future is quite certain, as projected by SWIM driven by the majority of 15 climate scenarios.
shows seasonal differences in water quality parameters between three scenario periods and the reference period as mean monthly values. The results are shown as means of simulations driven by all 15 ENSEMBLES scenarios (black lines) together with three uncertainty bands (light grey, darker grey and dark grey), as explained above for water discharge. A strong increasing trend throughout the century is obvious for water temperature, due to the overall increase in average air temperature, as already detected during evaluation of climate scenarios. The increase is highest for period p3, and reaches 2–3°C with a high probability.
Fig. 7 Impact of ENSEMBLES climate change scenarios on water quality parameters and nutrient loads coming into the Vistula Lagoon: average absolute changes in seasonal dynamics of water temperature (Twat), dissolved oxygen (DO), nitrate nitrogen (NO3-N), ammonium nitrogen (NH4-N) and phosphate phosphorus (PO4-P) calculated as the difference between SWIM-simulated mean monthly nutrient outputs under future climate conditions compared to results achieved under the simulated climate in the reference period.
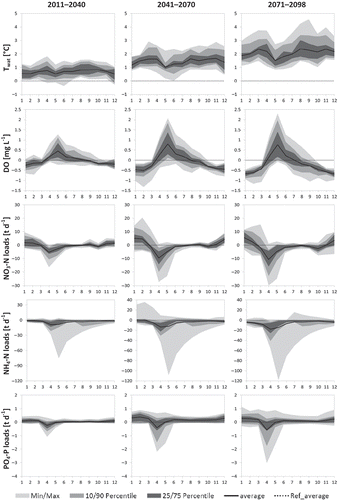
Changes in DO concentration can be linked to temperature change in the rivers, and could also be influenced by changes in NH4-N concentration in the river network. Dissolved oxygen concentrations are expected to decrease in winter months due to higher average water temperature with consequently lower values for saturated concentrations. Higher future DO concentrations than those in the reference period are simulated by SWIM for the period April–July. This can be explained by lower ammonium concentrations in the river network during these months.
Changes in NO3-N loads are quite similar to the changes in water discharge. In winter months (December–February) an increase in loads can be seen, followed by a decrease during April/May. During the drier summer months, almost no changes can be detected in the first two periods, and a very slight decrease in summer loads is visible for the last period 2071–2098. This is probably due to changing soil conditions influencing denitrification and mineralization potentials under a wetter and warmer climate. The behaviour of NO3-N resembles that of discharge due to its nature, being a pollutant that mainly comes from diffuse sources. Therefore, increasing precipitation results in higher NO3-N loads, as higher amounts are washed out from agricultural fields. The changes in loads are lower in the near future period 2011–2040, and reach larger amplitudes in the later periods that are accompanied by higher uncertainty ranges.
For NH4-N, a decreasing trend in loads can be observed. The maximum decrease is visible during the spring flood season, with minor negative changes throughout the year. This trend seems to be connected mainly to increasing temperatures in the course of the century, as mineralization processes and emergence of ammonium in soils are temperature related. Ammonium decrease could also be indirectly linked to a rising temperature, because higher temperatures increase evaporation potential in the region, cause lower water contents in soils and inhibit ammonium emergence during mineralization that is defined to occur at soil water contents above 80%. The min/max ranges shows high uncertainty amplitude for ammonium loads. This is caused mainly by one outlying future scenario (S14), which assumes much lower (winter) temperatures than the others in the reference period. This results in high ammonium occurrence, so that changes in ammonium loads for future periods in simulations driven by this scenario can be very high.
Phosphate phosphorus loads show a slightly increasing trend throughout the year, except April and May, when a decrease can be seen, as snowmelt happens less often in the projected future. The increase in loads is probably connected to increasing leaching processes, with higher precipitation amounts washing more phosphorus from sandy and highly permeable soils used for agriculture. The changes are smaller in the first future period due to smaller changes in precipitation, and higher in the second and third periods, with increasing uncertainty.
After applying the set of 15 climate scenarios to the Vistula Lagoon catchment, percentage changes of total outputs from the catchment (=inputs to the lagoon) were calculated for every scenario and every future period compared to the results achieved for the reference period of the same scenario. summarizes the effects of projected climate impacts on water quantity and quality. The average values of all changes per variable, as well as the uncertainty ranges estimated considering 15 simulations driven by 15 climate scenarios, are presented.
Fig. 8 Ranges of percentage changes of total discharge and nutrient inputs to the Vistula Lagoon as well as of average water temperatures and dissolved oxygen concentrations simulated with SWIM driven by 15 ENSEMBLES climate scenarios (future periods (p1, p2, p3) compared to reference period (p0) of the same scenario). The box plots visualize the following ranges: min/max, 25/75-percentile, median and average (dots).
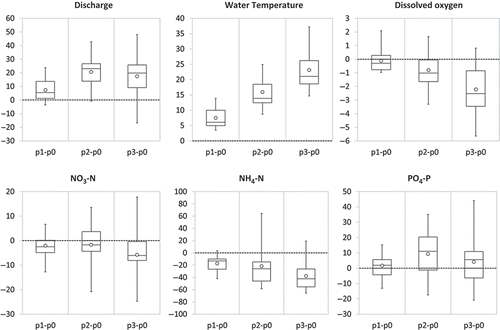
The model outputs can be divided into two groups, with regard to which climate parameter has the prevailing influence on its trend in future. The first group of variables (water temperature, DO and NH4-N) is obviously mainly connected to the increasing trend in temperature, though with different signs. Rising temperatures cause an increase in water temperature, but a decrease in transported ammonium loads, due to inhibited ammonium genesis in warmer soils in the catchment. Simulated future concentrations of DO in the rivers mainly result from higher temperature and, consequently, lower oxygen saturation concentration. Partly increasing oxygen concentrations during the course of the year as a result of lower ammonium content in the rivers do not reverse the overall trend.
The second group of variables (water discharge, NO3-N and PO4-P) is mostly connected to the precipitation trend projected for the future and the increasing water flows through catchment soils. For NO3-N, an increase in loads can be observed in winter months (conforming to the discharge behaviour), but, on average, a slight decrease is projected due to the lower or missing snowmelt peak in spring, and possibly increased loss of NO3-N by denitrification in soils due to higher soil water content in the wetter climate. Changes in PO4-P loads are obviously connected to the projected future precipitation changes.
illustrates some results of spatial analysis of the ENSEMBLES scenarios-driven outputs for the selected mid-term period 2041–2070 (p2). The percentage changes were calculated for the 12 main rivers flowing into the Vistula Lagoon by comparing the average discharge or load in the future period (p2) with the corresponding values simulated for the reference period (p0) of the same scenario. The graph shows the average change of all 15 scenarios per river catchment together with their minimum-maximum interval and the 25th and 75th percentile values.
Fig. 9 Spatial variability of projected climate change impacts on water discharge (Q), nitrate nitrogen loads (NO3-N), ammonium nitrogen loads (NH4-N), and phosphate phosphorus loads (PO4-P) for the mid-term scenario period 2041–2070. Compared are the reference and future average model outputs of the 12 main rivers draining to Vistula Lagoon per ENSEMBLE scenario S1–S15. The graphs show the average, minimum, and maximum changes of 15 scenarios in percentage terms as well as the 25- and 75-percentiles.
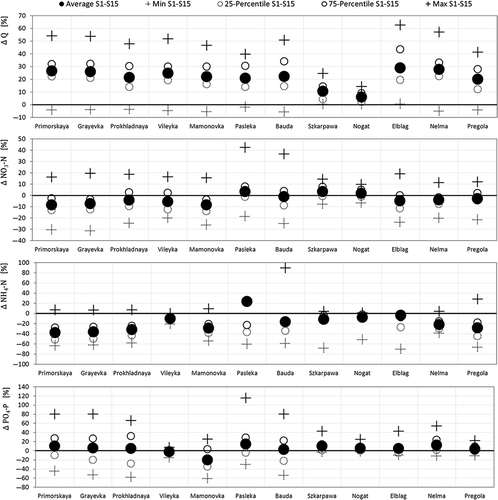
Looking at the graphs, the overall trend for the different model variables, as shown in , can generally be detected, but with some outlying catchments. Changes in discharge are smallest for the Szkarpawa and Nogat rivers, as a result of model set-up and implemented water transfer from the Weichsel River with constant monthly values, which masks the climate impacts. The other catchments show similar percentage increases in future river discharge as an average for the whole Vistula Lagoon catchment. Nutrient loads of most rivers also behave similarly to the overall trend. Some outliers are mainly the result of a single outlying scenario (e.g. scenario S1 generates unrealistically high changes in nitrogen loads for the Pasleka River basin). Regarding phosphorus loads, the Mamonovka basin shows the highest anomaly and a decreasing trend, on average.
The spatial analysis of scenario results shows that the model outputs are highly sensitive to local extreme climate events, as well as temporal undifferentiated management measures (e.g. constant water transfer from other catchments or constant input of point source emissions to the river reaches) that contribute to the general uncertainty of regional climate impact studies. The spatial climate variability, as projected by one single scenario or between different climate scenarios, can highly influence average results. But averaging model outputs driven by a set of scenarios diminishes the influence of a single scenario, and provides a better approach for climate impact assessment with more reliable results.
4.3 Discussion
The results of our study on projected impacts of climate change on water discharge and nutrient loads draining to the Vistula Lagoon can be compared with results of similar studies for the entire Baltic Sea drainage area or single river catchments in the Baltic region. There are some similarities and some differences between the model outputs, as climate impact assessments resulted in diverse future projections in this region, sometimes even in the directions of change. A special climate change impact assessment specifically for the Vistula Lagoon catchment could not be found in the literature.
In general, most climate scenarios project increasing temperature and precipitation for the entire Baltic region (e.g. Graham Citation2004, Meier et al. Citation2012, Arheimer et al. Citation2012), but with some spatial variability. Precipitation increase is projected to be lowest in the southern part of the Baltic Sea region. Graham (Citation2004) lists precipitation changes between +3% and +8% for the Baltic Proper area (the Vistula Lagoon catchment belongs to this region), whereas regions located in the north of the Baltic Sea region show precipitation changes by about +20% or even +30%. The moderate changes projected for the Baltic Proper region conform to the climate change signals calculated in our study.
A decrease in future nitrogen loads and a slight increase in phosphorus loads, as projected in our study, can be found also in Arheimer et al. (Citation2012), who performed climate impact assessment for the entire Baltic Sea drainage area with four different climate scenarios. The most probable reasons identified by the authors are natural removal of nitrogen along the water flow paths towards the sea in a climate with increased denitrification potential, increased nutrient mineralization due to higher temperatures, as well as increased phosphorus erosion due to more intensive rains.
Consistent with results of Graham (Citation2004), Arheimer et al. (Citation2012) detected the largest changes in water discharge for the northern part of the Baltic Sea basin (mainly in winter with less snow accumulation and lower or missing snowmelt peak), whereas projections for river discharge in the southern part of the Baltic region are negligible (Arheimer et al. Citation2012) or even negative (Graham Citation2004, Jakimavicius and Kriauciuniene Citation2013). This differs from our findings on future river discharge to the Vistula Lagoon, also located in the south of the Baltic Sea drainage area.
Piniewski et al. (Citation2013a) performed a combined climate and land-use change impact assessment for a small Polish river catchment draining to the Baltic Sea using the SWAT model, and investigated changes in water discharge and nitrate and phosphate loads under several scenarios. The scenarios covered different assumptions on potential future land uses and management measures, but used only one possible future climate derived from a combination of ECHAM5 (GCM) and RCA3 (RCM) for the period around 2050. Looking only at the climate impact of this scenario (with analogous climate change signals for precipitation, as in our 15 climate scenarios on average, but with a lower temperature increase than in our study), we can see that the direction of change is similar to our results for discharge and phosphate, but different for nitrate. Contrary to the results of our study, nitrate loads in Piniewski et al. (Citation2013a) increase together with discharge and phosphate loads, each by about 20%. The latter results for nitrogen and phosphorus conform with the results of Meier et al. (Citation2012), projecting an increase in nutrient loads coming from land to the Baltic Sea in all four climate projections due to increased flows (a rise of between 15 and 20%) caused by increased precipitation. Consequently, increased precipitation and river discharges would reduce salinity and enhance eutrophication in the coastal areas, along with the rising danger of increased flood intensity and frequency caused by sea-level rise (Voss et al. Citation2011).
However, all authors mentioned above detected a wide range of projected changes between simulations driven by different climate scenarios as well as a high spatial variability when looking at impacts driven by one scenario. Some regional trends can even be opposite to the overall trend for large areas (Arheimer et al. Citation2012), and some local effects can be masked by the large-scale aggregation (Piniewski et al. Citation2013a). They also noticed that the difference between the results achieved with one hydrological model under two or more different climate scenarios is sometimes larger than the difference between the results of different hydrological models or different management measures achieved by using one climate scenario (Arheimer et al. Citation2012, Piniewski et al. Citation2013a, Citation2013b). Hence, a large uncertainty is related to climate models and downscaling methods, which can be partly compensated for by applying an ensemble of different climate scenarios (Tebaldi and Knutti Citation2007, Huard Citation2011, Arheimer et al. Citation2012).
However, there is no consensus on the usefulness of the ensemble method within the research community, nor on the reliability of climate models and their scenarios. Some authors complain that scenarios from climate models are not ready for application in water resources management due to the high uncertainty of GCMs passed to RCMs, and to their poor fit to real observations of temperature and, especially, precipitation at regional and local scales, where climate impact assessment at the river basin scale usually takes place (Kundzewicz and Stakhiv Citation2010, Anagnostopoulos et al. Citation2010, Koutsoyiannis et al. Citation2011). Therefore, it is suggested that climate scenarios and hydrological models should not be applied uncritically (Kundzewicz and Stakhiv Citation2010, Wilby Citation2010), and it should be kept in mind that they probably hide or underestimate future uncertainty (Koutsoyiannis et al. Citation2011). However, due to the current absence of an ultimate and more reliable method to project future climate changes in regional impact assessment, the application of a set of state-of-the-art climate scenarios and the calculation of an average change and variability seems to be the best way at the moment to solve the research question of this study.
Nevertheless, even with possible improved climate models, the uncertainty about future socio-economic pathways hinders perfect regional hydrological projections, so that they still will be highly uncertain (Wilby Citation2010). This is especially the case for water quality modelling and can be already ranked as critical for the recent scenario period, in case this period would undergo a dramatic political and economic transformation process. Such a transition process, for example, could be observed during the last two decades in Poland. This is characterized by changes in both the agricultural sector and the handling of point-source emissions, and resulted in significant declining trends in nutrient loads and concentrations (Kowalkowski et al. Citation2012, Pastuszak et al. Citation2012). Despite the fact that the reference period is 30 years and covers such a transition period, our model was set up with data valid for the first decade of the 21st century. This procedure can be acceptable in this particular case, as water quality calibration mainly used data observed or estimated in or for the period 2000–2009, and all management measures could be assumed unchanged in climate impact assessment to investigate the ‘pure’ climate impact on the catchment processes. However, one should be aware that the absolute values of nutrient loads simulated for the reference period 1971–2000 only approximately represent the real nutrient amounts that entered the Vistula Lagoon during this period. Nevertheless, this method still delivers a sound basis for evaluation of relative changes in nutrient loads caused by changing climate conditions.
5 SUMMARY AND CONCLUSIONS
The main objective of the study—to perform a climate impact assessment for the catchment area of the Vistula Lagoon—was reached. For that, the commonly used technique for hydrological impact studies at the catchment scale was applied, using a set of 15 climate scenario data provided by the ENSEMBLES project covering three 30-year periods until the end of the 21st century, and the ecohydrological model SWIM. The obtained model outputs are provided to the Vistula Lagoon modellers in the framework of the European LAGOONS project for investigating climate impacts on the ecosystem of the lagoon.
An ecohydrological model used for impact assessment should be calibrated and validated in advance. Hydrological calibration of SWIM in the Vistula Lagoon catchment was a very complicated task. Primarily, this was due to mostly poor and inconsistent input data, missing information on small rivers, and the heterogeneity of spatial data. Despite all the difficulties, the results of model calibration/validation were quite satisfactory, creating a sound basis for the climate impact assessment. The applied method of calibration/validation can be used for other coastal areas and partly-gauged drainage basins.
Analysis of potential future climate, as projected by 15 scenarios for the Vistula Lagoon catchment, indicates an increasing trend for both temperature and precipitation. However, observing the climate change signals for 15 scenarios separately, we can see that there are significant differences between them: some models project a higher increase in temperature or precipitation, and other models, lower than average or even a decrease regarding precipitation.
The results of climate impact assessment on water discharge in the Vistula Lagoon catchment show a notable increase by 7, 21 and 18%, on average, in the three future scenario periods. The increasing trend in water temperature (projected between 8 and 23%) and the decreasing trend in dissolved oxygen concentrations (up to –2%) are consistent between scenarios. Nitrate nitrogen and NH4-N demonstrate decreasing trends in all three future periods, varying between –2% and –6% for NO3-N loads, and between –17% and –38% for NH4-N loads, on average, although the level of agreement between scenarios varies between periods and components. In contrast, PO4-P loads are expected to increase slightly, according to the obtained results, by 2–9%, on average. The uncertainty ranges are moderate.
Although expected changes in climate can be partly seen even as beneficial for this region, and the projected impacts seem to be not so strong, one should not forget about water-related extreme events such as floods and droughts, which were not investigated in this study. In particular, the flood intensity and frequency could increase in view of increasing precipitation, as projected by the ENSEMBLES scenarios, and increasing average monthly discharge (especially in winter time), as projected by SWIM in this study. Thus, it would be wise to extend this study to exploring the floods under climate change in this region.
According to the obtained results, impacts are expected to be less significant in the near future, but would increase in the middle of the century. Therefore, it is recommended to think about possible adaptation measures in the medium-term, which could be effectively supported by additional model application. The study on climate change impact could be extended to investigation of land use and management effects on water quantity and quality in the Vistula Lagoon catchment, which can be analysed separately or in combination with climate change and could help to find an adaptation strategy.
Disclosure statement
No potential conflict of interest was reported by the author(s).
Acknowledgements
We gratefully acknowledge data provision by the Institute of Meteorology and Water Management‐National Research Institute (IMGW-PIB), and the State Environmental Monitoring (PMŚ) in Poland, as well as the Kaliningrad Regional Center for Hydrometeorology and Environmental Monitoring (Kaliningrad HYDROMET) in Russia. The authors thank Vanessa Wörner and Lasse Scheele for their support in climate scenario data download, scenario evaluation and pre- and post-processing of SWIM input and output data.
Additional information
Funding
REFERENCES
- AG Boden, 2005. Bodenkundliche Kartieranleitung. 5th ed. Hannover: AG Boden.
- Anagnostopoulos, G.G., et al., 2010. A comparison of local and aggregated climate model outputs with observed data. Hydrological Sciences Journal, 55 (7), 1094–1110. doi:10.1080/02626667.2010.513518
- Anthony, A., et al., 2009. Coastal lagoons and climate change: ecological and social ramifications in U.S. Atlantic and Gulf coast ecosystems. Ecology and Society, 14 (1), 8. Available from: http://www.ecologyandsociety.org/vol14/iss1/art8/
- Arheimer, B., Dahné, J., and Donnelly, C., 2012. Climate change impact on riverine nutrient load and land-based remedial measures of the Baltic Sea action plan. Ambio, 41, 600–612. doi:10.1007/s13280-012-0323-0
- Arnold, J., Allan, P., and Bernhardt, G., 1993. A comprehensive surface–groundwater flow model. Journal of Hydrology, 142, 47–69. doi:10.1016/0022-1694(93)90004-S
- Burakowska, H., et al., 2005. BERNET CATCH regional report: RIVER PASŁĘKA, water management plan: provisional management plan pursuant to the water framework directive. Gdansk: Regional Water Management Board.
- Chapman, P.M., 2012. Management of coastal lagoons under climate change. Coastal Lagoons in a changing environment: understanding, evaluation and responding. Estuarine, Coastal and Shelf Science, 110, 32–35. doi:10.1016/j.ecss.2012.01.010
- Chubarenko, B., Navrotskaya, S., and Stont, Z., 2012. Long-term trends of meteorological and hydrological characteristics for coastal zone of the Kaliningrad Oblast (Russia): Report of subcontract work within the BaltAdapt Project between the Permanent International Secretariat of the Council of the Baltic Sea States (CBSS), the Client, and the Baltic Institute of Ecology of Hydrosphere (BIEH), the Contractor. Kaliningrad.
- Dailidienė, I., et al., 2011. Long term water level and surface temperature changes in the lagoons of the southern and eastern Baltic. Oceanologia, 53 (TI), 293–308. doi:10.5697/oc.53-1-TI.293
- Dojlido, J.R., Niemirycz, E., and Morawiec, P., 1994. Nutrient loads in the Vistula River—outflow into the Baltic Sea. In: N.E. Peters, et al., eds. Hydrological, chemical and biological processes of transformation and transport of contaminants in aquatic environments (Symposium proceedings, May, Rostov-on-Don). Wallingford, UK: International Association of Hydrological Sciences, IAHS Publ. 219, 205–215.
- Eriksson, H., et al., 2007. Nitrogen budgets of the Polish agriculture 1960–2000: implications for riverine nitrogen loads to the Baltic Sea from transitional countries. Biogeochemistry, 85, 153–168. doi:10.1007/s10533-007-9126-y
- FAO, 2003. Fertilizer use by crops in Poland. First version. Rome: FAO.
- Graham, L.P., 2004. Climate change effects on river flow to the Baltic Sea. Ambio, 33 (4), 235–241.
- Graham, L.P., et al., 2007. On interpreting hydrological change from regional climate models. Climatic Change, 81, 97–122. doi:10.1007/s10584-006-9217-0
- Hattermann, F.F., et al., 2005. Runoff simulations on the macroscale with the ecohydrological model SWIM in the Elbe catchment—validation and uncertainty analysis. Hydrological Processes, 19, 693–714. doi:10.1002/hyp.5625
- HELCOM, 2012. BaltHazAR II project, Component 2.2: Building capacity within environmental monitoring to produce pollution load data from different sources for e.g. HELCOM pollution load compilations. Appendix 3b: Testing of nutrient load model (FyrisNP) for the River Mamonovka catchment. Kaliningrad: Russian Academy of Sciences, Atlantic Branch of P.P.Shirshov Institute of Oceanology (ABIORAS), Laboratory for Coastal Systems Study.
- Hesse, C., et al., 2008. Eco-hydrological modelling in a highly regulated lowland catchment to find measures for improving water quality. Ecological Modelling, 218, 135–148. doi:10.1016/j.ecolmodel.2008.06.035
- Hesse, C., Krysanova, V., and Voß, A., 2012. Implementing in-stream nutrient processes in large-scale landscape modeling for the impact assessment on water quality. Environmental Modeling & Assessment, 17 (6), 589–611. doi:10.1007/s10666-012-9320-8
- Hirabayashi, Y., et al., 2008. Global projections of changing risk of floods and droughts in a changing climate. Hydrological Sciences Journal, 53 (4), 754–772. doi:10.1623/hysj.53.4.754
- Huang, S., et al., 2009. From meso- to macro-scale dynamic water quality modelling for the assessment of land use change scenarios. Ecological Modelling, 220, 2543–2558. doi:10.1016/j.ecolmodel.2009.06.043
- Huard, D., 2011. Discussion of “A comparison of local and aggregated climate model outputs with observed data” A black eye for the Hydrological Sciences Journal. Hydrological Sciences Journal, 56 (7), 1330–1333. doi:10.1080/02626667.2011.610758
- IPCC (Intergovernmental Panel on Climate Change), 2007. Climate change 2007: impacts, adaptation and vulnerability. Contribution of Working Group II to the Fourth Assessment Report of the Intergovernmental Panel on Climate Change. Cambridge: Cambridge University Press.
- IPCC-TGCIA, 1999. Guidelines on the use of scenario data for climate impact and adaptation assessment. Version 1. Prepared by T.R. Carter, M. Hulme, and M. Lal. Intergovernmental Panel on Climate Change, Task Group on Scenarios for Climate Impact Assessment.
- Jakimavicius, D. and Kriauciuniene, J., 2013. The climate change impact on the water balance of the Curonian Lagoon. Water Resources, 40 (2), 120–132. doi:10.1134/S0097807813020097
- Koutsoyiannis, D., et al., 2011. Scientific dialogue on climate: is it giving black eyes or opening closed eyes? Reply to “A black eye for the Hydrological Sciences Journal” by D. Huard. Hydrological Sciences Journal, 56 (7), 1334–1339. doi:10.1080/02626667.2011.610759
- Kowalkowski, T., et al., 2012. Differences in emission of nitrogen and phosphorus into the Vistula and Oder basin in 1995–2008—Natural and anthropogenic causes (MONERIS model). Journal of Marine Systems, 89 (1), 48–60. doi:10.1016/j.jmarsys.2011.07.011
- Kruk, M., Rychter, A., and Mroz, M., eds., 2011. Zalew Wislany. Środowisko przyrodnicze oraz nowoczesne metody jego badania na przykładzie projektu VISLA. Elbląg: Wydawnictwo Państwowej Wyższej Szkoły Zawodowej, 21–50.
- Krysanova, V., et al., 1989. Simulation modelling of the coastal waters pollution from agricultural watershed. Ecological Modelling, 49, 7–29. doi:10.1016/0304-3800(89)90041-0
- Krysanova, V., et al., 2000. SWIM (Soil and Water Integrated Model): user manual. Potsdam: Potsdam Institute for Climate Impact Research, PIK Report no. 69.
- Krysanova, V., et al., 2015. Modelling climate and land-use change impacts with SWIM: lessons learnt from multiple applications. Hydrological Sciences Journal, 60 (4). doi:10.1080/02626667.2014.925560
- Kundzewicz, Z.W. and Stakhiv, E.Z., 2010. Are climate models “ready for prime time” in water resources management applications, or is more research needed? Hydrological Sciences Journal, 55 (7), 1085–1089. doi:10.1080/02626667.2010.513211
- Lehmann, A., Getzlaff, K., and Harlaß, J., 2011. Detailed assessment of climate variability in the Baltic Sea area for the period 1958 to 2009. Climate Research, 46, 185–196. doi:10.3354/cr00876
- Lloret, J., Marín, A., and Marín-Guirao, L., 2008. Is coastal lagoon eutrophication likely to be aggravated by global climate change? Estuarine, Coastal and Shelf Science, 78 (2), 403–412. doi:10.1016/j.ecss.2008.01.003
- Mantra-East, 2004. Estimation of nutrient loads and future scenarios for the nutrient loads to the Vistula Lagoon. Przedrzymirska, J. and Lewandowski, A., Mantra-East Deliverable D5dVL.
- Margoński, P., et al., 2003. Database and technical report on rivers and the Vistula Lagoon water quality, Mantra-East Deliverable D3cVL.
- Markova, L. and Nechay, I., 1960. Hydrological sketch of estuary of Neman and Pregolya Rivers. Proceedings of GOIN, 49, 118–157.
- Marszelewski, W. and Skowron, R., 2006. Ice cover as an indicator of winter air temperature changes: case study of the Polish Lowland lakes. Hydrological Sciences Journal, 51 (2), 336–349. doi:10.1623/hysj.51.2.336
- Meier, H.E.M., et al., 2012. Modeling the combined impact of changing climate and changing nutrient loads on the Baltic Sea environment in an ensemble of transient simulations for 1961–2099. Climate Dynamics, 39, 2421–2441. doi:10.1007/s00382-012-1339-7
- Moriasi, D.N., et al., 2007. Model evaluation guidelines for systematic quantification of accuracy in watershed simulations. Transactions of the ASABE, 50 (3), 885–900. doi:10.13031/2013.23153
- Nash, J.E. and Sutcliffe, J.V., 1970. River flow forecasting through conceptual models part I: a discussion of principles. Journal of Hydrology, 10 (3), 282–290. doi:10.1016/0022-1694(70)90255-6
- Nicholls, R.J., et al., 2007. Coastal systems and low-lying areas. In: M.L. Parry, et al., eds. Climate change 2007: impacts, adaptation, and vulnerability. Contribution of Working Group II to the Fourth Assessment Report of the Intergovernmental Panel on Climate Change. Cambridge: Cambridge University Press.
- NRC, 2010. Adapting to the impacts of climate change. National Research Council. Washington, DC: The National Academies Press.
- Pastuszak, M., et al., 2012. Response of Polish rivers (Vistula, Oder) to reduced pressure from point sources and agriculture during the transition period (1988–2008). Journal of Marine Systems, 94, 157–173. doi:10.1016/j.jmarsys.2011.11.017
- Piniewski, M., et al., 2013a. Climate change and agricultural development: adapting Polish agriculture to reduce future nutrient loads in a coastal watershed. Ambio, 1–17. doi:10.1007/s13280-013-0461-z.
- Piniewski, M., et al., 2013b. Effect of modelling scale on the assessment of climate change impact on river runoff. Hydrological Sciences Journal, 58 (4), 737–754. doi:10.1080/02626667.2013.778411
- Rautio, L.M., et al., (ed.) 2006. BERNET CATCH Theme Report: Public participation and water management in the Baltic Sea region. Regional implementation of the EU Water Framework Directive in the Baltic Sea Catchment.
- Reihan, A., et al., 2007. Changes in water discharge of the Baltic States rivers in the 20th century and its relation to climate change. Nordic Hydrology, 38 (4–5), 401–412. doi:10.2166/nh.2007.020
- Robakiewicz, M., 2010. Vistula River Mouth—history and recent problems. Archives of Hydro-Engineering and Environmental Mechanics, 57 (2), 155–166.
- Scavia, D., et al., 2002. Climate change impacts on U.S. Coastal and Marine ecosystems. Estuaries, 25 (2), 149–164. doi:10.1007/BF02691304
- Schneider, U., et al., 2013. GPCC’s new land surface precipitation climatology based on quality-controlled in situ data and its role in quantifying the global water cycle. Theoretical and Applied Climatology. doi:10.1007/s00704-013-0860-x.
- Silicz, M.W., 1975. Water balance. In: N. Lazarenko and A. Majewski, eds. Hydrometeorological regime of Vistula Lagoon. Warsaw: Wydawnictwa Komunikacji i Łączności, 267–270 (in Polish).
- Simas, T., Nunes, J.P., and Ferreira, J.G., 2001. Effects of global climate change on coastal salt marshes. Ecological Modelling, 139, 1–15. doi:10.1016/S0304-3800(01)00226-5
- Stefan, H.G. and Preud’homme, E B., 1993. Stream temperature estimation from air temperature. Journal of the American Water Resources Association, 29 (1), 27–45. doi:10.1111/j.1752-1688.1993.tb01502.x
- Tebaldi, C. and Knutti, R., 2007. The use of the multi-model ensemble in probabilistic climate projections. Philosophical Transactions of the Royal Society, 365, 2053–2075. doi:10.1098/rsta.2007.2076
- Teutschbein, C. and Seibert, J., 2010. Regional climate models for hydrological impact studies at the catchment scale: a review of recent modeling strategies. Geography Compass, 4 (7), 834–860. doi:10.1111/j.1749-8198.2010.00357.x
- van der Linden, P. and Mitchell, J.F., eds., 2009. ENSEMBLES: climate change and its impacts: summary of research and results from the ENSEMBLES project. Exeter: Met Office Hadley Centre.
- Voss, M., et al., 2011. History and scenarios of future development of Baltic Sea eutrophication. Estuarine, Coastal and Shelf Science, 92 (3), 307–322. doi:10.1016/j.ecss.2010.12.037
- Weedon, G.P., et al., 2011. Creation of the WATCH forcing data and its use to assess global and regional reference crop evaporation over land during the twentieth century. Journal of Hydrometeorology, 12, 823–848. doi:10.1175/2011JHM1369.1
- Whitehead, P.G., et al., 2009. A review of the potential impacts of climate change on surface water quality. Hydrological Sciences Journal, 54 (1), 101–123. doi:10.1623/hysj.54.1.101
- Wibig, J. and Jakusik, E., eds., 2012. Climatic and oceanographic conditions in Poland and in South Baltic—expected changes and recommendations for development of adaptation strategies for the national economy. Warszawa: Instytut Meteorologii i Gospodarki Wodnej-Państwowy Instytut Badawczy, 283 (in Polish).
- Wilby, R.L., 2010. Evaluating climate model outputs for hydrological applications. Hydrological Sciences Journal, 55 (7), 1090–1093. doi:10.1080/02626667.2010.513212