ABSTRACT
Machine learning is a category of AI technology that is utilized in the product development cycle in numerous industries. It was reported that machine learning can be applied to predict the chemical properties of functional materials, for example, the self-assembly of surfactant solutions (Nanoscale, 2018, 10, 16013) and the chemical reactions of copper nanoparticles (ACS Energy Lett., 2018, 3, 2983). A liquid crystal (LC) molecule is a representative functional material. Monodisperse systems have been targeted in many theoretical studies; however, the resulting products usually have a polydisperse distribution. It has been reported in several studies that the properties exhibited by polydisperse systems are different from those observed in monodisperse systems. In this study, we focus on the physical properties of polydisperse systems i.e. the ratio of binary LC molecules on the phase transition temperature and the possibility of predicting the physical properties of the system using machine learning. A relatively high prediction accuracy was obtained using machine learning. This study demonstrates that machine learning can be utilized to predict the phase transition temperature of polydispersed LC systems. The results of this study will contribute to the further development of material science and molecular design via the application of machine learning.
Graphical Abstract
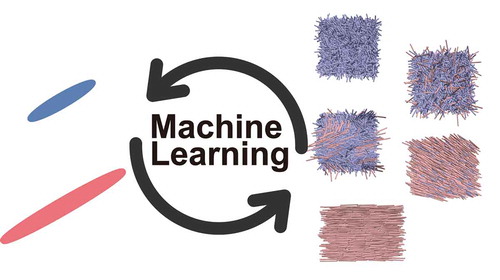
Disclosure statement
No potential conflict of interest was reported by the authors.