ABSTRACT
Large seasonal variability in precipitation patterns may help overcome data limitations and difficult conditions when characterizing hydrological flow pathways. We used a limited amount of weekly water chemistry as well as stable water isotope data to perform end-member mixing analysis (EMMA) in a generalized likelihood uncertainty estimation (GLUE) framework in a sub-catchment of the Kilombero Valley, Tanzania. While there were considerable uncertainties related to the characterization and mixing of end-members, some robust estimates could be made on contributions to seasonal streamflow variability. For example, there is a low connectivity between the deep groundwater and the stream system throughout the year. Also, a considerable wetting-up period is required before overland flow occurs. Thus, in spite of large uncertainties, our results highlight how improved system understanding of hydrological flows can be obtained even when working in difficult environments.
Editor M. C. Acreman; Associate editor L. Ruiz
1 Introduction
A global lack of data limits the capacity to develop robust water management plans that leverage current hydrological understanding. Unfortunately, this lack of data is typically largest in developing regions, where management and planning could benefit the most from a clear scientific basis. The question thus becomes how to utilize state-of-the-science approaches under the limitations in data and difficult working environments that typify developing regions.
A clear and critical case of this interplay between a need for improved hydrological understanding and a need for sustainable water management in the face of data limitations can be found in rural Tanzania. In Tanzania, like many developing countries, there is an ongoing governmental interest in expanding and intensifying agricultural production to increase food security, reduce poverty and increase incomes (SAGCOT Centre Ltd. Citation2011). There are, however, environmental risks related to an expanded and intensified agriculture, since increased water abstraction may lead to lowered water tables (Leng et al. Citation2014) and reduced water flows downstream of irrigated areas. These impacts can have adverse effects on local and regional ecosystems (ERB Citation2006). As such, concurrent achievement of goals for rapid agricultural growth and improved environmental sustainability requires rigorous water management planning underpinned by science.
In a story that is repeating itself globally, the regions being targeted for agricultural growth in Tanzania have limited and poor quality hydro-meteorological data, hampering the capacity for understanding the dominant hydrological processes and, in turn, limiting the development of adequate water management plans (Koutsouris et al. Citation2016). One option in this regard might be to use experimental design and short-term monitoring to take advantage of naturally occurring, large signal-to-noise ratios, whereby it becomes possible to probe variations in hydrological state utilizing methods that are more advanced.
From this perspective, end-member mixing analysis (EMMA) is a hydrological technique that may be particularly relevant in data-limited situations. EMMA, which can be used to characterize flow pathways and storage mixing in a catchment, relies on three key assumptions: (a) tracers are conservative in the studied environment and mix linearly; (b) EM chemical signatures are homogenous in time and space; and (c) EM chemical signatures can be distinguished from each other (Hooper Citation2003). However, these assumptions are often violated in natural hydrological systems (Hooper et al. Citation1990, Soulsby et al. Citation2003), rendering large uncertainties in the resulting EM contribution estimates (Bazemore et al. Citation1994, Durand and Juan Torres Citation1996, Genereux Citation1998, Uhlenbrook and Hoeg Citation2003, Delsman et al. Citation2013). There is thus clearly a need to incorporate a rigorous uncertainty analysis in any EMMA to understand the limitations of the results. In addition, as hydrological systems pass through various potentially steady states as a consequence of seasonality (e.g. Lyon et al. Citation2008), the large differences in behaviour exhibited between seasons allows us to relax some of EMMA’s key assumptions.
In this study, we explore the possibility of applying EMMA in truly data-limited environments targeted for development. We consider the Kiburubutu Catchment, a sub-catchment of Tanzania’s Kilombero Valley (Lyon et al. Citation2015), and attempt to leverage temporal and spatial homogeneity as well as a strong hydrological seasonality to overcome sampling limitations. Stable water isotopes as well as a number of geochemical tracers were used to analyse source water contributions to streamflow both throughout the year and seasonally. Uncertainties of measurements as well as of EM characterization were explicitly taken into account by applying the methodology developed by Delsman et al. (Citation2013). Specifically, EMMA was used within a generalized likelihood uncertainty estimation (GLUE) framework (Beven and Binley Citation1992), which from here forward is referred to as G-EMMA. Furthermore, we discuss the capability to extrapolate the improved system understanding from the Kiburubutu Catchment across the Kilombero Valley (KV) based on stream-water chemistry observations. The goal of this study is thus twofold. First, we aim to improve understanding of the hydrology of the Kiburubutu Catchment and KV system. Second, we aim to demonstrate that the apparent mismatch between state-of-the-science techniques and data limitations can potentially be bridged by leveraging experimental design and natural system variability. This is promising as we seek to advance science in more and more remote and in particular developing regions to allow for important improvements for management of less and less available resources.
2 Site description
The Kilombero Valley (KV) basin, which contains the Kiburubutu Catchment, is located in central Tanzania (). The valley is part of the East African Rift Valley and is defined by the Udzungwa Mountains and the Mahenge Escarpment in the northwest and the Mbarika Mountains in the southeast. The Kilombero River, the main river of the KV, drains the valley through the northeast. The groundwater table across the KV floor typically varies between 5 and 13 m below the land surface during the dry season (Hemlin Söderberg Citation2014). A prominent hydrological feature of the KV drainage basin is the seasonal wetland that covers a large part of the valley floor as well as its related flood plain. The wetland was designated as a Ramsar site in 2002 (www.ramsar.org) and is considered vital for endemic species as well as an important source of nutrition, water and income for many local villages.
Figure 1. Geographic location of the Kilombero Valley. Topography, based on SRTM data (Farr et al. Citation2007), and river network are shown together with the Kiburubutu Catchment and the catchments for the wetland inlet and outlet sample sites considered (solid black lines), as well as the alluvial plain (dotted line).
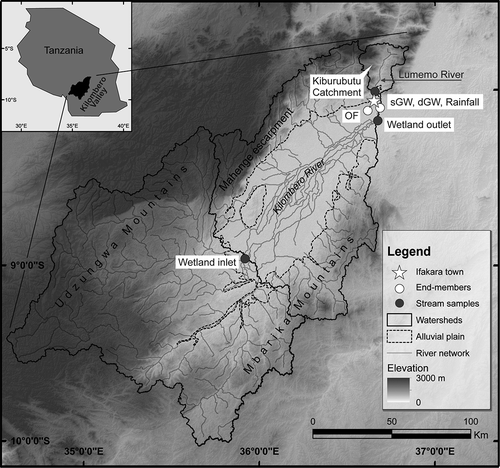
The Lumemo River is one of many tributaries to the Kilombero River and the main river of the Kiburubutu Catchment. It is situated in the northeastern part of the KV and joins the Kilombero River downstream of the main Kilombero Wetland (). The Lumemo River was sampled at the (unfortunately non-functioning) streamflow gauging station 1KB14, which has historically measured the Lumemo River at Kiburubutu. This streamflow gauge constitutes the outlet of the Kiburubutu Catchment, which has an area of 580 km2 with an elevation of 300–2500 m a.s.l. The annual average discharge during the period 1967–1988 was 2.9 m3 s−1, with the highest discharge during April with an average of 6.1 m3 s−1 and the lowest discharge during October with an average of 0.8 m3 s−1. The catchment is dominated by mountainous areas, footridges and footslopes, forming a single valley before its outlet in the alluvial plain. Seasonally flooded areas are also present in lower elevations. The mountainous areas are covered by well-drained Humi-Umbric Nitisols, while the footslopes are covered by Humi-Gleyic Fluvisols, which have the potential to flood seasonally (FAO Citation2003). Characteristics of Umbric Nitisols are high iron content and a high cation exchange capacity, the latter of which is especially large due to the high organic content of the Umbric horizon (FAO Citation2001). Nitisols also have high clay content, typically of at least 30%, though clay content over 60% is not uncommon. The characteristics of the Humi-Gleyic Fluvisols depend largely on the parent material, and can be assumed to be heavily influenced by the Nitisols. Furthermore, a clear stratification with alternating layers of sand and clay at lower elevations and through the alluvial plain can be seen along the riverbanks of the Kilombero River. Finally, similarly to the whole KV, granulitic gneiss underlies the whole of Kiburubutu Catchment (Beck Citation1964).
The climate is sub-humid tropical, with an average daily temperature of around 24.5°C and an average annual precipitation of about 1500 mm during the period 1967–1988. The main driver of seasonality is the passing of the Intertropical Convergence Zone (ITCZ) and its migration first southward and then northward (Camberlin and Philippon Citation2002). This causes distinct wet and dry periods, where the wet period shows a more or less pronounced bimodality, depending on the year. The period December–April typically provides 85–90% of precipitation (Koutsouris et al. Citation2016). Precipitation amounts were gauged at the same location as precipitation chemistry was sampled (see following section) in this study (). The total precipitation during the hydrological year of this current study was 1085 mm. This is in the 47th percentile of the historical rainfall observations.
3 Methodology and data
3.1 Sampling and sample analysis
Stream water was sampled from the Kiburubutu Catchment as well as the inlet and outlet of the large wetland of the Kilombero River (). Discontinuous weekly samples were taken during the period of October 2013 through October 2014 (). Only the stream-water samples from the Kiburubutu Catchment were used in the subsequent G-EMMA (see following sections), while samples collected at the inlet and outlet of the wetland were used for interpreting general flows across the larger KV system. In addition, a limited number of end-members (EMs) were determined based on some initial system understanding from previous studies (Lyon et al. Citation2015) and field visits (). As such, weekly water samples of three different EMs were taken in addition to the stream-water samples (). It should be noted that the sampling locations for the EMs were located outside the Kiburubutu Catchment due to the region’s remoteness and to allow for sampling convenience. These EMs are thus considered representative of the general sources of water contributing to streamflow assuming some large-scale homogeneity of the region. Local residents were contracted and trained to take the water samples in each location, since several of the locations were inaccessible from outside the region during the wet period due to flooding. Due to sampling inconsistencies and environmental difficulties, the resultant EM water samples available for mixing analysis (see following sections) were spread more sporadically across the entire sampling period relative to the stream-water sampling.
Figure 2. Stream-water and end-member (EM) sampling. Each dash represents one sample, or, in the case of rainfall, weekly composited values. Samples were analysed for cations and stable water isotopes (δD and δ18O).
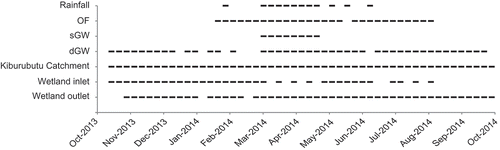
For this study, the deep groundwater (dGW) EM is represented by samples taken from the municipal groundwater well named “Site”, which draws water from the bedrock at a depth of 70 m below the ground level of the valley floor. The shallow groundwater (sGW) EM was taken from the shallow well called “Bethlehem”, which is a communal pumping well used by the local community for drinking water. This well has a depth of 15 m and draws water from the semi-consolidated aquifer. The overland flow (OF) EM was sampled from a flooded area, called the Nakasese area, just upstream of naturally inundated (non-irrigated) rice paddies. This flooded area can drain to the stream and is assumed to be representative of flood water as well as flow pathways at the land surface. Due to the remoteness of the Kiburubutu Catchment and in an attempt to facilitate access during the wet season, all EM sampling points were situated approximately 10 km downstream of the outlet of the Kiburubutu Catchment, nearer the main town of the KV, called Ifakara ().
In addition to the three directly-sampled EMs and similarly to Bazemore et al. (Citation1994), a fourth EM representing baseflow (BF) was defined using stream-water samples taken from the Kiburubutu Catchment during the tail end of the preceding dry period (October 2013 to late December 2013). While this EM potentially overlaps with dGW and sGW, BF likely contains valuable information about the deeper distributions of flow pathways. This is especially true considering the large seasonality expected in hydrological responses across scale (Lyon et al. Citation2015). The inclusion of BF as an EM is thus motivated in part by the limited available data in the region and by assuming the rainy season is representative of a single large-scale event with a considerable signal-to-noise ratio for stream-water chemistry. Finally, precipitation as rainfall was also sampled for comparison with the other water sources sampled. Rainfall was not considered as an EM due to the size of the catchment relative to the variability in rainfall (Koutsouris et al. Citation2016), the speed with which rainwater picks up the chemical signature of the land surface, and our emphasis on estimating potentials for storage mixing.
For all EMs, samples were taken in 2-mL glass vials with a PTFE/silicone/PTFE lid and 12-mL polystyrene vials. For each sample, bottles were rinsed three times with sample water before the final water sampling. All samples were analysed for cation concentrations using inductively coupled plasma (ICP) spectroanalysis with a Thermo iCAP 6500 DUO. The measurement error for two standard deviations is ±5%. Due to the remoteness of the various sample locations, in-field acidification and filtering of the samples was not possible. Furthermore, the samples were stored non-refrigerated for up to 3 months before being acidified and moved to cold storage. The samples were run with an internal standard (NIST 1640a). It should be noted that anion analysis was omitted due to a combination of cost and lab difficulties. As such, clearly not all limitations in this study are due to conducting research in developing regions!
In addition to the cations, samples were collected for stable water isotope analysis. These, again, were stored non-refrigerated for up to 3 months depending on the sample. All samples were collected with zero head space and sealed using parafilm in order to decrease evaporation from the vials during storage. The stable water isotope composition was determined using cavity ring down spectroscopy within an isotope water analyser L2140-i from Picarro at Stockholm University, Department of Geological Sciences. The results were normalized between Standard Light Antarctic Precipitation (SLAP) and Vienna Standard Mean Ocean Water (VSMOW) and reported in per mil deviation relative to VSMOW. The reproducibility of the measurements was calculated to be better than 0.6‰ for δD and 0.1‰ for δ18O.
3.2 End-member mixing analysis in a generalized likelihood uncertainty analysis framework (G-EMMA)
Hydrograph separation techniques have a long history and were first considered as two-component chemistry mass balance, or “mixing”, models to separate storm hydrographs into event and pre-event water (e.g. Johnson et al. Citation1969, Pinder and Jones Citation1969, Dinçer et al. Citation1970, Sklash and Farvolden Citation1979). The EMMA approach was then developed based on these mixing models. EMMA uses multiple tracers in order to estimate the contributions of different types of soil-water solutions, called end-members (EMs), to stream water (Christophersen et al. Citation1990, Hooper et al. Citation1990). Together, these two techniques cover the majority of conducted hydrological tracer studies (Barthold et al. Citation2011). An extensive review of previous hydrograph separation studies can be found in Buttle (Citation1994) as well as in the updated Klaus and McDonnell (Citation2013) and Fang et al. (Citation2015).
The EMMA approach was first developed in Christophersen et al. (Citation1990) and later refined in Hooper (Citation2003). EMMA is an analytical approach that uses principal component analysis (PCA) to first project the chemical composition of stream and EM water onto a lower dimensional U space, describing the water chemistry variability. The positioning of stream and EM water samples in the U space can then be used to determine the contribution from the EMs to the stream water using mass balance equations (Hooper Citation2003). The underlying assumptions of EMMA are that (a) EMs have distinct chemical compositions, (b) the mixing of water is linear, and (c) the tracers are conservative. Since EMMA uses a larger amount of tracers than the number of EMs to explicitly solve the mixing space, the system is overdetermined. This, in turn, renders a more robust EM contribution estimate (Barthold et al. Citation2011). Hooper’s diagnostic tools (Hooper Citation2003) are often used to help identify which tracers should be used in EMMA. More conservative behaviour of tracers can be identified by analysing the structure of residuals between original concentration values and concentration values projected onto the mixing space (Hooper Citation2003). Tracers with random residual patterns are then retained as plausible conservative tracers. Several studies have, however, concluded that the uncertainties in the resulting estimates of EM contribution to stream water can be considerable (e.g. Genereux Citation1998, Barthold et al. Citation2011). The uncertainties stem from either an inability to sufficiently determine the chemical composition of the EMs or that the underlying assumptions of EMMA are, to some degree, violated in the studied system. Furthermore, resulting EM contribution estimates have also been shown to depend on (a) which tracers (Genereux Citation1998, Lyon et al. Citation2009), (b) the number of tracers (Barthold et al. Citation2011), (c) measurement uncertainty (Bazemore et al. Citation1994, Genereux Citation1998) and (d) the number of EMs (Delsman et al. Citation2013) that are included in the analysis.
The method applied here uses EMMA within the generalized likelihood uncertainty estimation (GLUE) framework, which is referred to as G-EMMA (see Delsman et al. Citation2013 for more details). The GLUE framework, developed by Beven and Binley (Citation1992), manages uncertainty by accepting that there are a number of parameter sets that all render acceptable fits to observation data. Thus, by varying input parameters within a plausible range for each model run, the full range of plausible results can be explored with sufficient model runs. When EMMA is applied within GLUE to created G-EMMA, the main strength is that it takes into account, and highlights, both uncertainties in characterization of EMs (conceptual uncertainty) and uncertainties in chemical analysis (measurement uncertainties). The former are taken into account by allowing for time-variant Ems, while the latter are taken into account by varying the chemical signature of each EM in each model run. Therefore, G-EMMA is particularly useful in areas such as KV where local environmental conditions constrain the sampling capabilities and introduce large uncertainties. It helps ensure the robustness of results by explicitly highlighting the range of plausible results.
As described in Delsman et al. (Citation2013), a mixing model in G-EMMA solves the mixing space for a single observation point in time and space and consists of (a) a randomly selected subset of EMs; (b) a randomly selected subset of tracers and tracer concentrations; (c) EM fractions randomly sampled from a uniform Dirichlet distribution, where sampled fractions always sum to 1 in order to maintain mass balance closure; and (d) sampled EM concentrations based on each tracer’s uniform distribution. The uniform approach serves as a minimal assumption of uncertainty distribution (Delsman et al. Citation2013). For G-EMMA, each mixing model can be considered in matrix notation as in Delsman et al. (Citation2013):
for each iteration i, if k and p are the number of EMs and tracers, respectively, considered in that iteration, then li is a vector of EM fractions with length k, B is the k × p matrix of EM concentrations, and xi is a vector of stream-water sample tracer concentrations with length p.
Estimated tracer concentrations, derived from the mixing model, are then compared to stream-water samples (observations) to calculate the likelihood of the mixing model. G-EMMA applies a fuzzy likelihood measure where estimated tracer concentrations within one standard deviation, based on measurement error, of stream-water observations are set to a likelihood of 1, while linearly decreasing from 1 to 0 for estimated concentrations that are within two to three standard deviations. The limit of acceptability of a mixing model where it is deemed behavioural and thus retained is considered when all tracer estimations are within three standard deviations. G-EMMA then uses a Monte Carlo approach to generate a number of mixing models for each stream-water sample, where the resulting likelihood of each mixing model is defined as the average of all tracer estimation likelihoods.
In the current study, the diagnostic tools of Hooper (Citation2003) were first used to identify the most conservative tracers to be used in the G-EMMA. This standard analysis resulted in a set of eight chemical tracers (Ba, Ca, Cu, Mg, Na, S, δD and δ18O) that were considered in this study. In the G-EMMA, each time step consisted of 108 mixing models. For each mixing model, a subset ranging from three to eight tracers was used out of the full set of eight tracers considered in this study. Each mixing model also consisted of a subset of two to four EMs which were randomly selected. Finally, median, inter-quartile and the 5–95 percentile range of estimated EM contributions were calculated based on the retained mixing models.
4 Results
4.1 Hydrochemical characterization
Hydrochemical characteristics of stream water and EMs () were calculated based on the most consistent period of sampling taken during the main part of the wet period (beginning of March 2014 to end of April 2014; see ). All three stream-water sampling locations (namely Kiburubutu Catchment, Wetland inlet and Wetland outlet) showed variability when compared to each other. Several of the hydrochemical tracers had large differences between EMs (). As such, principal component analysis (PCA) based on a covariance matrix was used as described in Christophersen and Hooper (Citation1992) to determine the potential variability explained by the four EMs considered (analysis not shown). The data were centred and normalized prior to the PCA in order to avoid the effect of large differences in concentration ratios. From that analysis, the subsequent G-EMMA application in the Kiburubutu Catchment (see following section) can, at most, be expected to explain 75% of streamflow.
Table 1. Descriptive statistics of sampled rainfall, stream and EM water during the whole sampling period, with “n” as the number of samples. Tracers included in the GLUE end-member mixing analysis (Ba, Ca, Cu, Mg, Na, S, δD and δ18O) are marked with*.
4.2 EM contribution estimation
The G-EMMA model runs for the Kiburubutu Catchment yielded on average approximately 800 behavioural models out of a total of 108 models for each stream-water sample. While seemingly low, this is similar to the ratio of behavioural models to total runs reported in Delsman et al. (Citation2013). The low number of behavioural models is largely due to the number of tracers considered and the randomized sampling procedure of tracers considered for each mixing model. The behavioural models yielded in this study provide robust insight into the seasonal contributions of the various EMs to streamflow and related uncertainties in EM contribution estimates.
Looking at the EM contributions, the OF stream-water contribution was estimated to increase towards the end of the first mode of the bimodal rainy season, remain relatively constant, and then decrease after the peak of the heavy rains (). This response signifies a delayed wet-up period prior to the occurrence of a strong OF contribution in the Kiburubutu Catchment. Based on the median of the G-EMMA behavioural models, the OF contribution ranged from 0 to 10% during the preceding dry period and from 8 to 32% during the wet period. Averaging seasonally, OF (and almost all EMs) had large uncertainty ranges when considering the 5–95 percentile range of behavioural models (). Still, there was a clear increased contribution moving from the dry period to the wet period. The sGW showed an increased contribution to streamflow in response to the rains based on the median of the behavioural models as well as inter-quartile range estimates. This contribution was delayed in that it increased further when OF decreased towards the end of the wet period. Again considering the median of the behavioural models, sGW contributions to streamflow ranged between 5 and 17% during the preceding dry period and between 23 and 72% during the wet period (). The BF showed a strong response following from the increased OF and sGW contributions when looking at the median and inter-quartile range of the behavioural models. The BF contribution subsequently decreased during the wet period from a range in the median of behavioural models of 70–92% during the preceding dry period to 0–54% during the wet period. Finally, the estimated dGW contribution to streamflow was close to 0% throughout the year when looking at the median of the G-EMMA behavioural model estimates, and only up to 10% when considering the upper quartile. There was no clear seasonal shift in the dGW contribution as was seen in the other three EMs (Fig. 4).
4.3 Origin of the spatial and temporal variations of tracers
Based on the sampling conducted at different locations, there were three hydrochemical responses that typified the response of the stream water. These can be highlighted through consideration of the time series for Ba, Fe and δ18O (). These time series of hydrochemistry can be interpreted conceptually. For example, Ba showed little similarity in either concentrations or variability between the dGW and the stream water either at the Kiburubutu Catchment or at the wetland inlet and outlet. The Ba concentration in the dGW was an order of magnitude higher than in any of the stream-water samples, likely indicating that dGW is absent from the stream water through the entire valley system.
Figure 5. Time series of hydrochemical concentrations and isotopic compositions in stream, end-members (EMs) and rainfall water. The difference in response to the seasonality of precipitation patterns between storages gives an indication of regional water fluxes through different source water storages. Stream-water samples were taken at the outlet of Kiburubutu Catchment, the wetland inlet and the wetland outlet. Sampled EMs were overland flow (OF), shallow groundwater (sGW) and deep groundwater (dGW).
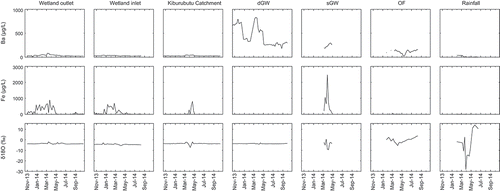
While Fe is considered non-conservative and thus excluded from the G-EMMA, the large differences between sGW Fe concentrations relative to stream water and other EM concentrations likely provides some qualitative information for tracing hydrological flows (). For example, as Fe content is high in the Umbric Nitisol soils (FAO, Citation2001) that dominate the upland and mountainous positions across the region, the apparent spatiotemporal patterns of hydrochemistry () indicate some potential for a flux of water through sGW zones (i.e. below the surficial flooding and above the deep bedrock). This flux could connect, for example, the upland mountainous region surrounding the alluvial plains (where mountain block and/or mountain front recharge occurs) with the streams.
Lastly, the δ18O signature from the rainfall sampling showed fluctuations ranging from +14.2 to −28.3‰ (). In comparison, this fluctuation was fairly dampened when looking at the stream water at different locations. For example, sGW appears to have a less dampened response relative to the stream water. The sGW samples ranged from +0.6 to −9.6‰ for δ18O composition. The OF exhibited a similar δ18O signal with compositions that ranged between +3.6 and −5.5‰ (). This response in sGW demonstrates the potential for localized recharge zones both in the alluvial fan and in footslopes, allowing precipitation to quickly move down through the soil profile.
5 Discussion
5.1 End-member mixing analysis and uncertainties
As seen in the results, estimates of EM contributions to streamflow had a large uncertainty range for many of the EMs (). Using the full range of behavioural models, for example, rendered estimates from 0% to near 100% during parts of the study period for both sGW and BF contributions to streamflow. While the inter-quartile ranges of the contribution estimates were better constrained, sGW and BF still had an inter-quartile range of contributions up to approximately ±22 percentage points of the median model on an annual basis. The uncertainties in this study derive from both uncertainties in the conceptual model (i.e. EMs chosen to sample) and measurement uncertainties. The former are in part a consequence of the difficulty found working in remote regions with limited accessibility and also reflect data and process understanding limitations (Lyon et al. Citation2015). The latter include both the capacity to adequately sample the selected EMs in space and time and analytical uncertainties (i.e. the difficulty of field and lab work).
Previous studies have shown that both types of uncertainties can be large, and this is something we can concur with based on the G-EMMA work presented here. The OF water chemistry can be used as an example of the potential prevalence of measurement uncertainty and limited representativeness of EMs. While OF is occurring during the main part of the wet period, there are also shorter periods of time where this water stagnates such that tracer concentrations could increase due to evaporation. Given that we sampled in a naturally inundated rice paddy to represent OF, there may be a disproportionate amount of time that the water is standing (and not flowing) at the land surface. This may to some extent explain the higher concentrations of some tracers in the OF relative to the sGW (). As such, our sampled EM may of course not be entirely representative of the full extent of OF water across the entire Kiburubutu Catchment. This clearly is a common EMMA issue (e.g. Hooper et al. Citation1990, Soulsby et al. Citation2003) and could bias our results. Still, given the increase in OF contribution moving from the dry through to the wet season (), we are confident that we have captured this EM to at least a first order, given the conceptual uncertainty associated with this study.
Such conceptual uncertainty is particularly prominent in larger catchments (Durand and Juan Torres Citation1996). Consequently, the upper limits for when EMMA is applicable have been a source of debate. Several authors have stated that care should be taken using mixing models in catchments even as small as 1 km2 (e.g. Burns et al. Citation2001). Others have implemented EMMA in catchments up to 2050 km2 (e.g. St Amour et al. Citation2005, Barthold et al. Citation2011). Regardless, the successful application of EMMA (such as in the 580 km2 Kiburubutu Catchment) may be attributed to a dampening of small-scale heterogeneities allowing for chemical characterization based on dominant flow pathways and strong seasonal signals (Soulsby et al. Citation2003, St Amour et al. Citation2005). We thus are leveraging the large signal-to-noise ratio due to the seasonality across KV in our experimental design for this application of G-EMMA. This does, of course, have implications on the potential validity of the underlying assumptions of the methodology considered, further motivating the need to quantify uncertainties.
Delsman et al. (Citation2013), for example, quantified conceptual uncertainty and found that it could have a large impact on EM contribution estimations. Bazemore et al. (Citation1994) estimated measurement uncertainty and found that mixing fractions ranged from 0 to 40% for sGW and from 0 to 69% for groundwater when implementing a Monte Carlo approach to a hydrograph separation for temperate headwater streams. Genereux (Citation1998) also had similar uncertainty bounds as Bazemore et al. (Citation1994) when applying a mathematical uncertainty analysis approach. The uncertainty ranges found here (considering the inter-quartile estimates in ) are thus in line with several previous studies. This demonstrates that even though there are large challenges in characterizing and measuring EMs in remote areas, a low-intensity sampling programme can be utilized to gain understanding of the hydrological system when executed in a system with a large signal-to-noise ratio while considering uncertainties.
Furthermore, we echo here the sentiment of Uhlenbrook and Hoeg (Citation2003), Soulsby et al. (Citation2003) and Inamdar et al. (Citation2013), who concluded that, due to the uncertainties, EMMA results should predominantly be used qualitatively for system understanding and not taken as true values. In addition, we posit a corollary that in cases of data limitation, particularly for regions facing rapid development, such qualitative system understanding may constitute a quantum leap forward when it comes to successful management of limited resources.
5.2. Still, can we make interpretations?
Often in hydrology we are faced with limitations imposed by funds allocated, analysis equipment available, remoteness of sampling locations, time available for field investigations or difficult conditions. This current study is no exception, since all of these have played a role. Further, as considered above, we might be pushing some of the upper limits of applicability with regards to EMMA approaches when considering the size of the catchment explored. Nevertheless, the differentiation between wet and dry period and the partitioning of flows that was seen in end-member contributions, even with rigorous uncertainty analysis (), showed that the strong signal-to-noise ratio and experimental design could be leveraged to gain insights into streamflow generation processes in the KV. The results of this study also complement between our analyses at different locations and system understanding obtained through previous hydrometric-based studies, such as in Lyon et al. (Citation2015).
For example, OF contributions are low during the first mode of the wet period and then have a larger contribution during the second mode for the Kiburubutu Catchment (). The lack of initial response for OF contributions could be explained by an initial requirement of wetting up the system to (a) overcome a predominantly vertical water flux driven by evapotranspiration during the latter part of the dry season; and (b) to allow for swelling of clay layers that subsequently impede infiltration in the latter part of the wet period. Similar results are seen in Lyon et al. (Citation2015) based on a hydrometric streamflow assessment for the smaller and upland catchments in the region. The OF and sGW time series signatures () indicate that, while flooding occurs for much of the region, precipitation can still likely bypass some places, providing local recharge in the alluvial plain and lower-lying regions. Conceptualization of a hydrological system exhibiting patchy infiltration across the KV is supported by double-ring infiltration measurements in Hemlin Söderberg (Citation2015). That study measured large differences in infiltration rates around the vicinity of where the EMs for this study were sampled. Specifically, more clay-rich positions had infiltration rates in the order of 10 mm h−1, while sand-rich positions had rates of around 100 mm h−1. Before OF can occur it appears that these clay layers require initial wetting in order to swell. This is reflected in the G-EMMA results in the delayed contribution of OF relative to the onset of the rainy period ().
Flipping over to the groundwater, dGW constitutes a small, almost negligible, fraction of streamflow according to G-EMMA estimations. In addition, stream-water measurements of Ba show consistently low concentrations across all three stream sampling locations (Kiburubutu Catchment, Wetland inlet, Wetland outlet) while Ba concentrations are of an order of magnitude larger in the deep (bedrock) groundwater. This difference is consistent during both the wet and the dry season. There are thus indications of little contact between these deep layers of bedrock and the river system across all scales. The hard granulitic gneiss bedrock underlying the region likely serves as an impervious basement to the sand–clay aquifer. As such, the renewable groundwater available for pumping to, for example, irrigate within the valley may be rather finite. It may be limited, as is the case in other Tanzanian and eastern African systems, to about 100 m of accessible aquifer, of which only a fraction is annually renewed (MacDonald et al. Citation2012) and which is by and large recharged in the upland, mountainous systems surrounding the clay-rich valley. As such, the long-term sustainability of excessive pumping of groundwater or reallocation of mountain front streams to support irrigation development for rapid agricultural intensification may be questionable.
Lastly, sGW had a marked increase in stream-water contribution at the start of the wet period (). Although there are considerable uncertainties due to the distance between sampling sites of the different EMs, there was also a large difference in the chemical signature of Fe concentrations between the sGW and the other EMs (). The chemical signature of stream-water samples showed increases in Fe concentrations across all scales during the wet season (). This, as previously presented and consistent with the G-EMMA results, likely indicates that water flowing through the sGW storage contributes to stream water throughout the wet season at all three stream-water sampling scales.
6 Concluding remarks
In spite of the difficult environment and the many potential sources for uncertainties in this study, new conceptual insights into rainfall–runoff processes could be gained. This highlights that there is a large potential in applying advanced techniques even with a relatively sparse sampling procedure under tough conditions. Specific to this study, the insights complement (and extend) previous hydrometric analysis and help resolve our understanding of this Tanzanian system. It is clear, however, that some of the variability in the storage mixing that generates streamflow across the Kiburubutu Catchment is missed in this analysis. Even so, fully exploring the uncertainty of the estimates can allow for some robust interpretations, which potentially enriches our ability to constrain model development and guides our future investigations. We feel that this is encouraging as society increasingly looks to science to help secure sustainability and climate resilience both now and into the future. With that, one take-home message here is that rather than shying away from difficult environments or data limitations with our more advanced techniques, researchers should be explicitly targeting them, since significant advances can be made both for the research community’s understanding and for society in general. A key to doing this might be to take advantage of large natural variability in, for example, climate signals through our experimental design and full consideration of uncertainty.
Acknowledgements
The authors would like to thank Joost Delsman for technical support with the G-EMMA software. All software and source code written to facilitate the G-EMMA procedure are available for download at http://g-emma.deltares.nl/. Further, Rick Hooper and Keith Beven are thanked for insights and comments on an early version of this work. The authors would also like to thank the University of Dar es Salaam (UDSM) for their support. Special thanks to Lukas Kolowa at UDSM for the invaluable support in the field that made this study possible and the companionship that made the long hours on bumpy roads enjoyable. Finally, we thank two anonymous reviewers and Laurent Ruiz for their efforts to help improve the presentation of the study.
Supplementary material
Hydrochemical data used in this study are available at the Bolin Centre Database (http://bolin.su.se/data/Koutsouris-2017).
Disclosure statement
No potential conflict of interest was reported by the authors.
Additional information
Funding
References
- Barthold, F.K., et al., 2011. How many tracers do we need for end member mixing analysis (EMMA)? A sensitivity analysis. Water Resources Research, 47 (8), W08519–W08519. doi:10.1029/2011WR010604
- Bazemore, D.E., et al., 1994. The role of soil water in stormflow generation in a forested headwater catchment: synthesis of natural tracer and hydrometric evidence. Journal of Hydrology, 162 (1–2), 47–75. doi:10.1016/0022-1694(94)90004-3
- Beck, A.D., 1964. The Kilombero Valley of south-central Tanganyika. East African Geographical Review, 2, 37–43.
- Beven, K. and Binley, A., 1992. The future of distributed models: model calibration and uncertainty prediction. Hydrological Processes, 6 (3), 279–298. doi:10.1002/hyp.3360060305
- Burns, D.A.M., et al., 2001. Quantifying contributions to storm runoff through end-member mixing analysis and hydrologic measurements at the Panola Mountain research watershed (Georgia, USA). Hydrological Processes, 15 (10), 1903–1924. doi:10.1002/hyp.246
- Buttle, J.M., 1994. Isotope hydrograph separations and rapid delivery of pre-event water from drainage basins. Progress in Physical Geography, 18 (1), 16–41. doi:10.1177/030913339401800102
- Camberlin, P. and Philippon, N., 2002. The east African march – may rainy season : associated atmospheric dynamics and predictability over the 1968 – 97 period. Journal of Climate, 15 (9), 1002–1019. doi:10.1175/1520-0442
- Christophersen, N., et al., 1990. Modelling stream water chemistry as a mixture of soilwater end-members — A step towards second-generation acidification models. Journal of Hydrology, 116 (1–4), 307–320. doi:10.1016/0022-1694(90)90130-P
- Christophersen, N. and Hooper, R., 1992. Multivariate analysis of stream water chemical data: the use of principal components analysis for the end‐member mixing problem. Water Resourses Research, 28 (1), 99–107. doi:10.1029/91WR02518
- Delsman, J.R., et al., 2013. Uncertainty estimation of end-member mixing using generalized likelihood uncertainty estimation (GLUE), applied in a lowland catchment. Water Resourses Research, 49 (8), 4792–4806. doi:10.1002/wrcr.20341
- Dinçer, T., et al., 1970. Snowmelt runoff from measurements of tritium and oxygen-18. Water Resourses Research, 6 (1), 110–124. doi:10.1029/WR006i001p00110
- Durand, P. and Juan Torres, J.L., 1996. Solute transfer in agricultural catchments: the interest and limits of mixing models. Journal of Hydrology, 181 (1–4), 1–22. doi:10.1016/0022-1694(95)02922-2
- ERB (Economic Research Bureau), 2006. A study to establishing mechanism for payments for water environmental services for the Rufiji River Basin in Tanzania. Dar es Salaam: University of Dar es Salaam.
- Fang, F., et al., 2015. Progress on the use of stable isotope techniques in catchment hydrograph separation: a review. Meteorological Environmental Research, 6 (2), 1–5.
- FAO (Food and Agriculture Organization of the United Nations), 2003. Multipurpose africover databases on environmental resources (MADE). Rome: FAO. Available from: http://www.africover.org/index.htm [ Accessed 13 Apr 2012].
- FAO (Food and Agriculture Organization of the United Nations), 2001. Lecture notes on the major soils of the world. World soil resources reports. Rome: FAO.
- Farr, T.G., et al., 2007. The shuttle radar topography mission. Reviews of Geophysics, 45 (2005), 1–33. doi:10.1029/2005RG000183
- Genereux, D., 1998. Quantifying uncertainty in tracer-based hydrograph separations. Water Resourses Research, 34 (4), 915–919. doi:10.1029/98WR00010
- Hemlin Söderberg, M., 2014. Estimating Groundwater Availability and Variability in Kilombero Valley, Tanzania. Unpublished thesis. Stockholm: Stockholm University.
- Hemlin Söderberg, M., 2015. Measuring Soil Infiltration Rates in cultivated land - A case study of Ifakara, Tanzania. Unpublished thesis. Stockholm: Stockholm University.
- Hooper, R.P., et al., 1990. Modelling stream water chemistry as a mixture of soilwater end-members — an application to the Panola Mountain catchment, Georgia, U.S.A. Journal of Hydrology, 116 (1–4), 321–343. doi:10.1016/0022-1694(90)90131-G
- Hooper, R.P., 2003. Diagnostic tools for mixing models of stream water chemistry. Water Resourses Research, 39 (3). doi:10.1029/2002WR001528
- Inamdar, S., et al., 2013. Temporal variation in end-member chemistry and its influence on runoff mixing patterns in a forested, Piedmont catchment. Water Resourses Research, 49 (4), 1828–1844. doi:10.1002/wrcr.20158
- Johnson, N.M., et al., 1969. A working model for the variation in stream water chemistry at the hubbard brook experimental forest, new hampshire. Water Resourses Research, 5 (6), 1353–1363. doi:10.1029/WR005i006p01353
- Klaus, J. and McDonnell, J.J., 2013. Hydrograph separation using stable isotopes: review and evaluation. Journal of Hydrology, 505, 47–64. Elsevier B.V. doi:10.1016/j.jhydrol.2013.09.006
- Koutsouris, A.J., et al., 2016. Comparing global precipitation data sets in eastern Africa: a case study of Kilombero Valley, Tanzania. International Journal of Climatology, 36 (4), 2000–2014. doi:10.1002/joc.4476
- Leng, G., et al., 2014. Modeling the effects of groundwater-fed irrigation on terrestrial hydrology over the conterminous United States. Journal of Hydrometeorology, 15 (3), 957–972. doi:10.1175/JHM-D-13-049.1
- Lyon, S.W., et al., 2008. Characterizing the response of a catchment to an extreme rainfall event using hydrometric and isotopic data. Water Resourses Research, 44 (6). doi:10.1029/2007WR006259
- Lyon, S.W., et al., 2009. A tale of two isotopes: differences in hydrograph separation for a runoff event when using δD versus δ 18 O. Hydrological Processes, 23 (14), 2095–2101. doi:10.1002/hyp.7326
- Lyon, S.W., et al., 2015. Interpreting characteristic drainage timescale variability across Kilombero Valley, Tanzania. Hydrological Processes, 29 (8), 1912–1924. doi:10.1002/hyp.10304
- MacDonald, A.M., et al., 2012. Quantitative maps of groundwater resources in Africa. Environmental Research Letters, 7 (2), 024009. doi:10.1088/1748-9326/7/2/024009
- Pinder, G.F. and Jones, J.F., 1969. Determination of the ground-water component of peak discharge from the chemistry of total runoff. Water Resourses Research, 5 (2), 438–445. doi:10.1029/WR005i002p00438
- SAGCOT (Southern Agricultural Growth Corridor of Tanzania) Centre Ltd., 2011. Southern agricultural growth investment blueprint. South. agric. growth corridor Tanzania - Invest. Bluepr. Dar es Salaam: SAGCOT Centre Ltd. Available from: http://www.sagcot.com/who-we-are/what-is-sagcot/
- Sklash, M.G. and Farvolden, R.N., 1979. The role of groundwater in storm runoff. Journal of Hydrology, 43 (1–4), 45–65. doi:10.1016/0022-1694(79)90164-1
- Soulsby, C., et al., 2003. Identifying and assessing uncertainty in hydrological pathways: a novel approach to end member mixing in a Scottish agricultural catchment. Journal of Hydrology, 274 (1–4), 109–128. doi:10.1016/S0022-1694(02)00398-0
- St Amour, N.A., et al., 2005. Isotopic time-series partitioning of streamflow components in wetland-dominated catchments, lower Liard River basin, Northwest Territories, Canada. Hydrological Processes, 19 (17), 3357–3381. doi:10.1002/hyp.5975
- Uhlenbrook, S. and Hoeg, S., 2003. Quantifying uncertainties in tracer-based hydrograph separations: a case study for two-, three- and five-component hydrograph separations in a mountainous catchment. Hydrological Processes, 17 (2), 431–453. doi:10.1002/hyp.1134