ABSTRACT
It is long known that facial configurations play a critical role when inferring mental and emotional states from others. Nevertheless, there is still a scientific debate on how we infer emotions from facial configurations. The theory of constructed emotion (TCE) suggests that we may infer different emotions from the same facial configuration, depending on the context (e.g. provided by visual and lexical cues) in which they are perceived. For instance, a recent study found that participants were more accurate in inferring mental and emotional states across three different datasets (i.e. RMET, static and dynamic emojis) when words were provided (i.e. forced-choice task), compared to when they were not (i.e. free-labelling task), suggesting that words serve as contexts that modulate the inference from facial configurations. The goal of the current within-subject study was to replicate and extend these findings by adding a fourth dataset (KDEF-dyn), consisting of morphed human faces (to increase the ecological validity). Replicating previous findings, we observed that words increased accuracy across the three (previously used) datasets, an effect that was also observed for the facial morphed stimuli. Our findings are in line with the TCE, providing support for the importance of contextual verbal cues in emotion perception.
Sayings like “the face is the picture of the mind”Footnote1 exemplify that faces are a rich source of information important for social interactions (Barrett et al., Citation2019; Todorov, Citation2017; Zhang et al., Citation2019). What we “read out” from someone’s face has a strong influence on what we infer about a person’s emotional and mental state (Barrett et al., Citation2019; Lindquist & Gendron, Citation2013). However, these inferences may not only be based on the mere specific facial configuration, but also on available information such as contextual (visual or lexical) cues or a priori expectations. In the current replication study, we aimed at investigating how the inference of mental and emotional states from face-like configurations across various stimulus sets is modulated by the information made available by comparing performance between tasks in which (mental- and emotion-related) words were embedded as anchors (forced-choice task) or not (free-labelling task).
In the field of face perception, the relation between facial configurations and emotions has received special attention (Barrett et al., Citation2019; Lindquist & Gendron, Citation2013). There are currently two prominent theoretical views on the relation between facial configurations and emotions that can be embedded within the theory of basic emotions and the theory of constructed emotion (TCE; see also context-sensitive hypothesis; [Le Mau et al., Citation2021]). For the last decades, emotion perception research has been mostly influenced by the theory of basic emotions (Barrett et al., Citation2019; Gendron & Feldman Barrett, Citation2009), which assumes that certain (i.e. basic) emotions are biologically innate as a result of evolutionary adaptation (e.g. Ekman & Cordaro, Citation2011). According to this view, when a basic emotion is evoked, a cascade of fast, automatic, and unique physiological (Ekman et al., Citation1983) and behavioural changes occur, including consistent and reliable facial configurations that are unique for each emotion (Ekman & Cordaro, Citation2011). Even though this theory assumes that culture may produce variation in the facial configuration associated to each emotion, a prototypical facial pattern is expected to remain stable beyond ontogenetic influences (Ekman & Friesen, Citation1971). The theory of basic emotions was inspired by earlier cross-cultural studies on emotion perception (Ekman, Citation1972; Ekman & Friesen, Citation1971; Ekman et al., Citation1969, Citation1980). In some of these studies, Ekman and colleagues used the so-called forced-choice task to investigate whether people inferred similar emotions from specific facial configurations independently of their cultural backgrounds (e.g. Ekman et al., Citation1969). In this task, participants were presented with “culture-free” pictures of posed facial configurations (e.g. a scowling face) and were instructed to choose from an array of six (basic) emotion words (i.e. surprise, happiness, anger, sadness, disgust, and fear) the one that best described the facial configuration. Ekman and colleagues observed that participants across cultures were able to match emotion words and target facial configurations above chance level, which led them to conclude the existence of universal (pan-cultural) elements of emotions in facial configurations (Ekman et al., Citation1987; Ekman & Cordaro, Citation2011).
The TCE, on the other hand, states that emotions are not innate, but rather abstract entities constructed based on instances accumulated throughout life experiences (Barrett, Citation2017a, Citation2017b). In turn, instances differing in their physical attributes or statistical regularities are considered as part of the same emotion category given their similarities within more abstract representations. Because behavioural, – and also physiological (Hoemann et al., Citation2020; see also Ventura-Bort et al., Citation2022) – responses may depend on the physical demands of the evoking event (e.g. location, surrounding people), as well as on the individual’s personal and cultural backgrounds (Barrett et al., Citation2011), facial configurations of the same emotion may differ from instance to instance. Constructionism assumes that facial configurations, although important, are not sufficient to make inferences about someone’s emotional states (Barrett et al., Citation2019; Lindquist & Gendron, Citation2013). Which emotional state is inferred from facial configurations will be determined, among other aspects, by the context in which they are perceived (Barrett, Citation2017b). Strong support for the importance of context comes from a series of studies by Aviezer et al. (Citation2008), in which stereotypical facial configurations of some “basic” emotions (e.g. disgust) were paired with different emotional contextual cues (e.g. a dirty diaper, a fist about to punch, a grave, a posing bodybuilder body) that could match with the target face or not. Participants in these studies were instructed to select, out of an array of emotion words, the one that best described the facial configuration. The authors observed that when the target face and context matched (e.g. stereotypical face of disgust and dirty diaper context), participants selected the expected word (i.e. disgust). However, when the face/context did not match (e.g. stereotypical face of disgust attached to a posing bodybuilder body), participants’ rarely chose the appropriate word expected from the facial configuration. Instead, participants’ choice was strongly dependent on the context (e.g. pride-related context), in which it was presented.
Importantly, not only visual, but also lexical cues can provide contextual information. From a constructionist view, words are not a mere expression of a mental representation, but they can help binding together in one category different instances that share few physical similarities (Barrett, Citation2006; Barrett et al., Citation2011; Lindquist et al., Citation2015; Lindquist & Gendron, Citation2013). For instance, different facial configurations such as a face with a bitter smile from the student who just received an unfair grade, or the scowl of a teacher responding to a misbehaving pupil can be grouped together through the word anger. Consequently, words can have a reverse effect, if they are made available, they may serve as contexts to categorise what otherwise may be ambiguous information. When the word anger (and its associated conceptual knowledge) becomes active, perceived facial configurations (e.g. a scowling face, a bitter smile) may be identified as facial expressions of anger (Fugate, Citation2013; Lindquist, Citation2017; Lindquist et al., Citation2015; Lindquist & Gendron, Citation2013)
Growing evidence has provided support for the role of words in categorising and contextualising facial configurations as instances of emotions in both infants and adults (Ruba et al., Citation2017, Citation2018; Russell & Widen, Citation2002; see for review Ruba & Pollak, Citation2020). In one study, Nook et al. (Citation2015) observed that words in an emotion perception task facilitated the perception of emotions from facial configurations (see also, Doyle et al., Citation2021). Relatedly, discrimination of two facial configurations that differed in intensity of expression was more difficult when they were primed with an emotion word, compared to a control word because the emotion word helped categorising them as part of the same emotion concept (Fugate et al., Citation2018; see also Fugate et al., Citation2010). In a related fashion, it has been observed that disrupting the access to conceptual information of emotions either by semantic satiation (Gendron et al., Citation2012; Lindquist et al., Citation2006), time constraints (Ruba et al., Citation2018), or due to neurodegenerative damage (Lindquist et al., Citation2014) diminishes emotion categorisation in emotion perception tasks.
Similarly, the presence of words in forced-choice tasks may also influence emotion perception (see Barrett et al., Citation2019 for an extended revision of the task). Anchored emotion words used in such tasks may provide a context that biases the inference of emotional states, making some states more likely to be inferred than others. Initial evidence in this regard comes from a study by Gendron and colleagues (Gendron et al., Citation2014). Participants from the United States and the Himba ethnic group from Namibia were instructed to sort posing faces of stereotypical facial expressions of the six basic emotions into piles based on emotion type. The sorting was carried out either in the presence or absence of anchored words. The authors observed that sorted piles were closer to the expected “universal” pattern (based on the theory of basic emotions) when anchored words were made available. Another piece of evidence comes from a recent study by Betz and colleagues (Citation2019). In this study, participants completed the Reading the Mind in the Eyes Test (RMET; Baron-Cohen et al., Citation1997) or viewed static and dynamic facial emojis while instructed to choose a word that best described the mental or emotional state of the target visual stimuli by either selecting an option from the available array of words (forced-choice task) or typing it in (free-labelling task). The effects of words on emotion perception were tested by comparing the accuracy – as measured by the level of agreement with the experimenters’ expectations – between tasks. Results showed that overall accuracy was higher in the forced-choice than in the free-labelling condition for the three stimulus sets, indicating that words modulate emotion perception from visual material, providing further evidence for the contextual effects of words in the forced-choice task when inferring emotions.
It must be noted that, although the stimulus sets used by Betz and colleagues (Citation2019) have been commonly employed in emotion perception tasks (e.g. Oakley et al., Citation2016) or were designed to facilitate emotion communication in online settings (Zolli, Citation2015), they did not show complete human faces, but rather sections of it or exaggerated facial traits. Thus, despite the robustness of the findings, it is unclear whether the same effects extend to real human configurations. Also, in Betz and colleagues’ study (Citation2019) tasks were performed by American, English-speaking participants. There is remarkable evidence that culture shapes the meaning of our emotions and the conceptual knowledge attached to emotion words (Kollareth et al., Citation2018; Mesquita et al., Citation2016). Such cultural differences exist between eastern and western societies, but also within westernised countries (Kitayama et al., Citation2005; Koopmann-Holm & Tsai, Citation2014; Scheibe et al., Citation2011) and they become even more pronounced when participants are not provided with contextual cues such as anchored words (e.g. Gendron et al., Citation2014). Keeping this in mind, the current study may also help expanding Betz et al.’s study (Citation2019) by testing whether the modulatory effects of words in emotion perception tasks can be replicated in another culture with a different language. Finally, it should also be pointed out that Betz and colleagues (Citation2019) used a between-subject design to compare the forced-choice and free-labelling tasks, leaving the possibility that the results were partly influenced by variations between samples. The aim of the current study was therefore to conceptually replicate and extend the findings by Betz et al. (Citation2019). In a within-subject design, we tested emotion perception using a free-labelling and forced-choice task in a German-speaking sample. In addition to the three stimulus sets used by Betz et al. (Citation2019), we added a fourth – more ecologically valid – stimulus set (KDEF-dyn; Calvo et al., Citation2018), consisting of morphed human faces varying from neutral expressions to stereotypical emotional expressions (anger, disgust, fear, happiness, sadness, surprise). We expected to replicate the overall effects by Betz et al. (Citation2019) showing higher accuracy when emotion words were presented as anchors (i.e. forced-choice > free-labelling). Furthermore, if the contextual effects of words also extend to full human faces, similar task effects should be observed for morphed human faces.
1. Methods
1.1. Participants
The sample size was estimated using G*Power (Faul et al., Citation2007, Citation2009) and calculated for two-tailed, paired comparisons (α = 0.05, power of 1-β = .80), based on the effect sizes reported by Betz et al., (Citation2019) for more conservative analysis (e.g. conceptual code scheme; median odds ratio = 1.77). The outcome of this analysis revealed an estimated sample size of N = 82 participants. We, however, decided to collect data from a few more participants in case the quality of the data was compromised. A total of 88 participants (67 female, 20 male, 1 diverse, age M = 24.12, SD = 4.72) took part in the online study, which was conducted via soscisurvey.de (Leiner, Citation2019). Participants were mostly students from the University of Potsdam (N = 78). Each individual provided informed consent for a procedure in accordance with a study protocol approved by the Ethics Committee of the University of Potsdam. Nine individuals were excluded from analysis because they did not complete the experiment. Individuals who reported one or more of the following criteria were also excluded (N = 13): German proficiency level lower than C1 (i.e. advanced level), history of neurological disorder, undergoing psychological treatment at the moment of the study or having suffered any psychological disorder during the previous year, and taking chronic or acute psychiatric drugs. Furthermore, the speed of completion of the online task was also checked (Leiner, Citation2019). Participants who completed the survey double as fast as the sample mean time of completion (N = 2) were further excluded (Leiner, Citation2019). The final sample consisted of 64 participants.
1.2. Materials
1.2.1. Reading the Mind in the Eyes Test
The Reading the Mind in the Eyes Test (RMET) (Baron-Cohen et al., Citation1997, Citation2001) has been created to measure individual differences in theory of the mind abilities (Baron-Cohen et al., Citation2001) and shown to discriminate between people with autistic traits and neurotypical controls (Baron-Cohen et al., Citation1997). Lately, this task has also been used as an emotion recognition task (Oakley et al., Citation2016). The RMET consists of 36 black-and-white pictures of the eye region of different actors and actresses posing a facial configuration of a mental state. The photos only display the eyes, the eyebrows, and part of the nose of the actors and actresses. For the forced-choice condition, the standard administration of the RMET (i.e. adapted German version; Bölte, Citation2005) was adopted. In this version, each of thirty-six trials consisted of a picture and four mental words used as anchored options. Participants were instructed to select the anchored word that best describes the mental state of the person of the picture. On each trial, participants were reminded of the definition of mental state (i.e. Mental states can be used to describe how a person is feeling, their attitudes toward something, or what they are thinking). For the free-labelling condition, the same trial structure was used. Instead of presenting the four anchored words, participants were presented with a gap and instructed to write down the mental state that fitted best with the picture.
1.2.2. Static emoji (Finch) set
The Finch set was developed for Facebook by Pixar illustrator Matt Jones (Jones, Citation2017) and psychologist Dacher Keltner. The design of this set was based on Darwin’s book, The Expression of the Emotions in Man and Animals (Darwin, Citation2005). The set consists of 16 facial configurations, each expressing sixteen different emotions by a “Finch” character with a round, freestanding yellow face with mouth, eyes, eyelids, eyebrows, and wrinkles. The Finch set contains the following emotions: admiration, amusement, anger, awe, boredom, confusion, disgust, embarrassment, excitement, gratitude, happiness, love, sadness, shyness, surprise, and sympathy. Of note, four emoji (anger, confusion, love, sadness) were originally created with embedded symbols to help conveying the expressed emotions. Symbolic elements that were not part of the facial expressions were removed to avoid confounding effects (see also supplementary material by Betz et al., Citation2019). In the forced-choice condition, each of the sixteen trials consisted of an emoji accompanied by sixteen emotional words as anchored options (anchored words were initially translated by the authors and validated by German independent researchers with expertise in the field of emotions). In the free-labelling task, no anchored words were presented. Participants were instructed to select (forced-choice condition) or write down (free-labelling condition) the emotional state that best reflected the emoji.
1.2.3. Dynamic emoji (Finch) set
In the animated version of the Finch set, expressions portray a dynamic movement (e.g. laughing to show happiness) for each of the 16 different emotions. These movements are clipped in 6- to 11-second videos. On each trial, participants were instructed to press the play button to see the emoji video. The emoji clip was repeated twice before pausing but participants were allowed to repeat the video if necessary. Trial structure, conditions, and instructions were identical to the static emoji set condition. Some of the animated trials (Finch expressions of anger, confusion, love, sadness) contained symbolic elements (anger: smoke coming out of the ears and changes in the colour of the emoji (yellow to orange); confusion: hand pointing to the forehead; love: read heart; sadness: a tear dropping) and were not considered for the analysis (see also Betz et al., Citation2019).
1.2.4. The Karolinska Directed Emotional Faces dynamic (KDEF-dyn)
In addition to the three stimulus sets used by Betz et al. (Citation2019), we also added a fourth one, which consisted of a series of dynamic, human facial expressions extracted from the KDEF-dyn (Calvo et al., Citation2018). The KDEF-dyn dataset contains a series of video-clips (each with a length of 1033 ms), portraying the posed expression of six emotions: happiness, anger, fear, sadness, disgust, and surprise. Each video starts with a neutral expression and dynamically changes until a full emotional expression is reached. For the current experiment, a total of 24 clips (i.e. four for each emotion) were selected. In these clips, emotions were portrayed by 12 different models (6 females and 6 males; two emotions were portrayed by each model). That is each emotion was rated 4 times (twice portrayed by female actresses and twice by male actors). We decided to use the current set based on prior empirical evidence showing that emotions were more easily inferred from morphed than from static faces (Calvo et al., Citation2018). We presented four instances of the same emotion to avoid any potential confounding effects attributed to the gender or identity of the posing person. On each trial, participants were instructed to press the play button to see the video (it could be repeated if necessary). In the forced-choice condition, each of the 24 trials was accompanied by the six emotional words as anchored options (as for the emoji sets, anchored words were initially translated by the authors and validated by German independent researchers with expertise in the field of emotions), whereas in the free-labelling condition no word was presented. Participants were instructed to select or write down the emotional state that reflected the human facial expression best.
1.3. Procedure
The online study took place in one single session that lasted about 45 min. Prior to the tasks, participants filled out a demographic questionnaire. To avoid potential carry-over effects of the anchored words, the free-labelling condition was always completed first. The order of the four datasets was counterbalanced across participants. For each participant, datasets were presented in the same order in both conditions (free-labelling and forced-choice). Within each dataset, the trial order was fully randomised. Participants had to provide a response in each trial in order to continue with the experiment. Although there was no time limit, participants were instructed to respond without much delay.
1.4. Data coding
To determine whether participants’ inferences from face-like stimuli were right or wrong, different assumptions are needed to be met. One important assumption is that the actual emotion or mental state that actors and actresses were experiencing matches with the facial expression (e.g. they are happy when they smile). Given that we could neither confirm that actors and actresses form the KDEF-dyn set and RMET were experiencing the emotion or mental state that they were supposed to express, nor assume that emojis felt any emotion, given that they are fictional creations, participants’ inferences could not be objectively judged as right or wrong. Therefore, we needed to define criteria to determine response accuracy. These criteria (i.e. experimenters’ expectations) were based on the purpose of the stimulus sets used. That is, if participants’ inference matched with the emotion or mental state that the stimuli were originally meant to represent, we considered it a match, e.g. inferring embarrassment from the emoji designed to express such emotion.
In the free-labelling condition, two coding schemes were used to identify matches: the semantic- and the conceptual-based scheme. In the semantic-based scheme, a response was considered a match if the given answer matched with the target emotion or was listed as a synonym of it (e.g. for the emotion Glück [happiness], the following words were considered a match: glücklich, fröhlich, zufrieden, freudig, froh, Freude). In the conceptual-based scheme, a response was considered a match if the given answer was in line with the target emotion, was listed as a synonym or was part of the list of related words (e.g. for the emotion Glück [happiness], the following words were considered a match: glücklich, fröhlich, zufrieden, freudig, froh, Freude, entzückt, angenehm, erheitert, heiter, selig, sonnig, strahlend, glückselig, gut gelaunt, vorfreudig; the lists of synonym and related words of each target emotion are available Section S1 and S2 of supplementary material). The lists of synonyms and related words were created based on two online German dictionaries: Open Thesaurus (https://www.openthesaurus.de), and Duden (https://www.duden.de). Two independent researchers classified the answers based on these lists. If an answer matched with the target emotion, the answer was coded with the name of the target emotion. For instance, if the emoji displaying anger was labelled as “zufrieden”, the coding for that answer was “happy”. If an answer did not match with the target emotion, the answer was coded with the name of the closest emotion from the remaining emotions of the set. For instance, if the anger emoji was labelled as “Aufgeregtheit”, the coding for that answer was “excitement”. If the answer was a slang expression, a description of the picture (i.e. a cry, smile) and/or a word not related to emotional or mental states, it was coded as “other”. Similarly, if the answer did not match with the target emotion and could not be identified with any of the remaining emotions, the answer was coded as “other”. If multiple responses (i.e. more than one word) were given, only the closest-related word to the target emotion was considered (i.e. synonyms were considered closer than related words). In the case of no match of the multiple responses with the target emotion, only the closest-related word to one of the remaining emotion words from the set was considered. Ambiguous answers as well as coding inconsistencies between researchers were discussed and resolved one by one. After coding each answer, responses were assigned as a match (1) or as a mismatch (0).
1.5. Data analysis
1.5.1. Statistical analysis
The effects of condition and emotion were tested with logistic mixed models using the lme4 package (Bates et al. Citation2015) for R (RStudio Team, Citation2020). Accuracy was treated as a dichotomous outcome. We included condition (i.e. forced-choice and free-label) and emotion as fixed effects as well as the emotion-condition interaction. We initially allowed the effects of emotion and condition (random intercepts) to vary across participants (random slope). For the RMET, static, and dynamic emoji sets, however, the models did not converge due to the complexity of the random structure, and we therefore decided to simplify it by using emotion as random intercepts and allowing the effects of condition (random intercepts) to vary across participants (random slope) (Matuschek et al., Citation2017). Emotion and condition levels were dummy-coded. For the factor condition, forced-choice served as reference. For the factor emotion, uneasy served as reference for the RMET, admiration for the static and dynamic emoji sets, and anger for the KDEF-dyn set. We performed this analysis for both the semantic and the conceptual coding schemes, separately. Odds ratios (OR) are provided as effect size estimates. Interacting effects were followed up by pairwise post hoc comparisons using lsmeans (Lenth, Citation2016) adjusting for multiple comparisons (Tukey’s honestly significance test; HSD; Tukey, Citation1949).
We also used logistic mixed models to compare accuracy across sets. We included condition (i.e. forced-choice and free-label) and set (i.e. RMET, static emoji, dynaic emoji, KDEF-dyn) as fixed effect as well as the condition-set interaction. We allowed the effects of set and condition (random intercepts) to vary across participants (random slope). Sets and tasks were dummy-coded. For the factor set, KDEF-dyn served as reference, given that performance in the task was the highest. Similarly, for the factor condition, forced-choice served as reference. Odds ratios (OR) are provided as effect size estimates. Interacting effects were followed up by pairwise post hoc comparisons using lsmeans (Lenth, Citation2016), adjusting for multiple comparisons (Tukey’s HSD).
2. Results
2.1. Reading the Mind in the Eyes Test
The results from the RMET revealed that participants inferred mental states more accurately in the forced-choice, compared to both schemes of the free-labelling task as indicated by a main condition effect: semantic-coded scheme OR = 0.01, (0.01), z = −4.55, p < .001, and conceptual-coded scheme, OR = 0.02, (0.02), z = −4.97, p < .001 (see ). An interaction between condition x emotion indicated differences in accuracy between conditions and mental states (for details see Section S3 in supplementary material). For interaction effects, conditions were compared for each emotion, separately (corrected for multiple comparisons). Results of the post-hoc comparisons are detailed in .
Table 1. Pairwise post hoc comparisons of condition performance (forced-choice vs free-labelling) for each emotion (German translation), and coding scheme in the RMET; p-values are adjusted for multiple comparisons, using Tukey’s HSD correction.
2.2. Static emoji set
In the forced-choice condition, a main effect of condition indicated that participants were more accurate identifying the target emotion relative to both coding schemes of the free-labelling condition: semantic-coded scheme, OR = 0.02 (0.01), Z = −5.31, p < .001; conceptual-coded scheme, OR = 0.04, (0.02), Z = −5.76, p < .001 (see ). A condition x emotion interaction revealed that the condition effects differed for each emotion (for details, see Section S4 in supplementary material). For interaction effects, conditions were compared for each emotion, separately (correcting for multiple comparisons). Results of post-hoc comparisons are shown in and . Unexpectedly, the emoji of excitement was more accurately identified in the free-label condition under the conceptual scheme, compared to the forced-choice condition.
Figure 1. Accuracy for each task and condition in all stimulus sets. Forced-choice task is coloured in dark grey, free-labelling conceptual scheme in middle-dark grey, and free-labelling semantic scheme in light grey. Abbreviations: RMET: Reading the Mind in the Eyes Test, Sta Emoji = Static emojis set, Dyn Emoji: Dynamic emoji set, KDEF-dyn: morphed face set.
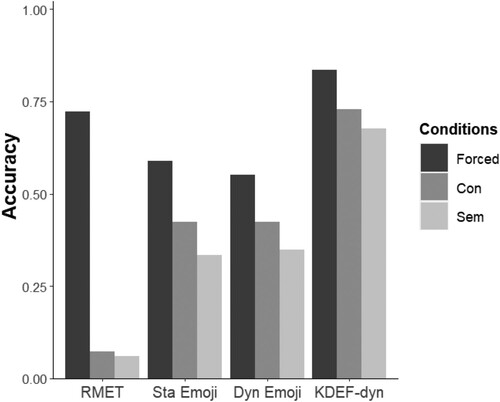
Table 2. Pairwise post hoc comparisons of condition performance (forced-choice vs free-labelling) for each emotion (German translation) and coding scheme in the static emoji dataset; p-values are adjusted for multiple comparisons, using Tukey’s HSD correction.
2.3. Dynamic emoji set.
A main effect of condition revealed higher accuracy scores in the forced-choice condition relative to the free-labelling condition: semantic-coded scheme, OR = 0.03 (0.02), z = −4.73, p < .001; conceptual-coded scheme, OR = 0.03 (0.02), z = −4.71, p < .001 (see ). As for the static emoji set, a condition x emotion interaction was observed (for details, see Section S5 in supplementary material). Following up on the interaction effects, conditions were compared for each emotion, separately (corrected for multiple comparisons). Results of post-hoc comparisons are illustrated in and . As for the static emoji set, some dynamic emojis (i.e. embarrassment, excitement, and happiness) were more accurately identified in the free-label, compared to the forced-choice condition.
Figure 2. Accuracy for each emotion and condition in the static emoji set. Forced-choice condition is coloured in dark grey, free-labelling conceptual scheme in middle-dark grey, and free-labelling semantic scheme in light grey. Abbreviations: Admi: admiration, Amus: amusement, Ange: anger, Bore: boredom, Conf: confusion, Disg: disgust, Emba: embarrassment, Exci: excitement, Grat: gratitude, Happ: happiness, Sadn: sadness, Shyn: shyness, Surp: surprise, Symp: sympathy. *p < .05; **p < .01; ***p < .001, ns: non-significant.
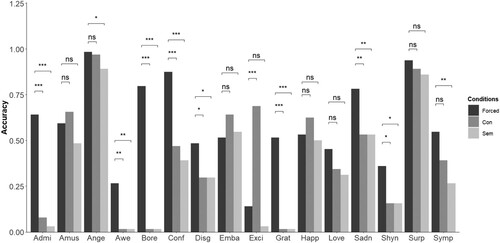
Table 3. Pairwise post hoc comparisons of condition performance for each emotion (German translation) and coding scheme in the dynamic emoji dataset; p-values are adjusted for multiple comparisons, using Tukey’s HSD correction.
2.4. The Karolinska Directed Emotional Faces dynamic (KDEF-dyn) set
Significant condition effects revealed higher accuracy in the forced-choice condition than in both schemes of the free-labelling task: semantic-coded scheme, OR = 0.15 (0.05), z = −5.49, p < .001; conceptual-coded scheme, OR = 0.25 (0.09), z = −3.9, p < .001 (See ). Condition x emotion interaction effects indicated that the effects of condition varied across emotions (for details, see Section S6 in supplementary material). Post hoc comparisons were performed to compare conditions for each emotion, separately. Results of post hoc comparisons are illustrated in and .
Figure 3. Accuracy for each emotion and condition in the dynamic emoji set. Forced-choice task is coloured in dark grey, free-labelling conceptual scheme in middle-dark grey, and free-labelling semantic scheme in light grey. Abbreviations: Admi: admiration, Amus: amusement, Bore: boredom, Conf: confusion, Disg: disgust, Emba: embarrassment, Exci: excitement, Grat: gratitude, Happ: happiness, Shyn: shyness, Surp: surprise, Symp: sympathy. *p < .05; **p < .01; ***p < .001.
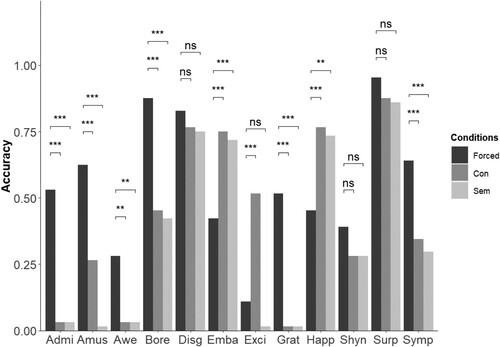
Table 4. Pairwise post hoc comparisons of condition performance (forced-choice vs free-labelling) for each emotion (German translation) and coding scheme in the KDEF-dyn dataset; p-values are adjusted for multiple comparisons, using Tukey’s HSD correction.
2.5. Comparison across sets.
For the semantic code scheme, performance comparison across sets revealed significant effects of condition, OR = 0.41 (0.04), z = −9.98, p < .001. Significant effects of set (ORs > 0.24 (0.03), zs < −6.97, ps < .001) and set x condition interaction effects were also found, particularly for the RMET set, OR = 0.06 (0.01), z = −21.15, p < .001, but not for the static emoji set, OR = 0.84 (0.11), z = −1.37, p = .17, or dynamic emoji set, OR = 1.03 (0.14), z = 0.24, p = .81. Results were followed up by post-hoc comparisons between sets for both the forced-choice and free-labelling condition (See ).
Table 5. Pairwise post hoc comparisons of set performance for each condition, separately; p-values are adjusted for multiple comparisons, using Tukey’s HSD correction.
For the conceptual code scheme, a significant effects of condition was observed, OR = 0.53 (0.09), z = −7.06, p < .001. Significant effects of set (ORs > 0.24(0.03), zs < −7.57, ps < .001) and set x condition interaction effects were also found, particularly for the RMET set, OR = 0.06 (0.01), z = −22.08, p < .001, but not for the static emoji set, OR = 0.96 (0.12), z = −0.33, p = .74 or dynamic emoji set, OR = 1.12 (0.15), z = 0.81, p = .42. Results were followed up by post-hoc comparisons between sets for both the forced-choice and free-labelling condition (See ).
3. Discussion
In the current study, we investigated whether mental- and emotion-related words facilitate the inference of mental and emotional states from facial configurations by comparing the performance in free-labelling and forced-choice conditions across different stimulus sets. Replicating and extending the results from Betz and colleagues (Citation2019) we observed – in a German-speaking sample – that in the RMET and emoji datasets participants were more accurate (as measured by the level of agreement with the experimenters’ expectations) in the forced-choice than in the free-labelling task. Furthermore, in a fourth dataset consisting of morphed facial configurations that mimic human facial movements more realistically, we also observed a task advantage (higher accuracy in forced-choice vs. free-labelling condition) when emotional states were inferred from morphed facial configurations, indicating that words play a modulatory role when making inferences from facial movements.
The findings are in line with the emerging literature highlighting the importance of contextual verbal cues in emotion perception (Fugate, Citation2013; Gendron et al., Citation2014; Lindquist, Citation2017; Lindquist & Gendron, Citation2013; Lindquist et al., Citation2015; Ruba & Pollak, Citation2020). These results provide empirical support to the TCE, according to which contextual cues are an important piece of information for the inference of emotions from facial configurations (Barrett, Citation2017b; Barrett et al., Citation2011). Strong, ecologically-valid evidence for such assumption also comes from a recent study by Le Mau et al. (Citation2021), in which the effects of contextual verbal cues (i.e. descriptive scenarios) on the inference of emotions from posed facial configurations were tested. Importantly, the facial posing was done by professional actors and actresses for whom “their success involves authentic portrayal of emotional experiences in movies, television and theatre, such as their acted facial movement are perceived as believable with highly informational value” (cf. Le Mau et al., Citation2021). The authors observed that the emotional meaning of contextual verbal cues was a stronger predictor of the accuracy than the emotional meaning of the faces alone, indicating that the emotional inference from facial configurations is largely influenced by the associated contextual information.
Our results are also in line with previous semantic priming studies (e.g. Fugate et al., Citation2018; Gendron et al., Citation2012), showing that emotion-related words can modulate emotion perception. The question may thus arise whether the current findings are more influenced by priming or contextual effects. We consider that methodological variations between other priming studies and ours (i.e. in priming studies, the priming stimuli are usually presented before the target stimuli, and not simultaneously; in priming studies, the priming stimuli are rarely used as part of the set of available responses, to avoid confounding effects) makes it difficult to extrapolate our findings to priming effects.
Although the general pattern of our results is line with the results by Betz and colleagues (Citation2019), we also found some differences. In the static and dynamic emoji sets, we observed that some emotions (e.g. embarrassment, happiness, excitement) were more accurately recognised in the free-labelling than in the forced-choice conditions. These differences could be due to variations in emotion concepts across samples (North American and German). Indeed, a closer look to the lists used for coding the free-labelling task suggested that certain emotions may be conceptualised somewhat different in German than in English. For instance, online dictionaries, from which word lists were created, categorised as synonyms two different target emotions (e.g. fröhlich was listed as synonym for amusement and happiness and as related word for excitement; some conceptual differences were also observed between the German and English version of the RMETFootnote2). These examples may suggest that in German some of the emotion concepts were less distinctive from each other. In turn, the same emotion word could have been correctly used to label two different configurations (hereby increasing the accuracy) in the free-labelling condition, but not in the forced-choice task (see Section S7 in supplementary material for a visual depiction supporting this hypothesis). This observation is also in line with evidence pointing to differences in emotion conceptualisation between North Americans and Germans (Kitayama et al., Citation2009; Koopmann-Holm & Tsai, Citation2014; Scheibe et al., Citation2011). For instance, Koopmann-Holm and Tsai (Citation2014) studied messages written in German and English sympathy cards to investigate cultural differences in the conceptualisation of sympathy. Results showed that German sympathy cards included negative words more often than North American ones, whereas North American cards reported more positive words than German ones (Koopmann-Holm & Tsai, Citation2014). Future studies, directly testing cultural differences for other emotion concepts could help understand these differences (e.g. Kollareth et al., Citation2018).
We further observed that differences between tasks were less pronounced in the KDEF-dyn set than in the other three datasets (also, accuracy was higher compared to the other stimulus sets; see and ). These findings may suggest that complete dynamic facial configuration may be particularly informative for inferring emotions compared to static faces (Calvo et al., Citation2018), and to particular sections of it or to exaggerated features. However, other methodological differences such as the number of configurations presented, or repetition vs non-repetition of the same facial configuration may have also influenced on the observed differences across sets. Another difference between the KDEF-dyn set and the RMET and emoji sets concerns the type of emotion concepts used. Whereas emoji sets used a broader variety of emotion concepts, the KDEF-dyn consisted of some of the emotion concepts categorised as “basic” by the theory of basic emotions. It could therefore be that participants are generally more exposed to stereotypical expressions of such emotions and thus tend to infer them more easily from specific facial configurations (see supplementary information S8 for exploratory analysis, grouping words based on basic and non-basic emotions in the emoji datasets).
Figure 4. Accuracy based on the weighted binary code for each emotion and condition in the morphed face dataset (KDEF-dyn). Forced-choice task is coloured in dark grey, free-labelling conceptual scheme in middle-dark grey, and free-labelling semantic scheme in light grey. Abbreviations: Ange: anger, Disg: disgust, Fear: fear, Happ: happiness, Sadn: sadness, Surp: surprise. *p < .05; **p < .01; ***p < .001.
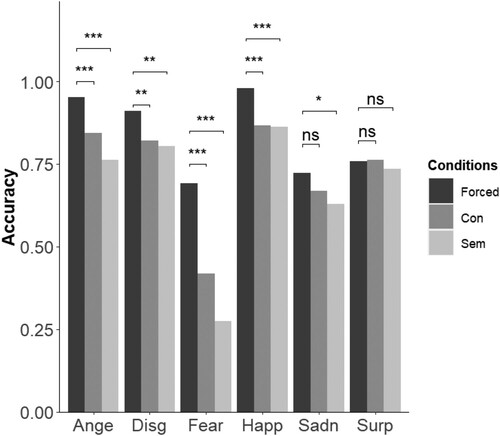
The current study is not without limitations. Firstly, our sample size mostly consists of female university students which constrains the generalisability of our results. Secondly, the use of a within-subject design that allowed all participants to perform both tasks could have produced some unwanted effects. Participants were always exposed to the same stimuli twice, first in the free-labelling condition and then in the forced-choice condition. Although they were unaware that the same stimuli were going to be presented twice, the repetition of the stimuli could have promoted the development of response strategies that could have biased the interpretation of the results. In the same vein, as discussed by Betz and colleagues (Citation2019), the forced-choice task could also lead to the use of strategies such as selection for elimination that could affect the interpretation of the findings (see also for discussion Barrett et al., Citation2019). Thirdly, even though the use of a dataset that included morphed facial configurations extending previous findings (Betz et al., Citation2019), it is important to note that the faces in the KDEF-dyn were created not by recording continuous, naturalistic human movements, but by artificially morphing together static images in sequence, resulting of actors enacting caricatured poses, rather than capturing spontaneously induced facial configurations. Future studies should therefore extend the present findings using even more naturalistic stimuli (e.g. Aviezer et al., Citation2012).
In summary, we observed that providing emotion labels facilitates emotion perception accuracy across a diversity of facial emotion expression stimuli. From a methodological perspective, these results suggest that providing forced-choice emotion word anchors in emotion perception tasks may guide participants towards more “accurate” results in line with experimenters’ expectations, rather than capturing the emotion categories that participants might more naturally provide themselves. More generally, these findings align with hypotheses from the TCE that words, when provided, can serve as contextual information that modulate the inference of emotional states from facial configurations.
Ethical approval
Data from the current study are part of a larger project involving human participants that was reviewed and approved by the Ethics Committee of the University of Potsdam. The participants provided their written informed consent to participate in this study.
Author contributions
CVB Conceived and designed the analysis, collected the data; DP and CVB performed the analysis. CVB wrote the paper. CVB and MW reviewed and edited the manuscript.
SupplMaterial1602.docx
Download MS Word (462.4 KB)Acknowledgements
We are grateful to Nicole Betz for providing parts of the stimulus material. We also thank Joshua Pepe Woller and Paula Schneider for their help in data coding, and Yura Kim, Lok Yan Lam, Adnan Balsheh, and Muhannad Said Taha for their assistance in the preparation of the study and data collection.
Disclosure statement
No potential conflict of interest was reported by the author(s).
Notes
1 The original sentence comes from a Latin proverb attributed to Cicero (106-43 B.C.): “Ut imago est animi voltus sic indices oculi”.
2 In the English version of the test, the words worried and concern were distinctively used for two different trials. In the German version, however, both words were translated with the same word (besorgt). We observed the same for distrustful and suspicious (both translated as misstrauisch), and for pensive and reflective (both translated as nachdenklich).
Reference
- Aviezer, H., Hassin, R. R., Ryan, J., Grady, C., Susskind, J., Anderson, A., Moscovitch, M., & Bentin, S. (2008). Angry, disgusted, or afraid? Studies on the malleability of emotion perception: Research article. Psychological Science, 19(7), 724–732. https://doi.org/10.1111/j.1467-9280.2008.02148.x
- Aviezer, H., Trope, Y., & Todorov, A. (2012). Body cues, not facial expressions, discriminate between intense positive and negative emotions. Science, 338(6111), 1225–1229. https://doi.org/10.1126/science.1224313
- Baron-Cohen, S., Wheelwright, S., Hill, J., Raste, Y., & Plumb, I. (2001). The “Reading the Mind in the Eyes” test revised version: A study with normal adults, and adults with Asperger syndrome or high−functioning autism. Journal of Child Psychology and Psychiatry, 42(2), 241–251. doi: 10.1111/1469-7610.00715
- Baron-Cohen, S., Wheelwright, S., & Jolliffe, T. (1997). Is there a “language of the eyes”? Evidence from normal adults, and adults with autism or Asperger syndrome. Visual Cognition, 4(3), 311–331. https://doi.org/10.1080/713756761
- Barrett, L. F. (2006). Are emotions natural kinds? Perspectives on Psychological Science, 1(1), 28–58. https://doi.org/10.1111/j.1745-6916.2006.00003.x
- Barrett, L. F.. (2017a). How emotions are made: The secret life of the brain. Houghton Mifflin Harcourt.
- Barrett, L. F. (2017b). The theory of constructed emotion: An active inference account of interoception and categorization. Social Cognitive and Affective Neuroscience, 12(1), 34–36. doi:10.1093/scan/nsw156
- Barrett, L. F., Adolphs, R., Marsella, S., Martinez, A. M., & Pollak, S. D. (2019). Emotional expressions reconsidered: Challenges to inferring emotion from human facial movements. Psychological Science in the Public Interest, 20(1), 1–68. https://doi.org/10.1177/1529100619832930
- Barrett, L. F., Mesquita, B., & Gendron, M. (2011). Context in emotion perception. Current Directions in Psychological Science, 20(5), 286–290. https://doi.org/10.1177/0963721411422522
- Bates, D., Machler, M., Bolker, B., & Walker, S. (2015). Fitting linear mixed-effects models using lme4. Journal of Statistical Software, 67, 1–48. http://doi.org/10.18637/jss.v067.i01
- Betz, N., Hoemann, K., & Barrett, L. F. (2019). Words are a context for mental inference. Emotion, 19(8), 1463–1477. https://doi.org/10.1037/emo0000510
- Bölte, S. (2005). Reading the Mind in the Eyes Test für Erwachsene (dt. Fassung) Von S. Baron-Cohen. J.W. Goethe-Universität.
- Calvo, M. G., Fernández-Martín, A., Recio, G., & Lundqvist, D. (2018). Human observers and automated assessment of dynamic emotional facial expressions: KDEF-dyn database validation. Frontiers in Psychology, 9, 2052. https://doi.org/10.3389/fpsyg.2018.02052
- Darwin, C. (2005). The expression of the emotions in man and animals. Digireads.com Publishing. http://doi.org/10.1037/10001-000 (Originalwork published 1872)
- Doyle, C. M., Gendron, M., & Lindquist, K. A. (2021). Language is a unique context for emotion perception. Affective Science, 2(2), 171–177. https://doi.org/10.1007/s42761-020-00025-7
- Ekman, P. (1972). Universals and cultural differences in facial expressions of emotions. In J. Cole (Ed.), Nebraska symposium on motivation (pp. 207–282).
- Ekman, P., & Cordaro, D. (2011). What is meant by calling emotions basic. Emotion Review, 3(4), 364–370. https://doi.org/10.1177/1754073911410740
- Ekman, P., Freisen, W. V. (1980). Facial signs of emotional experience. Journal of Personality and Social Psychology, 39(6), 1125–1134. https://doi.org/10.1037/h0077722
- Ekman, P., & Friesen, W. V. (1971). Constants across cultures in the face and emotion. Journal of Personality and Social Psychology, 17(2), 124–129. https://doi.org/10.1037/h0030377
- Ekman, P., Friesen, W. V., O’Sullivan, M., Chan, A., Diacoyanni-Tarlatzis, I., Heider, K., Krause, R., LeCompte, W. A., Pitcairn, T., Ricci-Bitti, P. E., Scherer, K., Tomita, M., & Tzavaras, A. (1987). Universals and cultural differences in the judgments of facial expressions of emotion. Journal of Personality and Social Psychology, 53(4), 712–717. https://doi.org/10.1037/0022-3514.53.4.712
- Ekman, P., Levenson, R. W., & Friesen, W. V. (1983). Autonomic nervous system activity distinguishes among emotions. Science, 221(4616), 1208–1210. https://doi.org/10.1126/science.6612338
- Ekman, P., Sorenson, E. R., & Friesen, W. V. (1969). Pan-cultural elements in facial displays of emotion. Science, 164(3875), 86–88. https://doi.org/10.1126/science.164.3875.86
- Faul, F., Erdfelder, E., Buchner, A., & Lang, A.-G. (2009). Statistical power analyses using G*Power 3.1: Tests for correlation and regression analyses. Behavior Research Methods, 41(4), 1149–1160. doi:10.3758/BRM.41.4.1149
- Faul, F., Erdfelder, E., Lang, A.-G., & Buchner, A. (2007). G*Power 3: A flexible statistical power analysis program for the social, behavioral, and biomedical sciences. Behavior Research Methods, 39(2), 175–191. doi:10.3758/BF03193146
- Fugate, J. M. B. (2013). Categorical perception for emotional faces. Emotion Review, 5(1), 84–89. https://doi.org/10.1177/1754073912451350
- Fugate, J. M. B., Gendron, M., Nakashima, S. F., & Barrett, L. F. (2018). Emotion words: Adding face value. Emotion, 18(5), 693–706. https://doi.org/10.1037/emo0000330
- Fugate, J. M. B., Gouzoules, H., & Barrett, L. F. (2010). Reading chimpanzee faces : Evidence for the role of verbal labels in categorical perception of emotion. Emotion, 10(4), 544–554. https://doi.org/10.1037/a0019017
- Gendron, M., & Feldman Barrett, L. (2009). Reconstructing the past: A century of ideas about emotion in psychology. Emotion Review, 1(4), 316–339. https://doi.org/10.1177/1754073909338877
- Gendron, M., Lindquist, K. A., Barsalou, L., & Barrett, L. F. (2012). Emotion words shape emotion percepts. Emotion, 12(2), 314–325. https://doi.org/10.1037/a0026007
- Gendron, M., Roberson, D., van der Vyver, J. M., & Barrett, L. F. (2014). Perceptions of emotion from facial expressions are not culturally universal: Evidence from a remote culture. Emotion, 14(2), 251–262. https://doi.org/10.1037/a0036052
- Hoemann, K., Khan, Z., Feldman, M. J., Nielson, C., Devlin, M., Dy, J., Barrett, L. F., Wormwood, J. B., & Quigley, K. S. (2020). Context-aware experience sampling reveals the scale of variation in affective experience. Scientific Reports, 10(1), 1–16. https://doi.org/10.1038/s41598-020-69180-y
- Jones, M. (2017). St. David’s day doodle! Retrieved March 2, 2017, from http://mattjonezanimation.blogspot.com/
- Kitayama, N., Vaccarino, V., Kutner, M., Weiss, P., & Bremner, J. D. (2005). Magnetic resonance imaging (MRI) measurement of hippocampal volume in posttraumatic stress disorder: A meta-analysis. Journal of Affective Disorders, 88(1), 79–86. https://doi.org/10.1016/j.jad.2005.05.014
- Kitayama, S., Park, H., Sevincer, A. T., Karasawa, M., & Uskul, A. K. (2009). A cultural task analysis of implicit independence: Comparing North America, Western Europe, and East Asia. Journal of Personality and Social Psychology, 97(2), 236–255. https://doi.org/10.1037/a0015999
- Kollareth, D., Fernandez-Dols, J. M., & Russell, J. A. (2018). Shame as a culture-specific emotion concept. Journal of Cognition and Culture, 18(3–4), 274–292. https://doi.org/10.1163/15685373-12340031
- Koopmann-Holm, B., & Tsai, J. L. (2014). Focusing on the negative: Cultural differences in expressions of sympathy. Journal of Personality and Social Psychology, 107(6), 1092–1115. https://doi.org/10.1037/a0037684
- Leiner, D. J. (2019). Too fast, too straight, too weird : Non-reactive indicators for meaningless data in internet surveys. Journal of the European Survey Research Association, 13(3), 229–248. https://doi.org/10.18148/srm/2019.v13i3.7403
- Le Mau, T., Hoemann, K., Lyons, S. H., Fugate, J. M. B., Brown, E. N., Gendron, M., & Barrett, L. F. (2021). Professional actors demonstrate variability, not stereotypical expressions, when portraying emotional states in photographs. Nature Communications, 12(1), 1–13. https://doi.org/10.1038/s41467-021-25352-6
- Lenth, R. V. (2016). Least-squares means: The R package lsmeans. Journal of Statistical Software, 69(1), 1–33. https://doi.org/10.18637/jss.v069.i01
- Lindquist, K. A. (2017). The role of language in emotion: existing evidence and future directions. Current Opinion in Psychology, 17, 135–139. https://doi.org/10.1016/j.copsyc.2017.07.006
- Lindquist, K. A., Barrett, L. F., Bliss-Moreau, E., & Russell, J. A. (2006). Language and the perception of emotion. Emotion, 6(1), 125–138. https://doi.org/10.1037/1528-3542.6.1.125
- Lindquist, K. A., & Gendron, M. (2013). What’s in a word? Language constructs emotion perception. Emotion Review, 5(1), 66–71. https://doi.org/10.1177/1754073912451351
- Lindquist, K. A., Gendron, M., Barrett, L. F., & Dickerson, B. C. (2014). Emotion perception, but not affect perception, is impaired with semantic memory loss. Emotion, 14(2), 375–387. https://doi.org/10.1037/a0035293
- Lindquist, K. A., Satpute, A. B., & Gendron, M. (2015). Does language do more than communicate emotion? Current Directions in Psychological Science, 24(2), 99–108. https://doi.org/10.1177/0963721414553440
- Matuschek, H., Kliegl, R., Vasishth, S., Baayen, H., & Bates, D. (2017). Balancing Type I error and power in linear mixed models. Journal of Memory and Language, 94, 305–315. https://doi.org/10.1016/j.jml.2017.01.001
- Mesquita, B., Boiger, M., & De Leersnyder, J. (2016). The cultural construction of emotions. Current Opinion in Psychology, 8(April), 31–36. https://doi.org/10.1016/j.copsyc.2015.09.015
- Nook, E. C., Lindquist, K. A., & Zaki, J. (2015). A new look at emotion perception: Concepts speed and shape facial emotion recognition. Emotion, 15(5), 569–578. https://doi.org/10.1037/a0039166
- Oakley, B. F. M., Brewer, R., Bird, G., & Catmur, C. (2016). Theory of mind is not theory of emotion: A cautionary note on the reading the mind in the eyes test. Journal of Abnormal Psychology, 125(6), 818–823. https://doi.org/10.1037/abn0000182
- RStudio Team. (2020). RStudio: Integrated development for R. RStudio, PBC, Boston, MA URL http://www.rstudio.com/
- Ruba, A. L., Johnson, K. M., Harris, L. T., & Wilbourn, M. P. (2017). Developmental changes in infants’ categorization of anger and disgust facial expressions. Developmental Psychology, 53(10), 1826–1832. https://doi.org/10.1037/dev0000381
- Ruba, A. L., & Pollak, S. D. (2020). The development of emotion reasoning in infancy and early childhood. Annual Review of Developmental Psychology, 2(1), 503–531. https://doi.org/10.1146/annurev-devpsych-060320-102556
- Ruba, A. L., Wilbourn, M. P., Ulrich, D. M., & Harris, L. T. (2018). Constructing emotion categorization: Insights from developmental psychology applied to a young adult sample. Emotion (Washington, D.C.), 18(7), 1043–1051. https://doi.org/10.1037/emo0000364
- Russell, J. A., & Widen, S. C. (2002). A label superiority effect in children’s categorization of facial expressions. Social Development, 11(1), 30–52. https://doi.org/10.1111/1467-9507.00185
- Scheibe, S., Blanchard-Fields, F., Wiest, M., & Freund, A. M. (2011). Is longing only for Germans? A cross-cultural comparison of sehnsucht in Germany and the United States. Developmental Psychology, 47(3), 603–618. https://doi.org/10.1037/a0021807
- Todorov, A. (2017). Face value: The irresistible influence of first impressions. Princeton University Press.https://doi.org/10.2307/j.ctvc7736t
- Tukey, J. W. (1949). Comparing individual means in the analysis of variance. Biometrics, 5(2), 99–114. https://doi.org/10.2307/3001913
- Ventura-Bort, C., Wendt, J., & Weymar, M. (2022). New insights on the correspondence between subjective affective experience and physiological responses from representational similarity analysis. Psychophysiology, 59, e14088. https://doi.org/10.1111/psyp.14088
- Zhang, L., Holzleitner, I. J., Lee, A. J., Wang, H., Han, C., Fasolt, V., DeBruine, L. M., & Jones, B. C. (2019). A data-driven test for cross-cultural differences in face preferences. Perception, 48(6), 487–499. https://doi.org/10.1177/0301006619849382
- Zolli, A. (2015). Darwin’s Stickers. Retrieved from http://www.radiolab.org/story/darwins-stickers/