ABSTRACT
Dynamic sitting, such as fidgeting and desk work, might be associated with health, but remains difficult to identify out of accelerometry data. We examined, in a laboratory study, whether dynamic sitting can be identified out of triaxial activity counts. Among 18 participants (56% men, 27.3 ± 6.5 years), up to 236 counts per minute were recorded in the anteroposterior and mediolateral axes during dynamic sitting using a hip-worn accelerometer. Subsequently, we examined in 621 participants (38% men, 80.0 ± 4.7 years) from the AGES-Reykjavik Study whether dynamic sitting was associated with cardio-metabolic health. Compared to participants who recorded the fewest dynamic sitting minutes (Q1), those with more dynamic sitting minutes had a lower BMI (Q2 = −1.39 (95%CI = −2.33;–0.46); Q3 = −1.87 (−2.82;–0.92); Q4 = −3.38 (−4.32;–2.45)), a smaller waist circumference (Q2 = −2.95 (−5.44;–0.46); Q3 = −3.47 (−6.01;–0.93); Q4 = −8.21 (−10.72;–5.71)), and a lower odds for the metabolic syndrome (Q2 = 0.74 [0.45;1.20] Q3 = 0.58 [0.36;0.95]; Q4 = 0.36 [0.22;0.59]). Our findings suggest that dynamic sitting might be identified using accelerometry and that this behaviour was associated with health. This might be important given the large amounts of time people spend sitting. Future studies with a focus on validation, causation and physiological pathways are needed to further examine the possible relevance of dynamic sitting.
Introduction
Since several years using hip-worn accelerometers for measuring daily activity has become commonplace. Although this provides accurate insight in total (in)activity levels, (Atkin et al., Citation2012; Butte, Ekelund, & Westerterp, Citation2012; Healy et al., Citation2012; Matthews et al., Citation2018) some specific activities remain difficult to identify out of activity counts. In particular activities that are executed with the upper body while being in a sedentary or sitting position. A well-known example is weight training, but it also includes commonly executed daily activities such as desk work or fidgeting. To classify these fidgeting-like types of behaviour, different terms are being used. Trembley et al. define “active sitting”, as “any waking activity in a sitting posture characterized by an energy expenditure >1.5 METs” (Tremblay et al., Citation2017). Also, the term “dynamic sitting” has been used for behaviour that transforms sitting into (more) active behaviour (CitationKoepp, Moore, & Levine, Citation2016, 2017).
To identify this “dynamic sitting” out of accelerometry data, a more comprehensive analysis of activity counts is needed. Usually, only activity counts of the vertical (up-and-down) direction are used to identify daily activity levels. However, triaxial accelerometers measure activity counts in three directions. Next to the vertical direction, these include the anteroposterior (back-to-front) and mediolateral (side-to-side) directions (Butte et al., Citation2012). When combining data of all three directions, identifying dynamic sitting might be possible. To examine this hypothesis, we performed a laboratory study in a sample of healthy adults using a triaxial accelerometer that was placed on the hip. We then analysed whether and to what extent activity counts were recorded in the anteroposterior and mediolateral axes during common sedentary activities.
When dynamic sitting can be identified out of triaxial activity counts, the study of sedentary behaviour and its health effects might be improved. It enables researchers to distinguish between individuals who show dynamic sitting and those who sit completely still, which may be relevant for health. Hypothetically, dynamic sitting increases energy expenditure above resting values which might be associated with more favourable health outcomes. To study the relevance of dynamic sitting for health, we examined associations of dynamic sitting with cardio-metabolic outcomes and the metabolic syndrome in a subsample of the Age, Gene/Environment Susceptibility (AGES)-Reykjavik Study.
Methods
Study populations
Laboratory study
A total of 18 healthy participants who were employees of Maastricht University or Maastricht University Medical Centre in the Netherlands and were aged between 18 and 48 years, were included. Participants with serious mobility limitations, defined as not able to walk, were excluded from participation. This study was approved by the Local Ethics Committee of Maastricht University Medical Centre. All participants gave written informed consent.
Age, gene/environment susceptibility (AGES)-Reykjavik study
The AGES-Reykjavik Study originates from the Reykjavik Study, which was started in 1967 and comprised a random sample of 30,795 participants born in 1907–1935 and residing in Reykjavik, Iceland. Measurements for the AGES-Reykjavik Study took place in 2002–2006 and in 2007–2011 (follow-up) among 5,764 and 3,411 participants, respectively. A full description of the AGES-Reykjavik Study can be found elsewhere (Harris et al., Citation2007). A subsample (658 participants without severe cognitive dysfunction (Mini Mental State Examination ≥ 20)) was asked to wear an accelerometer for seven consecutive days (Arnardottir et al., Citation2013). After excluding participants who had failed to record at least one valid day (at least 10h of monitoring) (n = 15) or who had missing data (n = 22), a total of 621 participants were included in the current analyses. All participants gave written informed consent. The AGES-Reykjavik Study was approved by the institutional review boards of the National Institute on Aging, the National Bioethics Committee (VSN: 00–063) of Iceland and the Data Protection Authority of Iceland.
Measurements
Laboratory study
Protocol
Participants were seated on a chair without armrests and wearing a triaxial ActiGraph accelerometer (GT3X, Fort Walton Beach, FL, USA) on the right hip. The protocol consisted of two imposed sitting activities (imposed sitting (5 min) and imposed fidgeting (4 min)) to determine whether the accelerometer records activity in the three axes as expected, and seven common sedentary activities (natural sitting (5 min), watching TV, reading, writing, typing, playing cards, and preparing food (all 7 min); see Supplemental Table 1). In-between the activities participants were asked to stand up three times. The total duration of the protocol was 56 minutes. The protocol was explained and directly observed by a trained research assistant. Before the protocol started weight, height, waist and hip circumference were measured.
Activity counts
The ActiGraph recorded activity on the vertical (V), anteroposterior (AP), and mediolateral (ML) axes. Data of all axes were uploaded using the ActiLife® 6.6.1 software and activity counts were averaged per minute. Each minute was categorized as a sedentary minute (<100 counts in the V, AP and ML axes), a dynamic sitting minute (<100 counts in the V axis and ≥100 counts in the AP and/or ML axes) or an active minute (≥100 counts in all axes).
Anthropometry
Body mass index (BMI) was calculated using height and weight data (kg/m2) which were measured without shoes and wearing light clothing using a scale and stadiometer to the nearest 0.5 kg or 0.1 cm (Seca, Hamburg, Germany). Circumferences of the waist and hip were measured with a flexible plastic tape measure (Seca, Hamburg, Germany), between the lower rib margin and the iliac crest at the end of expiration, and at the level of the widest circumference over the greater trochanter, both to the nearest 0.5 cm.
AGES-Reykjavik study
Cardio-metabolic outcomes
BMI was calculated using height and weight data (kg/m2) which were collected using standardized techniques. Waist circumference was measured twice using a tape that measures around the body covering the navel, while participants were standing. HDL cholesterol, triglycerides, plasma glucose levels, and C-reactive protein were measured in fasting blood samples and analysed on a Hitachi 912, using reagents from Roche Diagnostics. Triglycerides, fasting glucose and C-reactive were transformed using natural logarithm, due to a skewed distribution. Systolic and diastolic blood pressure were measured twice using a mercury sphygmomanometer. The metabolic syndrome was defined according to the ATPIII guidelines by the presence of 3 or more of the following criteria: (1) waist circumference ≥102 cm for men and ≥88 cm for women; (2) serum triglyceride level ≥1.7 mmol/L; (3) HDL cholesterol level <1.03 mmol/L for men and <1.3 mmol/L for women; (4) fasting glucose level ≥5.6 mmol/L or use of antidiabetic medications (insulin or oral agents); or (5) systolic blood pressure ≥130 mmHg and/or diastolic blood pressure ≥85 mmHg, and/or use of antihypertensive medications (Grundy et al., Citation2005).
Accelerometry data
Activity counts were recorded using the triaxial ActiGraph (GT3X, Ft. Walton Beach, FL, USA), which was attached to a belt and was worn on the right hip (Arnardottir et al., Citation2013). Participants were instructed to wear the accelerometer for seven consecutive days and only remove the monitor before going to bed and during showering, bathing, swimming and other water-related activities.
Raw accelerometry data were processed and averaged per minute using customized software written in MATLAB R2006a (The MathWorks, Inc.; Natick MA, USA). Non-wear time was defined as any interval ≥60 consecutive minutes of zero counts in all three axes, allowing a period of up to 2 minutes of 1–100 counts in the vertical axis. A valid day was defined as at least 10 hour of monitoring. Sedentary time was defined as <100 counts per minute (cpm) in the vertical axis; dynamic sitting as <100 cpm in the V axis and ≥100 cpm in the AP and/or ML axes. The measure used was the number of dynamic sitting minutes per day.
Covariates
Sex, age, level of education, smoking status, alcohol consumption, health status, mobility limitation and medication use were extracted from questionnaires. Level of education was categorized into college/university, secondary, and primary, and smoking status into never smoker, previous smoker, and current smoker. The intake of alcohol in grams/week was used for the alcohol consumption covariate. Health status was defined as having one or more of the following conditions: heart disease, transient ischemic attack (TIA), stroke, or type 1 or type 2 diabetes mellitus. Mobility limitation was defined as having difficulty walking 500 m or climbing 10 steps without resting. Medication use was dichotomized (yes/no) for the use of antidiabetic and antihypertensive medication. Daily minutes of moderate to vigorous physical activity (MVPA≥2020 cpm) were derived from the accelerometry data.
Statistical analyses
All analyses were conducted with IBM SPSS Statistics 22.0 (IBM Corp. Armonk, NY, USA).
Laboratory study
For each activity the average percentages sedentary, dynamic sitting, and active minutes were calculated (minutes divided by activity duration). The number of participants with one or more dynamic sitting minutes was counted. Next, for each activity the average counts per minute of each axis were described with the range, mean and standard deviation (SD), and median and interquartile range. Other data (sex, age, BMI, waist and hip circumference) were summarized and described as mean (SD) or numbers and percentages.
AGES-Reykjavik study
The exposure variable (minutes of dynamic sitting) was split into sex-specific quartiles because the associations were not linear and to obtain equal distributions of men and women (60 men and 95 women in each quartile). The ranges of the quartiles were: 48.0–112.7, 103.4–145.3, 129.4–177.4, and 161.3–330.9 min/day. Descriptive characteristics and accelerometry measures were summarized as mean with standard deviations (SD), as number and percentages or as median with the interquartile range. Chi-square tests, analysis of variance (ANOVA) and Kruskal-Wallis tests were conducted as appropriate to examine differences between the sex-specific quartiles of dynamic sitting. Linear regression analysis was used to examine whether dynamic sitting was associated with the cardio-metabolic outcomes. All analyses were adjusted for daily minutes of sedentary time (models 1). In models 2 the analyses were additionally adjusted for sex, age, level of education, and daily minutes of MVPA. Analyses were further adjusted for smoking status, alcohol consumption, health status (the metabolic syndrome was not adjusted for diabetes mellitus), and mobility limitation (models 3). In models 2 the analyses for the outcomes of HDL cholesterol, triglycerides, and C-reactive protein were also adjusted for BMI; fasting glucose was also adjusted for BMI and antidiabetic medication; and blood pressure was also adjusted for BMI and antihypertensive medication. Results are reported as unstandardized coefficients (B), back-transformed from the natural log scale for the non-normally distributed outcomes, and odds ratios (OR) for the metabolic syndrome. Interaction terms of the dynamic sitting quartiles with sex were not statistically significant (P > 0.10).
Results
Laboratory study
The 18 participants (10 men (55.6%)) had an average age of 27.3 ± 6.5 years. Men had on average a BMI of 22.6 ± 3.2 kg/m2, a waist circumference of 80.7 ± 8.5 cm, and a hip circumference of 86.8 ± 8.7 cm. Among women these measures were 23.1 ± 3.2 kg/m2, 72.4 ± 7.0 cm, and 81.4 ± 6.7 cm, respectively.
shows the percentages dynamic sitting, sedentary, and active minutes for each activity, as well as the number of participants who recorded dynamic sitting. During the activity “imposed sitting” the accelerometer recorded, as expected, predominantly sedentary minutes. In two participants the accelerometer recorded dynamic sitting. The activity “imposed fidgeting” resulted in dynamic sitting (12 participants), but more than 50% of time was recorded as active minutes (>100 counts in all axes). The other activities resulted predominantly in sedentary minutes. However, during the activities “watching TV”, “playing cards”, and “preparing food” dynamic sitting was recorded by 10 to 12 participants.
Figure 1. Percentage dynamic sitting, sedentary, and active minutes of total activity time. In brackets the number of participants who recorded dynamic sitting.
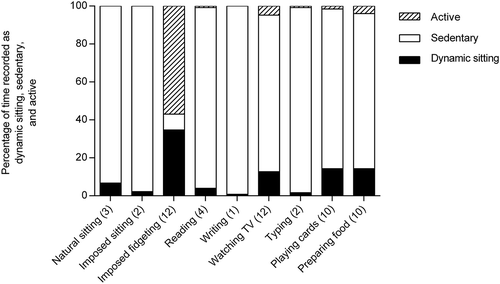
shows descriptive characteristics of the average counts per minute (cpm) per activity for each axis. The maximum average cpm in the V axis was for all activities except “imposed fidgeting” <100 cpm, while this was >100 cpm in the AP or ML axes for the activities “natural sitting”, “watching TV”, “playing cards”, “preparing food”, and “imposed fidgeting”. The mean and median of the average cpm were for all activities in all axes <100 cpm, except for the activity “imposed fidgeting” which showed in all axes a mean and median >100 cpm.
Table 1. Descriptive characteristics of the average counts per minute in each axis for each activity.
AGES-Reykjavik study
The 621 participants (239 men (38%)) had an average age of 80.0 ± 4.7 years. Almost 50% of this sample (49.9%) provided seven valid days of accelerometry data (>10 h of monitoring/day), with 27.9% providing six days, 10.0% five days, 4.0% four days and 4.3% three days. Participants recorded on average 2.4 ± 0.8 h/day as dynamic sitting. Descriptive characteristics of the study sample according to the quartiles of dynamic sitting are presented in . When expressed as percentages of sitting time, 15%, 21%, 25% and 33% of sitting time was dynamic sitting in the respective quartiles. Participants who recorded more dynamic sitting were less likely to be obese and less often had limited mobility. They also had a lower BMI, a smaller waist circumference, a higher HDL cholesterol level, and lower levels of triglycerides, fasting glucose, and C-reactive protein (all P < 0.05). Systolic and diastolic blood pressure were not statistically significantly different between the quartiles. The metabolic syndrome was present in 51% of the participants who recorded the fewest dynamic sitting minutes (quartile 1). This percentage was lower for those who recorded more dynamic sitting; 43.5% (quartile 2), 38.8% (quartile 3), and 27.8% (quartile 4).
Table 2. Characteristics of the AGES-Reykjavik study sample.
shows that compared to participants who recorded the fewest dynamic sitting minutes (quartile 1), those with more dynamic sitting (quartiles 2, 3, 4) had a up to 3.4 kg/m2 lower BMI (B2 = −1.39 (95%CI = −2.33; −0.46); B3 = –1.87 (−2.82; −0.92); B4 = −3.38 (−4.32; −2.45)) and a up to 8.2 cm smaller waist circumference (B2 = −2.95 (−5.44; −0.46); B3 = −3.47 (−6.01; −0.93); B4 = −8.21 (−10.72; −5.71)), after adjustment for sedentary time, MVPA, demographic factors and health factors (models 3). Having more dynamic sitting (quartile 4) was in models 3 also associated with a higher HDL cholesterol level (B4 = 0.13 (0.04; 0.22)), a lower triglycerides level (B4 = 0.91 times lower level; (0.84; 0.99); back-transformed from log scale), and a higher systolic blood pressure (B4 = 5.10 (0.12; 10.07)). Lastly, participants who recorded more dynamic sitting had a 42 to 64% lower odds for the metabolic syndrome in model 3 compared to participants with the fewest dynamic sitting minutes (ORQ3 = 0.58 [0.36 to 0.95]; ORQ4 = 0.36 [0.22 to 0.59]).
Table 3. Associations between dynamic sitting and cardio-metabolic outcomes.
In additional analyses the associations between dynamic sitting minutes and all outcomes were further adjusted for wear time and also for the number of sedentary breaks (defined as each minute in which ≥100 counts were recorded in the V axis and which was preceded by at least 1 minute of <100 counts). This resulted in similar associations (data not shown).
Discussion
Since several years accelerometry has become a widely used tool for measuring physical activity and sedentary behaviour. Using activity counts to identify different activity levels, from sitting to vigorous activity, provides often an accurate insight in daily activity levels (Aguilar-Farías, Brown, & Peeters, Citation2014; Carr & Mahar, Citation2012; Crouter, DellaValle, Haas, Frongillo, & Bassett, Citation2013; Godfrey, Culhane, & Lyons, Citation2007; Koster et al., Citation2016; Kozey-Keadle, Libertine, Lyden, Staudenmayer, & Freedson, Citation2011). Nevertheless, the field of accelerometry research is relatively young and should be moved forward to further increase its value for public health and clinical practice.
One of the research opportunities is improving identification of common daily activities which include upper body movement while sitting or being in a sedentary position. Some examples include fidgeting, desk work and playing cards. This behaviour can be classified as “active sitting” if the energy expenditure exceeds 1.5 METs (Tremblay et al., Citation2017). Another term that has been used is “dynamic sitting”, which refers to behaviour that transforms sitting into (more) active behaviour (CitationKoepp et al., Citation2016, 2017). In our study we used the term dynamic sitting, because energy expenditure was not measured, and this behaviour was characterized by movement of the upper body in the back-to-front (anteroposterior (AP)) and side-to-side (mediolateral (ML)) directions with no or only little movement in the vertical direction.
In this study we have examined in a laboratory setting, the existence and magnitude of activity counts in the anteroposterior and mediolateral axes during dynamic sitting. The results show that a hip-worn accelerometer recorded activity counts with an intensity level up to 236 cpm in the AP axis and 139 cpm in the ML axis. When using a cut-off point of <100 cpm in the vertical axis and ≥100 in the AP or ML axis to define dynamic sitting, the accelerometer recorded dynamic sitting in all participants and during all activities. The accelerometer recorded the most dynamic sitting during the not imposed activities “watching TV”, “playing cards” and “preparing food”. Although our experimental study was conducted in only a few participants, it suggest that a triaxial accelerometer worn on the hip can record significant amounts of activity counts in AP and ML axes during dynamic sitting. We therefore used it to identify dynamic sitting out of activity counts using a cut-off point of <100 cpm in the vertical axis and ≥100 cpm in the AP or ML axis.
In order to examine the relevance of dynamic sitting, we studied associations of dynamic sitting with cardio-metabolic health outcomes in the AGES-Reykjavik Study. The study was conducted among a sample of older participants who have shown to spend large amounts of time in sitting positions (Arnardottir et al., Citation2013) and were therefore eligible to have considerable amounts of dynamic sitting. Indeed, participants recorded on average 10 hours of sitting time per day, 2.4 hours of which was dynamic sitting. The amount of dynamic sitting ranged from 48 minutes to 5.5 hours. When expressed as percentage of sitting time, the amount of dynamic sitting ranged from 15% in quartile 1 to 33% in quartile 4. Participants who recorded more dynamic sitting compared to quartile 1, had an up to 3.0 kg/m2 lower BMI and an up to 8 cm smaller waist circumference after adjustment for confounding factors. Other metabolic outcomes which were associated with dynamic sitting were HDL-cholesterol, triglycerides, and systolic blood pressure. Although the effect sizes of these separate variables were small and therefore may not be clinically relevant, together they contributed to the association between dynamic sitting and the metabolic syndrome. Participants who recorded more dynamic sitting had a considerably lower odds for the metabolic syndrome compared to those who recorded the fewest amounts of dynamic sitting. Taken together, we have shown that dynamic sitting might be identified out of activity counts and that this behaviour was associated with more favourable health outcomes. These findings might be important, given the large amounts of time that people spend in a sitting or sedentary position (Arnardottir et al., Citation2013; Davis et al., Citation2011; Matthews et al., Citation2008).
The study of Bankoski and colleagues (Bankoski et al., Citation2011) was a first indication that dynamic sitting could be identified and be relevant. They examined the degree of activity during sedentary time (based on activity counts in only the vertical axis) and found that a higher degree of activity was associated with a decreased likelihood of the metabolic syndrome (Bankoski et al., Citation2011). In addition, it has been reported that self-reported fidgeting modified the association between self-reported sitting time and mortality (Hagger-Johnson, Gow, Burley, Greenwood, & Cade, Citation2016). Although fidgeting and sitting time were self-reported, the authors hypothesized that movement involved in fidgeting may protect against detrimental effects of sedentary behaviour (Hagger-Johnson et al., Citation2016). A few experimental studies on non-exercise activity have shown that fidgeting-like movement is associated with substantial increases in energy expenditure and is inversely related to body weight and body gain (Johannsen & Ravussin, Citation2008; Levine, Citation2004; Levine, Schleusner, & Jensen, Citation2000). In another experimental study it was demonstrated that fidgeting of the leg was sufficient to counteract the detrimental effects of prolonged sitting on leg endothelial function (Morishima et al., Citation2016). Lastly, Koepp and colleagues evaluated energy expenditure of a chair and an under-table device which were designed to promote fidgeting and they concluded that energy expenditure can increase by ~20–30% (CitationKoepp et al., Citation2016, 2017). The results of these studies and ours support the notion of the importance of limited movement for health.
In our study, associations were seen between dynamic sitting and BMI, waist circumference and the metabolic syndrome. The results for levels of HDL cholesterol and triglycerides were small and therefore probably not clinically relevant. However, due to additional adjustment for BMI, these results may have been to some extent subject to overadjustment bias, as BMI may be part of the pathway between dynamic sitting and the metabolic outcomes. The interpretation of the blood pressure findings is difficult, as healthy blood pressure levels defined for young and middle-aged adults (systolic blood pressure <130 mmHg and/or diastolic blood pressure <85 mmHg) may not apply for old or very old adults (Muller, Smulders, De Leeuw, & Stehouwer, Citation2014). The physiological mechanisms underlying the combined effects of being in a sitting position and activity have not yet been studied. It is therefore difficult to clarify the findings of the present study. The mechanisms may be similar to those suggested in studies of non-exercise activity, in which lipoprotein lipase (LPL) activity levels were mentioned (Villablanca et al., Citation2015). Since LPL is an essential enzyme that contributes to the metabolism and transport of lipids, modulation of this enzyme might have a variety of effects on metabolism (Hamilton, Hamilton, & Zderic, Citation2004). Further examination of the underlying mechanisms requires physiological studies.
Our laboratory study as well as the cohort study has strengths and limitations which should be mentioned. A major strength is its combination of a laboratory study with a cohort study and the unique combination of experimental and observational triaxial accelerometry data. Although most currently available accelerometers record data in three axes, such data have rarely been used to identify different states of sedentary time. Another strength of the cohort study was that almost all participants (92%) provided at least four valid days of accelerometry data. In addition, the adjustments for sedentary time and MVPA, as well as several demographic and health factors, exclude the possibility that these covariates account for the association of dynamic sitting with the cardio-metabolic outcomes.
An important limitation of both the laboratory and cohort study was the definition of dynamic sitting as having <100 cpm in the vertical axis and ≥100 cpm in the AP or ML axis. It can be discussed whether a cut-off point of <100 cpm in the V axis should be used to identify sedentary time. Although this cut-off point has been widely used and has also been consistently associated with health outcomes (Biswas et al., Citation2015; Brocklebank, Falconer, Page, Perry, & Cooper, Citation2015; Wilmot et al., Citation2012), evidence for the accuracy of this cut-off point is limited. To date, two studies recommended a cut-off point of ≤150 cpm for adults (Carr & Mahar, Citation2012; Kozey-Keadle et al., Citation2011), while three others argued for ≤50 cpm for adults (Crouter et al., Citation2013), and <25 cpm or ≤22 cpm for older adults (Aguilar-Farías et al., Citation2014; Koster et al., Citation2016). Further, since data of the AP and ML axes have rarely been used, validation studies on cut-off points for AP and ML data recorded with a hip-worn accelerometer have not yet been conducted. Although our results suggest that dynamic sitting might be identified in a laboratory setting using this definition, studies are needed to validate and standardize (measurement of) dynamic sitting, for example determination of cut-off points. Clearly, this includes studies in larger populations, in a variety of participants including different age-groups, and outside the laboratory setting. Furthermore, energy expenditure should be measured. This can provide insight which activities with a low intensity level can increase energy expenditure enough to have an effect on health.
Another limitation of the cohort study was the uncertainty regarding the sitting position of participants. A hip-worn accelerometer may not accurately distinguish between sitting and standing, thus activity counts of the AP and ML axes assumed to be recorded during dynamic sitting, may have been recorded during standing as well as during sitting (Koster et al., Citation2016). To overcome this limitation, posture-based devices should be used. Since these devices discriminate between sitting and upright positions, misclassification of standing as sitting time is prevented and estimations of sitting time are more accurate (Godfrey et al., Citation2007; Kozey-Keadle et al., Citation2011). However, activity counts are lacking when using solely posture-based measurement. Therefore, future studies ideally should use a combination of posture- and acceleration-based measurement which provides an accurate and comprehensive assessment of daily activity levels.
Other limitations of the cohort study were its cross-sectional design, which limits the possibility of identifying causal relationships, and residual confounding due to unmeasured, but possibly relevant factors such as diet. Finally, the study sample consisted of a relatively healthy subsample of older adults, which could limit the generalization of our results.
In summary, we used data of a laboratory and cohort study to examine dynamic sitting. In the laboratory study we showed that dynamic sitting might be identified out of triaxial activity counts recorded with a hip-worn accelerometer. Subsequently, the results of the cohort study show that this behaviour was associated with more beneficial health outcomes, including BMI, waist circumference, and the metabolic syndrome. These findings suggest that dynamic sitting might affect health, which could be important given the large amounts of time people spend sitting. Future studies with a focus on validation, causation and physiological pathways are needed to further examine the relevance and importance of dynamic sitting.
Supplemental Material
Download PDF (77.8 KB)Acknowledgments
The AGES-Reykjavik study has been funded by NIH contract N01-AG-12100, the NIA Intramural Research Program, Hjartavernd (the Icelandic Heart Association), and the Althingi (the Icelandic Parliament). A Koster has received funding from the European Union Seventh Framework Programme (FP7-PEOPLE-2011-CIG) under grant agreement PCIG09-GA-2011-293621.
All authors declare: no support from any organization for the submitted work; no other relationships or activities that could appear to have influenced the submitted work.
Disclosure statement
No potential conflict of interest was reported by the authors.
Supplementary material
Supplementary data for this article can be accessed here
Additional information
Funding
References
- Aguilar-Farías, N., Brown, W., & Peeters, G. (2014). ActiGraph GT3X+ cut-points for identifying sedentary behaviour in older adults in free-living environments. Journal of Science and Medicine in Sport, 17, 293–299.
- Arnardottir, N. Y., Koster, A., Van, D. D., Brychta, R. J., Caserotti, P., Eiriksdottir, G., … Sveinsson, T. (2013). Objective measurements of daily physical activity patterns and sedentary behaviour in older adults: Age, Gene/Environment Susceptibility-Reykjavik Study. Age and Ageing, 42, 222–229.
- Atkin, A. J., Gorely, T., Clemes, S. A., Yates, T., Edwardson, C., Brage, S., … Biddle, S. J. (2012). Methods of measurement in epidemiology: Sedentary behaviour. International Journal of Epidemiology, 41, 1460–1471.
- Bankoski, A., Harris, T. B., McClain, J. J., Brychta, R. J., Caserotti, P., Chen, K. Y., … Koster, A. (2011). Sedentary activity associated with metabolic syndrome independent of physical activity. Diabetes Care, 34, 497–503.
- Biswas, A., Oh, P., Faulkner, G., Bajaj, R., Silver, M., Mitchell, M., & Alter, D. A. (2015). Sedentary time and its association with risk for disease incidence, mortality, and hospitalization in adults: A systematic review and meta-analysis. Annals of Internal Medicine, 162, 123–132.
- Brocklebank, L. A., Falconer, C. L., Page, A. S., Perry, R., & Cooper, A. R. (2015). Accelerometer-measured sedentary time and cardiometabolic biomarkers: A systematic review. Preventive Medicine, 76, 92–102.
- Butte, N. F., Ekelund, U., & Westerterp, K. R. (2012). Assessing physical activity using wearable monitors: Measures of physical activity. Medicine and Science in Sports and Exercise, 44, 5–12.
- Carr, L. J., & Mahar, M. T. (2012). Accuracy of intensity and inclinometer output of three activity monitors for identification of sedentary behavior and light-intensity activity. Journal of Obesity, 2012, 460271.
- Crouter, S. E., DellaValle, D. M., Haas, J. D., Frongillo, E. A., & Bassett, D. R. (2013). Validity of ActiGraph 2-regression model, Matthews cut-points, and NHANES cut-points for assessing free-living physical activity. Journal of Physical Activity & Health, 10, 504–514.
- Davis, M. G., Fox, K. R., Hillsdon, M., Sharp, D. J., Coulson, J. C., & Thompson, J. L. (2011). Objectively measured physical activity in a diverse sample of older urban UK adults. Medicine and Science in Sports and Exercise, 43, 647–654.
- Godfrey, A., Culhane, K., & Lyons, G. (2007). Comparison of the performance of the activPAL™ professional physical activity logger to a discrete accelerometer-based activity monitor. Medical Engineering & Physics, 29, 930–934.
- Grundy, S. M., Cleeman, J. I., Daniels, S. R., Donato, K. A., Eckel, R. H., Franklin, B. A., et al. (2005). Diagnosis and management of the metabolic syndrome: An American Heart Association/National Heart, Lung, and Blood Institute scientific statement. Circulation, 112, 2735–2752.
- Hagger-Johnson, G., Gow, A. J., Burley, V., Greenwood, D., & Cade, J. E. (2016). Sitting time, fidgeting, and all-cause mortality in the UK Women’s Cohort Study. American Journal of Preventive Medicine, 50, 154–160.
- Hamilton, M., Hamilton, D., & Zderic, T. (2004). Exercise physiology versus inactivity physiology: An essential concept for understanding lipoprotein lipase regulation. Exercise and Sport Sciences Reviews, 32, 161–166.
- Harris, T. B., Launer, L. J., Eiriksdottir, G., Kjartansson, O., Jonsson, P. V., Sigurdsson, G., et al. (2007). Age, Gene/Environment Susceptibility-Reykjavik Study: Multidisciplinary applied phenomics. American Journal of Epidemiology, 165, 1076–1087.
- Healy, G. N., Clark, B. K., Winkler, E. A. H., Gardiner, P., Brown, W. J., & Matthews, C. E. (2012). Measurement of adults’ sedentary time in population-based studies. American Journal of Preventive Medicine, 41, 216–227.
- Johannsen, D., & Ravussin, E. (2008). Spontaneous physical activity: Relationship between fidgeting and body weight control. Current Opinion in Endocrinology, Diabetes, and Obesity, 15, 409–415.
- Koepp, G. A., Moore, G., & Levine, J. A. (2017). An under-the-table leg-movement apparatus and changes in energy expenditure. Frontiers in Physiology, 8, 1–6.
- Koepp, G. A., Moore, G. K., & Levine, J. A. (2016). Chair-based fidgeting and energy expenditure. British Association of Sport and Exercise Medicine, 2, e000152.
- Koster, A., Shiroma, E., Caserotti, P., Matthews, C., Chen, K., Glynn, N., et al. (2016). Comparison of sedentary estimates between activPAL and hip- and wrist-worn actigraph. Medicine and Science in Sports and Exercise, 48, 1514–1522.
- Kozey-Keadle, S., Libertine, A., Lyden, K., Staudenmayer, J., & Freedson, P. S. (2011). Validation of wearable monitors for assessing sedentary behavior. Medicine and Science in Sports and Exercise, 43, 1561–1567.
- Levine, J. A. (2004). Nonexercise activity thermogenesis (NEAT): Environment and biology. American Journal of Physiology. Endocrinology and Metabolism, 286, E675–E685.
- Levine, J. A., Schleusner, S., & Jensen, M. (2000). Energy expenditure of nonexercise activity. The American Journal of Clinical Nutrition, 72, 1451–1454.
- Matthews, C. E., Chen, K. Y., Freedson, P. S., Buchowski, M. S., Beech, B. M., Pate, R. R., et al. (2008). Amount of time spent in sedentary behaviors in the United States, 2003–2004. American Journal of Epidemiology, 167, 875–881.
- Matthews, C. E., Kozey-Keadle, S., Moore, S., Schoeller, D., Carroll, R., Troiano, R. P., & Sampson, J. N. (2018). Measurement of active and sedentary behavior in context of large epidemiologic studies. Medicine and Science in Sports and Exercise, 50, 266–276.
- Morishima, T., Restaino, R. M., Walsh, L. K., Kanaley, J. A., Fadel, P. J., & Padilla, J. (2016). Prolonged sitting-induced leg endothelial dysfunction is prevented by fidgeting. American Journal of Physiology. Heart and Circulatory Physiology, 311, H177–82.
- Muller, M., Smulders, Y. M., De Leeuw, P. W., & Stehouwer, C. D. A. (2014). Treatment of hypertension in the oldest old. A critical role for frailty? Hypertension, 63, 433–441.
- Tremblay, M. S., Aubert, S., Barnes, J. D., Saunders, T. J., Carson, V., Latimer-Cheung, A. E., et al. (2017). Sedentary Behavior Research Network (SBRN) – Terminology consensus project process and outcome. The International Journal of Behavioral Nutrition and Physical Activity, 14, 1–17.
- Villablanca, P. A., Alegria, J. R., Mookadam, F., Holmes, D. R., Wright, R. S., & Levine, J. A. (2015). Nonexercise activity thermogenesis in obesity management. Mayo Clinic Proceedings. Mayo Clinic, 90, 509–519.
- Wilmot, E. G., Edwardson, C. L., Achana, F. A., Davies, M. J., Gorely, T., Gray, L. J., et al. (2012). Sedentary time in adults and the association with diabetes, cardiovascular disease and death: Systematic review and meta-analysis. Diabetologia, 55, 2895–2905.