ABSTRACT
We examined associations between youth 24-hour activity behaviour compositions and mental health. Data were collected from 359 participants (aged 9–13 years). Activity behaviours (sleep, sedentary time (ST), light physical activity (LPA) and moderate-to-vigorous physical activity (MVPA)) were assessed using wrist-worn accelerometers. Questionnaires and a computerized cognitive test battery assessed mental health outcomes. Linear mixed models examined associations between activity behaviour compositions and mental health. Post-hoc analyses modelled the influence of reallocating fixed durations of time between activity behaviours on mental health. ST was associated with worse internalizing problems (all participants; p< 0.05) and poorer prosocial behaviour (primary school participants only; p< 0.05), relative to the other activity behaviours. LPA was associated with worse cognitive test scores among primary school participants; p< 0.05). For all participants, reallocating time to ST from sleep and MVPA was associated with higher internalizing problems. Among primary school participants, reallocating time to ST from any other behaviour was associated with poorer prosocial behaviour, and reallocating time to LPA from any other behaviour was associated with lower executive function. Children’s mental health may be promoted by schools integrating opportunities for MVPA throughout the day. Our results provide further evidence for the influence of daily activity behaviours on youth mental health.
Introduction
The introduction of 24-hour movement guidelines for children and adolescents (Australian Government Department of Health, Citation2019; Canadian Society for Exercise Physiology, Citation2016; New Zealand Ministry of Health, Citation2017) has heralded a shift in the study of physical activity, sedentary behaviour, and sleep. In youth this has corresponded with a growth in research into the associations between health and 24-hour activity behaviour compositions (Carson, Tremblay et al., Citation2016; Dumuid, Stanford et al., Citation2018; Fairclough et al., Citation2017; Talarico & Janssen, Citation2018; Taylor et al., Citation2019). Most studies to date have focused on physical health outcomes, such as obesity, cardiometabolic risk, fitness, and musculoskeletal health (Carson, Tremblay et al., Citation2016; Dumuid, Stanford et al., Citation2018; Fairclough et al., Citation2017; Saunders et al., Citation2016). This research demonstrates that generally, higher volumes and intensities of physical activity, less time spent in sedentary behaviours, and longer sleep are beneficial for physical health in youth (Carson, Tremblay et al., Citation2016; Dumuid, Stanford et al., Citation2018; Fairclough et al., Citation2017; Saunders et al., Citation2016). Less is known about the influence of activity behaviours on aspects of youth mental health, such as anxiety, depression, self-esteem, health-related quality of life, and cognitive function (Biddle & Vergeer, Citation2020). In Europe, 12.8% of youth have at least one mental health disorder (Kovess-Masfety et al., Citation2016) with anxiety, depression, and low self-esteem particularly prevalent (Government Statistical Service, Citation2018). This is mirrored in Australia (13.9%) (Lawrence et al., Citation2015), while among American youth prevalence of a diagnosed mental health disorder ranges from 3.2% (depression) to 9.4% (hyperactivity) with the incidence of anxiety and depression increasing temporally (Centers for Disease Control and Prevention, Citation2020). Mental health problems during childhood and adolescence increase with age (Inchley et al., Citation2020), and are likely to impair mental and physical health and limit opportunities to thrive in adulthood (WHO, Citation2019). Therefore, it is important to identify ways to prevent youth mental health problems, with activity behaviours suggested as viable intervention approaches (Bauducco et al., Citation2020; Ekkekakis, Citation2013; Stiglic & Viner, Citation2019).
The relationship between mental health and youth physical activity is complicated by the interplay between different mental health outcomes, personal characteristics, and physical activity type, intensity, and context (Biddle & Vergeer, Citation2020; Weatherson et al., Citation2020). A recent review of reviews found inconsistent associations between youth physical activity and depression and self-esteem but stronger positive associations with cognitive function (Biddle et al., Citation2019). No evidence was observed for a physical activity dose–response relationship with depression, self-esteem, or cognitive function, although positive changes in these outcomes as a result of physical activity interventions were reported (Biddle et al., Citation2019). This concurs with Rodriguez and colleagues’ 2019 systematic review and meta-analysis, which observed a small but significant intervention effect for physical activity and youth mental health (Rodriguez-Ayllon et al., Citation2019). Moreover, physical activity interventions in school settings have significant moderate to strong beneficial effects on resilience, positive mental health, anxiety, and wellbeing (Andermo et al., Citation2020). Youth sedentary time (ST) is significantly associated with depression and psychological wellbeing (Asare, Citation2015; Rodriguez-Ayllon et al., Citation2019), while recent meta-analyses reported small but significant associations between overall ST and anxiety (Stanczykiewicz et al., Citation2019). Time spent using screens (e.g., TV viewing, video gaming, computer use) has different associations with health than overall ST (Stanczykiewicz et al., Citation2019), and among youth there is moderately strong and moderate evidence for screen time associations with depression and quality of life, respectively (Stiglic & Viner, Citation2019). Further, unfavourable behavioural conduct, poor self-esteem, and low social support are associated with screen use in youth (Carson, Hunter et al., Citation2016; Costigan et al., Citation2013; Suchert et al., Citation2015). Sleep duration is consistently associated with quality of life and emotional regulation (Chaput et al., Citation2016), internalizing and externalizing behavioural problems (Astill et al., Citation2012), although associations with cognitive function are inconsistent (Astill et al., Citation2012; Chaput et al., Citation2016; Short et al., Citation2018). However, other sleep dimensions such as quality, timing, and architecture and their interactions (Chaput et al., Citation2016) may also have important influences on youth mental health.
For the first time, the combined associations between physical activity, sedentary behaviour, and sleep with youth mental health were recently reviewed (Sampasa-Kanyinga et al., Citation2020). Meeting public health recommendations for all three behaviours was associated with better mental health when compared to youth who did not meet any of the recommendations, and a dose–response gradient was observed between the number of recommendations achieved and more positive mental health (Sampasa-Kanyinga et al., Citation2020). To strengthen the evidence on this topic, recommendations from Sampasa-Kanyinga et al.’s review included the use of robust measures of activity behaviours, validated mental health indicators, and compositional analysis approaches (Sampasa-Kanyinga et al., Citation2020). Our study reflects these recommendations and adds to the current knowledge in the area by employing device-based assessment of activity behaviours and examining their association with established measures of mental health and cognitive function within a compositional data analytical framework. The study aims were to (i) examine the cross-sectional associations between device-measured 24-hour activity compositions and validated mental health outcomes in a sample of children and adolescents, and (ii) investigate the predicted differences in mental health outcomes when the time was reallocated between the activity behaviours.
Materials and Methods
Participants and settings
This cross-sectional study took place in the West Lancashire region of northwest England between April 2019 and March 2020. Ten primary schools and two secondary schools from two neighbouring semi-urban towns with similar sociodemographic characteristics were recruited. All children and adolescents aged 9–11 years (Years 5 and 6 of primary school) and 12–13 years (Year 8 of secondary school) in the 12 schools attended project presentations and were invited to take part. All of the primary schools received curricular and extra-curricular physical activities and school sport provision from the local School Sport Partnership which employs physical education and physical activity specialists to deliver sessions. Physical activity resources and delivery in the two secondary schools were undertaken by the specialist physical education teachers in each school’s physical education department. As such, the physical activity opportunities were similar between the participating primary and secondary schools, respectively. To be eligible to participate the students needed to be able to participate in activities of daily living and to provide written parent/carer informed consent and their own written assent. Six hundred and ninety-one students were informed about the project, 382 provided informed consent to participate (55.3% response rate), with 23 of these excluded from the analysis due to absence on the data collection days. As an incentive to take part in the project, each participant received a £10 shopping voucher following data collection. The study received ethical approval from the Edge Hill University Research Ethics Committee (# SPA-REC-2018-007) which aligned to the principles of the Declaration of Helsinki.
Demographic measures
Participants’ dates of birth, home post codes, and ethnicity were obtained from the schools’ information management systems. Decimal age and 2019 Indices of Multiple Deprivation (IMD) scores (Ministry of Housing, Communiities and Local Government, Citation2019) were calculated using data collection dates and home post codes, respectively. IMD scores provide an area-level relative measure of deprivation based on income, employment, education, health, crime, housing, and living environment (Department for Communities and Local Government, Citation2015). Area-level socioeconomic status (SES) was represented by the IMD decile for each participant.
Anthropometric measures
Height was measured to the nearest 0.1 cm using a portable stadiometer (Leicester Height Measure, Seca, Birmingham, UK), and body mass was measured to the nearest 0.1 kg using calibrated scales (813 model, Seca). Body mass index (BMI) was calculated for each participant, BMI z-scores were calculated (Cole et al., Citation1995) and IOTF BMI cut-points applied to classify the participants’ weight status (Cole et al., Citation2000). For all measurements, the participants wore shorts and t-shirt with shoes removed.
Activity behaviours
Participants wore ActiGraph GT9X triaxial accelerometers (ActiGraph, Pensacola, FL, USA) on the non-dominant wrist for 24 h·day−1 over 7 days with accelerations recorded at 100 Hz. Wrist-worn accelerometers have demonstrated excellent validity against energy expenditure (Esliger et al., Citation2011) and can accurately classify physical activity intensities in youth (Hildebrand et al., Citation2014; Okely et al., Citation2018). Data were downloaded using ActiLife version 6.11.9 (ActiGraph, Pensacola, FL, USA), and saved as GT3X files, before being converted to raw csv file format for signal processing. These csv files were processed in R (http://cran.r-project.org) using GGIR v1.9.0 (Migueles, Rowlands et al., Citation2019) which carried out autocalibration procedures (Van Hees et al., Citation2014), identified non-wear (Van Hees et al., Citation2013), and converted the raw triaxial accelerometer signals into 1 omnidirectional measure of acceleration (Euclidean Norm Minus-One; ENMO) expressed in milligravitational units (mg) (Van Hees et al., Citation2013). The ENMO metric has been used extensively (Fairclough et al., Citation2017, Citation2020, Citation2019; Hildebrand et al., Citation2014; Rowlands et al., Citation2018, Citation2019), and ENMO values derived from non-dominant wrist accelerations share 87–97% of the explained variance with alternative raw acceleration metrics (Migueles, Cadenas-Sanchez et al., Citation2019). ENMO values were reduced to 5 s epochs and averaged over each of the 7 monitored days to represent average acceleration. Participants were excluded if accelerometer post-calibration error was >10 mg (Van Hees et al., Citation2014) and if less than 3 valid week or weekend days of wear (i.e., at least 16 h⋅day−1 (Rowlands, Citation2018)) were recorded (Rich et al., Citation2013). Accelerometer non-wear was determined based on the SD and value range of the accelerations at each axis, calculated for 60-minute windows with a 15-minute sliding window (Van Hees et al., Citation2013). If for at least 2 out of the 3 axes the SD was less than 13 mg or the value range was less than 50 mg, the time window was classified as non-wear (Van Hees et al., Citation2013). By default, GGIR imputed non-wear data by the average at similar time points on other days of the week (5.0 ± 4.0% of data per day were imputed). Youth-specific non-dominant wrist ENMO cutpoints of 50 mg (Hurter et al., Citation2018) and 200 mg (Hildebrand et al., Citation2014) defined estimated ST/light physical activity (LPA), and moderate-to-vigorous physical activity (MVPA), respectively. Sleep duration was estimated using a polysomnography-validated accelerometer algorithm based on the distribution of change in the z-angle (i.e., corresponding to the axis positioned perpendicular to the skin surface) (Van Hees et al., Citation2018).
Mental health outcomes
Three questionnaires assessing mental health outcomes were completed by the children in class following scripted instructions from the second author in the presence of a teacher and two other researchers who were present to assist.
Self-esteem
The Rosenberg Self-Esteem Scale (RSES) (Rosenberg, Citation1965) assessed self-esteem. The RSES is a 10-item scale which asks about feelings of self-worth in the last 14 days to produce a unidimensional measure of global self-esteem. The scale ranges from 0 to 30 with scores between 15 and 25 within “normal range” and those below 15 suggesting low self-esteem (Rosenberg, Citation1965). The RSES has demonstrated its criterion and construct validity and test–retest reliability (Bagley & Mallick, Citation2001; Rosenberg, Citation1965) and has been previously used with youth (Bagley & Mallick, Citation2001). Internal consistency of the RSES in our sample was Cronbach’s α = 0.85.
Depression
Depressive symptoms were assessed using the Mood and Feelings Questionnaire (MFQ) (Angold et al., Citation1995), which uses 33 phrases to ask participants how they have been feeling or acting in the last 14 days. Scores range from 0 to 66, with higher scores suggesting more severe depressive symptoms. Scoring 27 or higher may indicate the presence of depression (Angold et al., Citation1995). Validity and reliability of the MFQ have been demonstrated in clinical and community samples of children and adolescents (Angold et al., Citation1995; Burleson Daviss et al., Citation2006). Internal consistency of the MFQ in our sample was Cronbach’s α = 0.95.
Emotional and behavioural problems
Emotional and behavioural aspects of mental health were measured using the Strengths and Difficulties Questionnaire (SDQ) (Goodman, Citation2001). The SDQ comprises five scales of five items each related to perceived emotional problems, behavioural problems, hyperactivity, peer relationship problems, and prosocial behaviour in the last six months. For each scale the scores range from 1 to 10 while overall emotional and behavioural mental health is scored from 0 to 40 (i.e., the sum of all scale scores except for prosocial behaviour). Higher scores indicate unfavourable mental health, with the opposite true for prosocial behaviour. The SDQ is widely used and in community samples it is advised to report the broad constructs of internalizing problems (emotional problems and peer relationships), externalizing problems (behavioural problems and hyperactivity), and prosocial behaviour, as well as overall emotional and behavioural mental health (Goodman et al., Citation2010). Internal consistency of the SDQ in our sample was Cronbach’s α = 0.72, 0.76, 0.59, and 0.80 for internalizing problems, externalizing problems, prosocial behaviour, and overall emotional and behavioural mental health, respectively.
Cognitive function
Executive functions are a subset of higher-order cognitive functions that are involved in goal-directed motor outputs. Three core executive functions are switching between tasks or mental sets, updating and monitoring of spatial working memory, and inhibition of dominant or prepotent responses (Miyake et al., Citation2000). The CANTAB Connect (Cambridge Cognition Ltd, Cambridge, UK) computerized executive function test battery was used to assess switching, spatial working memory, and inhibition. Switching was assessed using the Intradimensional/Extradimensional Set-Shifting task, which involves discrimination and reversal learning across nine stages. The number of stages completed can be age-dependent across childhood and adolescence (Luciana & Nelson, Citation2002). To account for potential variable stage completion, our outcome measure was the total number of errors adjusted for the number of stages completed. The Spatial Working Memory test assesses the ability to maintain and manipulate information in working memory using a self-ordered searching task. The outcome was the total number of errors, which accounted for the different types of errors made during the task. Inhibition was measured using the Multitasking Test, which required participants to manage different sources of conflicting information. The inhibition outcome was the total number of errors calculated across all assessed trials and for all error types. For each of the three executive function tasks, lower error scores indicated better performances. The three tests were completed individually by each participant using an Apple iPad (iPad 6th generation, iOS 13.3.1; Apple Inc., CA, USA) under the direction of a trained researcher.
Data analysis
Twenty-three participants were absent on data collection days and were discounted from the analysis. Of the remaining 359 participants (210 primary, 149 secondary), the number with missing data for the covariates and mental health outcomes ranged from 1 (0.3%) (BMI z-score) to 9 (2.5%) (IMD decile), and 2 (0.6%) (inhibition) to 11 (1%) (switching), respectively. Eighty-eight participants (24.5%) did not meet the accelerometer wear inclusion criteria and their activity behaviour data were coded as missing. A dummy variable was created for the accelerometer data to indicate which participants had observed (0) or missing values (1), and t-tests were computed with the continuous covariates and mental health outcomes as the dependent variables. Chi-squared tests examined the association between sex and missing accelerometer data. There were no significant differences in any of the covariates, or the internalizing problems and depression scores between participants with observed and missing accelerometer data. Emotional and behavioural mental health (p= .001), externalizing problems (p< .001), prosocial behaviour (p< .001), self-esteem (p= .03), and working memory (p= .04) were significantly different, with participants with missing accelerometer data scoring more poorly on these outcomes. Further, significantly more boys than girls had missing accelerometer data (p= .004). This suggested that these variables were missing at random (MAR) and multiple imputation was therefore performed for all exposure, outcome, and covariate variables in IBM SPSS Statistics (version 25, IBM Inc., NY, USA) using the Expectation Maximization algorithm.
Compositional analyses were conducted using R open-source software (v. 3.6.2, www.r-project.org) with the packages, “compositions” (v. 1.40–5) (Van den Boogaart & Tolosana-Delgado, Citation2008) and “robCompositions” (v. 2.2.1) (Templ et al., Citation2011). Time-use compositions were expressed as four specific sets of three isometric log ratio (ILR) coordinates called pivot coordinates (Chastin et al., Citation2015; Hron et al., Citation2017). The first coordinate of each set contained one activity behaviour (either sleep, ST, LPA, MVPA) relative to all remaining activity behaviours. The pivot coordinates were created so that each set included a different behaviour relative to all remaining behaviours as the first ILR. The sets of pivot co-ordinates were then used as explanatory variables in subsequent regression analyses. To accommodate the nesting of participants within the 12 schools, a mixed model approach (“school” as the single, random intercept) was used to analyse the association of each mental health outcome with the time-use ILRs. Adjustment was made for age, sex, BMI z-scores, and IMD deciles in each model, which is consistent with similar studies (Bang et al., Citation2020). There was no correlation above r= 0.25 between any of the explanatory variables so collinearity was not considered to be an issue in the models. In models where sex was significantly associated with the mental health outcome, follow-up sex-stratified analyses were performed to assess the moderating influence of sex. Further analyses were performed by stratifying by type of school, with age removed from these models (primary: n = 210; secondary: n = 149). All mixed model analyses were performed in R with the ‘lme4ʹ package (v. 1.1.3) (Bates et al., Citation2015), with statistical significance determined by the “lmerTest” package (v. 3.1.2) (Kuznetsova et al., Citation2017).
Models were checked to ensure assumptions were not violated for the use of linear mixed effects (“lmer” function in ‘lme4ʹ package). This led to some models being expressed as generalized linear mixed effects (“glmer” function in ‘lme4ʹ package). If the ANOVA table of the model fit showed that the set of time-use ILRs were not significantly associated with the selected mental health indicator, no further analysis was carried out. If significance was observed, four models were carried out, each model using a different set of pivot coordinates, so that each model examined associations with one activity behaviour, relative to all remaining behaviours. The regression coefficient and p-value of the first coordinate (ILR_1) representing one behaviour relative to remaining behaviours was examined for each behaviour to determine which behaviour was most dominant in the relationship with the mental health indicator. This behaviour was then the focus of compositional isotemporal substitution analyses to model the influence of reallocating fixed time durations (5, 10, 15, 20 minutes) between it and the other three behaviours (Dumuid et al., Citation2019). Effect-sizes (ES) for predicted differences in mental health indicators were calculated as ratios of the model residual standard deviation (Dumuid, Maher et al., Citation2018).
Results
Descriptive results
Of the imputed analytical sample (N = 359), 96.9% of the participants were White British, of medium-high SES (IMD decile = 7.3 ± 2.3), 50.7% were girls, and almost 25% were overweight or obese (). Geometric means of activity behaviours (linearly adjusted to collectively sum to 1440 minutes) and descriptive mental health outcomes are presented in . Participants spent 44.1% of the 24-hour day in ST and 37% of the time sleeping. LPA and MVPA represented 14.6% and 3.5% of daily time, respectively. Primary school participants spent longer sleeping and less time being sedentary than secondary school peers. Less than 25% of the participants averaged at least 60 minutes MVPA per day (Department of Health and Social Care, Citation2019) and almost 75% had less than 9 hours sleep⋅night−1 (Paruthi et al., Citation2016). Participants were highly compliant to wearing the accelerometers, averaging almost 6 days of valid wear for 22.8 ± 1.0 hour·day−1. Emotional and behavioural mental health scores from the SDQ were in the “close to average” range (Meltzer et al., Citation2003), while MFQ and RSES scores indicated no presence of depressive symptoms (Burleson Daviss et al., Citation2006) and “normal” range for self-esteem (Rosenberg, Citation1965), respectively. The secondary school participants performed better on each of the cognitive tests of executive function than their younger peers.
Table 1. Descriptive characteristics of the participants (M (SD) unless indicated otherwise)
Table 2. Descriptive accelerometer and mental health variables (M (SD) unless indicated otherwise)
Compositional regression analyses
summarizes the results from the regression models for each mental health indicator using data for primary, secondary, and all schools combined (see Supplementary file 1 for full model outputs). The activity composition ILR coordinates were significantly associated with internalizing problems (all schools, p= 0.01), prosocial behaviour (p= 0.03), and the switching (p= 0.02) and inhibition (p< 0.001) aspects of executive function among primary school participants. No significant associations were observed between the secondary school participants’ activity compositions and mental health outcomes. Sex was a significant predictor in the models for externalizing problems (p= 0.01) and prosocial behaviour (p< 0.001), but sex-stratified analyses revealed non-significant associations between the activity compositions and these mental health outcomes (p= 0.60–0.75).
Table 3. Associations between activity composition and mental health outcomes
Beta coefficients for the first pivot coordinate of the models showing significant associations between activity composition and outcomes are presented in . For all participants, ST, relative to the other activity behaviours was associated with worse internalizing problems (β1 = 0.32, p= 0.03). Among primary school participants, ST, relative to the other behaviours, was associated with poorer prosocial behaviour (β1 = −2.25, p= 0.007), and LPA, relative to the other behaviours, was associated with more switching and inhibition test errors (β1 = 51.89 p= 0.004; β1 = 23.56, p< 0.001).
Table 4. Relationship between significant mental health outcomes and the activity behaviour isometric log-ratio regression coefficients
Compositional isotemporal substitution analyses: One-for-one reallocations
Using the average composition () as the starting durations for each activity, and Supplementary file 2 show predicted differences in mental health outcomes when incremental durations of time (5, 10, 15, 20 minutes) were added/subtracted from the most influential activity behaviour in , and redistributed from/to one other activity, keeping all remaining activities constant. The 95% CIs did not cross zero for any estimated differences in the outcomes (Supplementary file 2; CIs not shown on Figures to avoid overcrowding). For all participants, time reallocations to ST from either sleep or MVPA were associated with more internalizing problems (ES = 0.27 (5 min) to 1.33 (20 min when MVPA replaced ST). Among primary school participants, there were positive predicted differences in prosocial behaviour when ST was replaced with any of the other three behaviours (ES = 0.03 (5 min) to 0.10 (20 min) when MVPA replaced ST). In relation to executive function, when LPA replaced sleep, ST, and MVPA unfavourable predicted differences in primary school participants’ performances on the switching (ES = 2.74 (5 min) to 11.77 (20 min) when LPA replaced MVPA) and inhibition tasks (ES = 1.47 (5 min) to 6.26 (20 min) when LPA replaced MVPA) were observed. In all of these compositional isotemporal substitution analyses, predicted differences in the outcomes were greatest when MVPA was replaced with either ST or LPA, rather than when the time was reallocated to MVPA.
Figure 1. Estimated difference in mental health associated with time reallocation between pairs of behaviours: difference in all participants’ internalizing problems associated with the difference in ST to each of the remaining behaviours. For example, adding 20 minutes to ST at the expense of MVPA (green line) while keeping sleep and LPA constant was associated with a predicted increase in internalizing problems of 0.69 units
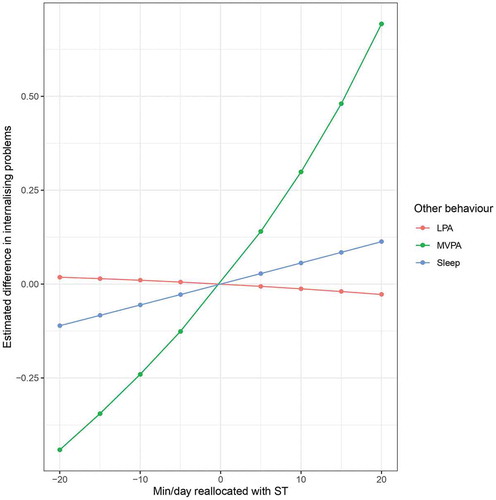
Figure 2. Estimated difference in mental health associated with time reallocation between pairs of behaviours: difference in primary school participants’ prosocial behaviour associated with the difference in ST to each of the remaining activities
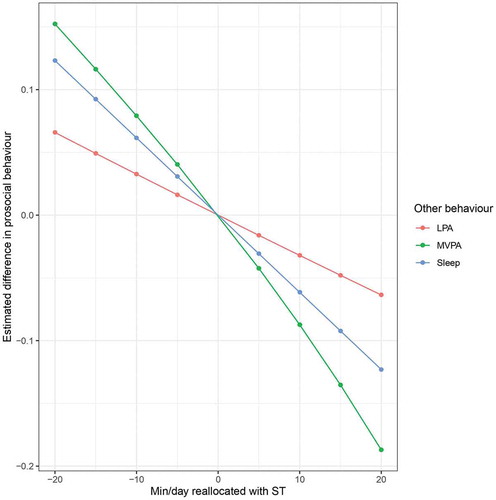
Figure 3. Estimated difference in executive function associated with time reallocation between pairs of movement behaviours: difference in primary school participants’ inhibition errors associated with LPA to each of the remaining activities. For example, adding 20 minutes to LPA at the expense of MVPA (red line) while keeping sleep and ST constant was associated with a predicted increase of 9.5 errors on the inhibition task
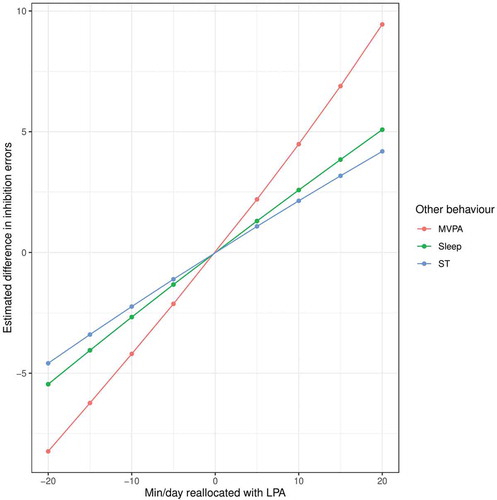
Discussion
This study investigated associations between 24-hour activity behaviours and a range of mental health outcomes in youth, which extend beyond those previously examined in compositional analysis studies (i.e., overall mental wellbeing (Carson, Tremblay et al., Citation2016) and health-related quality of life (Dumuid, Maher et al., Citation2018)). Overall, we found that activity compositions were significantly associated with internalizing problems, while among primary school participants they were associated with prosocial behaviours, and the switching and inhibition aspects of executive function. Relative to other activity behaviours, the time spent in ST was significantly associated with increased risk of internalizing problems, and inversely associated with prosocial behaviour (primary school participants only). Further, more time engaged in LPA by the primary school participants was significantly associated (relative to other behaviours) with poorer performances on the switching and inhibition tasks.
Internalizing problems encompass emotional and peer relationship difficulties and there is limited literature reporting associations between ST and these aspects of mental health. There is though, systematic review evidence supporting a direct positive relationship between sedentary screen time and increased risk of internalizing problems in school-aged youth (Suchert et al., Citation2015). Further, longitudinal findings from the UK show that children who watched TV and/or played video games for more than 3 hour/day were more likely to report emotional and peer-relationship difficulties 2 years later (Parkes et al., Citation2013). Similarly, US children and adolescents with more than 1 hour/day of daily screen time had lower emotional stability and increased difficulty making friends, with associations typically stronger among adolescents (Twenge & Campbell, Citation2018). Our findings of higher internalizing problems associated with higher ST (relative to other behaviours) may reflect the unfavourable influence of more sedentary screen time. It is possible that in analyses stratified by primary and secondary school, these relationships were only observed among primary school participants because there was more observed variance in the activity exposure and mental health outcome variables (see variation matrix in Supplementary file 3), and a lack of statistical power for the smaller secondary school sample.
ST was also inversely associated with prosocial behaviour but only in primary school participants. This is consistent with the associations between TV viewing/gaming and prosocial behaviours reported in Carson et al.’s 2016 systematic review (Carson, Hunter et al., Citation2016) and longitudinal data from the UK Millennium Cohort Study (Parkes et al., Citation2013). While these studies provide some support for our findings it is important to recognize that total ST as reported in our compositional analysis is a different measure than screen time; screen time can occur in non-sedentary postures (Tremblay et al., Citation2017), and high ST is largely independent of screen time (Hoffmann et al., Citation2019). It is unclear whether total ST (Stanczykiewicz et al., Citation2019) or specific types of ST are more strongly related to mental health outcomes (Teychenne et al., Citation2020). To address this, future studies should employ methodologies such as ecological momentary assessment or device-based pattern recognition approaches to identify specific types of sedentary behaviours (Teychenne et al., Citation2020), which may provide a more precise picture of the compositional relationships between activity behaviours and mental health.
The daily activity compositions were not associated with overall emotional and behavioural mental health, externalizing problems, depression, or self-esteem. Contrastingly, non-compositional studies have reported associations between these outcomes and sleep, ST, and physical activity (Asare, Citation2015; Biddle et al., Citation2019; Carson, Hunter et al., Citation2016; Chaput et al., Citation2016), but none accounted for the mutually exclusive and compensatory nature of these behaviours, which are relative to one another over a 24-hour day. Recently, this has been examined with studies showing that youth who met multiple guidelines for sleep, ST, and MVPA were likely to have better mental health than peers meeting fewer recommendations (Bang et al., Citation2020; Sampasa-Kanyinga et al., Citation2020; Weatherson et al., Citation2020). However, as our sample had a low prevalence of meeting physical activity and sleep guidelines, it is unlikely that this explanation would hold true with our data. Another possible explanation for the lack of observed associations is the relatively similar and favourable mental health questionnaire scores, which indicated that our sample was at low risk for poor mental health. Further, their SES was moderate to high, which is consistent with previous research reporting positive SES and youth mental health relationships (Bang et al., Citation2020; Reiss, Citation2013). The relatively high SES of our participants may have predisposed them to have favourable mental health status, which could have limited the precision and ability to detect associations between activity behaviours and the mental health outcomes (i.e., a ceiling effect) (Bang et al., Citation2020). We recognize though that the IMD was not sensitive to individual family level SES, hence, we exercise some caution with this supposition.
Among the primary school participants, the switching and inhibition aspects of executive function were positively associated with LPA relative to sleep, ST, and MVPA. Moreover, when time was reallocated between behaviours, the effect sizes were large and more substantial than for internalizing problems and prosocial behaviour. For example, a modelled reallocation of 10 minutes of LPA to MVPA estimated a − 4.20 difference in switching error score with an ES of 5.24. In contrast, a 10 minutes reallocation of ST to MVPA with prosocial behaviour as the outcome, estimated a 0.08 unit difference, with a substantially smaller ES of 0.05. Hence, in our sample the effect of reallocating more time to MVPA from ST or LPA appears to have been be more beneficial for executive function than for indictors of mental ill health.
Evidence exists for a relationship between physical activity and cognition and brain structures that support complex processes in controlled laboratory environments (Donnelly et al., Citation2016). Translating such findings to practice is important, particularly in school settings where there is great interest in how replacing sedentary class time with physical activity can promote learning through improved cognitive performance. Currently though, there is inconsistent evidence of the beneficial effects of such interventions (De Greeff et al., Citation2018; Singh et al., Citation2019). Similarly, evidence for associations between sleep duration and cognitive function is equivocal (Chaput et al., Citation2016) with the most recent meta-analysis of objectively assessed sleep and cognition reporting small, non-significant effects (Short et al., Citation2018). It has been proposed that because most youth sleep for less than the recommended duration (Paruthi et al., Citation2016) the cognitive performance of those with adequate sleep has been underrepresented in studies to date. Therefore, reported associations between “typical” sleep and cognition were attenuated because of the restricted range of sleep durations observed (Short et al., Citation2018). As less than 25% of our participants achieved the recommended sleep duration this attenuation of a sleep–cognition relationship may have also occurred in our sample.
In the compositional isotemporal substitution analyses, reallocations between MVPA and ST/LPA had the greatest predicted associations with internalizing problems, prosocial behaviours, switching, and inhibition (relative to the other behaviours), and estimated differences increased incrementally with the duration of time reallocated. MVPA is characterized by much higher energy expenditure than sleep, ST, and LPA; it may therefore provide a more powerful physiological stimulus for structural and hormonal changes that are favourable for cognition (Lubans et al., Citation2016). Moreover, MVPA for youth (e.g., sport, structured activities) often involves participation with peers, parental encouragement, and enhancement of emotional status (Hilland & Costigan, Citation2020; Weatherson et al., Citation2020), which are consistent with better internalizing mental health and prosocial behaviours. While these are possible explanations for the influence of MVPA on the outcomes in this study, it is acknowledged that the mechanistic evidence for this is currently limited (Lubans et al., Citation2016). Interestingly, associations with the outcomes were greatest when MVPA was replaced by ST or LPA, rather than when MVPA replaced these behaviours. These asymmetrical associated differences in health outcomes involving MVPA have previously been observed in youth studies considering adiposity, fitness, cardiometabolic risk, mental health, and health-related quality of life (Carson, Tremblay et al., Citation2016; Dumuid, Maher et al., Citation2018; Fairclough et al., Citation2017). This was likely due to MVPA constituting a relatively small proportion (50.9 minutes or 3.5%) of the activity composition. Therefore, a 20 minute reallocation in MVPA represents a change of 39%, whereby the equivalent absolute change in SB reflected only 3% of the geometric mean proportion of ST of 635 min⋅day−1 (Dumuid et al., Citation2019).
Strengths of this study included device-based assessment of 24-hour activity behaviours and use of compositional data analysis to examine how the full activity composition related to mental health. Moreover, ours is the first compositional analysis study to investigate multiple mental health indicators, measured using validated instruments and including objectively assessed cognitive function. We also used multiple imputation methods which when the data are MAR and covariates are available, can provide unbiased results compared to complete case analysis, and maintain statistical power because the sample size is not reduced (Sterne et al., Citation2009). There were also limitations which warrant consideration. The cross-sectional design precludes any claims of causal inferences and directionality between the activity composition and mental health indicators. We had a 55% participation rate and an imbalanced sample of primary and secondary school participants, who were relatively homogenous in respect of area-level SES, which may have been reflected in their relatively high mental health status and the limited associations with the activity compositions. Most of the mental health outcomes were measured using questionnaires which although validated, have the potential for social desirability bias. Lastly, the activity behaviours were defined using validated wrist acceleration cutpoints which for LPA and MVPA, reflect absolute intensity rather than relative intensity for each participant. This is likely to result in some misclassification of activity behaviours among the sample. Use of machine learning to label activity behaviours and intensities would contribute to addressing this issue in future studies.
Conclusions
Relative to other activity behaviours, ST and LPA had the greatest influence on some mental health outcomes. In stratified analyses, associations were only observed among primary school participants. Our results provide some support for the influence of daily activity behaviours on youth mental health. When the time was incrementally reallocated between the activity behaviours, the largest predicted changes in the outcomes occurred when MVPA was replaced by ST or LPA. The results provide further support for schools to promote children’s mental health by integrating opportunities for MVPA throughout the school day, particularly during periods such as classroom lessons that would traditionally be spent with children engaged in ST or LPA. From a practical perspective approaches such as active classroom breaks and walking/running initiatives (e.g., The Daily Mile) have great potential to displace ST or LPA with MVPA and confer mental health benefits (Morris et al., Citation2019). Our findings highlight the value of compositional data analysis for understanding the collective influence of activity behaviours on mental health outcomes, and guiding the focus of behaviour change interventions. Promoting these behaviours using conceptually developed family- (Rhodes et al., Citation2020) and school-based frameworks (Daly-Smith et al., Citation2020) is advocated. Future studies should study associations between activity behaviours and mental health longitudinally in samples that are representative of key demographic characteristics of the target population.
Disclosure of interest
The authors report no conflict of interest.
Supplemental Material
Download MS Word (15.4 KB)Supplemental Material
Download MS Word (25.1 KB)Supplemental Material
Download MS Word (34.7 KB)Availability of data and materials
The datasets used and/or analysed during the current study are available from the corresponding author on reasonable request.
Supplemental data
Supplemental data for this article can be accessed online https://doi.org/10.1080/02640414.2021.1890351.
Additional information
Funding
References
- Andermo, S., Hallgren, M., Nguyen, T. T. D., Jonsson, S., Petersen, S., Friberg, M., Romqvist, A., Stubbs, B., & Elinder, L. S. (2020). School-related physical activity interventions and mental health among children: A systematic review and meta-analysis. Sports Medicine - Open, 6(1), 25. https://doi.org/10.1186/s40798-020-00254-x
- Angold, A., Costello, E. J., Messer, S. C., Pickles, A., Winder, F., & Silver, D. (1995). The development of a short questionnaire for use in epidemiological studies of depression in children and adolescents. International Journal of Methods in Psychiatric Research, 5, 237–249.
- Asare, M. (2015). Sedentary behaviour and mental health in children and adolescents: A meta-analaysis. Journal of Child and Adolescent Health, 3(6). https://doi.org/10.4172/2375-4494.1000259
- Astill, R. G., Van der Heijden, K. B., Van Ijzendoorn, M. H., & Van Someren, E. J. (2012). Sleep, cognition, and behavioral problems in school-age children: A century of research meta-analyzed. Psychological Bulletin, 138(6), 1109–1138. https://doi.org/10.1037/a0028204
- Australian Government Department of Health. (2019). Australian 24-hour movement guidelines for children and young people (5-17 years) - An Integration of physical activity, sedentary behaviour and sleep. Australian Government Department of Health: Canberra. Retrieved June 15, 2020, from https://www1.health.gov.au/internet/main/publishing.nsf/Content/health-24-hours-phys-act-guidelines
- Bagley, C., & Mallick, K. (2001). Normative data and mental health construct validity for the Rosenberg self-esteem scale in British adolescents. International Journal of Adolescence and Youth, 9 (2–3), 117–126. https://doi.org/10.1080/02673843.2001.9747871
- Bang, F., Roberts, K. C., Chaput, J. P., Goldfield, G. S., & Prince, S. A. (2020). Physical activity, screen time and sleep duration: Combined associations with psychosocial health among Canadian children and youth. Health reports, 31(5), 9–16. https://doi.org/10.25318/82-003-x202000500002-eng
- Bates, D., Mächler, M., Bolker, B., & Walker, S. (2015). Fitting linear mixed-effects models using lme4. Journal of Statistical Software, 67(1), 48. https://doi.org/10.18637/jss.v067.i01
- Bauducco, S. V., Flink, I. K., Boersma, K., & Linton, S. J. (2020). Preventing sleep deficit in adolescents: Long-term effects of a quasi-experimental school-based intervention study. Journal of Sleep Research, 29(1), e12940. https://doi.org/10.1111/jsr.12940
- Biddle, S. J. H., & Vergeer, I. (2020). Mental health benefits of physical activity for young people. In T. A. Brusseau, S. J. Fairclough, & D. R. Lubans (Eds.), The routledge handbook of youth physical activity (pp. 121–147). Routledge.
- Biddle, S. J. H., Ciaccioni, S., Thomas, G., & Vergeer, I. (2019). Physical activity and mental health in children and adolescents: An updated review of reviews and an analysis of causality. Psychology of Sport and Exercise, 42, 146–155. https://doi.org/10.1016/j.psychsport.2018.08.011
- Burleson Daviss, W., Birmaher, B., Melhem, N. A., Axelson, D. A., Michaels, S. M., & Brent, D. A. (2006). Criterion validity of the mood and feelings questionnaire for depressive episodes in clinic and non-clinic subjects. Journal of Child Psychology and Psychiatry, 47(9), 927–934. https://doi.org/10.1111/j.1469-7610.2006.01646.x
- Canadian Society for Exercise Physiology. (2016). Canadian 24-hour movement guidelines for children and youth (ages 5–17 years). Canadian Society for Exercise Physiology: Ottawa Ontario. Retrieved June 15, 2020, from https://csepguidelines.ca/children-and-youth-5-17/
- Carson, V., Hunter, S., Kuzik, N., Gray, C. E., Poitras, V. J., Chaput, J.-P., Saunders, T. J., Katzmarzyk, P. T., Okely, A. D., Connor Gorber, S., Kho, M. E., Sampson, M., Lee, H., & Tremblay, M. S. (2016). Systematic review of sedentary behaviour and health indicators in school-aged children and youth: An update. Applied Physiology, Nutrition, and Metabolism, 41(6(Suppl. 3)), S240–S65. https://doi.org/10.1139/apnm-2015-0630
- Carson, V., Tremblay, M. S., Chaput, J.-P., & Chastin, S. F. M. (2016). Associations between sleep duration, sedentary time, physical activity, and health indicators among Canadian children and youth using compositional analyses. Applied Physiology, Nutrition, and Metabolism, 41(6(Suppl. 3)), S294–S302. https://doi.org/10.1139/apnm-2016-0026
- Centers for Disease Control and Prevention. (2020). Data and Statistics on Children’s Mental Health. Retrieved June 15, 2020, from https://www.cdc.gov/childrensmentalhealth/data.html
- Chaput, J.-P., Gray, C. E., Poitras, V. J., Carson, V., Gruber, R., Olds, T., Weiss, S. K., Connor Gorber, S., Kho, M. E., Sampson, M., Belanger, K., Eryuzlu, S., Callender, L., & Tremblay, M. S. (2016). Systematic review of the relationships between sleep duration and health indicators in school-aged children and youth. Applied Physiology, Nutrition, and Metabolism, 41(6(Suppl. 3)), S266–S82. https://doi.org/10.1139/apnm-2015-0627
- Chastin, S. F. M., Palarea-Albaladejo, J., Dontje, M. L., & Skelton, D. A. (2015). Combined effects of time spent in physical activity, sedentary behaviors and sleep on obesity and cardio-metabolic health markers: A novel compositional data analysis approach. PLoS ONE, 10(10), e0139984. https://doi.org/10.1371/journal.pone.0139984
- Cole, T., Bellizzi M, Flegal K, & Dietz W. (2000). Establishing a standard definition for child overweight and obesity worldwide: International survey. BMJ, 320(7244), 1240-1240. https://doi.org/10.1136/bmj.320.7244.1240
- Cole, T., Freeman, J., & Preece, M. (1995). Body mass index reference curves for the UK, 1990. Archives of Disease in Childhood, 73(1), 25–29. https://doi.org/10.1136/adc.73.1.25
- Costigan, S. A., Barnett, L., Plotnikoff, R. C., & Lubans, D. R. (2013). The health indicators associated with screen-based sedentary behavior among adolescent girls: A systematic review. Journal of Adolescent Health, 52(4), 382–392. https://doi.org/10.1016/j.jadohealth.2012.07.018
- Daly-Smith, A., Quarmby, T., Archbold, V. S. J., Corrigan, N., Wilson, D., Resaland, G. K., Bartholomew, J. B., Singh, A., Tjomsland, H. E., Sherar, L. B., Chalkley, A., Routen, A. C., Shickle, D., Bingham, D. D., Barber, S. E., van Sluijs, E., Fairclough, S. J., & McKenna, J. (2020). Using a multi-stakeholder experience-based design process to co-develop the Creating Active Schools Framework. International Journal of Behavioral Nutrition and Physical Activity, 17(1), 13. https://doi.org/10.1186/s12966-020-0917-z
- de Greeff, J. W., Bosker, R. J., Oosterlaan, J., Visscher, C., & Hartman, E. (2018). Effects of physical activity on executive functions, attention and academic performance in preadolescent children: A meta-analysis. Journal of Science and Medicine in Sport, 21(5), 501–507. https://doi.org/10.1016/j.jsams.2017.09.595
- Department for Communities and Local Government. (2015). The english indices of deprivation. Department for Communities and Local Government: London. Retrieved June 15, 2020, from https://www.gov.uk/government/statistics/english-indices-of-deprivation-2015
- Department of Health and Social Care. (2019). UK chief medical officers’ physical activity guidelines. DHSC.
- Donnelly, J. E., Hillman, C. H., Castelli, D., Etnier, J. L., Lee, S., Tomporowski, P., Lambourne, K., & Szabo-Reed, A. N. (2016). physical activity, fitness, cognitive function, and academic achievement in children: A systematic review. Medicine & Science in Sports & Exercise, 48(6), 1197–1222. https://doi.org/10.1249/MSS.0000000000000901
- Dumuid, D., Maher, C., Lewis, L. K., Stanford, T. E., Martin Fernandez, J. A., Ratcliffe, J., Katzmarzyk, P. T., Barreira, T. V., Chaput, J.-P., Fogelholm, M., Hu, G., Maia, J., Sarmiento, O. L., Standage, M., Tremblay, M. S., Tudor-Locke, C., & Olds, T. (2018). Human development index, children’s health-related quality of life and movement behaviors: A compositional data analysis. Quality of Life Research, 27(6), 1473–1482. https://doi.org/10.1007/s11136-018-1791-x
- Dumuid, D., Pedišić, Ž., Stanford, T. E., Martín-Fernández, J.-A., Hron, K., Maher, C. A., Lewis, L. K., & Olds, T. (2019). The compositional isotemporal substitution model: A method for estimating changes in a health outcome for reallocation of time between sleep, physical activity and sedentary behaviour. Statistical Methods in Medical Research, 28(3), 846–857. https://doi.org/10.1177/0962280217737805
- Dumuid, D., Stanford, T. E., Pedišić, Ž., Maher, C., Lewis, L. K., Martín-Fernández, J.-A., Katzmarzyk, P. T., Chaput, J.-P., Fogelholm, M., Standage, M., Tremblay, M. S., & Olds, T. (2018). Adiposity and the isotemporal substitution of physical activity, sedentary time and sleep among school-aged children: A compositional data analysis approach. BMC Public Health, 18(1), 311. https://doi.org/10.1186/s12889-018-5207-1
- Ekkekakis, P. (2013). Physical activity as a mental health intervention in the era of managed care. In P. Ekkekakis (Ed.), Routldge handbook of physical activity and mental health (pp. 1–32). Routledge.
- Esliger, D. W., Rowlands, A. V., Hurst, T. L., Catt, M., Murray, P., & Eston, R. G. (2011). Validation of the GENEA Accelerometer. Medicine & Science in Sports & Exercise, 43(6), 1085–1093. https://doi.org/10.1249/MSS.0b013e31820513be
- Fairclough, S. J., Dumuid, D., Taylor, S., Curry, W., McGrane, B., Stratton, G., Maher, C., & Olds, T. (2017). Fitness, fatness and the reallocation of time between children’s daily movement behaviours: An analysis of compositional data. International Journal of Behavioral Nutrition and Physical Activity, 14(1), 64. https://doi.org/10.1186/s12966-017-0521-z
- Fairclough, S. J., Taylor, S., Rowlands, A. V., & Boddy, L. M. (2020). Cutpoint-free accelerometer metrics to assess children’s physical activity: An example using the school day. Scandinavian Journal of Medicine & Science in Sports, 30(1), 117–125. https://doi.org/10.1111/sms.13565
- Fairclough, S. J., Taylor, S., Rowlands, A. V., Boddy, L. M., & Noonan, R. J. (2019). Average acceleration and intensity gradient of primary school children and associations with indicators of health and well-being. Journal of Sports Sciences, 37(18), 2159–2167. https://doi.org/10.1080/02640414.2019.1624313
- Goodman, A., Lamping, D. L., & Ploubidis, G. B. (2010). When to use broader internalising and externalising subscales instead of the hypothesised five subscales on the Strengths and Difficulties Questionnaire (SDQ): Data from British parents, teachers and children. Journal of Abnormal Child Psychology, 38(8), 1179–1191. https://doi.org/10.1007/s10802-010-9434-x
- Goodman, R. (2001). Psychometric properties of the strengths and difficulties questionnaire. Journal of the American Academy of Child & Adolescent Psychiatry, 40(1), 1337–1345. https://doi.org/10.1097/00004583-200111000-00015
- Government Statistical Service. (2018). Mental Health of Children and Young People in England, 2017. Retrieved June 15, 2020, from https://files.digital.nhs.uk/A6/EA7D58/MHCYP%202017%20Summary.pdf
- Hildebrand, M., Van Hees, V. T., Hansen, B. H., & Ekelund, U. (2014). Age-group comparibility of raw accelerometer output from wrist- and hip-worn monitors. Medicine & Science in Sports & Exercise, 46(9), 1816–1824. https://doi.org/10.1249/MSS.0000000000000289
- Hilland, T. A., & Costigan, S. A. (2020). Children’s and adolescents’ interpersonal-level correlates of physical activity behavior. In T. A. Brusseau, S. J. Fairclough, & D. R. Lubans (Eds.), The routledge handbook of youth physical activity (pp. 191–212). Routledge.
- Hoffmann, B., Kobel, S., Wartha, O., Kettner, S., Dreyhaupt, J., & Steinacker, J. M. (2019). High sedentary time in children is not only due to screen media use: A cross-sectional study. BMC Pediatrics, 19(1), 154. https://doi.org/10.1186/s12887-019-1521-8
- Hron, K., Filzmoser, P., Caritat, P., Fiserov·, E., & Gardlo, A. (2017). Weighted pivot coordinates for compositional data and their application to geochemical mapping. Mathematical Geosciences, 49(6), 797–814. https://doi.org/10.1007/s11004-017-9684-z
- Hurter, L., Fairclough, S. J., Knowles, Z., Porcellato, L., Cooper-Ryan, A., & Boddy, L. (2018). Establishing raw acceleration thresholds to classify sedentary and stationary behaviour in children. Children, 5(12), 172. https://doi.org/10.3390/children5120172
- Inchley, J., Currie, D., Budisavljevic, S., Torsheim, T., Jåstad, A., Cosma, A., Kelly, C., Arnarsson, A., and Samdal, O. (2020). Spotlight on adolescent health and well-being. Findings from the 2017/2018 Health Behaviour in School-aged Children (HBSC) survey in Europe and Canada. International report. Volume 1. Key Findings. WHO Regional Office for Europe
- Kovess-Masfety, V., Husky, M. M., Keyes, K., Hamilton, A., Pez, O., Bitfoi, A., Carta, M. G., Goelitz, D., Kuijpers, R., Otten, R., Koç, C., Lesinskiene, S., & Mihova, Z. (2016). Comparing the prevalence of mental health problems in children 6-11 across Europe. Social Psychiatry and Psychiatric Epidemiology, 51(8), 1093–1103. https://doi.org/10.1007/s00127-016-1253-0
- Kuznetsova, A., Brockhoff, P. B., & Christensen, R. H. B. (2017). lmerTest package: Tests in linear mixed effects models. Journal of Statistical Software, 82(13), 26. https://doi.org/10.18637/jss.v082.i13
- Lawrence, D., Johnson, S., Hafekost, J., Boterhoven De Haan, K., Sawyer, M., Ainley, J., and Zubrick, S. (2015). The mental health of children and adolescents. Report of the second Australian child and adolescent survey of mental health and wellbeing. Department of Health
- Lubans, D., Richards, J., Hillman, C., Faulkner, G., Beauchamp, M., Nilsson, M., Kelly, P., Smith, J., Raine, L., & Biddle, S. (2016). Physical activity for cognitive and mental health in youth: A systematic review of mechanisms. Pediatrics, 138(3), e20161642. https://doi.org/10.1542/peds.2016-1642
- Luciana, M., & Nelson, C. A. (2002). Assessment of neuropsychological function through use of the Cambridge Neuropsychological Testing Automated Battery: Performance in 4- to 12-year-old children. Developmental Neuropsychology, 22(3), 595–624. https://doi.org/10.1207/S15326942DN2203_3
- Meltzer, H., Gatward, R., Goodman, R., & Ford, T. (2003). Mental health of children and adolescents in Great Britain. International Review of Psychiatry, 15(1–2), 185–187. https://doi.org/10.1080/0954026021000046155
- Migueles, J. H., Cadenas-Sanchez, C., Rowlands, A. V., Henriksson, P., Shiroma, E. J., Acosta, F. M., Rodriguez-Ayllon, M., Esteban-Cornejo, I., Plaza-Florido, A., Gil-Cosano, J. J., Ekelund, U., van Hees, V. T., & Ortega, F. B. (2019). Comparability of accelerometer signal aggregation metrics across placements and dominant wrist cut points for the assessment of physical activity in adults. Scientific Reports, 9(1), 18235. https://doi.org/10.1038/s41598-019-54267-y
- Migueles, J. H., Rowlands, A. V., Huber, F., Sabia, S., & Van Hees, V. T. (2019). GGIR: A research community–driven open source r package for generating physical activity and sleep outcomes from multi-day raw accelerometer data. Journal for the Measurement of Physical Behaviour, 2(3), 188–196. https://doi.org/10.1123/jmpb.2018-0063
- Ministry of Housing, Communiities, and Local Government. (2019). English Indices of Deprivation 2019. Retrieved June 15, 2020, from http://imd-by-postcode.opendatacommunities.org/imd/2019
- Miyake, A., Friedman, N. P., Emerson, M. J., Witzki, A. H., Howerter, A., & Wager, T. D. (2000). The unity and diversity of executive functions and their contributions to complex “frontal lobe” tasks: A latent variable analysis. Cognitive Psychology, 41(1), 49–100. https://doi.org/10.1006/cogp.1999.0734
- Morris, J. L., Daly-Smith, A., Archbold, V. S. J., Wilkins, E. L., & McKenna, J. (2019). The Daily Mile™ initiative: Exploring physical activity and the acute effects on executive function and academic performance in primary school children. Psychology of Sport and Exercise, 45, 101583. https://doi.org/10.1016/j.psychsport.2019.101583
- New Zealand Ministry of Health. (2017). New Zealand Physical Activity Guidelines. Retrieved June 15, 2020, from https://www.health.govt.nz/our-work/preventative-health-wellness/physical-activity#kids
- Okely, A. D., Batterham, M. J., Hinkley, T., Ekelund, U., Brage, S., Reilly, J. J., TROST, S. G., JONES, R. A., JANSSEN, X., CLIFF, D. P., & VAN Loo, C. M. T. (2018). Wrist acceleration cut points for moderate-to-vigorous physical activity in youth. Medicine & Science in Sports & Exercise, 50(3), 609–616. https://doi.org/10.1249/MSS.0000000000001449
- Parkes, A., Sweeting, H., Wight, D., & Henderson, M. (2013). Do television and electronic games predict children’s psychosocial adjustment? Longitudinal research using the UK Millennium Cohort Study. Archives of Disease in Childhood, 98(5), 341–348. https://doi.org/10.1136/archdischild-2011-301508
- Paruthi, S., Brooks, L. J., D’Ambrosio, C., Hall, W. A., Kotagal, S., Lloyd, R. M., Malow, B. A., Maski, K., Nichols, C., Quan, S. F., Rosen, C. L., Troester, M. M., & Wise, M. S. (2016). Recommended amount of sleep for pediatric populations: A consensus statement of the American academy of sleep medicine. Journal of Clinical Sleep Medicine, 12(6), 785–786. https://doi.org/10.5664/jcsm.5866
- Reiss, F. (2013). Socioeconomic inequalities and mental health problems in children and adolescents: A systematic review. Social Science & Medicine, 90, 24–31. https://doi.org/10.1016/j.socscimed.2013.04.026
- Rhodes, R. E., Guerrero, M. D., Vanderloo, L. M., Barbeau, K., Birken, C. S., Chaput, J.-P., Faulkner, G., Janssen, I., Madigan, S., Masse, L., McHugh, T-L., Perdew, M., Stone, K., Shelley, J., Spinks, N., Tamminen, K.A., Tomosone, J.R., ward, H., Welsh, F., and Tremblay, M.S.. (2020). Development of a consensus statement on the role of the family in the physical activity, sedentary, and sleep behaviours of children and youth. The International Journal of Behavioral Nutrition and Physical Activity, 17(1), 74. https://doi.org/10.1186/s12966-020-00973–0
- Rich, C., Geraci, M., Griffiths, L., Sera, F., Dezateux, C., Cortina-Borja, M., & Dasgupta, K. (2013). Quality control methods in accelerometer data processing: Defining minimum wear time. PLoS ONE, 8(6), e67206. https://doi.org/10.1371/journal.pone.0067206
- Rodriguez-Ayllon, M., Cadenas-Sánchez, C., Estévez-López, F., Muñoz, N. E., Mora-Gonzalez, J., Migueles, J. H., Molina-García, P., Henriksson, H., Mena-Molina, A., Martínez-Vizcaíno, V., Catena, A., Löf, M., Erickson, K. I., Lubans, D. R., Ortega, F. B., & Esteban-Cornejo, I. (2019). Role of physical activity and sedentary behavior in the mental health of preschoolers, children and adolescents: A systematic review and meta-analysis. Sports Medicine, 49(9), 1383–1410. https://doi.org/10.1007/s40279-019-01099-5
- Rosenberg, M. (1965). Society and the adolescent self-image. Princeton University Press.
- Rowlands, A. V. (2018). Moving Forward with accelerometer-assessed physical activity: Two strategies to ensure meaningful, interpretable, and comparable measures. Pediatric Exercise Science, 30(4), 450–456. https://doi.org/10.1123/pes.2018-0201
- Rowlands, A. V., Mirkes, E. M., Yates, T., Clemes, S., Davies, M., Khunti, K., & EDWARDSON, C. L. (2018). Accelerometer-assessed physical activity in epidemiology: Are monitors equivalent? Medicine & Science in Sports & Exercise, 50(2), 257–265. https://doi.org/10.1249/MSS.0000000000001435
- Rowlands, A. V., Plekhanova, T., Yates, T., Mirkes, E. M., Davies, M., Khunti, K., & Edwardson, C. L. (2019). Providing a basis for harmonization of accelerometer-assessed physical activity outcomes across epidemiological datasets. Journal for the Measurement of Physical Behaviour, 2(3), 131–142. https://doi.org/10.1123/jmpb.2018-0073
- Sampasa-Kanyinga, H., Colman, I., Goldfield, G. S., Janssen, I., Wang, J., Podinic, I., Tremblay, M. S., Saunders, T. J., Sampson, M., & Chaput, J.-P. (2020). Combinations of physical activity, sedentary time, and sleep duration and their associations with depressive symptoms and other mental health problems in children and adolescents: A systematic review. International Journal of Behavioral Nutrition and Physical Activity, 17(1), 72. https://doi.org/10.1186/s12966-020-00976-x
- Saunders, T. J., Gray, C. E., Poitras, V. J., Chaput, J.-P., Janssen, I., Katzmarzyk, P. T., Olds, T., Connor Gorber, S., Kho, M. E., Sampson, M., Tremblay, M. S., & Carson, V. (2016). Combinations of physical activity, sedentary behaviour and sleep: Relationships with health indicators in school-aged children and youth. . Applied Physiology, Nutrition, and Metabolism, 41(6(Suppl. 3)), S283–S93. https://doi.org/10.1139/apnm-2015-0626
- Short, M. A., Blunden, S., Rigney, G., Matricciani, L., Coussens, S. M., Reynolds, C., & Galland, B. (2018). Cognition and objectively measured sleep duration in children: A systematic review and meta-analysis. Sleep Health, 4(3), 292–300. https://doi.org/10.1016/j.sleh.2018.02.004
- Singh, A. S., Saliasi, E., van den Berg, V., Uijtdewilligen, L., de Groot, R. H. M., Jolles, J., Andersen, L. B., Bailey, R., Chang, Y.-K., Diamond, A., Ericsson, I., Etnier, J. L., Fedewa, A. L., Hillman, C. H., McMorris, T., Pesce, C., Pühse, U., Tomporowski, P. D., & Chinapaw, M. J. M. (2019). Effects of physical activity interventions on cognitive and academic performance in children and adolescents: A novel combination of a systematic review and recommendations from an expert panel. British Journal of Sports Medicine, 53(10), 640–647. https://doi.org/10.1136/bjsports-2017-098136
- Stanczykiewicz, B., Banik, A., Knoll, N., Keller, J., Hohl, D. H., Rosińczuk, J., & Luszczynska, A. (2019). Sedentary behaviors and anxiety among children, adolescents and adults: A systematic review and meta-analysis. BMC Public Health, 19(1), 459. https://doi.org/10.1186/s12889-019-6715-3
- Sterne, J. A. C., White, I. R., Carlin, J. B., Spratt, M., Royston, P., Kenward, M. G., Wood, A. M., & Carpenter, J. R. (2009). Multiple imputation for missing data in epidemiological and clinical research: Potential and pitfalls. BMJ, 338(jun29 1), b2393. https://doi.org/10.1136/bmj.b2393
- Stiglic, N., & Viner, R. M. (2019). Effects of screentime on the health and well-being of children and adolescents: A systematic review of reviews. BMJ Open, 9(1), e023191. https://doi.org/10.1136/bmjopen-2018-023191
- Suchert, V., Hanewinkel, R., & Isensee, B. (2015). Sedentary behavior and indicators of mental health in school-aged children and adolescents: A systematic review. Preventive Medicine, 76, 48–57. https://doi.org/10.1016/j.ypmed.2015.03.026
- Talarico, R., & Janssen, I. (2018). Compositional associations of time spent in sleep, sedentary behavior and physical activity with obesity measures in children. International Journal of Obesity, 42(8), 1508–1514. https://doi.org/10.1038/s41366-018-0053-x
- Taylor, R. W., Haszard, J. J., Farmer, V. L., Richards, R., Te Morenga, L., Meredith-Jones, K., and Mann, J.I. (2019). Do differences in compositional time use explain ethnic variation in the prevalence of obesity in children? Analyses using 24-hour accelerometry. International Journal of Obesity, 4(1), 94–103. https://doi.org/10.1038/s41366-019-0377–1
- Templ, M., Hron, K., & Filzmoser, P. (2011). robCompositions: An R-package for robust statistical analysis of compositional data. In V. Pawlowsky-Glahn & A. Buccianti (Eds.), Compositional data analysis: Theory and applications (pp. 341–355). John Wiley & Sons, Ltd.
- Teychenne, M., White, R. L., Richards, J., Schuch, F. B., Rosenbaum, S., & Bennie, J. A. (2020). Do we need physical activity guidelines for mental health: What does the evidence tell us? Mental Health and Physical Activity, 18, 100315. https://doi.org/10.1016/j.mhpa.2019.100315
- Tremblay, M. S., Aubert, S., Barnes, J. D., Saunders, T. J., Carson, V., Latimer-Cheung, A., Chastin, S. F. M., Altenburg, T. M., & Chinapaw, M. J. M. (2017). Sedentary behavior research network (SBRN) – terminology consensus project process and outcome. International Journal of Behavioral Nutrition and Physical Activity, 14(1), 75. https://doi.org/10.1186/s12966-017-0525-8
- Twenge, J. M., & Campbell, W. K. (2018). Associations between screen time and lower psychological well-being among children and adolescents: Evidence from a population-based study. Preventive Medicine Reports, 12, 271–283. https://doi.org/10.1016/j.pmedr.2018.10.003
- van den Boogaart, K. G., & Tolosana-Delgado, R. (2008). ‘Compositions’: A unified R package to analyze compositional data. Computers & Geosciences, 34(4), 320–338. https://doi.org/10.1016/j.cageo.2006.11.017
- van Hees, V. T., Fang, Z., Langford, J., Assah, F., Mohammad, A., da Silva, I. C., Trenell, M. I., White, T., Wareham, N. J., & Brage, S. (2014). Autocalibration of accelerometer data for free-living physical activity assessment using local gravity and temperature: An evaluation on four continents. Journal of Applied Physiology, 117(7), 738–744. https://doi.org/10.1152/japplphysiol.00421.2014
- van Hees, V. T., Gorzelniak, L., León, E. C., Eder, M., Pias, M., Taherian, S., Ekelund, U., Renström, F., Franks, P. W., Horsch, A., & Brage, S. (2013). Separating movement and gravity components in an acceleration signal and implications for the assessment of human daily physical activity. PLoS ONE, 8(4), e61691. https://doi.org/10.1371/journal.pone.0061691
- van Hees, V. T., Sabia, S., Jones, S. E., Wood, A. R., Anderson, K. N., Kivimäki, M., Frayling, T.M., Pack, A.I., Bucan, M., Trenell, M.I., Mazzotti, D.R., Gehrman, P.R., Singh-Manoux, B.A., and Weedon, M.N. (2018). Estimating sleep parameters using an accelerometer without sleep diary. Scientific Reports, 8(1), 12975. https://doi.org/10.1038/s41598-018-31266–z
- Weatherson, K., Gierc, M., Patte, K., Qian, W., Leatherdale, S., & Faulkner, G. (2020). Complete mental health status and associations with physical activity, screen time, and sleep in youth. Mental Health and Physical Activity, 19, 100354. https://doi.org/10.1016/j.mhpa.2020.100354
- WHO. (2019). Adolescent mental health. Retrieved June 15, 2020, from https://www.who.int/news-room/fact-sheets/detail/adolescent-mental-health