ABSTRACT
Increased demand associated with population or economic growth, and decreased supply under some climatic shifts, obviously contribute to water scarcity. As a fresh perspective, we offer a generic theoretical treatment using a computational “maquette”, employing parameterizations to avoid assumptions about the origin and scale of climate and demand changes. The results suggest a distinct (and more subtle) point: the sensitivities of water stress to changes in both the mean and the variance of hydroclimate are modulated by demand level. Theoretical behaviours generated by the reduced-complexity model are surprisingly intricate, including profound nonlinearities and bifurcations. These may form a lower bound on the dynamical complexity of the demand–supply–scarcity nexus. Overall, the outcomes suggest that demand growth substantially intensifies and nonlinearizes water stress sensitivities to secular climate variation, and, in particular, that the interactions between demand changes and second-order hydroclimatic non-stationarity may produce non-intuitive water scarcity impacts requiring much closer study.
EDITOR A. Castellarin; ASSOCIATE EDITOR N. Ilich
1 Introduction
Understanding future water resource availability requires information about hydroclimatic supply and human demand. The stakes are high. Global population is about 7 billion and will exceed 9 billion by 2050, yet water scarcity is already a major issue for much of the world. Climate exhibits large natural swings and the current consensus among numerical climate modellers is that anthropogenic contributions to atmospheric greenhouse gases have become sufficiently large to alter planetary climate. Hence, factors such as population growth and, increasingly, climate change are routinely considered in practical water resource planning. Similarly, the number of academic studies regarding various climatic influences on surface water and groundwater hydrology is tremendous. However, as noted by Beck and Bernauer (Citation2011), few studies have rigorously examined the large-scale responses of standard water stress measures, such as relative water demand (RWD), to simultaneous shifts in both demand and climate; how the effects of climatic change compare to those of population growth; or the water scarcity implications of potential interactions between demand growth and climate variability or change. Examples of this much smaller literature include Vörösmarty et al. (Citation2000), Arnell (Citation2004), Beck and Bernauer (Citation2011), Kummu et al. (Citation2010), Wiltshire et al. (Citation2013), Böhmelt et al. (Citation2014) and Shen et al. (Citation2014). Interestingly, these studies often point to population growth and other demand-side factors as being a stronger control on overall scarcity than climatic changes.
One might additionally propose that as existing water systems operate closer to the margins under demand growth, a given climatically driven fluctuation or change in supply (if in the wrong direction) may become more likely to push availability over the edge from surplus to deficit. That is, demand growth might also influence water scarcity indirectly, by increasing how strongly it responds – that is, its sensitivity – to climatic or other supply-side changes. While some prior work seems to hint at such effects (Arnell Citation2004), to our knowledge previous studies have not directly considered how demand changes may modify climate–RWD relationships.
On the supply side, prior work has focused heavily on the water resource implications of changes only in mean climate. Relatively few studies (e.g. Pagano and Garen Citation2005, Luce and Holden Citation2009, Fleming and Sauchyn Citation2013) have examined the implications of hydroclimatic heteroscedasticity, that is, long-term shifts in the variance, perhaps in part because such changes have proven more challenging to predict numerically (e.g. Latif and Keenlyside Citation2009). This limitation may be crucial, as long-term changes in the mean supply may have subtle societal and environmental impacts, whereas changes in the year-to-year variance in supply – expressed, for example, as shifts in the magnitude or frequency of extreme events – could have large impacts for ecosystems and natural resource managers (e.g. Pagano and Garen Citation2005, Perkins et al. Citation2009). Even variations that may lie within the historical envelope, such as the precipitation fluctuations primarily responsible for the current California drought (Mao et al. Citation2015), can create tremendous concern and repeatedly make national and international headlines. Possible systematic shifts in the severity of such inter-annual hydroclimatic variability should therefore be of central interest.
In this short paper, we heuristically explore how demand growth may alter the sensitivities of water scarcity to both first- and second-order non-stationarity in climate. The demand–climate–water nexus spans the physical, biological, engineering and social sciences, and a correspondingly broad array of perspectives can be brought to bear. A common and entirely reasonable response is to adopt a bottom-up approach which attempts to explicitly represent all these discipline-by-discipline features, in as much detail as pragmatically achievable, to form a complicated and location-specific modelling chain. Without in any way questioning the usefulness of such a modelling philosophy, we take a different tack here. Inspired by the success of streamlined modelling paradigms for some complex problems in nonlinear dynamics, statistical mechanics, mathematical sociology, econophysics, geophysics, and mathematical ecology, for example, we apply a generic, top-down, systems-based approach which emulates complex dynamics associated with intricate climatic, hydrological, demographic and economic processes by parameterizing these processes and then applying manual perturbations to those parameter values. This generic and streamlined model configuration facilitates easy geophysical–sociophysical model coupling, direct computation of analytical derivatives that compactly describe fundamental system behaviours, rapid Monte Carlo simulation for efficiently exploring the implications of climatic uncertainty and variability, comparatively straightforward sensitivity analysis and improved ability to unambiguously identify and interpret nonlinear interactions between the impacts of climatic and demographic changes on water scarcity, and flexibility and general relevance in the sense that it has the capacity to represent a variety of potential climatic and demographic forcing mechanisms, including climatic heteroscedasticity, without a requirement to specify their nature, scale, longevity or origin in any detail. These advantages of the model as an instrument for speculative mathematical examination of the broad dynamics of the demand–climate–water nexus come at the expense of not providing detailed site-specific information; it is not a practical planning tool. That is, this blue-skies research is presented as a complement, not an alternative, to standard approaches, and it is intended primarily for the purpose of tracing out certain theoretical foundations, which to our knowledge have not been clearly identified before, around how demand growth may modify the impacts of secular climate shifts on net water scarcity. The overall aim of this numerical thought experiment is to formulate relevant new hypotheses that may inform future lines of investigation. The value of the approach taken seems apparent in the discovery of several new theoretical features, including the emergence of unanticipated nonlinear behaviours that belie the simplicity of the modelling paradigm employed.
2 Method
2.1 Maquette definition
For the purposes of our numerical thought experiment, consider a potentially available water supply, S, described by the following time series model, with β0, β1 > 0; ε is a zero-mean, unit-variance random process; and S(t) > 0 ∀ t = 1,N:
One can view S as (for example) dry-season streamflow for a local watershed, or regionally integrated annual runoff, or globally available combined surface water and groundwater supplies, or whatever else as circumstances or interest dictate. Mean hydroclimatic state is represented by β0, and year-to-year variability in hydroclimate is captured by β1ε(t).
How do we emulate climatic shifts using such a straightforward white-noise process? First, we can represent first-order non-stationarity in available water supplies by increasing or decreasing the value of β0 to specify a change to wetter or drier conditions, respectively. Second, given the foregoing ε definition, β1 serves as a multiplier that controls the severity of inter-annual supply variations, and a change in β1 would therefore capture second-order hydroclimatic non-stationarity. That is, we can increase β1 to specify a more volatile climate in our thought experiment, or decrease its value to stipulate a water supply that is more hydroclimatically stable from year to year. Note that β0 and β1 are treated as parameters, not variables or time series; climate changes are therefore specified to the model, rather than predicted by it. More will be said on this very shortly.
We must then couple this hydroclimatic representation to a metric of net water availability. Relative water demand (RWD) is a common measure that expresses effective water scarcity in terms of the proportion of available supply, as described above, taken up by demand, D:
RWD is (variously) very similar to, or synonymous with, a number of other indices, including the use-to-resource ratio, water scarcity index, mobilization level, relative water stress index, water resources vulnerability index, exploitation index, criticality ratio, and withdrawals-to-availability ratio. RWD is a proxy for average water-related stress on both ecosystems and socioeconomic systems. In this well-established framework (e.g. Falkenmark and Lindh Citation1976, Falkenmark Citation1997, Raskin et al. Citation1997, Shiklomanov Citation1997, Vörösmarty et al. Citation2000, Alcamo et al. Citation2003, Oki and Kanae Citation2006, Rijsberman Citation2006, Shen et al. Citation2014), values between 0.2 and 0.4 indicate medium to high stress, corresponding to conditions under which water stress can begin to be a limiting factor on economic development insofar as management costs start to be large in relation to the national economy; and RWD > 0.4 represents severe water limitation, indicating the onset of heavy competition between water users. Note that this critical value of RWD is less than unity, i.e. when many water uses and broader scarcity implications are taken into account, severe water stress can occur well before absolute demand exceeds absolute supply. A few other water scarcity indicators have been proposed (Rijsberman Citation2006). RWD offers the advantages of widespread adoption; comparatively easy calculation; incorporation of a range of considerations beyond basic human survival needs; a highly intuitive interpretation as the fraction of the available water supply that is being used up, with 0 indicating no water stress, and 1 or higher indicating complete exhaustion of the resource; and a fixed, apparently universally agreed-upon extreme scarcity threshold value of 0.4. As is the case for β0 and β1, D is treated here as a parameter, not a variable or time series, and demand changes are specified to the model, rather than predicted by it. Again, we will elaborate on this latter point very shortly.
This combination of a linear stochastic parameterization of hydroclimatic dynamics and a ratio-based indicator of water scarcity is compact, containing (as described above) only three parameters: the social parameter D and the geophysical parameters β0 and β1. Such reduced-complexity models, which we call mathematical “maquettes” after the scale models fashioned by sculptors as preparatory or demonstration exercises, can be useful for capturing signature features of a complex system and can themselves sometimes generate complex behaviours (e.g. Lorenz Citation1963, May Citation1976, Watson and Lovelock Citation1983, Bak et al. Citation1988, Harte Citation1988, Fleming Citation2009, Lewis Citation2013, Ziliani et al. Citation2013, Larsen et al. Citation2014). Amenability to both analytical treatments and efficient Monte Carlo probabilistic experiments also seems useful to our application, as does the generality bred by relative simplicity.
2.2 Climate and demand change emulation
In particular, by perturbing β0 or β1 about their baseline values, we may generically explore the implications of a wide range of climatic changes without the need to make any assumptions regarding the origin, scale and nature of such changes. As noted above, both first- and second-order hydroclimatic non-stationarity are accommodated. Further, such climatic shifts may be either natural or anthropogenic, local or global, or effectively permanent at human timescales or of finite duration. Consider a multi-decadal (say, 30-year) numerical simulation performed using this model. A manually prescribed reduction (for example) in the value assigned to β0 for the duration of the model run might be used to emulate a hydroclimatic shift associated with (variously) a local land-use change, a global anthropogenic climate change, one phase of some potentially climatically relevant astronomical cycle such as sunspot variability, a given phase of some natural decadal-scale internal cycle in the climate system such as the Pacific Decadal Oscillation, or an extended run of below-average conditions in a stochastic long-memory hydroclimatic process such as the Hurst effect.
Although this analysis is therefore not restricted to predicted anthropogenic global warming, it may be helpful by way of interpretive context to note that such climate changes may involve drying in some regions and wetting in others, or hydrological timing shifts that could generate acute seasonal water scarcity even if total local annual supply remains undiminished or even increases under a warming-induced increase in the intensity of the hydrological cycle (e.g. Wiltshire et al. Citation2013). Conversely, we also note that while our interpretive focus here lies with secular climatic variation, which is reasonable insofar as the freshwater amounts potentially available to societies are controlled by the hydrological cycle, the manual application of shifts to the values of β0 or β1 in the model may be viewed as emulations of other, non-climatic types of supply changes. De facto water supply reduction by contamination (e.g. Wolf Citation1998), which can render the physically available quantity of supply unusable, might conceivably be the most important of these overall. Indeed, the global crisis in access to clean water claims more lives through disease than any war claims through guns (Watkins Citation2006). Other possibilities include increased supply through water re-use, or infrastructure (e.g. reservoir, wellfield, desalinization plant) improvement or expansion; or changes in land surface hydrology associated with ecosystem or land use change.
Similar considerations apply to the demand component of the maquette: by manually perturbing D about its baseline value, we can flexibly represent a wide variety of processes. Water demand has historically been driven by human population, which may continue to be the case moving forward (e.g. Vörösmarty et al. Citation2000, Kummu et al. Citation2010, Böhmelt et al. Citation2014; see also for context), so demographic change can form a reasonable focus for interpreting the meaning of D increases manually imposed on the model. However, demographically driven demand growth may, in the maquette, flexibly represent either overall growth in the total global human population; or alternatively, more complicated, regional- or local-scale population dynamics such as demographic arms races (e.g. Wolf Citation1995) or migration to megacities (e.g. Vörösmarty et al. Citation2000).
Figure 1. Historical and projected global population and water use. Population data are from US Census Bureau (World Population, www.census.gov/population/international/data/worldpop/table_population.php, last accessed 3 September 2014), and water use data are from UNESCO (World Water Resources and Their Use, webworld.unesco.org/water/ihp/db/shiklomanov/, last accessed 3 September 2014).
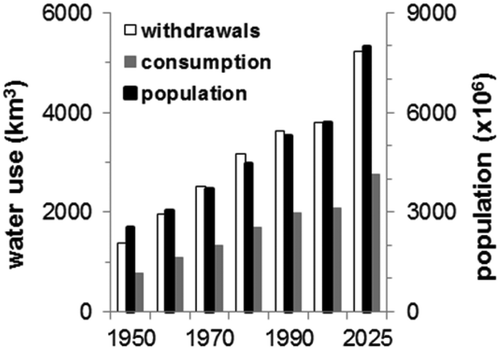
Furthermore, the manual D perturbations applied in our modelling additionally represent the bulk effect of many other factors influencing local, regional or global water demand and scarcity. This list can include (e.g. Rijsberman Citation2006, Kummu et al. Citation2010, Böhmelt et al. Citation2014) the following: increased demand associated with increased agricultural production, perhaps softened by certain water-conserving biotechnologies or sharpened by production of some water-intensive biofuels; increased demand associated with economic development, particularly in low- and middle-income countries; demand mitigation associated with water use efficiencies generated through social, technological or pricing means undertaken as water stresses mount, particularly in high-income countries; similarly, elasticity of demand in situations where a significant proportion of water use is non-essential; either exacerbation or mitigation of water scarcity and, potentially, water conflict or cooperation by social, political and governance capacities; and either amelioration or inducement of scarcity, depending on the direction of trade and comparative regional water availabilities, through virtual water transfers associated with inter-regional agricultural trade.
In terms of demand, the focus of our discussion below lies mainly with increases, which are generally more relevant going forward than demand decreases. In some regions, such as the United States, water use appears to have stabilized overall for the time being, but this is not the global tendency. That said, both increases and decreases in demand are considered in the maquette in the interest of theoretical thoroughness.
2.3 Assumptions and limitations
As noted above, our model design is relatively assumption-free insofar as it is not limited to one mechanism, or spatial or temporal scale, of supply or demand change. Readers may therefore view the outcomes of the maquette as a sort of generic dynamical template, to be interpreted in terms of whichever of the aforementioned processes or scales seem most relevant to the particular water scarcity problem in which they are most interested.
It bears noting, however, that some of the scarcity factors mentioned above are entangled. For instance, population growth and the anthropogenic climate changes anticipated by the climate modelling community are not fully independent. Rather, demographic projections are a key element of the greenhouse gas emission scenarios underlying GCM simulations of global climate, and, in fact, personal reproductive choices are the most influential decisions most of us can make about our individual carbon legacies (Murtaugh and Schlax Citation2009). Such topics are not directly broached here. Obviously, this places some limits the practical applicability of our outcomes; as noted at the outset, the study is theoretical in nature.
We also note that Equation (1) assumes serial dependence, which is approximately valid for many annually discretized hydroclimatic time series and suitable for the needs of this study. It could easily be modified for other purposes (daily flow data are strongly autocorrelated due mainly to physical catchment water storage, for example) by the inclusion of a linear autoregressive term, for instance. Also note, however, that in long-term climate and water resource studies, the most important role of system memory is the generation of stochastic regime shifts, involving extended runs of above- or below-average values. This behaviour is characterized by the power-law Fourier spectra diagnostic of dynamical fractals and a slowly decaying autocorrelation function typical of long-memory processes such as the Hurst effect (e.g. Cohn and Lins Citation2005, Fleming Citation2009). As noted in Section 2.2, perturbations of the geophysical model parameters can be used to emulate the appearance of such long-term regimes.
2.4 Implementation
Solely to facilitate numerical experimentation and provide some intuitive context, we implement the maquette using numbers for Chapman Creek (SCRD Citation2013). This watershed provides over 90% of the water supply to the Sunshine Coast, a semi-rural but rapidly growing and urbanizing area near the major urban centre of Vancouver, Canada. We focus on monthly average August conditions, when need is greatest and supply is least here. Historical data from Water Survey of Canada gauge 08GA060 give an inter-annual mean and standard deviation of monthly average August streamflow of 97.5 ML/d and 82.2 ML/d, which we take as baseline values of β0 and β1, respectively. August peak demand c. 2011 was 27.1 ML/d, and available planning estimates, given a 2% annual population growth rate, call for an increase to 33.3–44.4 ML/d depending on assumptions. Late-summer water resource availability is projected to decline in the region (Schnorbus et al. Citation2014). Higher-frequency inter-annual variability, associated in this area with such climatic phenomena as El Niño-Southern Oscillation (Fleming and Dahlke Citation2014), may also experience shifts, but this is less clear (e.g. Latif and Keenlyside Citation2009). Late-summer flows are at times already sufficiently low to cause concern for aquatic habitat quantity and water quality in this area (SCRD Citation2006); overall, future climate and population changes are anticipated to drive RWD upward in the region. We emphasize, however, that the Sunshine Coast’s Chapman Creek is used solely as a source of geophysically and sociophysically plausible numbers to run the model. This is not a case study. The model is not intended or presented as a reliable operational representation of hydroclimatic dynamics in this basin, and absolutely nothing in this article should be taken to suggest a site-specific water resource planning exercise.
3 Results and discussion
3.1 Some preliminary analytical considerations
One of the advantages of a relatively simple and compact mathematical model is that it enables the pencil-and-paper calculation of analytical derivatives, facilitating a deeper intuitive understanding of fundamental system characteristics. We thus begin with a heuristic analytical treatment which suggests that the effects of climatic changes upon water scarcity are explicit functions of demand level. Although a simple result, it seems noteworthy as it could have major implications and, to our knowledge, it has not been explicitly identified before. The sensitivity of water stress to climatic shifts may be expressed as the partial derivatives of RWD with respect to β0 and β1. From Equations (1) and (2), the unit rate of water scarcity increase in response to first-order non-stationarity in climate is easily found using the quotient rule:
We see that the sensitivity of RWD to a change in baseline climate state is an explicit function of demand level. As population grows, the rate at which RWD decreases with a unit increase in β0, or increases with a unit decrease in β0, also grows larger. Interestingly, (3) is also a function of the hydroclimatic volatility parameter β1. Alternatively, if we are interested in only the average water supply over several years (and thus mean RWD), the expectation value of S ~ β0 because ε(t) was defined above to be a zero-mean process, such that upward short-term supply fluctuations some years are balanced, over the long run, by downward fluctuations other years. In that case (3) simplifies to:
Either way, the D-dependence in the analytical derivatives is consistent with the intuitive notion suggested in our introduction: as existing water systems operate closer to the margins under demand growth, a given climatically driven change in supply (if in the wrong direction) pushes availability farther away from surplus to deficit, and vice versa.
Similarly, the rate of water scarcity increase in response to a change in β1, representing second-order non-stationarity in climate, is:
D again appears in the climatic sensitivity derivative. Alternatively, if we are interested only in mean S and therefore mean RWD over a number of years, by the foregoing logic this becomes:
Thus, the sensitivity of mean RWD to a change in the hydroclimatic variance is nil and thus not a function of demand. This seems reasonable: as noted above, increased hydroclimatic volatility gives more extreme high-flow years but also more extreme low-flow years, which in principle mutually compensate over the long run if ε(t) is a zero-mean process. That said, in any given year (or an integral time frame insufficiently long for Σβ1ε(t) → 0), this is not the case, as suggested by Equation (5). We thus see that the sensitivity of RWD to changes in the volatility of inter-annual climatic variations is also an explicit function of D: as demand grows, the magnitude of the rate at which RWD changes with β1 also increases. The direction of the sensitivity depends on whether that year’s climatic excursion, ε(t = t*), is positive (short-term supply increase) or negative (supply decrease). This is again consistent with the notion suggested in our introduction: as existing water systems operate closer to the margins under growing demand, a unit fluctuation in water supply becomes more likely to temporarily drive net availability across the line separating surplus from deficit, which in turn renders the system more sensitive to changes in volatility.
3.2 Monte Carlo simulations
A more thorough assessment may be obtained by conducting numerical Monte Carlo experiments using (1) and (2). The procedure was to fix β0, β1 and D; generate via Equation (1) a synthetic annual time series, S(t), of length N = 30 corresponding to a standard 30-year climatic normal period; produce NMC = 500 such realizations for a total of 1.5 × 104 samples to help ensure stable statistics are obtained; and evaluate the median and interquartile range of corresponding RWD values. This was repeated over a range of β0 (to investigate first-order climatic non-stationarity) or β1 (second-order non-stationarity), and then the entire process was repeated again for a few values of D (representing demand increases associated, for instance, with population growth). As noted above, the model is run (solely for convenience) using numbers from the Sunshine Coast’s Chapman Creek, such that S(t) can be taken to loosely represent a yearly time series of August mean discharge. Bearing in mind the central limit theorem and that August mean flow is derived from non-Gaussian daily flows by an additive process, it was sufficient for our purposes to assume a truncated standard normal distribution for the stochastic forcing: ε(t) ~ (0,1) with occasional S(t) < 0 replaced by a uniformly distributed random value lying between zero and the minimum historically observed August mean flow. Wide parameter ranges were considered, to thoroughly trace out the functional forms; in practice, progressive shifts in D, β0 and β1 for a given problem (say, Chapman Creek) seem likely to occupy only a small portion of the range displayed in .
Figure 2. Relative water demand, RWD, under changes in the (a) mean and (b) volatility of hydroclimatic water supply, for various demand levels. In column (a), β1 is held constant at the historical drought-season Chapman Creek value of 82.2 ML/d; in column (b), β0 is fixed at the historical drought-season value of 97.5 ML/d. Each row corresponds to a different demand level, denoted on the right-hand side of the row; the historical drought-season value is 27.1 ML/d. Note that the D, β0 and β1 changes prescribed here are arbitrary. The bold line gives median RWD, and lower and upper envelopes give 25th and 75th percentile values.
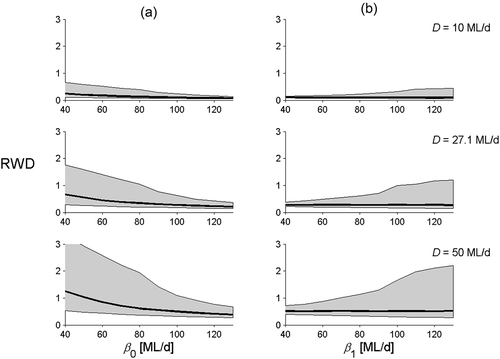
Obviously, water scarcity tends to increase with both decreasing supply and increasing demand, holding volatility constant ((a)). Note, however, that the relationship between β0 and RWD is nonlinear. If we start at, say, the historical Chapman Creek β0 value of 97.5 ML/d, scarcity decreases slowly with increasing supply, but increases rapidly with decreasing supply ((a)). For a given demand level, further incremental increases in supply eventually become largely irrelevant to RWD, as there is already more water than needed; the converse occurs for diminishing supply.
The important points here are that by jumping from one demand level to a higher one: (i) the slope of the β0–RWD relationship becomes generally steeper, indicating a heightened sensitivity of scarcity to climate change; and (ii) the β0–RWD relationship becomes significantly nonlinear over a wider β0 interval. This overall behaviour appears consistent with the analytical derivatives given in Equations (3) and (4). Such nonlinearity, and its population dependence, might be pragmatically important. For example, in a drying climate, large increases in scarcity could “sneak up” on water managers – what initially appear to be small changes accelerate into progressively larger water supply deficits, out of proportion to the rate at which climate is drying – and the severity of this surprise, so to speak, increases with the size of the demand. We also note that as climate dries the RWD distribution grows wider, indicating increased year-to-year uncertainty in water scarcity and increased risk of extreme scarcity events; higher demand greatly magnifies that effect.
(b) gives the relationship between β1 and RWD, holding β0 constant at its historical value, for various demand levels. The effects of such second-order climatic non-stationarity on water scarcity seem to be far less studied relative to those of first-order non-stationarity, so we briefly catalogue some of these phenomena before moving on to identify how demand growth might influence those relationships. The median value of RWD exhibits no apparent sensitivity to β1, as expected on the basis of Equation (6) and presumably for the same reasons. The results for non-typical years, however, are quite different. The 25th percentile and in particular 75th percentile values of RWD, denoted here as Q25(RWD) and Q75(RWD), show a clear relationship, which appears consistent with Equation (5). (b) also illustrates a two-fold nonlinearity in the sensitivity of water scarcity to the magnitude of year-to-year variations in hydroclimatic supply. First, the sensitivity of water scarcity to the typical magnitude of climatic excursions is asymmetric about the median for a given volatility, i.e. climatic sensitivities are not the same for positive and negative ε(t). Second, this asymmetry grows with β1. More specifically, as β1 increases, the slope of the β1–Q75(RWD) relationship increases. Put another way, Q75(RWD) increases at a rate that is out of proportion to that at which low-flow years grow more extreme. Thus, the β1–RWD relationship is direct, strong and powerfully nonlinear for the dry years corresponding to Q75(RWD); but inverse, weak and nearly linear for the wet years corresponding to Q25(RWD).
This context having been established, we can now examine how increasing demand affects relationships between the degree of climatic volatility and the severity of water scarcity. (b) suggests that greater demand: (i) has little or no impact on the dependence of mean or median scarcity on hydroclimatic volatility, as evidently no such relationship exists; (ii) increases the water scarcity sensitivity of non-average years to changes in hydroclimatic variability, subtly for wet years but dramatically for dry years, as the slopes of the volatility–scarcity curves grow in magnitude as D grows; and (iii) increases the degree of nonlinearity in those relationships, especially for Q75(RWD).
Overall, the numerical simulations suggest that climate-scarcity relationships are nonlinear, that growing demand increases not only scarcity but also the sensitivity of scarcity to climate, and that growing demand increases the nonlinearity of scarcity–climate relationships. Broadly speaking, these results hold true for both first- and second-order non-stationarity in climate, although the impacts for the latter are more complex. The impacts of increasing demand on the climatic dependencies of water stress appear clearest for high-scarcity years, which seems intuitively reasonable. An important corollary to the latter point is that the statistical distribution of RWD seems to become gradually more skewed (long-tailed) in a progressively drying or more volatile hydroclimate, and this effect is accelerated by population and other demand growth.
3.3 Numerical simulation of threshold effects
We then extended the Monte Carlo simulations to contemplate threshold effects. As discussed above, RWD = 0.4 is widely taken to indicate the onset of extreme water stress. The precise value of this cut-off may be somewhat arbitrary; various factors, briefly summarized earlier in this paper, can edge the balance between supply and demand toward one side or the other of this threshold; and there is nothing to suggest that RWD > 0.4 will trigger some sort of Malthusian catastrophe. That said, the existence of a fundamental tipping point is widely accepted in the literature and does not seem in question: at some basic level, either there is sufficient water to meet human and ecological demands, or there is not. In any event, the numerical exercise of exploring threshold effects can, at a minimum, be expected to provide some interesting insights into system dynamics. We therefore consider the probability, P, of exceeding this threshold, evaluated for a particular synthetic time series as the proportion of samples for which RWD > 0.4.
While it is entirely unsurprising that an increasing demand or a drier climate yields a greater likelihood of extreme water scarcity, all else being equal, we also see two other, more interesting features in (a): the probability of exceeding the critical water scarcity value is a nonlinear function of mean water supply, and the degree and nature of this nonlinearity are controlled by demand level. For smaller demands (e.g. D = 2 ML/d), nonlinearity is limited to an increase in the magnitude of the slope of the β0–P curve as climate dries past a certain point. With an increased demand (e.g. D = 27.1 ML/d), this slope change becomes far more severe, with P increasing at a much faster rate as β0 decreases. For the largest demands (e.g. D = 65 ML/d), the basic form of the relationship switches to an approximately sigmoidal form. Under such high water demand, the curve asymptotes to P ~ 1 for very low β0, presumably because additional drying past a certain point becomes irrelevant, as extreme scarcity is already pervasive. Behaviours under wet climates are generally similar: for all D, the β0–P relationship saturates at or near P ~ 0 for very high β0, presumably because additional wetting of climate past a certain point becomes irrelevant to the probability of extreme water stress, there already being sufficient water in all years. Overall, a larger demand level makes the relationship between mean water supply availability and the probability of extreme scarcity more nonlinear, and hence generally trickier to manage for. These outcomes are intuitively consistent with those illustrated in (a).
Figure 3. Probability of extreme water stress under changes in (a) the climatic mean and (b) volatility, for various demand levels. In (a), β1 is held constant at the historical drought-season Chapman Creek value of 82.2 ML/d; in (b), β0 is fixed at the historical value of 97.5 ML/d. For reference, the historical value of D is 27.1 ML/d.
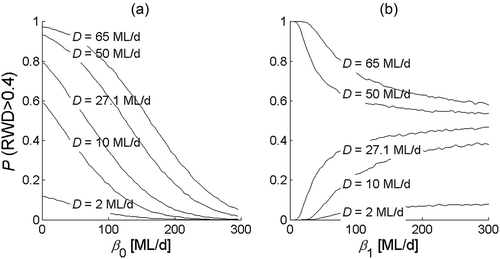
The probability of experiencing severe water stress is also a nonlinear function of the climatic volatility parameter and exhibits an extremely nonlinear dependence on demand ((b)). As β1→ 0, P becomes approximately binary, approaching either nil or unity. Roughly speaking, at very low degrees of variability, the stochastic component of Equation (1) is negligible. In this case, it appears that S is largely dictated instead by the fixed value of β0, yielding a nearly binary P outcome of about 0 or 1 depending on the demand level, i.e. on whether D/β0 is above or below 0.4. Upon injecting more significant year-to-year hydroclimatic variability into the system, however, it can generate occasional low-scarcity years even if mean scarcity is high, or occasional high-scarcity years if mean scarcity is low. The curves therefore partially converge toward, and stabilize at, more intermediate probabilities at higher β1. Approaching it from the opposite direction, then, lessening climatic volatility results in a bifurcation in the relationship between β1 and P; which direction is taken from that point is controlled by the combination of demand and mean climate. The highest local rates of change of P with β1 occur for low β1, and these seem to be greatest for intermediate demand levels (e.g. 27.1 or 50 ML/d in (b)).
Specifically for large demand (e.g. D = 50 ML/d), there may initially seem to be an inconsistency between the forms of the volatility–scarcity relationships illustrated in (b) versus (b). The former implies increasing scarcity with increasing volatility, at least for dry years, but the latter implies the opposite. This apparent discrepancy presumably reflects the fact that (for large populations) P is controlled (diminished) by increased wet years under a more volatile climate, all else held equal, as discussed immediately above. In contrast, Q75(RWD), a measure of the magnitude of high-scarcity events, is controlled (amplified) by increased dry years under that same, more volatile, climate. The outcome is fundamentally different under low demand levels, such as D = 10 ML/d; we then find sgn[∂P(RWD > 0.4)/∂β1] = sgn[∂Q75(RWD)/∂β1]. Presumably, when per-capita supply is (generally) ample, wetter wet years have little effect on scarcity but drier dry years do, such that both the severity and probability of extreme water scarcity increase with higher climatic volatility.
A curious implication is that – for high-demand conditions – there may exist some intermediate volatility such that the magnitude of high-RWD events is, in some abstract sense, optimally balanced against the probability of occurrence of extreme scarcity. A series of particle swarm optimizations was used to map this optimal β1 landscape, by finding β1 such that Q75(RWD) and P(RWD > 0.4) are simultaneously minimized for each combination of a range of β0 and D values. The outcomes () appear to confirm that, as loosely suggested by visual inspection of and (b), optimal β1 is obviously ~0 under low-D conditions, and ~45–85 ML/d under high-D conditions. The particular demand level at which this optimal-β1 transition occurs tends to increase with β0, loosely maintaining RWD of ~0.4–0.5, which approximately correlates with the onset of severe scarcity. The physical interpretation may be counterintuitive. Water supplies in high-variability river basins (for example) are often considered unreliable, unpredictable and difficult to manage – yet our results suggest that under high-demand conditions, significant hydroclimatic volatility (but not too much) could in principle prove advantageous by providing occasional relief through happenstance high-supply years without increasing the severity of high-scarcity years unduly. Thus, moderately high volatility might conceivably provide a sort of buffering capacity in strongly pressured water resource systems.
Figure 4. Optimal β1 landscape. Dimensions are ML/d. For a given combination of β0 and D, a particle swarm optimization (PSO) was used together with Monte Carlo simulations of Equations (1) and (2) to estimate β1 | min[P(RWD > 0.4) + 1/3Q75(RWD)]. The factor of 1/3 rescales Q75 such that P and Q75 have comparable influence in the simple multi-objective parameter estimations. Many restarts were performed for each PSO to help ensure global minima. Steps, including cost function penalties, were taken to guide optimizations toward β1 > 0 under all conditions. Roughly speaking, the response surface appears to organize into low- and high-volatility regimes approximately corresponding to low- and high-demand conditions, respectively.
![Figure 4. Optimal β1 landscape. Dimensions are ML/d. For a given combination of β0 and D, a particle swarm optimization (PSO) was used together with Monte Carlo simulations of Equations (1) and (2) to estimate β1 | min[P(RWD > 0.4) + 1/3Q75(RWD)]. The factor of 1/3 rescales Q75 such that P and Q75 have comparable influence in the simple multi-objective parameter estimations. Many restarts were performed for each PSO to help ensure global minima. Steps, including cost function penalties, were taken to guide optimizations toward β1 > 0 under all conditions. Roughly speaking, the response surface appears to organize into low- and high-volatility regimes approximately corresponding to low- and high-demand conditions, respectively.](/cms/asset/8afc0649-85a2-4596-903b-51517ea45340/thsj_a_1164316_f0004_oc.jpg)
The most pertinent feature of (b) and perhaps the most intriguing inference in this study, however, is that a given incremental change in volatility can generate either an increase or a decrease in the probability of water scarcity – depending on the demand level. This outcome might have significant practical ramifications. For the sake of argument, consider an increase in Chapman Creek β1 from the historical value of 82.2 ML/d, holding β0 constant. If D < 35–45 ML/d or so, then this climatic change will produce an increased probability of water scarcity. However, if D is greater than this value, then the opposite outcome, a decreased probability of water scarcity, would in principle arise. Further, if D increases over time alongside β1, then the probability of water scarcity could increase even more rapidly at first, but it may then begin to decline again if the apparent tipping point at D ~ 35–45 ML/d is eventually crossed. Demand-side factors, such as population size and water use efficiency, therefore appear in some sense a master variable in a heteroscedastic climate, controlling the fundamental direction of changes in water scarcity likelihood in response to changes in hydroclimatic volatility.
4 Conclusions
The demand–climate–water nexus is a complex coupled geophysical–sociophysical system, and its profound implications to both ecosystems and human societies locally, nationally and globally demand diligence in exploring multiple means and methods of understanding. The physics community has a tradition of contemplating fundamental questions around long-term resource use and environmental impacts under increasing population and economic pressures, often adopting a comparatively big-picture approach (e.g. Bartlett Citation2004, Weichman Citation2010). As such, it is intriguing to speculate whether system-level interactions between climate, population and water stress could prove amenable to treatment by a paradigm somewhat more typical of physics research. By this we mean the development, in response to some puzzle of nature, of a strongly idealized and abstract mathematical representation of basic system elements, followed by generation of specific hypotheses on the basis of quantitative predictions from that theoretical model, and finally the design and completion of corresponding experiments to test those specific hypotheses and thus the underlying model. This often occurs in a series of distinct and specialized investigative steps undertaken by many research groups in a process that may span decades. Obvious examples include the discoveries of time dilation in relativistic physics, quantum teleportation in quantum mechanics, and the Higgs boson in particle physics. This overall research philosophy contrasts with typical procedure in water resources research, especially that which takes place at or beyond the scale of individual river basins, which seldom makes such clear distinctions between theory, hypothesis and experiment. Rather, the case study-based and results-focused paradigm of water resource research instead often takes the form (though there are of course notable exceptions) of either post-hoc analysis and modelling of whatever long-term observational data happen to be available from uncontrolled natural experiments, or strongly practical and project-specific engineering innovation. There are excellent, entirely legitimate reasons why water resources science has moved in these directions: the complex open systems it studies often seem unamenable to compact theoretical treatment or experimentation in isolated laboratory settings, and historically the field has been driven largely by specific, if often ambitious, assigned tasks – developing an operational flood forecast model of a particular river for particular client with particular needs, for example. It is also a highly efficient approach for rapidly developing useful answers and implementing effective solutions to socially relevant questions. In the long run, however, such data-driven or highly pragmatic R&D philosophies may incur deep costs. A verified prediction is far more intellectually compelling than the explanation of a previously observed phenomenon (e.g. Mackenzie Citation2015), and pure, blue-skies research tends to be uncommon in water resource science, limiting the potential for long-term fundamental advances. Here – without in any way questioning the general utility of standard practices in water resource science – we instead explore the applicability of a more physics-oriented framework by introducing a conceptually streamlined hypothesis-generating theoretical model of the overall population–climate–water nexus. We do not test this set of hypotheses, and indeed it is not obvious whether the observational data necessary to do so convincingly and globally exist yet. As such, these hypotheses should be collectively viewed as a potential guiding instrument for future work, including experimental data collection.
As a complement to complicated multi-disciplinary modelling chains, and inspired by the prior use of reduced-complexity models in various fields of study to isolate fundamental system dynamics, an exploratory maquette is proposed. The focus lies with emphasizing general behaviours over detailed predictions; clarifying how demand growth affects the way that water scarcity responds to shifts in both the mean and, perhaps more notably, the variance of climate; and the generation of specific research hypotheses to help guide future investigations. The wider implications of the theoretical numerical sketch offered here lie mainly with the research directions it suggests.
Some of these theoretical outcomes are straightforward, whereas others are surprisingly intricate, and several do not appear to have been identified before. Specific hypothesized behaviours include population-driven increases in the nonlinearity of climate vs. scarcity relationships; and a switch, as population grows past a critical level, from increasing to decreasing probability of extreme scarcity with increasing climatic volatility, or by the same token, a demand-controlled bifurcation in volatility–scarcity relationships with decreasing climatic volatility. Broadly speaking, the maquette suggests that, in principle, demand growth intensifies and nonlinearizes water stress sensitivities to climatic variation. A particularly important outcome is that the most complex features of the relationships between demand levels, secular climate changes, and water scarcity arise in the context of second-order hydroclimatic non-stationarity, suggesting that changes in the climatic variance require much closer scrutiny than they have enjoyed to date. Given the relative simplicity of the maquette, this set of behaviours may collectively form a lower bound on the dynamical complexity of the demand–climate–water nexus: an important point in itself, as it provides very strong and clear quantitative reinforcement to the common qualitative notion that interactions between climate, water and society are challenging to study and manage.
We close with some remarks about the generality of the maquette and how this relates to the formation of policy research directions. The study works toward tracing out a full range of theoretical possibilities for the dynamical behaviour of the demand–climate–water nexus. Doing so is facilitated by the use of a streamlined, generalized, abstract model, the applicability of which is not restricted to only a specified scenario or particular set of assumptions. In the physical sciences, models that are highly general – in the sense that they are capable of capturing a wide diversity of potential phenomena and behaviours, and of doing so using only modifications of parameter values or model forcing or boundary conditions, without basic model redefinition or restructuring – are usually viewed as strongly desirable. Perhaps the epitome of this concept is the physicist’s search for an overarching framework that subsumes the seemingly distinct and in some sense mutually incompatible theories of relativity and quantum mechanics; a grand theory that elegantly explains many things is viewed as being vastly preferable to set of smaller theories each explaining a different and smaller thing. A more everyday example might be numerical weather prediction (NWP) models: while a few modelling packages exist, these represent relatively minor variations on a theme, there being a basically agreed-upon and fully general set of governing partial differential equations. This fundamental mathematical description encapsulates the fluid dynamical principles underlying atmospheric circulation and is valid irrespective of whether the forecast is for the Amazon or Siberia, and of whether one is expecting sun or rain. In contrast, the social sciences often require specific details for policy analysis purposes in some fixed context, representing a particular set of circumstances or assumptions, and in this case generality can imply ambiguity and may seem a hindrance. That is, the specification of the natural or anthropogenic factors behind a particular set of assumptions or scenarios can be crucially important for policy, as some of these factors can be managed by adaptive and anticipatory policies and programmes (e.g. water allocation, demand management programmes, or water-saving technologies). Although it is not our emphasis in this paper, it bears noting that the maquette introduced here might additionally be a useful mechanism for scoping-level scenario-based examination of complex relationships between water demand, supply and scarcity. That is, while the model is built to enable meaningful probabilistic simulations over a wide parameter space, any given parameter set represents (in isolation) a compact and specific set of assumptions representing a particular demand–climate scenario which may be policy-relevant in some particular case.
Consider, for instance, the example of late-summer flow volumes in the Sunshine Coast’s Chapman Creek, which we used simply as a convenient source of realistic parameter values in our numerical simulations. We noted previously that this is not a case study and in no way do the simulations presented here constitute any kind of practical water planning exercise for this area. We reiterate that caveat here, but in the continued spirit of theoretical exploration that motivates this paper, let us nevertheless conduct a preliminary thought experiment about how our numerical outcomes might inform policy research directions for this region. As discussed earlier, planning-focused projections generated in prior studies of this area call for lower late-summer supply due to changes in hydroclimatic seasonality, and increased demand due to local population growth, even after accounting for improved water conservation and efficiency measures. Phrased in terms of our maquette, this particular scenario corresponds to a particular parameter set, involving an increase in D and a decrease in β0 relative to their historical values. In principle, we can see from the simulations performed here that, under this scenario, not only might we anticipate an overall increase in water stress in this region, but also, as population increases, a progressive nonlinearization of the relationship between hydroclimatic changes and effective water stress, with gradually greater summertime water stress responses per unit climate change, in addition to a wider statistical distribution of RWD outcomes under hydroclimatic uncertainty (see again preceding discussion and (a)). These outcomes suggest substantially heightened water management challenges relative to historical experience and, given the local context, imply a need for corresponding policy investigations around local population-driven water demand growth. Several elements of Canadian governance, such as the federal child tax benefit and British Columbia provincial family bonus, financially reward and thus incentivize, or at least facilitate, reproduction in excess of the replacement rate. Furthermore, while Canada’s total land area is very large and its total population is comparatively small, various socioeconomic forces have caused this population to become increasingly heavily concentrated in a handful of large metropolitan centres, and intense local-scale population growth on the Sunshine Coast reflects in part the area’s gradual transition into a bedroom community to the larger Vancouver area. Thus, one of the key hypotheses generated by the maquette – the increasing nonlinearity and uncertainty, under local population growth, in water scarcity responses to seasonal hydroclimate drying – may in turn suggest some particular questions for policy researchers in this region to explore, perhaps supported by more detailed, complete and area-specific models of hydroclimatic supply, social and ecological demand, and water supply infrastructure. Analogous considerations may apply elsewhere.
Acknowledgements
The author thanks J. Jones and R.D. Moore for valuable discussions. The manuscript benefited greatly from comments by R. Saleth, N. Ilich and an anonymous reviewer.
Disclosure statement
No potential conflict of interest was reported by the author.
References
- Alcamo, J., et al., 2003. Global estimates of water withdrawals and availability under current and future “business-as-usual” conditions. Hydrological Sciences Journal, 48, 339–348. doi:10.1623/hysj.48.3.339.45278
- Arnell, N.W., 2004. Climate change and global water resources: SRES emissions and socio-economic scenarios. Global Environmental Change, 14, 31–52. doi:10.1016/j.gloenvcha.2003.10.006
- Bak, P., Tang, C., and Wiesenfeld, K., 1988. Self-organized criticality. Physical Review A, 38, 364–374. doi:10.1103/PhysRevA.38.364
- Bartlett, A., 2004. Thoughts on long-term energy supplies: scientists and the silent lie. Physics Today, 57, 53–55. doi:10.1063/1.1784303
- Beck, L. and Bernauer, T., 2011. How will combined changes in water demand and climate affect water availability in the Zambezi river basin? Global Environmental Change, 21, 1061–1072. doi:10.1016/j.gloenvcha.2011.04.001
- Böhmelt, T., et al., 2014. Demand, supply, and restraint: determinants of domestic water conflict and cooperation. Global Environmental Change, 29, 337–348. doi:10.1016/j.gloenvcha.2013.11.018
- Cohn, T.A. and Lins, H.F., 2005. Nature’s style: naturally trendy. Geophysical Research Letters, 32, L23402. doi:10.1029/2005GL024476
- Falkenmark, M., 1997. Meeting water requirements of an expanding world population. Philosophical Transactions of the Royal Society B: Biological Sciences, 352, 929–936. doi:10.1098/rstb.1997.0072
- Falkenmark, M. and Lindh, G., 1976. Water for a starving world. Boulder: Westview Press.
- Fleming, S.W., 2009. Exploring the nature of Pacific climate variability using a “toy” nonlinear stochastic model. Canadian Journal of Physics, 87, 1127–1131. doi:10.1139/P09-095
- Fleming, S.W. and Dahlke, H.E., 2014. Parabolic northern-hemisphere river flow teleconnections to El Niño-Southern Oscillation and the Arctic Oscillation. Environmental Research Letters, 9, 104007. doi:10.1088/1748-9326/9/10/104007
- Fleming, S.W. and Sauchyn, D.J., 2013. Availability, volatility, stability, and teleconnectivity changes in prairie water supply from Canadian Rocky Mountain sources over the last millennium. Water Resources Research, 49, 64–74. doi:10.1029/2012WR012831
- Harte, J., 1988. Consider a spherical cow: a course in environmental problem solving. Sausalito: University Science Books.
- Kummu, M., et al., 2010. Is physical water scarcity a new phenomenon? Global assessment of water shortage over the last two millennia. Environmental Research Letters, 5, 034006. doi:10.1088/1748-9326/5/3/034006
- Larsen, L., et al., 2014. Exploratory modeling: extracting causality from complexity. Eos, 95, 285–286. doi:10.1002/2014EO320001
- Latif, M. and Keenlyside, N.S., 2009. El Nino/Southern Oscillation response to global warming. Proceedings of the National Academy of Sciences, 106, 20578–20583. doi:10.1073/pnas.0710860105
- Lewis, K.C., 2013. Forgotten merits of the analytical viewpoint. Eos, 94, 71–72. doi:10.1002/2013EO070004
- Lorenz, E.N., 1963. Deterministic nonperiodic flow. Journal of the Atmospheric Sciences, 20, 130–141. doi:10.1175/1520-0469(1963)020<0130:DNF>2.0.CO;2
- Luce, C.H. and Holden, Z.A., 2009. Declining annual streamflow distributions in the Pacific Northwest United States, 1948–2006. Geophysical Research Letters, 36. doi:10.1029/2009GL039407
- Mackenzie, R., 2015, General relativity turns 100. Physics in Canada, 71, 125–126.
- Mao, Y., Nijssen, B., and Lettenmaier, D.P., 2015. Is climate change implicated in the 2013–2014 California drought? A hydrologic perspective. Geophysical Research Letters, 42, 2805–2813. doi:10.1002/2015GL063456
- May, R.M., 1976. Simple mathematical models with very complicated dynamics. Nature, 261, 459–467. doi:10.1038/261459a0
- Murtaugh, P.A. and Schlax, M.G., 2009. Reproduction and the carbon legacies of individuals. Global Environmental Change, 19, 14–20. doi:10.1016/j.gloenvcha.2008.10.007
- Oki, T. and Kanae, S., 2006. Global hydrological cycles and world water resources. Science, 313, 1068–1072. doi:10.1126/science.1128845
- Pagano, T. and Garen, D., 2005. A recent increase in western U.S. streamflow variability and persistence. Journal of Hydrometeorology, 6, 173–179. doi:10.1175/JHM410.1
- Perkins, T.R., Pagano, T.C., and Garen, D.C., 2009. Innovative operational seasonal water supply forecasting technologies. Journal of Soil and Water Conservation, 64, 15A–17. doi:10.2489/jswc.64.1.15A
- Raskin, P., et al., 1997. Water futures: assessment of long-range patterns and problems. Stockholm: Stockholm Environment Institute.
- Rijsberman, F.R., 2006. Water scarcity: fact or fiction? Agricultural Water Management, 80, 5–22. doi:10.1016/j.agwat.2005.07.001
- Schnorbus, M., Werner, A., and Bennett, K., 2014. Impacts of climate change in three hydrologic regimes in British Columbia, Canada. Hydrological Processes, 28, 1170–1189. doi:10.1002/hyp.v28.3
- SCRD, 2006. Chapman Creek Source Assessment. Prepared by Triton Environmental Consultants for Sunshine Coast Regional District (SCRD), Sechelt.
- SCRD, 2013. Comprehensive Regional Water Plan. Prepared by Opus DaytonKnight Consultants for Sunshine Coast Regional District (SCRD), Sechelt.
- Shen, Y., et al., 2014. Projection of future world water resources under SRES scenarios: an integrated assessment. Hydrological Sciences Journal, 59, 1775–1793. doi:10.1080/02626667.2013.862338
- Shiklomanov, I.A., 1997. Comprehensive assessment of the freshwater resources of the world. Geneva: World Meteorological Organization.
- Vörösmarty, C.J., et al., 2000. Global water resources: vulnerability from climate change and population growth. Science, 289, 284–288. doi:10.1126/science.289.5477.284
- Watkins, K., 2006. Human development report 2006, beyond scarcity: Power, poverty, and the global water crisis. New York: United Nations Development Programme.
- Watson, A.J. and Lovelock, J.E., 1983. Biological homeostasis of the global environment: the parable of Daisyworld. Tellus B, 35B, 284–289. doi:10.1111/teb.1983.35B.issue-4
- Weichman, F., 2010, Ecological footprint. Physics in Canada, 66, 167–170.
- Wiltshire, A., et al., 2013. The importance of population, climate change and CO2 plant physiological forcing in determining future global water stress. Global Environmental Change, 23, 1083–1097. doi:10.1016/j.gloenvcha.2013.06.005
- Wolf, A.T., 1995. Hydropolitics along the Jordan River: Scarce Water and its impact on the Arab-Israeli Conflict. Tokyo: United Nations University Press.
- Wolf, A.T., 1998. Conflict and cooperation along international waterways. Water Policy, 1, 251–265. doi:10.1016/S1366-7017(98)00019-1
- Ziliani, L., et al., 2013. Reduced-complexity modeling of braided rivers: assessing model performance by sensitivity analysis, calibration, and validation. Journal of Geophysical Research, 118, 2243–2262. doi:10.1002/jgrf.20154