ABSTRACT
Different satellite-based radiation (Makkink) and temperature (Hargreaves-Samani, Penman-Monteith temperature, PMT) reference evapotranspiration (ETo) models were compared with the FAO56-PM method over the Cauvery basin, India. Maximum air temperature (Tmax) required in the ETo models was estimated using the temperature–vegetation index (TVX) and an advanced statistical approach (ASA), and evaluated with observed Tmax obtained from automatic weather stations. Minimum air temperature (Tmin) was estimated using ASA. Land surface temperature was employed in the ETo models in place of air temperature (Ta) to check the potency of its applicability. The results suggest that the PMT model with Ta as input performed better than the other ETo models, with correlation coefficient (r), averaged root mean square error (RMSE) and mean bias error (MBE) of 0.77, 0.80 mm d−1 and −0.69 for all land cover classes. The ASA yielded better Tmax and Tmin values (r and RMSE of 0.87 and 2.17°C, and 0.87 and 2.27°C, respectively).
Editor R. Woods Associate editor P. Srivastava
1 Introduction
It is essential to assess the key components of the hydrological cycle and also to manage water resources efficiently at the basin scale. Thus, it is crucial to have an accurate estimation of reference evapotranspiration (ETo) over the Cauvery basin, which is one of the important river basins of peninsular India. ETo is a climatic factor, estimated from climatic variables such as air temperature (Ta), solar radiation (Rs), relative humidity and wind speed. Owing to the difficulty in direct measurement of ETo, indirect methods have been proposed, classified on the basis of their requirements of climatic variables, such as combination-type, radiation-type, temperature-type and mass-transfer-type methods. The Penman-Monteith (PM) method of the Food and Agriculture Organization (FAO) (FAO56-PM) is a combination method, and has a record of deriving accurate ETo in most climatic regions, since it considers all the above-mentioned climatic variables. It is also recommended as a standardized method by the FAO. Most of the climatic variables required for the FAO56-PM method are hard to obtain because of the difficulty in maintaining the prescribed hypothetical conditions required for the estimation of ETo at weather stations. Hence, alternative methods have been proposed by various researchers (Blaney and Criddle Citation1962, Hargreaves and Samani Citation1985, De Bruin Citation1987), as these require fewer climatic variables and are readily available for most of the climatic conditions and regions. But many of these alternative methods are site-specific and require calibration before application to another region. Consequently, many researchers have compared various methods and validated them with the FAO56-PM method at point scale for their particular study region (Gavilan et al. Citation2006, Douglas et al. Citation2009, Aguilar and Polo Citation2011, Fotios and Andreas Citation2011, Valipour Citation2012, Citation2014, Citation2015a, Citation2015b, Citation2017, Todorovic and Pereira Citation2013, Raziei and Pereira Citation2013a, Kisi Citation2014, Senatore et al. Citation2015, Almorox et al. Citation2015, Valipour et al. Citation2017). In India, Nandagiri and Kovoor (Citation2006) evaluated seven models for different ranges of Indian climate at the point scale.
Climatic variables obtained from meteorological stations are at the point scale. Using these, it is difficult to obtain spatial variations of ETo. Even though spatial interpolation techniques have been developed to obtain spatial variations of climatic variables, these require a dense network of high-quality meteorological stations (Raziei and Pereira Citation2013b). Such networks are costly to install and maintain in developing countries. In contrast, remote sensing techniques can provide the required data for estimation of ETo at high spatial and temporal resolution in a feasible way. Hence, some authors have proposed a simplified methodology for the estimation of ETo using remotely sensed data, employing available ETo models. Rivas and Caselles (Citation2004) examined the utility of land surface temperature (LST) over Argentina to estimate spatial ETo using an equation based on the PM model. Temperature-based semi-empirical ETo models were examined over Kenya by Maeda et al. (Citation2011) using only Moderate Resolution Imaging Spectroradiometer (MODIS) LST. A few researchers have used Ta and geostationary satellite Rs data in radiation-based models to estimate ETo for different study regions (e.g. Bois et al. Citation2008, De Bruin et al. Citation2010, Cammalleri and Ciraolo Citation2013, Cruz-Blanco et al. Citation2014a, Citation2014b). In some studies, numerical weather forecast data were successfully used in temperature- and radiation-based ETo models (Silva et al. Citation2010, Cruz-Blanco et al. Citation2014a, Citation2015, Citation2014b). However, numerical weather forecast data are available at a coarse spatial resolution. In the above-mentioned studies, either satellite-based radiation or temperature-based ETo models were employed for the particular study region, but none of the studies compared the performance of these models. Thus, there is an imperative need to study ETo models, because many earlier studies were site-specific and performance varies depending on the prevailing weather variables. Moreover, these studies utilized meteorological station Ta at the point scale, or very coarse resolution forecast Ta data.
The Ta measured at 2 m above the ground surface is one of the important influencing parameters for ETo. Zaksek and Schroedter-Homscheidt (Citation2009) broadly classified the satellite-based derivation of Ta into three groups: (a) statistical approaches (simple and advanced); (b) temperature–vegetation index (TVX) approaches; and (c) energy balance approaches. Statistical approaches are site-specific and need local parameterization. The TVX approach is applicable during daytime (Prihodko and Goward Citation1997). Energy balance approaches are complex, time-consuming and require a large amount of data. Several authors have studied these approaches individually for different climatic regions (Mostovoy et al. Citation2006, Stisen et al. Citation2007, Vancutsem et al. Citation2010, Nieto et al. Citation2011, Benali et al. Citation2012, Lin et al. Citation2012, Shah et al. Citation2013, Wenbin et al. Citation2013, Rhee and Jungho Citation2014, Zeng et al. Citation2015). However, to date, no study has compared the performance of these approaches. Hence, there is a need to examine the usage of satellite-based Ta methods, since Ta is an indispensable parameter for ETo estimation and is available at fine spatial and temporal resolutions. Generally, LST is used for Ta estimation in the above-mentioned approaches, due to its regional–global availability from satellites and its close relationship to Ta (Gallo et al. Citation2011).
The main objective of this study is to obtain ETo at high spatio-temporal resolution using limited weather variables. Therefore, performance evaluation of radiation-based (Makkink and Makkink-Advection), temperature-based (Hargreaves-Samani, HS), simple LST-based equation (SLBE), and Penman-Monteith temperature (PMT) models was carried out with the FAO56-PM method. For these ETo models, minimum Ta (Tmin) and maximum Ta (Tmax) are crucial parameters. The quality of Ta data influences the ETo values. Hence, comparison of the advanced statistical (ASA) and TVX approaches for estimation of Tmax and Tmin was conducted. Furthermore, LST was replaced by Ta in the ETo models to check its pertinence. Comparison between ETo values estimated by employing the above-mentioned ETo models using LST and Ta (obtained from the best approach among TVX and ASA) as inputs was carried out. A schematic representation of these procedures is provided in .
2 Study area
The Cauvery River is one of the major rivers of peninsular India and the river basin extends between 10°05′–13°30′N and 75°30′–79°45′E. The River basin covers an area of 81 155 km2 and lies in the states of Karnataka, Kerala, Tamil Nadu and Pondicherry. It is one of the largest rivers of southern India and depends heavily on monsoon rains; hence it is prone to droughts when the monsoon fails. There is a serious conflict between Karnataka and Tamil Nadu states on the issue of sharing the river water. Due to this, accurate water management has become essential. In terms of physiography, the basin can be divided into three parts: the Western Ghats area, the Plateau of Mysore and the Delta area (CWC and NRSC (Central Water Commission and National Remote Sensing Centre) Citation2014). The Delta forms the lower part of the Cauvery basin and is the most fertile tract, while the Western Ghats consists of a mountainous region and runs parallel to the western coast ()). The mean Tmax and Tmin are 34.31 and 17.15°C, respectively, for the period 1969–2004 (http://www.india-wris.nrsc.gov.in). Precipitation varies substantially over the basin; while the western part of the basin receives the southwest monsoon (June–September), the northeast monsoon (October–December) serves the eastern part. The rainfall during other periods is insignificant. The basin receives mean annual precipitation of about 1075 mm/year. Annual rainfall (1970–2004) varies from 1700 to 3800 mm/year in the Western Ghats and 600 to 800 mm/year in the Plateau of Mysore, while the Delta area receives 500–1000 mm/year (www.india-wris.nrsc.gov.in). Land use/land cover of the basin is broadly classified as agricultural, non-agricultural, forest and habitation land. Data for the year 2012 show more than 60% of the land in the Cauvery basin is cultivable, 1.15% is urban/built-up, 17.91% is forest regions and the remainder is non-cultivable. Finger millet and paddy are the principal crops of the Mysore Plateau and Delta regions, respectively.
Figure 2. (a) Location of the study area with the automatic weather stations and land cover types indicated by numbers. (Stations 1–5 belong to land cover class F, 6–14 – class C, 15–18 – class U/BP and 19–35 – class C/NV. (b) MODIS land use/land cover map of the study area showing location of the Berambadi site, details of land cover classes, and elevation of the study area. F: forest, C: croplands and U/B: urban/built-up, C/NV: croplands/natural vegetation.
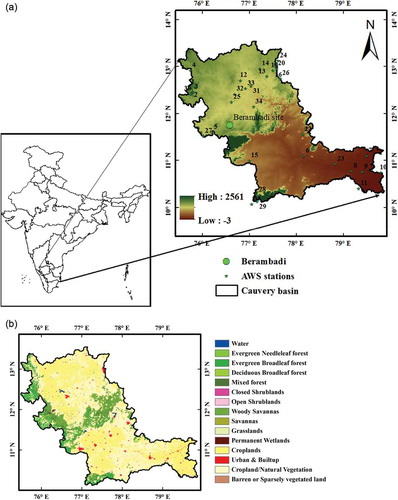
3 Methodology
3.1 Data used and pre-processing
Data on Tmax, Tmin, relative humidity, wind speed and sunshine hours required for the estimation of ETo using the FAO56-PM method were acquired from the automatic weather stations (AWS) installed by the Indian Space Research Organization (ISRO). A total of 35 AWSs located within the basin were considered in this study (). According to the Indian Council of Agricultural Research (ICAR), Cauvery basin comprises three agro-climatic zones, namely the southern plateau and hill region, the east coast plains and hill region, and the west coast plain and ghats region (Ghosh Citation1991). This categorization is based on soil type, climate, temperature and its variations, rainfall and other agro-meteorological characteristics, as well as water demand and supply characteristics, including quality of water and aquifer conditions. Available stations within the Cauvery basin were grouped into three climatic regions according to ICAR, namely semi-arid, semi-arid to humid, and humid. In addition, observed Rs data obtained from a pyranometer for the years 2013 and 2014 were collected for the Berambadi site, which is located in the Gundalpet taluk of Karnataka State (11.76°N; 76.58°E) at an altitude of 870 m a.s.l. ()). The LST (MYD11A1) and reflectance (MYD09GA) values of near-infrared (NIR), Red, Blue and SWIR2 data were obtained from the MODIS sensor of the Aqua satellite. Since 2002, MODIS has been carried on the NASA (US National Aeronautics and Space Administration) polar-orbiting Aqua satellite, which passes from south to north at about 01:30/13:30 h local solar time each day in sun-synchronous orbit. The MODIS sensor, with 36 bands, provides near-daily global coverage with high spatial resolution. Since the passing time of the satellite over the study region is in the afternoon, maximum LST can be seen during this time. Minimum LST occurs in the early morning. The difference between minimum LST and observed LST at around 02:00 h is the least. In this study, night-time LST refers to minimum LST and daytime LST refers to maximum LST; Tmax and Tmin measurements were selected corresponding to these. The Rs data were taken from the Kalpana-1 satellite, a geostationary satellite that was launched in 2002. It carries a Very High Resolution Radiometer (VHRR)/2 sensor and receives information from visible, infrared and thermal infrared bands at spatial resolutions of 2 km and 8 km. The MODIS LULC (MCD12Q1) product was used for segregating the LST pixels according to the International Geosphere Biosphere Programme (IGBP) classification (Friedl et al. Citation2010). Digital elevation data were obtained from the Shuttle Radar Topography Mission (SRTM). All the datasets considered are from the period 2012–2014. Details of the datasets used are provided in .
Table 1. Description of land cover types and climatic characteristics of automatic weather stations in Cauvery basin. Lat.: latitude; Lon.: longitude; LULC: land use/land cover, F: forest, C: croplands, U/B: urban/built-up area, C/NV: croplands/natural vegetation; H: humid, SA: semi-arid, SA-SH: semi-arid/sub-humid.
Table 2. Details of the datasets used. LST: land surface temperature (°C); Rs: solar radiation (MJ m−2 d−1).
The MODIS LST (day/night) projections were changed from sinusoidal to geographical projection by employing a nearest-neighbour method using the MODIS reprojection tool developed by NASA (Dwyer and Schmidt Citation2006). These were aggregated from 1 km to the Kalpana-1/VHRR data at a spatial resolution of 2 km by spatial averaging. Reflectance values of NIR, Red, Blue and SWIR2 spatial resolutions were aggregated from 500 m to 2 km using spatial averaging, and these were used to derive vegetation indices. The MODIS LULC data were upscaled from 500 m to 2 km, and later used to segregate the pixels based on the IGBP classification. The SRTM elevations used in the estimation of Ta (max/min) were aggregated from 90 m to 2 km.
3.2 Estimation of satellite-based air temperature
In this study ASA and TVX approaches were employed for Ta (max/min) estimation and the results were validated with Ta (max/min) obtained from the AWS.
3.2.1 Statistical approaches
Most of the statistics-based approaches developed are based on relating satellite-based LST (max/min) and auxiliary data as predictors, with Ta (max/min) obtained from meteorological stations as the predictand (Mostovoy et al. Citation2006, Cristobal et al. Citation2008, Fu et al. Citation2011, Benali. et al. Citation2012, Rhee and Jungho Citation2014, Xu et al. Citation2014, Zeng et al. Citation2015). In this study, different statistical approaches from simple (one predictor variable, LST) to multiple (more than one predictor) were tested. In addition to LST (day/night), other auxiliary variables such as elevation, latitude, longitude and Julian day were selected as predictors for the estimation of Ta (max/min). Out of 14 models developed by Benali. et al. (Citation2012), eight were selected for analysis of their performance for the study region (). Benali. et al. (Citation2012) used a mixed bootstrap method with jack-knife resampling; however, in this study, only the bootstrap technique was employed to generate samples, since sufficient observed Ta values obtained from the AWSs were available over the study region (Bai and Wei. Citation2008). The eight models were selected depending on the availability of data for the study region: 70% of the available data (for 2012 and 2013) was used for calibration and the remaining 30% for validation of the models, and these datasets were employed in the generation of respective calibration and validation of 1000 bootstrap samples. The Levenberg-Marquardt algorithm was employed to minimize the square of the absolute differences between predictions and observations. The obtained coefficients were later applied to estimate Tmax and Tmin from the validation datasets. The statistical relationship between estimated Tmax/Tmin and observed Tmax/Tmin was quantitatively evaluated by computing statistical error indices. Information about the statistical error indices employed is given in Section 3.4.
Table 3. Goodness-of-fit indicators computed between Ta values obtained from eight models and from AWSs. r: Pearson correlation coefficient; RMSE: root mean square error; NSE: Nash-Sutcliffe efficiency; a to e: model coefficients (see Appendix 3 for values used in ASA); LSTday: daytime land surface temperature, LSTnight: night-time land surface temperature; peak and phase are coefficients of the cosine function; JD: Julian day; ele: elevation.
3.2.2 Temperature–vegetation index (TVX) approach
Prihodko and Goward (Citation1997) proposed a contextual method by fitting a linear relationship between spectral vegetation index (VI) and LST. Estimation of Tmax is based on the assumption that the bulk temperature of an infinitely thick vegetation canopy is close to the ambient air temperature. Several authors have applied the TVX method to check the potential of the method for various climatic regions using data from different sensors (Stisen et al. Citation2007, Vancutsem et al. Citation2010, Nieto et al. Citation2011, Shah et al. Citation2013, Wenbin et al. Citation2013). Nevertheless, the mandatory negative linear relationship between the normalized difference vegetation index (NDVI) and LST is difficult to attain in several conditions due to the influence of seasonality, ecosystem type and soil moisture variability (Benali. et al. Citation2012). Thus, Tmax was calculated by applying maximum VI (VImax) from a simple linear equation, which was obtained by ascertaining the linear relationship between LST and VI. In the past, only NDVI has been considered for Tmax estimation (Prihodko and Goward Citation1997, Stisen et al. Citation2007, Vancutsem et al. Citation2010, Nieto et al. Citation2011). Conventional NDVI is saturated when leaf area index > 3.5 and this can be avoided by employing the enhanced VI (EVI) and global vegetation moisture index (GVMI) (Le Naire et al. Citation2006, Guerschman et al. Citation2009). The GVMI is asymptotically linked to equivalent water thickness (Guerschman et al. Citation2009) and the presence of vegetation moisture influences the variations in Ta. Here, an attempt is made to examine the potential of two other vegetation indices, namely EVI and GVMI, in estimating Tmax. The accuracy of this approach depends on two important points. Firstly, there should be a strict negative relationship between LST and VI and, secondly, the value of VImax, since the assumption of this approach is that LST is of thick vegetation, is close to Ta. Many researchers have considered different values of maximum NDVI depending on their study region, and this varied from 0.65 to 0.90 (Prihodko and Goward Citation1997, Stisen et al. Citation2007, Vancutsem et al. Citation2010, Wenbin et al. Citation2013). In this study, maximum values of NDVI (NDVImax), EVI (EVImax) and GVMI (GVMImax) varied between 0.40 and 0.90 for the various land cover classes individually in the estimation of Tmax for the study region.
where NIR, RED, BLUE, SWIR2 are the reflectances of their respective bands. In this study, the values of G, C1, C2 and L in the EVI equation (Equation (2)), which account for aerosol scattering and absorption (Guerschman et al. Citation2009), are 2.5, 6.0, 7.5 and 1.0, respectively.
3.3 Reference evapotranspiration models
Reference evapotranspiration (ETo) is “the rate of evapotranspiration from a hypothetical reference crop with an assumed crop height of 0.12 m, a fixed surface resistance of 70 sec m−1 and albedo of 0.23, closely resembling the evapotranspiration from an extensive surface of green grass of uniform height, actively growing, well watered and completely shading the ground” (Allen et al. Citation1998, McMahon et al. Citation2013, Pereira et al. Citation2015). The standardized reference evapotranspiration equation can be expressed as (McMahon et al. Citation2013):
where ETo is the daily reference crop evapotranspiration (mm d−1), Tmean is the mean daily Ta (°C) at 2 m, u2 is the average daily wind speed (m s−1) at 2 m, Rn is the net daily radiation (MJ m−2 d−1), G is the soil heat flux (MJ m−2 d−1), es − ea is the vapour pressure deficit (kPa), γ is the psychometric constant (kPa °C−1) and Δ is the slope of the saturation vapour pressure curve (kPa °C−1). In this study, the FAO56-PM equation was applied to estimate ETo at the point scale by applying data of AWSs as inputs. These results were then used for validation of ETo obtained using satellite-based approaches (details are given in the following sections).
3.3.1 Radiation-based models
In 1957, Makkink proposed a radiation-based formula by modifying the Penman equation which requires only Rs and Ta data (Arellano and Irmak Citation2016). De Bruin (Citation1987) further simplified the Makkink equation to estimate ETo accurately under non-water stress conditions (Cruz-Blanco et al. Citation2014b), which can be expressed as:
where is a simplification parameter, which varies from 0.63 to 0.90 for different climatic conditions (De Bruin Citation1987, De Bruin et al. Citation2010, Stewart et al. Citation1999, Schuttemeyer et al. Citation2007, Bois et al. Citation2008, Cammalleri and Ciraolo Citation2013), Rs is solar radiation. and the other parameters are as explained in Section 3.3. De Bruin et al. (Citation2012b) introduced the advection-based revised Makkink equation by accounting for advection effects under semi-arid conditions, which can be expressed as:
3.3.2 Temperature-based models
In the absence of radiation measurements, the simplified temperature models work better in semi-arid and arid climates, as reported by Verhoef and Feddes (cited from Di Stefano and Ferro Citation1997). These models require only Ta or LST data for the estimation of ETo. Many temperature-based models are available and they have been successfully applied worldwide (e.g. Thornthwaite Citation1945, McCloud Citation1955, Hamon Citation1961, Blaney and Criddle Citation1962, Hargreaves and Samani Citation1985). In most cases, the Hargreaves-Samani (HS) equation performed better than other models (Di Stefano and Ferro Citation1997, Gavilan et al. Citation2006, Aguilar and Polo Citation2011, Maeda et al. Citation2011, Todorovic. and Pereira Citation2013, Kisi Citation2014, Almorox et al. Citation2015). Hence, in this study, the HS model was selected among the available empirical temperature-based equations.
3.3.2.1 The Hargreaves-Samani (HS) equation
The HS equation is an empirical equation and requires only Tmean, Tmax, Tmin and extraterrestrial radiation (Ra) for derivation of ETo. This equation tends to overestimate in humid climates, since it requires only Ta data and varies with it, therefore requiring regional calibration for better performance. The HS equation can be written as:
where Ra is extraterrestrial solar radiation (MJ m−2 d−1) and CH is the Hargreaves empirical coefficient, set to 0.0023 for arid and semi-arid regions.
3.3.2.2 The Penman-Monteith temperature (PMT) model
The inputs required in the FAO56-PM equation for ETo estimation are Rn, es − ea and u2, and these may be estimated by employing alternative methods, which use only Tmax and Tmin, in the case of non-availability of other climatic variables over the study region. This approach is known as the Penman-Monteith temperature (PMT) model (Todorovic. and Pereira Citation2013, Raziei and Pereira Citation2013a). In this study, the procedures evolved by Todorovic and Pereira (Citation2013) and Raziei and Pereira (Citation2013a) were followed to estimate the parameters Rn, es − ea and u2, and these were then employed in the FAO56-PM equation (Equation (4)) to derive ETo for the Cauvery basin. The method is elaborated in Appendix 1.
3.3.2.3 The simple LST-based equation (SLBE)
Rivas and Caselles (Citation2004) proposed a new simplified equation for estimation of ETo with LST and local standard meteorological data.
where LST is land surface temperature obtained from satellite data. The parameters a and b depend on the local meteorological data, obtained by segregating the PM equation into a radiation term and an aerodynamic term, and these can be expressed as:
where εs is the emissivity of the surface, σ is the Stefan-Boltzmann constant (4.9 × 10−9 MJ m−2 K−4 d−1), rc is the crop canopy resistance (s m−1), ra is the aerodynamic resistance (s m−1), α is the albedo of the surface, and the other parameters are as elaborated previously.
In this study, in place of Ta data, LST values were employed in the HS (HS_LST), PMT (PMT_LST) and Makkink (Makk_LST) models. As mentioned in Section 3.2, the best Ta values obtained between the two approaches were employed in the HS (HS_Ta), PMT (PMT_Ta), simple Makkink (Makk_Ta) and advection-revised Makkink (Makk_adv_Ta) equations.
3.4 Performance evaluation indices
Estimated Tmax and Tmin were validated with observed Tmax and Tmin values obtained from the AWSs. Furthermore, ETo estimated using temperature- and radiation-based methods was evaluated with ETo calculated using the FAO56-PM model at the AWSs (referred to herein as observed values). The Pearson correlation coefficient (r) was used to measure the correlation between the estimated and observed values. The value of r varies from −1 to +1, where 0 indicates no correlation and ±1 high correlation. The Nash-Sutcliffe efficiency criterion (NSE; Nash and Sutcliffe Citation1970) varies from −∞ to 1. An efficiency of 1 represents a perfect match between estimated and observed values and 0 indicates that the estimated values are equal to the mean of the observed values. Negative NSE indicates that the observed mean is better than the estimated values. This is sensitive to means and variances of the differences found between the observed and estimated values. Root mean square error (RMSE) is one of the commonly used error indices. Lower RMSE indicates better performance of the model (Moriasi et al. Citation2007). Mean absolute percentage error (MAPE) is a measure of prediction accuracy. Mean bias error (MBE) is the average difference between the estimated and observed values. The equations for calculating these goodness-of-fit indicators are given in Appendix 2.
4 Results and discussion
4.1 Estimation of satellite-based air temperature
The values of Tmax and Tmin were estimated using ASA and TVX approaches (as described in Section 3.2). These estimated Tmax and Tmin were validated with the corresponding AWS Ta data. The performance of the selected methodologies was analysed by considering data of all the stations using the statistical error indices r, RMSE and NSE. The better Tmax results obtained among the mentioned approaches and Tmin were utilized in the estimation of ETo.
The error indices of both Tmax and Tmin obtained by the eight models are given in . The results indicate that, of these, Models 3 and 8 performed best, with high r values of 0.84 and 0.83, NSE of 0.71 and 0.68, and low RMSE of 2.25 and 2.29°C, respectively. Together, LSTday and LSTnight could explain 82 and 84% of variability in Tmax and Tmin, respectively. This indicates a strong relationship between LST and Ta. The addition of Julian day and elevation into the model increased the accuracy in Tmax estimation, but did not improve accuracy in Tmin estimation. Coefficients of the considered models (for the estimation of Tmax and Tmin) are provided in Appendix 3. Scatterplots between predicted Tmax, Tmin (obtained from the best models) and observed Tmax, Tmin for the year 2014 are shown in . The results in indicate that Model 3 and Model 8 () estimated Tmin and Tmax more accurately (r, RMSE and NSE of 0.87, 2.27°C and 0.76, and 0.87, 2.17°C and 0.75, respectively) .
Spatial variations of Tmax and Tmin for the year 2014 representing different seasons are shown in ) and (). The Western Ghats have low Tmax and Tmin compared to other regions. This is due to higher elevation and forest cover. Summer season (DOY 89) has higher Tmax and Tmin than other seasons. One of the disadvantages of using satellite data is the presence of cloud cover causing gaps in the datasets, represented by white, as may be seen in ) and (). The accuracy of Tmax and Tmin estimation depends on the quality of the LST product and the satellite passing time. In this study, the Aqua satellite LST product was used, which improves the Tmin estimation compared to other satellites, as its passing time is closer to the time of Tmin.
Figure 4. Spatial distributions of (a) LSTday (K), (b) LSTnight (K), (c) Tmax (°C), (d) Tmin (°C), and (e) Rs (MJ m−2 d−1) on days 36, 89, 175 and 274 of the year 2014, representing different seasons.
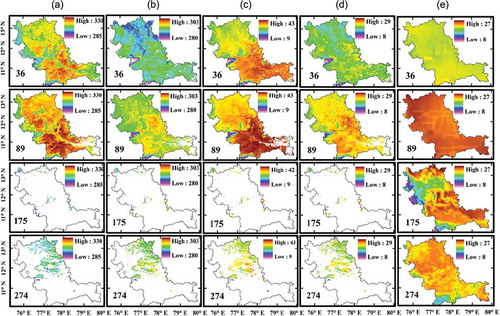
The Tmax was estimated using the TVX approach by varying the maximum values of the three vegetation indices NDVI, EVI and GVMI (Equations (1)–(3)). Statistical analysis was performed between these estimated Tmax values and the observed Tmax, and the results are depicted in . Scatter plots between estimated Tmax using the best maximum vegetation index and observed Tmax for forest (F), croplands (C) and croplands/natural vegetation (C/NV) land cover classes are shown in . Maximum vegetation index values for the considered land cover classes were selected individually depending on the accuracy of Tmax estimation. Accuracy was adjudicated based on RMSE and r, and these were computed for the F, C and C/NV land cover classes by varying the maximum vegetation index values from 0.9 to 0.4, with an interval of 0.05 ().
Figure 5. (1) Scatter plots between predicted Tmax and AWS Tmax; and (2) variations of RMSE and r values obtained between predicted Tmax and AWS Tmax for varying VImax for (a) forest, (b) croplands, and (c) croplands/natural vegetation land cover classes.
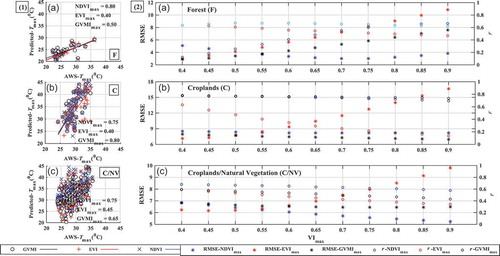
As the NDVImax was reduced for the forest class, RMSE decreased from 3.84 to 3.01°C at NDVImax = 0.8 and, after this, began to increase from 3.12 to 5.10°C. However, r gradually decayed from 0.66 to 0.12 as NDVImax was reduced (). In the case of the croplands class, RMSE showed less variation for different NDVImax values and RMSE was lowest at NDVImax = 0.75, and r values gradually increased from 0.70 to 0.78 as NDVImax was decreased (). For the C/NV class, RMSE and r gradually increased from 5.25 to 6.84°C and 0.58 to 0.68, respectively, and an optimal value of NDVImax = 0.75 was selected (). Thus, NDVImax values of 0.8, 0.75 and 0.75 were selected for F, C and C/NV classes, respectively. At these NDVImax values, r and RMSE between estimated Tmax and observed Tmax were found to be 0.63 and 3.19°C, 0.72 and 8.15°C, and 0.60 and 5.44°C for F, C, C/NV classes, respectively ().
For the EVI, as the EVImax was reduced from 0.9 to 0.4 for the forest class, RMSE decreased from 10.84 to 3.01°C and r increased from 0.47 to 0.64 (). However, for the croplands class, RMSE abruptly decreased from 16.63 to 7.13°C and r increased from 0.14 to 0.63 (). In the case of croplands/natural vegetation, RMSE reduced from 9.81 to 6.22°C and r increased from 0.34 to 0.60 (). Therefore, optimal EVImax values of 0.4, 0.4 and 0.45 were selected for F, C and C/NV classes, respectively. At these values of EVImax, r and RMSE between estimated Tmax and observed Tmax were calculated as 0.63 and 3.47°C, 0.55 and 7.30°C, and 0.57 and 6.14°C for F, C and C/NV classes, respectively ().
As the value of GVMImax was reduced from 0.9 to 0.4, for the forest land cover class, RMSE gradually decreased from 7.60 to 2.85°C and r increased from 0.62 to 0.67 to a level of GVMImax = 0.55, and eventually decreased to 0.60 (). For the croplands class, RMSE and r gradually increased from 6.98 to 8.03°C and from 0.74 to 0.78, respectively (). In the case of croplands/natural vegetation, the improvement in RMSE values, even after gradual reduction in GVMImax, was small, whereas r showed a gradual increase from 0.43 to 0.61 (). Therefore, the optimal values of GVMImax of 0.5, 0.8 and 0.65 were selected for F, C and C/NV classes, respectively. At these values of GVMImax, r and RMSE between estimated Tmax and observed Tmax were calculated as 0.67 and 3.39°C, 0.77 and 7.67°C, and 0.56 and 6.64°C, for F, C and C/NV classes, respectively (). Among the vegetation indices considered, GVMI predicted better Tmax for the F and C land cover classes compared to EVI and traditional NDVI vegetation indices for the study region, but no notable improvement was seen. This indicates that moisture content of vegetation influences Tmax more than the density of vegetation. The GVMI represents vegetation moisture content, whereas EVI and NDVI represent density of vegetation; therefore, GVMI performed better than EVI and NDVI. However, the TVX method yielded poor r with high RMSE values for the considered optical vegetation indices, compared to the ASA. Hence Tmax and Tmin values obtained using the ASA approach were used for the estimation of ETo.
4.2 Validation of satellite-based solar radiation
Solar radiation (Rs) values obtained from the Kalpana-1 satellite were evaluated with the observed data, to assess the potential of their application for the estimation of ETo. Observed Rs data were available only for Berambadi station, located in the croplands land cover class, for the years 2013 and 2014. Therefore, analysis was only performed for the croplands class. Linear regression between the AWS and satellite Rs values generated a slope of 0.68 (RMSE = 2.76 MJ m−2 d−1, r = 0.86) ()). Temporal patterns of the satellite Rs (Sat-Rs) data for both years were analysed; the statistical analysis indicated that Sat-Rs corresponded well with the observed Rs, with RMSE = 2.87 MJ m−2 d−1, r = 0.88 for 2013 and RMSE = 2.63 MJ m−2 d−1, r = 0.79 for 2014, as shown in ). This shows that Sat-Rs can be applied in radiation-based models over the Cauvery basin, as it is mostly covered by croplands. However, underestimation of Sat-Rs was found for the years 2013 and 2014, with relative RMSE values of 18.66 and 15.84, respectively.
Figure 6. (a) Scatter plot between satellite and observed Rs values and (b) temporal variations of satellite and observed Rs for the years 2013 and 2014.
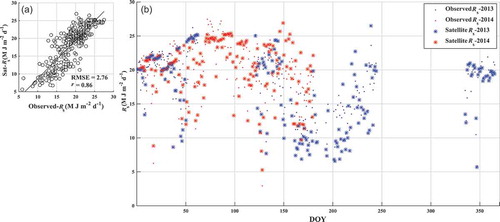
Spatial variation in Sat-Rs for 2014, representing different seasons, is shown in ), illustrating that Rs values are smaller for the winter season (DOY 36), with very small spatial variation, whereas for the summer (DOY 89) they are greater than in other seasons, with less spatial variation for all land cover classes. In the case of the monsoon (DOY 175) and post-monsoon (DOY 274) seasons, Rs values are smaller for the Western Ghats region than for the other regions. During the monsoon season, the upper part of the basin has smaller Rs than the lower part because of the southwest monsoon. In contrast, during the post-monsoon season, the upper part of the basin has higher Rs than the lower part of the basin due to the northeast monsoon ()). During the monsoon and post-monsoon seasons, estimation of Rs is difficult because of the presence of dense clouds.
4.3 Evaluation of ETo models
The considered LST- and Ta-based temperature (SLBE, HS, PMT) and radiation (Makk, Makk_adv) ETo models were compared with the FAO56-PM ETo estimated using AWS data at the point scale. Analysis of the estimated ETo using satellite data was carried out separately by considering different land cover classes of the study region with various climatic conditions.
4.3.1 Statistical evaluation of ETo for different land cover classes
The data for the years 2012, 2013 and 2014 were used to estimate ETo by the temperature- and radiation-based models at 2 km spatial resolution. The ETo values estimated using different datasets were segregated based on MODIS LULC data to assess the ETo for various land cover classes. Based on the availability of satellite and AWS data, the analysis was performed for three land cover classes, namely forest, croplands and croplands/natural vegetation. Scatter plots between LST-based and AWS ETo and between Ta-based and AWS ETo for different land cover classes are presented in and , respectively. Initially, LST was employed in temperature- and radiation-based ETo models instead of Ta, to examine the adoptability of LST data. Thereafter, Tmax and Tmin estimated using the ASA from LSTday and LSTnight with auxiliary variables were applied in the ETo models. The performance of these LST- and Ta-based temperature- and radiation-based ETo models was assessed through the goodness-of-fit indicators RMSE (mm d−1), r, MAPE (%) and MBE (mm d−1) for the considered land cover classes. The statistical results imply that the LST-based SLBE model underestimated ETo for forest, croplands and croplands/natural vegetation classes (F: RMSE = 1.06, r = 0.53, MAPE = 23.98 and MBE = 0.92; C: RMSE = 0.42, r = 0.77, MAPE = 8.42 and MBE = 0.25; and C/NV: RMSE = 1.06, r = 0.73, MAPE = 18.38 and MBE = 0.64) ()). The HS_LST, PMT_LST and Makk_LST models predominantly overestimated ETo for F, C and C/NV land cover classes ()–()). Among the considered LST-based ETo models, SLBE satisfactorily predicted ETo with smaller RMSE and MAPE for the considered land cover classes because (a) aerodynamic and (b) radiation terms in this equation were obtained using local weather station data, and these were applied for the entire basin. The HS_LST and PMT_LST methods depend solely on LST data; hence the ETo values were found to be higher than the FAO56-PM ETo, and this is due to higher LST than Ta over the study region. Nevertheless HS_LST and PMT_LST yielded high r values for the forest class. Statistical error indices showed that the Makk_LST model yielded better ETo values than the HS_LST and PMT_LST models because Rs data were utilized along with LST.
Figure 7. Scatter plots between estimated ETo using different LST-based ETo models from satellite data (Sat-LST-ETo) and ETo values obtained from the FAO56-PM model using observed AWS data (AWS-ETo) for forest, croplands and croplands/natural vegetation.
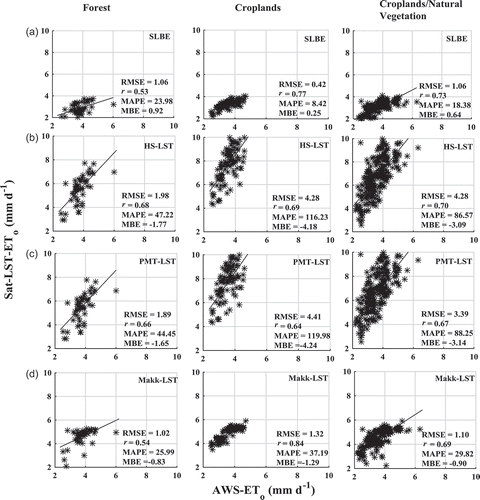
Figure 8. Scatterplots between estimated ETo using different Ta-based ETo models from satellite data (Sat-Ta-ETo) and ETo values obtained from FAO56-PM using observed AWS data (AWS-ETo) for forest, croplands and croplands/natural vegetation.
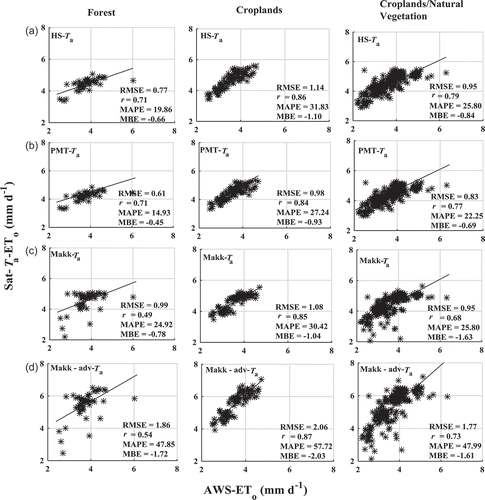
illustrates the goodness-of-fit indicators computed by evaluating Ta-based radiation- and temperature-based ETo models with PM ETo. Compared to LST-based ETo models, Ta-based models, such as HS_Ta, PMT_Ta, Makk_Ta and Makk-adv_Ta models, gave satisfactory ETo values for all the land cover classes with smaller RMSE, MAPE and MBE (). High r values were observed for all land cover classes for all Ta-based ETo models except for the Makk_Ta and Makk-adv_Ta equations for the forest class. This may be due to insufficient weather variables required to estimate ETo. And also Sat_Rs data used in these two equations needed evaluation for the forest class. Tmax and Tmin were estimated using the ASA approach by minimizing errors between LSTday/LSTnight and Tmax/Tmin, which resulted in better performance of the Ta-based ETo models. For the HS_Ta model, RMSE, MAPE and r ranged from, respectively, 0.77, 19.86 and 0.71 for forest to 1.14, 31.83 and 0.86 for croplands, with a mean bias of −0.87 ()). The PMT_Ta model yielded RMSE, MAPE and r values ranging, respectively, from 0.61, 14.93 and 0.71 for forest to 0.98, 27.24 and 0.84 for croplands, with a mean bias of −0.69 ()). In contrast, the Makk_Ta (Makk-adv_Ta) models gave RMSE values ranging from 0.95 (1.77) for C/NV to 1.08 (2.06) for croplands, with r in the range 0.49 (0.54) for forest to 0.85 (0.87) for croplands, and MAPE in the range 24.92 (47.85) for forest to 30.42 (57.72) for croplands, with a mean bias of −1.15 (−1.78) () and ()). Overall, the statistical error indices imply that the PMT_Ta model performed slightly better than other ETo models for all considered land cover classes. However, for the croplands class, the SLBE ranked first and the PMT_Ta model ranked second by more accurately predicting ETo values than other LST- and Ta-based ETo models (). Considering the overall results, the PMT_Ta ETo model performed better, followed by the SLBE, HS_Ta and Makk_Ta, in that order.
Table 4. Ranking of ETo models depending on the selected goodness-of-fit indicators obtained between ETo_LST, and ETo_Ta model-based estimates and FAO56 PM ETo. SLBE: simple LST-based equation; PMT: Penman-Monteith temperature; HS: Hargreaves-Samani; Makk-adv: Makkink-Advection.
4.3.2 Statistical evaluation of ETo for different climatic regions
In order to examine the performance of LST- and Ta-based ETo models, the data for all available stations were grouped into three climatic regions according to ICAR, namely semi-arid, semi-arid to sub-humid, and humid. The climatic characteristic of each station are given in . According to the statistical analysis, for the semi-arid climate the SLBE performed slightly better than the other ETo models (). This is because more of the meteorological stations considered for parameterization of aerodynamic and radiation terms have semi-arid climates, hence better ETo values were estimated. The value of r was higher for the PMT_Ta model than the SLBE, implying that PMT_Ta can also perform better in semi-arid regions, as indicated in . Apart from the SLBE model, the other LST-based ETo models provided poor estimates of ETo, with higher RMSE values. In semi-arid to sub-humid climates, PMT_Ta ranked first, while HS_Ta and Makk_Ta ranked second and third, respectively, and the performance indices indicate satisfactory results of ETo models under semi-arid to sub-humid climate (). Similar results were obtained for humid climates, with smaller RMSE, MAPE and MBE values for the PMT_Ta model, but slightly higher r values for the HS_Ta model than PMT_Ta and other ETo models. In both the semi-arid to sub-humid and humid climates, considering the effect of air humidity, using Tdew instead of Ta and considering the effect of wind speed in PMT_Ta yielded better ETo values. Overall, statistical analysis considering climatic characteristics suggested that the PMT_Ta model would more often estimate ETo better than the other ETo models in comparison with the FAO56-PM ETo estimates, as indicated consistently with smaller RMSE, MAPE and MBE relative to those of the HS_Ta and Makk_Ta models, which ranked second and third, respectively. It is noteworthy that, in all climates, r values for the PMT_Ta model were slightly low compared to the HS_Ta model. Among the LST-based ETo models, the SLBE performed better than the other models. The LST-based HS and PMT models consistently overestimated ETo across all climatic regions relative to the other Ta- and LST-based ETo models.
Table 5. Statistical analysis of LST- and Ta-based ETo models for semi-arid, semi-arid to sub-humid, and humid climates. RMSE (mm d−1): root mean square error; r: Pearson correlation coefficient; MAPE: mean absolute percentage error; MBE: mean bias error. See for model abbreviations. Bold indicates best performing models.
4.3.3 Spatial and temporal analysis of ETo models
illustrates the spatial distribution of estimated ETo using LST- and Ta-based ETo models for various days of the year (DOY: 36, 89, 175 and 274), representing winter, summer, rainy season and post-monsoon season, respectively. All ETo models yielded low ETo over the Western Ghats and high ETo in the croplands and croplands/natural vegetation land cover classes, as shown in . The Western Ghats area is covered with forest, has higher elevation and humid climates and this resulted in lower ETo estimates from the considered ETo models. Most of the C and C/NV land classes are at lower elevations with semi-arid climates and therefore higher ETo was observed for all the ETo models. The LST-based ETo models – HS_LST and PMT_LST – predominantly overestimated ETo for all land cover classes and for all climatic regions, as shown by the statistical analysis. The next two models – Makk_LST and Makk_adv_Ta – consistently overestimated ETo over the study region. The SLBE model slightly underestimated ETo for all land cover classes compared to the other ETo models.
Figure 9. Spatial variation of ETo obtained from the LST- and Ta-based models for days 36, 89, 175 and 274 of the year 2014, representing different seasons.
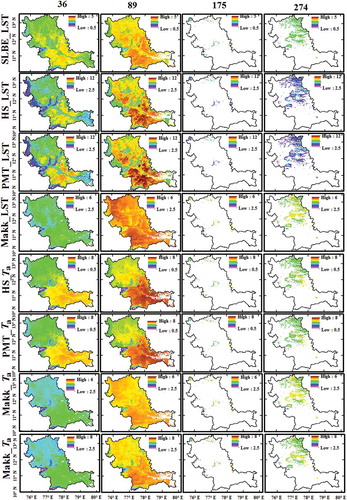
For the summer season, larger ETo estimates were obtained and smaller ETo values were observed for the rainy season for all ETo models. Due to the presence of clouds, thermal sensors failed to provide LST data and hence Tmax, Tmin and ETo values could not be estimated for cloudy pixels, as indicated by white in and . Therefore, for the monsoon and post-monsoon seasons, it was hard to evaluate the spatial distribution of ETo. The ETo obtained for the lower part of the Cauvery basin was higher than that for the upper part and this was observed for all ETo estimates from the considered models (). The ETo values ranged from 0.5 to 5.0 mm d−1 for the SLBE model, being a slight underestimation of observed ETo, whereas, for the Ta-based HS and PMT models, ETo values ranged from 0.5 to 8.0 mm d−1, showing slightly better spatial patterns than the other ETo models. Similarly, estimates by Ta- and LST-based Makkink models ranged from 2.5 to 6.0 mm d−1, showing less spatial variation across the study region.
Furthermore, ETo values estimated using LST- and Ta-based ETo models were temporally evaluated with the FAO56-PM ETo computed using AWS data. Temporal variations in ETo obtained from the ETo models considered are shown in Figure 10 for stations 15F07B and 15F34C, which belong to the C/NV and C land classes, respectively. According to , the PMT_LST and HS_LST models overestimated ETo compared to the Makk_LST and SLBE models for both C and C/NV land cover classes. Among the ETo models considered, PMT_Ta, HS_Ta and Makk_Ta performed better than the other ETo models for croplands (croplands/natural vegetation) land cover classes, with r, RMSE (mm d−1), MAPE and MBE (mm d−1) of 0.89 (0.91), 0.37 (1.03), 8.01 (29.47) and −0.19 (−1.01), and 0.90 (0.92), 0.46 (1.18), 10.25 (33.97) and −0.35 (−1.17), and 0.71 (0.87), 0.60 (1.06), 12.28 (30.12) and −0.32 (−1.03), respectively, as shown in .
Figure 10. Temporal variation of ETo obtained from satellite data and ETo obtained from the FAO56-PM method for stations belonging to croplands/natural vegetation and croplands land cover classes. Gaps in the ETo values are due to non-availability of LST, Rs and AWS data.
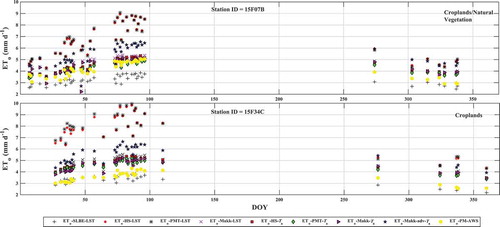
The accuracy of ETo estimation depends on the quality of the input data. Hence, it is necessary to check the quality of input data before application in ETo models. Satellite-based ETo models depend on the quality of LST data. In this study, LST data were used for both Ta and ETo estimation. The use of Ta estimated from satellite data, rather than direct LST data, in the ETo estimations could increase the accuracy of ETo calculation and this has been amply proven in this study. Overall, statistical and spatial analysis showed that the PMT_Ta model gave slightly better accuracy of ETo estimates relative to the HS_Ta, Makk_Ta and SLBE models, and each of these four ETo models performed better than other ETo models considered in this study.
5 Summary and conclusions
In this study, air temperature (Ta) was estimated using remote sensing-based temperature–vegetation index (TVX) and the advanced statistical approach (ASA) and the results were validated with automatic weather station (AWS) Ta data. In the TVX approach, the relationship between different vegetation indices (NDVI, EVI and GVMI) and land surface temperature (LST) was examined by varying the maximum vegetation index values to estimate the maximum Ta (Tmax) for the study region. The GVMI-based TVX approach performed better than the other vegetation index methods for the estimation of Tmax. The ASA was used to estimate both Tmax and Tmin. In this approach, a bootstrap technique was employed to generate calibration and validation samples. The validation samples were used to validate the predicted Tmax and Tmin. This approach showed improvement in the estimation of Tmax compared to the TVX approach, with r, RMSE and NSE values of 0.87, 2.17°C and 0.75, respectively. Further, the ASA estimated Tmin efficiently, with r, RMSE and NSE of 0.87, 2.27°C and 0.76, respectively. The Tmax and Tmin estimates from this approach were then used in the estimation of ETo. Temperature-based models, namely HS, PMT and SLBE, and radiation-based Makkink models were considered for estimation of ETo over the Cauvery basin. The Ta and Rs, being the inputs required for these models, were obtained from satellite data. Initially, LSTday and LSTnight were used in the temperature- and radiation-based models. Thereafter, Tmax and Tmin estimated from LSTday and LSTnight with auxiliary variables were used in the temperature- and radiation-based models for the estimation of ETo. These LST- and Ta-based ETo models were evaluated with reference to the FAO56-PM ETo obtained using observed climatic variables. Statistical analysis implied that the Ta-based PMT model performed better than the other ETo models for various land cover classes and for different climatic conditions with smaller RMSE, MAPE and MBE values. The SLBE and HS_Ta models ranked second and third, respectively. The LST-based HS and PMT models consistently overestimated for all the land cover classes, with higher RMSE, MAPE and MBE values. However, the r values were more or less similar to those of the Ta-based models.
This study has demonstrated the applicability of satellite-based Ta and ETo estimation over an Indian river basin that had not been examined previously. Further comparison of various satellite-based Ta and ETo models was performed in this study. In the TVX approach only NDVI and EVI had been reported in the literature, whereas here the GVMI has been used in the estimation of Tmax, in the absence of weather station data. Therefore, this study will be useful for selecting proper satellite-based ETo and Ta models for water resource management, irrigation scheduling and climate change studies. However, it is very difficult to obtain ETo and Ta values under cloudy conditions, since these depend on LST, because thermal sensors fail to provide LST data under cloudy conditions. This creates gaps in the ETo and Ta values. Future work will include estimation of ETo under cloudy conditions, in order to eliminate the gaps in the satellite-based ETo models.
Acknowledgements
We would like to thank Professor Muddu Shekar (Department of Civil Engineering, Indian Institute of Science, Bangalore) and his team for providing observed solar radiation data for the Berambadi site. We also thank NASA Land Process Distributed Active Archive Center for rendering available MODIS LST, Reflectance and LULC data, and ISRO’s MOSDAC for the supply of AWS data.
Disclosure statement
No potential conflict of interest was reported by the authors.
References
- Aguilar, C. and Polo, M.J., 2011. Generating reference evapotranspiration surfaces from the Hargreaves equation at watershed scale. Hydrology and Earth System Sciences, 15, 2495–2508. doi:10.5194/hess-15-2495-2011
- Allen, R.G., et al., 1998. Crop Evapotranspiration: guidelines for computing crop water requirements. Rome: Italy: Food and Agriculture Organization of the United Nations, Irrigation and Drainage Paper 56. https://appgeodb.nancy.inra.fr/biljou/pdf/Allen_FAO1998.pdf
- Almorox, J., Quej, V.H., and Marti, P., 2015. Global performance ranking of temperature based approaches for evapotranspiration estimation considering koppen climate classes. Journal of Hydrology, 528, 514–522. doi:10.1016/j.jhydrol.2015.06.057
- Arellano, M.G., and Irmak, S., 2016. Reference (potential) evapotranspiration. 1: comparison of temperature, radiation and combination-based energy balance equations in humid, subhumid, arid, semiarid and mediterranean type climates. Journal of Irrigation and Drainage Engineering, 142 (4), 04015065. doi: 10.1061/(ASCE)IR.1943-4774.0000978
- Bai, H. and Wei., P., 2008. Resampling methods revisited: advancing the understanding and applications in educational research. International Journal of Research and Method in Education, 31 (1), 45–62. doi:10.1080/17437270801919909
- Benali., A., et al., 2012. Estimating air surface temperature in Portugal using MODIS LST data. Remote Sensing of Environment, 124, 108–121. doi:10.1016/j.rse.2012.04.024
- Blaney, H.F. and Criddle, W.D., 1962. Determining consumptive use and irrigation water requirements. Beltsville, MD: US department of agriculture. US Department of Agriculture Technical Bulletin, 1275, 1–59.
- Bois, B., et al., 2008. Using remotely sensed solar radiation data for reference evapotranspiration estimation at a daily time step. Agricultural and Forest Meteorology, 148, 619–630. doi:10.1016/j.agrformet.2007.11.005
- Cammalleri, C. and Ciraolo, G., 2013. A simple method to directly retrieve reference evapotranspiration from geostationary satellite images. International Journal of Applied Earth Observation and Geoinformation, 21, 149–158. doi:10.1016/j.jag.2012.08.008
- Cristobal, J., Ninyerola, M., and Pons, X., 2008. Modeling air temperature through a combination of remote sensing and GIS data. Journal of Geophysical Research, 113, D13106. doi:10.1029/2007JD009318
- Cruz-Blanco, M., et al., 2014a. Assessment of reference evapotranspiration using remote sensing and forecasting tools under semi-arid conditions. International Journal of Applied Earth Observation and Geoinformation, 33, 280–289. doi:10.1016/j.jag.2014.06.008
- Cruz-Blanco, M., et al., 2015. Uncertainty in estimating reference evapotranspiration using remotely sensed and forecasted weather data under the climatic conditions of Southern Spain. International Journal of Climatology, 35, 3371–3384. doi:10.1002/joc.4215
- Cruz-Blanco, M., Lorite, I.J., and Santos, C., 2014b. An innovative remote sensing based reference evapotranspiration method to support irrigation water management under semi-arid conditions. Agricultural Water Management, 131, 135–145. doi:10.1016/j.agwat.2013.09.017
- CWC and NRSC (Central Water Commission and National Remote Sensing Centre), 2014. Cauvery Basin report. [online]. Source. Available from: www.india-wris.nrsc.gov.in [Accessed 17 November 2016].
- De Bruin, H.A.R., 1987. From penman to makkink. In: J.C. Hooghart, Ed., Proceedings and Information: TNO Committee on Hydrological Research No. 39. The Netherlands Organization for Applied Scientific Research, Den Haag: TNO, 39, 5–31.
- De Bruin, H.A.R., et al., 2010. Reference crop evapotranspiration derived from geo-stationary satellite imagery: a case study for the forgea flood plain, NW-Ethiopia and the Jordan valley, Jordan. Hydrology and Earth System. Sciences, 14, 2219–2228. doi:10.5194/hess-14-2219-2010
- De Bruin, H.A.R., et al., 2012b. Reference crop evapotranspiration estimated from geostationary satellite imagery. In: Remote Sensing and Hydrology (Proceedings of a symposium held at Jackson Hole, Wyoming, USA, September 2010), Wallingford, UK: International Association of Hydrological Sciences, IAHS Publ. 352, 111–114.
- Di Stefano, C. and Ferro, V., 1997. Estimation of evapotranspiration by Hargreaves formula and remotely sensed data in semi arid Mediterranean areas. Journal of Agricultural Engineering Research, 68 (3), 189–199. doi:10.1006/jaer.1997.0166
- Douglas, M.E., et al., 2009. A comparison of models for estimating potential evapotranspiration for Florida land cover types. Journal of Hydrology, 373, 366–376. doi:10.1016/j.jhydrol.2009.04.029
- Dwyer, J. and Schmidt, G., 2006. The MODIS Reprojection Tool. In: J.J. Qu, et al., eds. Earth Science Satellite Remote Sensing. Berlin, Heidelberg: Springer.
- Fotios, X. and Matzarakis, A., 2011. Evaluation of 13 empirical reference potential evapotranspiration equations on Island of crete in Southern Greece. Journal of Irrigation and Drainage Engineering, 137 (4), 211–222. doi:10.1061/(ASCE)IR.1943-4774.0000283
- Friedl, M.A., et al., 2010. MODIS Collection 5 global land cover: algorithm refinements and characterization of new datasets. Remote Sensing of Environment, 114 (1), 168–182. doi:10.1016/j.rse.2009.08.016
- Fu, G., et al., 2011. Estimating air temperature of an alpine meadow on the Northern Tibetan Plateau using MODIS land surface temperature. Acta Ecologica Sinica, 31, 8–13. doi:10.1016/j.chnaes.2010.11.002
- Gallo, K., et al., 2011. Evaluation of relationship between air and land surface temperature under clear and cloudy conditions. Journal of Applied Meteorological Climatology, 50, 767–775. doi:10.1175/2010JAMC2460.1
- Gavilan, P., et al., 2006. Regional calibration of Hargreaves equation for estimating reference ET in a semiarid environment. Agricultural Water Management, 81, 257–281. doi:10.1016/j.agwat.2005.05.001
- Ghosh, S.P., 1991. Agro-c1imatic zone specific research India perspective under NARP. ICAR, 539 (NewDelhi), 550.
- Guerschman, J.P., et al., 2009. Scaling of potential evapotranspiration with MODIS data reproduces flux observations and catchment water balance observations across Australia. Journal of Hydrology, 369, 107–119. doi:10.1016/j.jhydrol.2009.02.013
- Hamon, W.R., 1961. Estimating potential evapotranspiration. Journal of Hydraulics Division, Proceedings of the American Society of Civil Engineers, 871, 107–120.
- Hargreaves, G.H. and Samani, Z.A., 1985. Reference crop evapotranspiration from temperature. Transaction of ASAE, 1 (2), 96–99.
- Kisi, O., 2014. Comparison of different empirical methods for estimating daily reference evapotranspiration in Mediterranean climate. Journal of Irrigation and Drainage Engineering, 140 (1), 04013002. doi:10.1061/(ASCE)IR.1943-4774.0000664
- Le Naire, G., et al., 2006. Forest leaf area index determination: a multiyear satellite-independent method based on within sand normalized difference vegetation index spatial variability. Journal of Geophysical Research, 111, G02027.
- Lin, S., et al., 2012. Evaluation of estimating daily maximum and minimum air temperature with MODIS data in east Africa. International Journal of Applied Earth Observation and Geoinformation, 18, 128–140. doi:10.1016/j.jag.2012.01.004
- Maeda, E.E., Wiberg, D.A., and Pellikka, P.K.E., 2011. Estimating reference evapotranspiration using remote sensing and empirical models in a region with limited ground data availability in Kenya. Applied Geography, 31, 251–258. doi:10.1016/j.apgeog.2010.05.011
- McCloud, D.E., 1955. Water requirements of field crops in Florida as influenced by climate. Proceedings Soil and Crop Science Society of Florida, 15, 165–172.
- McMahon, T.A., et al., 2013. Estimating actual, potential, reference crop and pan evaporation using standard meteorological data: a pragmatic synthesis. Hydrology and Earth System Sciences, 17, 1331–1363. doi:10.5194/hess-17-1331-2013
- Moriasi, D.N., et al., 2007. Model evaluation guidelines for systematic quantification of accuracy in watershed simulations. Transactions of the American Society of Agricultural and Biological Engineers, 50 (3), 885–900.
- Mostovoy, V.G., et al., 2006. Statistical estimation of daily maximum and minimum air temperatures from MODIS LST data over the state of Mississippi. GIScience & Remote Sensing, 43, 78–110. doi:10.2747/1548-1603.43.1.78
- Nandagiri, L. and Kovoor, M.G., 2006. Performance evaluation of reference evapotranspiration equations across a range of Indian climates. Journal of Irrigation and Drainage Engineering, 132 (3), 238–249. doi:10.1061/(ASCE)0733-9437(2006)132:3(238)
- Nash, J.E. and Sutcliffe, J.V., 1970. River flow forecasting through conceptual models part I — A discussion of principles. Journal of Hydrology, 10 (3), 282–290. doi:10.1016/0022-1694(70)90255-6
- Nieto, H., et al., 2011. Air temperature estimation with MSG-SEVIRI data: calibration and validation of the TVX algorithm for the Iberian Peninsula. Remote Sensing of Environment, 115, 107–116. doi:10.1016/j.rse.2010.08.010
- Pereira, S.L., et al., 2015. Crop evapotranspiration estimation with FAO56: pastand future. Agricultural Water Management, 147, 4–20. doi:10.1016/j.agwat.2014.07.031
- Prihodko, L. and Goward, S.N., 1997. Estimation of air temperature from remotely sensed surface observations. Remote Sensing of Environment, 60, 335–346. doi:10.1016/S0034-4257(96)00216-7
- Raziei, T. and Pereira, L.S., 2013a. Estimation of ETo with Hargreaves-samani and FAO-PM temperature methods for a wide range of climates in Iran. Agricultural Water Management, 121, 1–18. doi:10.1016/j.agwat.2012.12.019
- Raziei, T. and Pereira, L.S., 2013b. Spatial variability of reference evapotranspiration in Iran utilizing fine resolution gridded datasets. Agricultural Water Management, 126, 104–118. doi:10.1016/j.agwat.2013.05.003
- Rhee, J. and Jungho, I., 2014. Estimating high spatial resolution air temperature for regions with limited in situ data using MODIS products. Remote Sensing, 6, 7360–7378. doi:10.3390/rs6087360
- Rivas, R. and Caselles, V., 2004. A simplified equation to estimate spatial reference evaporation from remote sensing based surface temperature and local meteorological data. Remote Sensing of Environment, 93, 68–76. doi:10.1016/j.rse.2004.06.021
- Schuttemeyer, D., et al., 2007. Satellite based actual evapotranspiration over drying semiarid terrain in West Africa. Journal of Applied Meteorological Climate, 46, 97–111. doi:10.1175/JAM2444.1
- Senatore, A., et al., 2015. Regional scale modeling of reference evapotranspiration: intercomparison of two simplified temperature and radiation based approaches. Journal of Irrigation and Drainage Engineering, 141 (12), 04015022. doi:10.1061/(ASCE)IR.1943-4774.0000917
- Shah, D.B., et al., 2013. Estimating minimum and maximum air temperature using MODIS data over Indo-Gangetic Plain. Journal of Earth System Sciences, 122, 1593–1605. doi:10.1007/s12040-013-0369-9
- Silva, D., Meza, F.J., and Varas, E., 2010. Estimating reference evapotranspiration (ETo) using numerical weather forecast data in central Chile. Journal of Hydrology, 382, 64–71. doi:10.1016/j.jhydrol.2009.12.018
- Stewart, J.B., et al., 1999. Use of satellite data to estimate radiation and evaporation for north-west Mexico. Agricultural Water Management, 38, 181–193. doi:10.1016/S0378-3774(98)00068-7
- Stisen, S., et al., 2007. Estimation of diurnal air temperature using MSG SEVIRI data in West Africa. Remote Sensing of Environment, 110, 262–274. doi:10.1016/j.rse.2007.02.025
- Thornthwaite, C.W., 1945. Discussion of evaporation from a free water surface. Proceedings of American Society of Civil Engineering, 71 (3), 343–348.
- Todorovic., B.K. and Pereira, L.S., 2013. Reference evapotranspiration estimate with limited weather data across a range of Mediterranean climates. Journal of Hydrology, 481, 166–176. doi:10.1016/j.jhydrol.2012.12.034
- Valipour, M., 2012. Ability of box-jenkins models to estimate of reference potential evapotranspiration (A case study: mehrabad synoptic station, Tehran, Iran). IOSR Journal of Agriculture and Veterinary Science, 1 (5), 01–11. doi:10.9790/2380
- Valipour, M., 2014. Application of new mass transfer formulae for computation of evapotranspiration. Journal of Applied Water Engineering and Research, 2 (1), 33–46. doi:10.1080/23249676.2014.923790
- Valipour, M., 2015a. Importance of solar radiation, temperature, relative humidity, and wind speed for the calculation of reference evapotranspiration. Archives of Agronomy and Soil Science, 61 (2), 239–255. doi:10.1080/03650340.2014.925107
- Valipour, M., 2015b. Study of different climatic conditions to assess the role of solar radiation in reference crop evapotranspiration equations. Archives of Agronomy and Soil Science, 61 (5), 679–694. doi:10.1080/03650340.2014.941823
- Valipour, M., 2017. Analysis of potential evapotranspiration using limited weather data. Applied Water Science, 7 (1), 187–197. doi:10.1007/s13201-014-0234-2
- Valipour, M., Sefidkouhi, M.A.G., and Raeini-Sarjaz, M., 2017. Selecting the best model to estimate potential evapotranspiration with respect to climate change and magnitudes of extreme events. Agricultural Water Management, 180, 50–60. doi:10.1016/j.agwat.2016.08.025
- Vancutsem, C., et al., 2010. Evaluation of MODIS land surface temperature data to estimate air temperature in different ecosystems over Africa. Remote Sensing of Environment, 114, 449–465. doi:10.1016/j.rse.2009.10.002
- Wenbin, Z., Aifeng, L., and Shaofeng, J., 2013. Estimation of daily maximum and minimum air temperature using MODIS land surface temperature products. Remote Sensing of Environment, 130, 62–73. doi:10.1016/j.rse.2012.10.034
- Xu, Y., Knudby, A., and Ho, H.C., 2014. Estimating daily maximum air temperature from MODIS in British Columbia, Canada. International Journal of Remote Sensing, 35 (24), 8108–8121. doi:10.1080/01431161.2014.978957
- Zaksek, K. and Schroedter-Homscheidt, M., 2009. Parameterization of air temperature in high temporal and spatial resolution from a combination of the SEVIRI and MODIS instruments. ISPRS Journal of Photogrammetry and Remote Sensing, 64, 414–421. doi:10.1016/j.isprsjprs.2009.02.006
- Zeng, L., et al., 2015. Estimation of daily air temperature based on MODIS land surface temperature products over the corn belt in the US. Remote Sensing, 7, 951–970. doi:10.3390/rs70100951
Appendix 1
Estimation of ETo using the Penman-Monteith temperature (PMT) model
The procedure given by Todorovic and Pereira (Citation2013) was followed to estimate ETo using the PMT model. The variables required in FAO56-PM equation were calculated using only temperature (LST/Ta) as input, as detailed below.
Calculation of net radiation, actual and saturation vapour pressure using satellite data
where Rns is the net shortwave radiation (MJ m−2 d−1), Rnl is the net longwave radiation (MJ m−2 d−1), and Rn is the net radiation (MJ m−2 d−1).
The Rns results from the balance between incoming and reflected solar radiation and is given by:
where α is the albedo or canopy reflection coefficient, assumed as 0.23 for grass canopy cover, and Rs is solar radiation, which can be expressed as:
where kRs is the empirical radiation adjustment coefficient (°C−0.5), considered as 0.16 for the interior and as 0.19 for coastal regions (Allen et al. Citation1998); Tmax is the maximum air temperature, and Tmin is the minimum air temperature.
The Rnl results from the balance between the downwelling longwave radiation from the atmosphere and outgoing longwave radiation from the vegetation and the soil, and is given by:
where is the net emissivity of the surface, and f is a cloudiness factor, which represents the ratio between actual net longwave radiation and the net longwave radiation for a clear sky day, and is expressed as:
where Rso is the shortwave radiation for a clear sky day (MJ m−2 d−1). The coefficients ac ≈ 1.35 and bc ≈ −0.35 are recommended for average climatic conditions. Rso can be expressed as:
where Z is the elevation (m a.s.l.), and Ra is extraterrestrial radiation (MJ m−2 d−1). The net emissivity of the surface, (Equation (A4)), is given by:
where ea is the actual vapour pressure (kPa):
where is the saturation vapour pressure (kPa);
is saturation vapour pressure at the mean daily maximum air temperature (Tmax) or maximum land surface temperature (LSTmax) (kPa), and
is saturation vapour pressure at the mean daily minimum air temperature (Tmin) or minimum land surface temperature (LSTmin) (kPa).
Appendix 2
The performance indices used to measure goodness-of-fit of the models are calculated as follows:
where xi is the observed value, is the mean of the observed value, yi is the estimated value, and n is the number of observations.
Appendix 3
The coefficients of the fitted models (1–8, ) using the advanced statistical approach (ASA) to estimate Tmax and Tmin are given in and below.
Table A1. Coefficients of the fitted models to estimate Tmax.
Table A2. Coefficients of the fitted models to estimate Tmin.