ABSTRACT
This study focuses on the variability of lake evaporation and also the periodic relationships among hydro-meteorological variables. The monthly hydro-meteorological data of Lake Keban were investigated by wavelet transforms. The results show that the main periodicity is on an annual scale. This periodicity is weaker for precipitation and wind speed but higher for evaporation, temperature, runoff and relative humidity. In addition to this, the continuous wavelet figures show some weak periodicities on the almost 10-year scale level but they are not continuous over time. Also, strong events on a short-term monthly scale are seen for evaporation, precipitation and runoff in 1988. This event in 1988 may be explained by the 1988 La Niña event, which was one of the strongest on record. Also, the periodicities on the 2–8-month scales in the precipitation data can be interpreted as being connected with the strong El Niño events of 1982 and 1992.
Editor D. Koutsoyiannis; Associate editor A. Carsteanu
1 Introduction
Evaporation is the process by which water changes from its liquid form to its gas form. Approximately 80% of all evaporation is from the oceans, with the remaining 20% coming from inland water and vegetation.
Knowledge of variability in evaporation is important to many researchers in water resources, agriculture and climate change. Variations in lake evaporation have a significant impact on the energy and water budgets of lakes (Lenters et al. Citation2005). Although more than two-thirds of the world is covered with water, the amount of useable water is not adequate in some places (Gökbulak and Özhan Citation2006). Because of this, analysis and variability of the water loss through evaporation from the water surfaces of lakes and dammed reservoirs is critically important. The rate of evaporation depends especially upon wind speed (the higher the wind speed, the greater the evaporation), temperature (the higher the temperature, the greater the evaporation) and humidity (the lower the humidity, the greater the evaporation). Furthermore, evaporation is associated with precipitation over the lake or dammed reservoir and the surrounding watershed and streamflow. Also, the long-term variability in lake evaporation together with varying climatic and hydrological characteristics must be studied in order to better understand the variations in evaporation (Lenters et al. Citation2005).
Generally, studies on lake evaporation include different methods of evaporation measurement, such as mass transfer, water balance methods and energy budget methods (Winter et al. Citation1995, Citation2003). Lenters et al. (Citation2005) researched a 10-year analysis of the seasonal, intra-seasonal, and inter-annual variations in lake evaporation for Sparkling Lake in northern Wisconsin (USA) using the energy budget method. These researchers found that intra-seasonal changes in evaporation are mainly associated with synoptic weather variations. The seasonal variations in evaporation are considered to be primarily through temperature and radiation. Mohamed et al. (Citation2004) studied spatial variability of evaporation in the swamps of the upper Nile by remote sensing techniques. In their studies, the derived actual evaporation, using the Surface Energy Balance Algorithm for Land (SEBAL), was used to verify moisture exchanges between the land surface and the atmosphere in a regional climate model. Assouline and Mahrer (Citation1996) characterized the variability in meteorological variables (air temperature, humidity, water surface temperature and wind velocity) and evaporation rates over Lake Kinneret, Israel. Some studies have shown that atmospheric circulation and rainfall are importantly affected by large-scale variations in evaporation and soil moisture (Mohamed et al. Citation2004).
The hydro-meteorological time series is known as a natural series and generally its statistical characteristics change over time. The wavelet transform is a useable tool for time-scale analysis of non-stationary signals, such as hydrological time series (Daubechies Citation1990, Polikar Citation1999, Pisoft et al. Citation2004). Wavelet analysis clearly shows a three-dimensional time–frequency image and overcomes the difficulty of determining variability using traditional methods (Holmgren et al. Citation2003). There are many applications for wavelet analysis in the literature. For example, wavelet transform was employed for streamflow characterization by Smith et al. (Citation1998), for analysis of El Niño Southern Oscillation by Torrence and Compo (Citation1998), and for research on the inter-decadal and inter-annual characteristics of precipitation (Lu Citation2002, Xingang et al. Citation2003, Penalba and Vargas Citation2004). Wavelet transforms are a very useful definition especially with respect to connections between hydro-meteorological variables, for example Nakken (Citation1999), Kulkarni (Citation2000), Labat et al. (Citation2000) and Yan et al. (Citation2004). In another study, Drago and Boxall (Citation2002) researched the relationship between sea-level variability and the meteorological variables of Malta. In their study, the atmospheric variables (wind speed, wind direction, air pressure, temperature, relative humidity and solar radiation) and the hourly averaged sea levels were decomposed by discrete wavelet transform and compared with each other in detail. None of these studies in the literature addressed the application of time-scale analysis to evaporation and the relationships between evaporation and hydro-meteorological data by wavelet transforms.
The approach adopted in this study aims to explore the periodic fluctuations in evaporation from the Lake Keban reservoir (Turkey). Also, periodic relationships between the relevant hydro-meteorological variables such as precipitation, relative humidity, wind speed, temperature, lake streamflow and evaporation are investigated. Continuous wavelet transformation presents the observed time series in terms of both time and scale. This involves a transform from a one-dimensional time series (or frequency spectrum) to a three-dimensional time–frequency image (Torrence and Compo Citation1998). Wavelet analysis will thus clearly show frequency and amplitude modulation of the climatic oscillations over time (Holmgren et al. Citation2003). Also, the evaporation data and the hydro-meteorological data can be decomposed into the wavelet sub-time series by the discrete wavelet transformation. So, the statistical interpretation of the wavelet components is presented. Continuous wavelet analysis on Turkey’s hydro-meteorological data has only recently been adopted for research into periodicities and short- or long-term variability.
2 Methods
2.1 Continuous wavelet transform
The wavelet transform is a time–frequency decomposition that uses wavelet functions localized in both time and frequency as the basis (Daubechies Citation1990). The wavelet transform, developed over the past few decades, appears to be a more effective tool than the Fourier transform (FT), which does not provide accurate time–frequency analysis for non-stationary signals (Coulibaly and Burn Citation2004). Wavelet analysis detects periodicities in the time series and also shows their time dependences (Taleb and Druyan Citation2003). Continuous wavelet transform provides an ideal opportunity to examine the process of energy variations in terms of where and when hydrological events occur (Küçük and Ağıralioğlu Citation2006).
A wavelet function ψ (τ,s) can be written as:
where t is time, s is wavelet scale, and τ is the time step in which the window function is iterated (Meyer Citation1993).
The basic wavelet function in wavelet analysis is obtained by dilation and translation of a Morlet wavelet function. The Morlet wavelet is complex and non-orthogonal:
where ω0 is non-dimensional frequency, and η is a non-dimensional time parameter (Anctil and Tape Citation2004). The advantage of the Morlet wavelet function is that it gives a good definition of the signal in spectral space (Coulibaly and Burn Citation2004).
The continuous wavelet coefficient is given by the convolution of x(t) with a scaled and translated ψ (τ,s):
where * indicates the conjugate complex function. By smoothly varying both s and τ, one can construct a two-dimensional picture of wavelet power, |W(τ,s)|2, showing the frequency (or scale) of the peaks in the spectrum of x(t), and how these peaks change with time (Drago and Boxall Citation2002).
The lower scales refer to a compressed wavelet and allow us to trace the abrupt changes or high-frequency component of a signal. On the other hand, the higher scales composed by the stretched version of a wavelet and the corresponding coefficients represent slowly progressing occurrences or low-frequency components of the signal.
The significance of the wavelet spectra can be determined by significance testing based on the background (or noise) spectrum (Coulibaly and Burn Citation2004). The noise spectrum depends on the nature of the data (Lafreniere and Sharp Citation2003). For the process, if the lag-1 autocorrelation coefficient (ρ) (lag-1 autoregressive parameter) is positive (ρ > 0), then the process is red noise (increasing variance with increasing scale). If the lag-1 autocorrelation coefficient is 0, then the process is white noise (constant variance across all scales) (Torrence and Compo Citation1998, Lafreniere and Sharp Citation2003). The continuous wavelet spectra of the time series are compared with the spectrum of the background process at the 95% confidence level (Lafreniere and Sharp Citation2003). For the noise process, more detailed knowledge is presented by Torrence and Compo (Citation1998) or Lafreniere and Sharp (Citation2003).
2.2 Discrete wavelet transform
In the discrete wavelet transforms, the wavelet coefficients are only obtained at defined scales. The most common wavelet scales are dyadic scales. This transform is called the discrete wavelet transform and is described by:
where m and n are integers that control, respectively, the scale and time; s0 is a specified fixed dilation step greater than 1; and τ0 is the location parameter and must be greater than zero. The most common choice for the parameters s0 and τ0 is 2 and 1 (time steps), respectively.
Discrete wavelet transforms that present the power of two logarithmic scalings of the translations are the most efficient solution for practical purposes (Mallat Citation1989). For a discrete time series xi, where xi occurs at discrete time i (i.e. here integer time steps are used), the discrete wavelet transform becomes:
where, is the wavelet coefficient for the discrete wavelet of scale
and location
. The discrete wavelet transform provides one or more detailed series and an approximation at different scales.
2.3 Global wavelet spectrum
Assuming a vertical slice through a wavelet plot as a measure of the local spectrum, the time-averaged wavelet spectrum over all specified periods or all the local wavelet spectra is then expressed as:
where T is the number of points in the time series. The time-averaged wavelet spectrum is generally called the global wavelet spectrum (GWS) (Torrence and Compo Citation1998). The smoothed Fourier spectrum approaches the GWS when the amount of necessary smoothing decreases with increasing scale. So, the GWS provides an unbiased and consistent estimation of the true power spectrum, which is a useful tool in the analysis of non-stationary time series. Spectral components are defined as the frequency in a power spectrum and periodic components are ordered according to period scales in a GWS. A global spectrum is calculated via the continuous spectrum; therefore, the initial and final time of the periodic components can also be determined.
3 Description of data
The daily evaporation rate from the water’s surface varies in the range 1–10 mm depending on the meteorological conditions (Bayazıt Citation2001). The evaporation rate is very important for lakes or dammed reservoirs. Water loss, especially through evaporation from lakes or reservoirs, is very serious across the globe, as for Turkey. For instance, water evaporation from the Keban Dam reservoir is estimated at almost 800 million m3 each year (Bayazıt Citation2001). shows total evaporation distribution (for the period May–October) over Turkey for 1975–2004 (State Meteorological Service). The Lake Keban reservoir (marked by the box in ) has significant water loss through evaporation. From it can be seen that the annual total evaporation for most of Turkey is more than 1000 mm.
Figure 1. (a) Total evaporation over Turkey for 1975–2004 (May–October). (b) Location of Lake Keban.
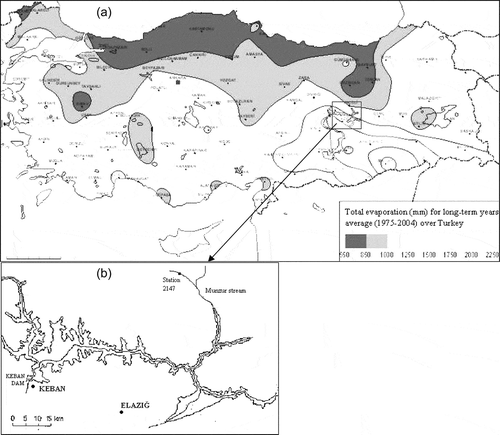
The evaporation amount cannot be measured directly from the water’s surface due to several difficulties. Evaporation can be determined using water balance or energy balance or mass transfer methods. Alternatively, it can be computed on the basis of measurements of pan evaporation by a coefficient (Bayazıt Citation2001). The pan coefficient varies between 0.70 and 0.75 for lake or reservoir surfaces (Gökbulak and Özhan Citation2006).
In this study, the wavelet transforms were undertaken on data for the Keban station, operated by the State Meteorological Service (DMI). The Keban meteorological station is located almost 3 km from the Keban Dam reservoir, which is in the East Anatolia Region (), located in the GAP (Southeast Anatolia Project) Region. The surface area of this reservoir is approximately 675 km2. The lake volume is approximately 31 000 hm3. The river runoff (R) data come from the Dedikusagi station (station no. 2147) on the Munzur stream in the Fırat basin ().
In addition, the meteorological time series for the monthly average of daily mean temperature (T), monthly total specific humidity (H), monthly total evaporation (E), monthly average of daily mean wind speed (W) and monthly total precipitation (P) are employed in the whole study. The time period of the monthly hydro-meteorological data is 30 years from 1973 to 2002 (runoff data are available for the period 1973–1997). The data were found to be homogeneous for this period (Partal and Cigizoglu Citation2009). Monthly values of the hydro-climatic variables in the period 1973–2002 are depicted in . Related statistical information for the hydro-meteorological data is presented in . The mean monthly total evaporation is 140 mm, while the maximum monthly total evaporation is 492 mm. On the other hand, the mean total precipitation is 30.4 mm, while the maximum monthly total evaporation is 174.4 mm. The average monthly runoff is 33 m3/s. It can be seen from that the runoff data show a scattered distribution (the skewness coefficient for the runoff data is 1.70). However, the skewness coefficients of the average temperature and the average relative humidity show quite low values. The cross-correlation between the monthly mean runoff and the monthly total precipitation is 0.31. The cross-correlation between the monthly mean temperature and the monthly total evaporation records has a significant value (0.93).
Table 1. Statistical information for the monthly data observed over 30 years (runoff data are available for the period 1973–1997). (Mean, Max, SD, Cs and r1 denote mean, maximum, standard deviation, skewness and lag-1 autocorrelation, respectively.) Also shown are the cross-correlations between the evaporation and the relevant hydro-meteorological data.
4 Application
4.1 Wavelet analysis of hydro-meteorological data
For the subject of this study, the continuous wavelet spectrum and GWS of the data were researched. The Morlet wavelet was used as the main wavelet function. The effective periodic events are seen as light regions on the continuous wavelet spectrum (CWS) and peaks of the GWS. The CWS and GWS of the monthly evaporation data are shown in . The dominance of the annual cycle (12 months) is evident in the GWS and the CWS of the data. The dashed white line is the cone of influence of the wavelet analysis depending on the red-noise process (Coulibaly and Burn Citation2004). This means that the white line presents periodic events statistically significant at the 95% confidence level for a red-noise process with determined lag-1 autocorrelation coefficient 0.82. The periodicities between the 16- and 128-month scale levels are seen between 1970 and 1979 and also after the 1990s. In addition, slightly weak inter-decadal periodicity is seen at the 250-month (nearly 20-year) scale level for the CWS and the GWS and it is continuous over time. However, this periodicity is not statistically significant and is not supported by the red-noise procedure. Vertical light lines between the 4- and 8-month scale levels can be seen from this figure. These lines show the probability of strong evaporation losses. The mentioned light lines appear for 1974, 1988 and the 1990s. In the GWS figure, a very weak peak appears in the region of the 120-month (nearly 10-year) scale level.
Figure 3. CWS (left) and GWS (right) of (a) monthly total evaporation, and (b) monthly average flow. White dashed lines indicate the 95% confidence limit for red noise (lag-1 coefficient is 0.82).
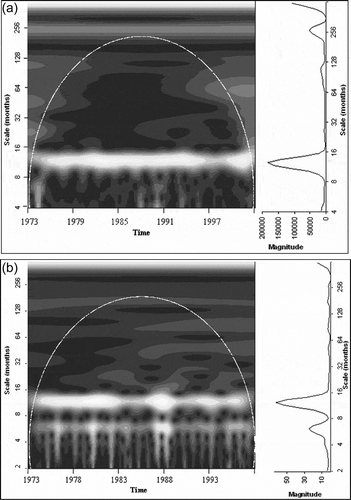
presents the results of the CWS and the GWS for the monthly average runoff at station 2147. The strong annual periodicity (12 monthly) is clearly apparent in the CWS and GWS. Some weak periodicities are seen between the 16- and 128-month scale levels. Also, weak periodicity appears in the region of the 128-month scale level. The vertical light lines seen in the figure refer to seasons with high runoffs. In particular, the strongest successive fluctuations in the flow records are seen in 1988. In addition, some of the short-term periodic events are seen in 1982 and 1993. The two strong peaks in 1982–1983 in the monthly runoff series are also easily seen in . The two peaks of the GWS show 6-monthly and 12-monthly strong periodicities in the runoff data.
and show the CWS and the GWS of the monthly hydro-meteorological data. The GWS of the precipitation clearly presents two peaks at the nearly 6-month and 12-month (annual) scale levels (). Weak periodicities are seen between the 16-month and 64-month scales. Strong precipitation events (seen as vertical bright light lines), such as the 2–8-month scales, are seen in the CWS. It is supposed that these events repeat every 3–5 years. One of these events appears in 1988, when strong runoff and evaporation events also occurred. In addition, strong events on the 2–8-month scale are seen in 1983, 1993 and 1997. The figures for wind speed and relative humidity show weak periodicity in the region of the 120-month scale (nearly 10-year) level but this is not seen continuously over time ( and ). In addition, annual periodicity is not continuous over time for wind speed, but it is strongly evident from 1973 to 1979. However, it becomes weaker, even unseen, after 1979. Other periodicities generally appear from 1973 to 1979, as seen in the CWS figures. The CWS and GWS of the temperature show a very strong periodicity on the annual scale ().
Figure 4. CWS (left) and GWS (right) of (a) monthly total precipitation, and (b) monthly averaged wind speed. White dashed lines indicate the 95% confidence limit for red noise (lag-1 coefficients are 0.31 and 0.65, respectively).
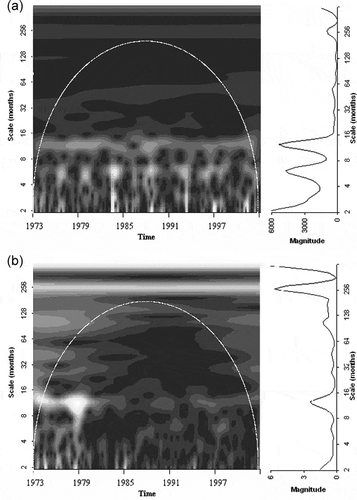
Figure 5. CWS (left) and GWS (right) of (a) monthly total relative humidity, and (b) monthly averaged temperature. White dashed lines indicate the 95% confidence limit for red noise (lag-1 coefficients are 0.75 and 0.84, respectively).
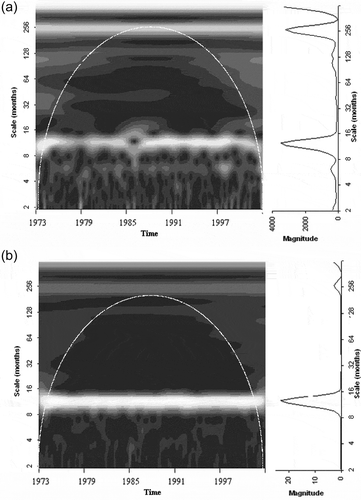
To compare two series, xn and yn, the cross-wavelet spectrum of the two series can be determined. The cross-wavelet spectrum can be computed as:
The evaporation–relative humidity cross-wavelet spectrum and cross-global wavelet spectra are shown in . The annual cycle can be clearly seen in the wavelet spectra. The 20-year periodicity is also seen but it is weaker. The cross-wavelet spectra of the evaporation and the runoff are seen in . The strong runoff events in the period 1986–1988 are evident in . After 1983, some weak periodicities are also seen between the 16-month and 128-month scale levels in .
4.2 Analysis of decomposed wavelet components of hydro-meteorological data
All of the data were decomposed into the various wavelet time series by the discrete wavelet transformation (DWT) for analysis of the relationships between the periodic components. The original signal is first decomposed into an approximation and the accompanying detail. While the approximation coefficients are obtained with a decomposition low-pass filter, the detail coefficients are obtained with a decomposition high-pass filter. The decomposition process is then iterated, with successive approximations being decomposed in turn, so that the original signal is broken down into many lower-resolution components (Mallat Citation1989). The wavelet sub-time series (D) presents variations of the data at the different scale levels. In this study, eight wavelet sub-time series (at the 2–4-, 8–16-, 32–64-, 128- and 256-month scale levels) and one approximation series were obtained by the DWT. Daubechies wavelet is the basis function for decomposition into different parts on different scales. shows the correlation coefficients between the wavelet components of the hydro-meteorological data (runoff, precipitation, humidity, wind speed and temperature) and of the evaporation series. For example, the correlation between the D1 component of the runoff and the D1 component of the evaporation is 0.411. In , while the D1 wavelet component refers to a 2-month scale level, D2 refers to a 4-month scale level. indicates that the D8 wavelet component of the runoff has the highest correlation (its value is 0.831). The D8 component comprises 256 monthly modes, namely nearly 20 years, and its mark can be seen from the wavelet spectra of the relevant data. The D3 component (8-month scale level) has the second highest correlation (its value is 0.529). For all the hydro-meteorological data, it can be clearly seen that the D3 component has the highest correlation with the evaporation wavelet component. The relationship between the D3 component of the relative humidity and that of the evaporation series is quite high but negative (its value is −0.960). For the average temperature, the correlation of the D3 component is 0.987. Similar results can be seen for the precipitation and wind speed. These results clearly show that the correlation results are parallel to the wavelet spectrum figures. For instance, the 8-month modes and 20-year modes are clearly significant for the evaporation–wind speed connection and similar results can be found for the other parameters.
Table 2. Correlations between the D components of the hydro-meteorological data and the evaporation series. Bold denotes the highest correlations.
also presents the correlations between the D components of the hydro-meteorological data and the observed evaporation data. The strong relationship between the D3 components can be seen clearly in . For instance, the D3 component of the precipitation data emphasizes a significant relationship with the observed evaporation data (the correlation coefficient is −0.724). However, the D8 component of the hydro-meteorological data does not show a connection with the observed evaporation data.
The time series of the wavelet coefficients (D components) are shown in for the specific periods. The long time periods (64 and 128 months) were selected for comparison of the wavelet coefficients. Differences in the wavelet coefficients are presented as a function of frequency within the intra-seasonal periods. For evaporation, runoff and precipitation, a clear peak is seen from the beginning of the 1980s to the beginning of the 1990s. In 64-month bands, the peak in the wavelet power of the runoff and the precipitation is clearly seen in 1988 (it refers to a strong runoff event). The relationship between the wind speed and the relative humidity is close. Fluctuations in the 128-month periods do not appear to correspond with the variables.
4.3 Tele-connections with North Atlantic Oscillation (NAO) and Southern Oscillation Index (SOI) and evaluation of the results
In this section, the wavelet components of the Turkish hydro-meteorological variables (evaporation, precipitation, streamflow, relative humidity, wind speed and temperature) are analysed in association with the SOI and the NAO. The effects of the SOI and NAO are commonly known to orchestrate large variations in climate. The extreme phases of the SOI (very negative or positive) are associated with El Niño (La Niña) events. Tele-connections with Turkey’s climatic data for the NAO and SOI were studied by Karabork et al. (Citation2005). This research indicated potential relationships for both precipitation and streamflow variables in connection with the NAO. The SOI showed weaker relationships in comparison with those for the NAO. In addition, they showed that the influence of the SOI on Turkish temperature data is negligible. Further, Kahya and Karabork (Citation2001) and Karabork and Kahya (Citation2003) researched the effects of El Niño and La Niña events on Turkey’s streamflow and precipitation data.
The SOI and NAO index for 1973–2002 are illustrated in . The short-term periodicities, such as the 2–8-month scales, in the Keban precipitation data can be related to the 1982 and 1992 strong El Niño events (). The strong short-term periodicities in the 1988 runoff data may be explained by the 1988 La Niña event, which was the one of the strongest La Niñas on record (). The same periodicity in 1988 can also be seen from the evaporation CWS (). Also, the 2–8-month periodicity in 1974 in the Keban evaporation data may be considered to be related to the strong La Niña event ().
The correlations between the D component of the hydro-meteorological data and the original SOI and NAO index were researched for the period 1973–2002 (). The observed data show correlations with the SOI at 0.08 or lower. These correlations are not significant at the α = 0.05 level. As seen in , only the D8 components of evaporation and runoff data have significant correlations at the α = 0.05 significance level. The correlation magnitude of the long-term periodicities (such as the 256-month scale) is more noticeable when compared with short-term periodicities. It can also be seen that the approximate series shows the correlation significance (equal to −0.25 for evaporation). This indicates that low frequencies of the hydro-meteorological time series are significantly correlated with the SOI. shows that the wavelet components of the hydro-meteorological data do not show significant correlation in association with the NAO index at the α = 0.05 significance level. In flow data, the magnitude of the correlation values of the 4-month component (D2) is more satisfactory when compared with one of the other components. It can be said that the influence of the NAO on the Keban hydro-meteorological data can be ignored.
Table 3. Correlation between the D components of the hydro-meteorological data and the original SOI or NAO index (anomaly) 1973–2002.
5 Conclusion
In this study, wavelet analysis was studied to determine the short- and long-term variability in lake evaporation. In addition, the periodic relationships between the evaporation pattern and the hydro-meteorological patterns in Lake Keban were investigated. The primary periodicity is annual for all the hydro-meteorological patterns. The CWS and GWS of the evaporation data show the inter-annual periodicities between the 16-month and 128-month scale levels and also between the 2-month and 8-month scales. Additionally, some weak periodicities are seen at the 64- and 128-month (10-year) scales. Furthermore, weaker periodicity is seen in the 256-month scale which is not statistically significant with the red-noise procedure. The periodicity at the 256-month scale is similar for all the hydro-meteorological data. While it is stronger for the wind speed and relative humidity, it is weaker for the runoff, precipitation and temperature data. The CWS and GWS of the wind speed and relative humidity show some weak periodicities in the region of the 64-month and also almost 10-year scale levels but they are not continuous over time. As a result, it is clear for the Keban hydro-meteorological data that the major inter-annual periodicity is short term (between the 2- and 16-month scales). On the other hand, some strong runoff events appear in the CWS of the runoff records, especially around 1988. Similarly, strong power in the 2–8-month scales in the wavelet spectrum is seen for evaporation and precipitation in 1988. These signs can be considered to be related to the strong 1988 La Niña event. The periodicities in the 2–8-month scales in the precipitation data can also be interpreted as connected with the 1982 and 1992 strong El Niño events.
The time series of the wavelet components were compared to assess the connection between evaporation and the hydro-meteorological data. The correlation analysis on the wavelet components shows that the D3 component (the 8-month scale) of the hydro-meteorological data shows a significant relationship with the D8 component of the evaporation series. Only the D8 components of the evaporation and runoff data have significant correlation with the SOI at the α = 0.05 significance level. Also, the 8-month periodic components of the hydro-meteorological data show a considerable correlation with the observed evaporation data. As a result, the evaporation data and the hydro-meteorological data of Lake Keban control some short-term (2–16 months) and long-term (20 years) periodicities. These results shows that intra-annual changes in evaporation are mainly associated with climatic variations, especially wind speed and relative humidity variations. Short-term variations in evaporation mainly correspond to precipitation and runoff.
The wavelet analysis of the hydro-meteorological data of Lake Keban clearly shows the periodic structure of the data and the variations in the data. Some connections were identified between the hydro-meteorological data and the SOI and NAO index. It is hoped that the improvements found will help to realize the uncertainties in the relationships between past evaporation and hydro-meteorological data. Future work must focus on the detection of evaporation response to climate change.
Acknowledgements
The author would like to thank Ondokuz Mayıs University for the support provided during this study.
Disclosure statement
No potential conflict of interest was reported by the author.
References
- Anctil, F. and Tape, D.G., 2004. An exploration of artificial neural network rainfall-runoff forecasting combined with wavelet decomposition. Journal of Environmental Engineering and Science, 3, 121–128. doi:10.1139/s03-071
- Assouline, S. and Mahrer, Y., 1996. Spatial and temporal variability in microclimate and evaporation over Lake Kinneret: experimental evaluation. Journal of Applied Meteorology, 35, 1076–1084. doi:10.1175/15200450(1996)035<1076:SATVIM>2.0.CO;2
- Bayazıt, M., 2001. Hydrology. Istanbul: Istanbul Technical University Civil Engineering Faculty.
- Coulibaly, P. and Burn, D.H., 2004. Wavelet analysis of variability in annual Canadian streamflows. Water Resources Research, 47, W03105. doi:10.1029/2003WR002667
- Daubechies, I., 1990. The wavelet transform, time-frequency localization and signal analysis. IEEE Transactions on Information Theory, 36 (5), 961–1005. doi:10.1109/18.57199
- Drago, A.F. and Boxall, S.R., 2002. Use of the wavelet transform on hydro-meteorological data. Physics and Chemistry of the Earth, 27, 1387–1399. doi:10.1016/S1474-7065(02)00076-1
- Gökbulak, F. and Özhan, S., 2006. Water loss through evaporation from water surfaces of lakes and reservoirs in Turkey. Official publication of European Water Association (EWA). Brussels: E-WATER, 1–6.
- Holmgren, K., et al., 2003. Persistent millennial-scale climatic variability over the past 25,000 years in Southern Africa. Quaternary Science Reviews, 22, 2311–2326. doi:10.1016/S0277-3791(03)00204-X
- Kahya, E. and Karabork, M.C., 2001. The analysis of El Niño and La Niña signals in streamflow of Turkey. International Journal of Climatology, 21, 1231–1250. doi:10.1002/joc.663
- Karabork, M.C. and Kahya, E., 2003. The teleconnections between extreme phases of southern oscillation and precipitation patterns over Turkey. International Journal of Climatology, 23, 1607–1625. doi:10.1002/joc.958
- Karabork, M.C., Kahya, E., and Karaca, M., 2005. The influences of the Southern and North Atlantic, oscillations on climatic surface variables in Turkey. Hydrological Processes, 19, 1185–1211. doi:10.1002/hyp.5560
- Küçük, M. and Ağıralioğlu, N., 2006. Wavelet regression techniques for streamflow predictions. Journal of Applied Statistic, 33 (9), 943–960. doi:10.1080/02664760600744298
- Kulkarni, J.R., 2000. Wavelet analysis of the association between the Southern Oscillation and the Indian summer monsoon. International Journal of Climatology, 20, 89–104. doi:10.1002/(SICI)1097-0088(200001)20:1<89::AID-JOC458>3.0.CO;2-W
- Labat, D., Ababou, R., and Mangin, A., 2000. Rainfall-runoff relations for karstic springs. Part II: continuous wavelet and discrete orthogonal multiresolution analyses. Journal of Hydrology, 238, 149–178. doi:10.1016/S0022-1694(00)00322-X
- Lafreniere, M. and Sharp, M., 2003. Wavelet analysis of inter-annual variability in the runoff regimes of glacial and nival-stream catchments, Bow Lake, Alberta. Hydrological Processes, 17, 1093–1118. doi:10.1002/hyp.1187
- Lenters, J.D., Kratz, T.K., and Bowser, C.J., 2005. Effects of climate variability on lake evaporation: results from a long term energy budget study of Sparkling Lake, northern Wisconsin (USA). Journal of Hydrology, 308, 168–195. doi:10.1016/j.jhydrol.2004.10.028
- Lu, R.Y., 2002. Decomposition of interdecadal and interannual components for North China rainfall in rainy season. Chinese Journal of Atmosphere (in Chinese), 26, 611–624.
- Mallat, S.G., 1989. A theory for multiresolution signal decomposition: the wavelet representation. IEEE Transactions on Pattern Analysis and Machine Intelligence, 11 (7), 674–693. doi:10.1109/34.192463
- Meyer, Y., 1993. Wavelets algorithms & applications. Philadelphia: Society for Industrial and Applied Mathematics.
- Mohamed, Y.A., Bastiaanssen, W.G.M., and Savenije, H.H.G., 2004. Spatial variability of evaporation and moisture storage in the swamps of the upper Nile studied by remote sensing techniques. Journal of Hydrology, 289, 145–164. doi:10.1016/j.jhydrol.2003.11.038
- Nakken, M., 1999. Wavelet analysis of rainfall–runoff variability isolating climatic from anthropogenic patterns. Environmental Modelling & Software, 14, 283–295. doi:10.1016/S1364-8152(98)00080-2
- Partal, T. and Cigizoglu, H.K., 2009. Prediction of daily precipitation data (Turkey) using wavelet-neural networks method. Hydrological Science Journal, 54 (2), 234–246. doi:10.1623/hysj.54.2.234
- Penalba, O.C. and Vargas, W.M., 2004. Interdecadal and interannual variations of annual and extreme precipitation over central-northeastern Argentina. International Journal of Climatology, 24, 1565–1580. doi:10.1002/joc.1069
- Pisoft, P., Kalvova, J., and Brazdil, R., 2004. Cycles and trends in the Czech temperatures series using wavelet transform. International Journal of Climatology, 24, 1661–1670. doi:10.1002/joc.1095
- Polikar, R., 1999. The story of wavelets, in Physics and modern topics in mechanical and electrical engineering, Mastorakis, N. World Scientific and Engineering Society Press, 192–197.
- Smith, L.C., Turcotte, D.L., and Isacks, B.L., 1998. Stream flow characterization and feature detection using a discrete wavelet transform. Hydrological Processes, 12, 233–249. doi:10.1002/(ISSN)1099-1085
- Taleb, E.H. and Druyan, L.M., 2003. Relationship between rainfall and West African wave disturbances in station observation. International Journal of Climatology, 23, 305–313. doi:10.1002/joc.883
- Torrence, C. and Compo, G.P., 1998. A practical guide to wavelet analysis. Bulletin of the American Meteorological Society, 79, 61–78. doi:10.1175/1520-0477(1998)079<0061:APGTWA>2.0.CO;2
- Winter, T.C., et al., 2003. Evaporation determined by the energy-budget method for Mirror Lake, New Hampshire. Limnology and Oceanography, 48 (3), 995–1009. doi:10.4319/lo.2003.48.3.0995
- Winter, T.C., Rosenberry, D.O., and Sturrock, A.M., 1995. Evaluation of 11 equations for determining evaporation for a small lake in the North Central United States. Water Resources Research, 31, 983–993. doi:10.1029/94WR02537
- Xingang, D., Ping, W., and Jifan, C., 2003. Multiscale characteristics of the rainy season rainfall and interdecadal decaying of summer monsoon in north China. Chinese Science Bulletin, 48, 2730–2734. doi:10.1007/BF02901765
- Yan, Z., Tsimplis, M.N., and Woolf, D., 2004. Analysis of the relationship between the North Atlantic Oscillation and sea-level changes in northwest Europe. International Journal of Climatology, 24, 743–758. doi:10.1002/joc.1035