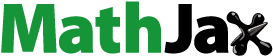
Abstract
Functional classification is widely recognized as an important approach for characterizing phytoplankton community response to environmental change in freshwater ecosystems. However, there are few studies of that type examining the northeast (NE) China wetlands. The study aimed to evaluate the capacities of phytoplankton functional groups (FGs), morpho-functional groups (MFGs), and morphology-based functional groups (MBFGs), to characterize phytoplankton response to environmental heterogeneity in two wetlands with different nutrient levels. Water quality variables and phytoplankton were examined in the Longfeng and Hulanhe wetlands located on the Songnen Plain, NE China, in 2013. Multivariate analyses (RDA) were performed to compare three functional classification approaches to environmental heterogeneity. Our results revealed that all three functional classification approaches (FGs, MFGs, and MBFG) could characterize the phytoplankton community response to environmental heterogeneity. MBFG had the highest cumulative percentage variance of species composition. We suggest using MBFG as a routine tool to characterize environmental heterogeneity in wetlands NE China. Furthermore, total nitrogen (TN), total phosphorus (TP), electrical conductivity (EC), and pH were key predictors for regulating the ecological distribution of the phytoplankton community pattern in the study area. This study further highlights that MBFG is a relevant tool in assessing ecological health in wetland ecosystems.
Introduction
The function, health, and integrity of wetlands around the globe are strongly influenced by intense anthropogenic activity, including non-point pollutant sources, land use changes, and a booming human population (Gu et al. Citation2020, Ji et al. Citation2021, Olofsson et al. Citation2014, Puglisi et al. Citation2005). Wetlands provide several important ecosystem functions such as wildlife habitats, water filtration, and flood protection (Bunn and Arthington Citation2002, Dudgeon et al. Citation2006). In one of the most densely populated areas in China, the wetlands of Songnen Plain have been significantly reduced due to intensive anthropogenic activities (Gong et al. Citation2010). Previous studies have shown that 50% of the world's wetlands have been lost due to development, agricultural practices, and other causes (Zedler and Kercher Citation2005). Northeast China has a high density of industry and agricultural activities (Liu et al. Citation2010, Xiao et al. Citation2015). As the most densely populated wetlands in Heilongjiang Province, the Longfeng and Hulanhe wetlands have experienced serious disturbances due to intensive human alteration and other anthropogenic activities (Wang and Zang Citation2014). As a result, phytoplankton communities had been damaged, causing loss of ecosystem function, health, and integrity. Given the importance of water protection, as well as the increasing attention on wetland ecosystem health and integrity, it is necessary to develop aquatic organism matrices to indicate the environmental changes in wetlands (Reid et al. Citation2019, Zhang and Zang Citation2015, Zhao et al. Citation2018).
Phytoplankton plays a key role in wetland food webs (Dudgeon et al. Citation2006, Zhao et al. Citation2018). Due to their short life cycle and sensitivity to environmental change, their distribution patterns are widely used to predict wetland water quality, health, function, and integrity (Sim et al. Citation2021, Wilson et al. Citation2021, Zhong et al. Citation2021). Many studies have concluded that functional classifications of phytoplankton can reflect aquatic ecological characteristics (Abonyi et al. Citation2014). Species in the same functional classification are considered to have a similar niche (Reynolds et al. Citation2002). Phytoplankton functional (FGs), morpho-functional (MFGs), and morphology-based functional groups (MBFGs) have been widely used in comparative ecological studies and to facilitate the development of biological indicators for assessing environmental change in rivers, lakes, reservoirs, and wetlands (Amorim et al. Citation2019, Bortolini and Bueno Citation2017, Reynolds et al. Citation2002). However, the information necessary for using a functional approach to characterize phytoplankton community response to environmental changes is lacking for wetland ecosystem from NE China (Chengxue et al. Citation2011). Additionally, the efficiency of functional classification to characterize water quality is unclear (Izaguirre et al. Citation2012). For instance, an FGs approach was considered useful for characterizing nutrient status in the shallow eutrophic lakes in cold, arid regions China (Jin et al. Citation2020); MBFGs proved to be the best tool to characterize shallow lake-dwelling phytoplankton on the Amazonian floodplain (Pereira Souza Lobo et al. Citation2018). Therefore, we used FGs, MFGs, and MBFG approaches to characterize the phytoplankton community in two anthropogenic influenced wetlands in NE China. We hypothesized that different functional approaches (FGs, MFGs, and MBFGs) would have different capacities to characterize phytoplankton dynamics in anthropogenically influenced wetlands. The research questions are as follows:
Which of the functional approaches best characterizes phytoplankton community spatial dynamics in two anthropogenic eutrophic urban wetlands?
Which environmental factors regulate the phytoplankton community structure?
Materials and methods
Studied areas
Agricultural and industrial development combined with climatic variations and rising population have resulted in a substantial loss of marshes in NE China over the past decades (Gong et al. Citation2010). The declining area, fragmentation, and heterogeneity of marshes are closely associated with phytoplankton community assembly (Zedler and Kercher Citation2005). Our study was conducted on the Longfeng wetland (LFW) and the Hulanhe wetland (HLHW), two typical anthropogenically-influenced regions in NE China (). The study regions have a temperate monsoon climate with obvious changes between seasons, such as warm summers and cold winters. The air temperature ranges from −22 °C to 35 °C, and the mean annual temperature is 3.9 °C. The annual precipitation is approximately 400 mm. The LFW has an area of 10 km2 and is located in the center of Daqing City. It is also shallow (1.2 m of mean depth), eutrophic, and alkaline with an annual mean rainfall of 435 mm. The average depths in dry and rainy seasons in the LFW are 1.5 m and 0.8 m, respectively. Hummocks of grass are widespread in the LFW. The HLHW is located on the Songnen plain north of the city of Harbin. The HLHW has an area of 19 km2 and is bordered by the Songhua River to the south and the Hulan River to the north. It is shallow (1.3 m mean depth), mesotrophic, and neutral, with an annual mean rainfall of 480 mm. Similar to the HLHW, the average depths in the dry and rainy seasons in the LFW are 1.7 m and 0.7 m, respectively. Water samples were collected from a depth of 0.5 m by plastics water samplers from a total of fifteen stations in the two wetlands; five stations in the LFW, and ten stations in the HLHW (). Samples were collected in both the dry (May) and rainy (August) seasons in 2013. Phytoplankton counts were done using microscope (Nikon-1100, Nikon, Tokyo). Classification and identification of phytoplankton species were based on (Anonymous Citation2011).
Table 1. Geographic position, main morphometric features, environmental information and city of two wetlands.
Sampling and lab analysis
Duplicate water sampling was carried out quarterly during the dry (May) and rainy (August) seasons in 2013. All samples were collected from approximately 11:00 AM to 12:30 PM. A 1-L water sample was preserved with 1% Lugol’s solution, and concentration in 30 ml to count the abundance of phytoplankton. Phytoplankton identification and enumeration were carried out using an inverted microscope (at 400 × magnification) in 10-ml sedimentation chambers (Utermöhl Citation1958) (Utermöhl Citation1958). Phytoplankton that could not be accurately identified were classified to the level of genus. The water temperature (WT), pH, conductivity (EC), and Dissolved Oxygen (DO) were measured at 0.5 m below the water surface using a YSI (Perkin Elmer Inc., USA). Total nitrogen (TN), total phosphorus (TP), chemical oxygen demand (COD), and biochemical oxygen demand (BOD5) were determined according to Chinese national standards for water quality (Zhang and Zang Citation2015).
Multivariate statistical analysis
Ordination analysis (DCA, PCA, RDA) was performed to explore the relationship among FG, MBFG, MFG, and environmental factors. CANOCO for Windows 5.0 software was used. DCA was used to determine whether the relationship fit a linear model (RDA) or unimodal model (CCA) (Lai Citation2013). A Monte Carlo test permutation test was performed to determine the confidence interval (P < 0.05). An independent-samples t test (t test) was performed to determine the effect of environmental factors. Water quality was assessed using the trophic state index (TSI) (Wang Citation2002). The equations for TSI are as follows:
Evaluation standard: 0 < TSIM ≤ 30 oligotrophic, 30 < TSIM ≤ 50 mesotrophic, TSIM > 50 eutrophic, 50 < TSIM ≤ 60 light eutrophic, 60 < TSIM ≤ 70 middle eutrophic, TSIM > 70 high eutrophic.
Results
Environmental characterization and heterogeneity
All environmental factors information is shown in . The t test showed that five of the eight environmental factors (excluding WT, DO, and COD) showed significant variation between two wetlands. The t test revealed that, except for WT (P < 0.05), the other seven physical and chemical factors showed no significant difference between rainy and dry seasons. The highest value of pH, EC, BOD5, TN, and TP were recorded in LFW (mean 7.63, 1016.40 uS/cm, 13.00 mg/L, 5.65 mg/L and 1.33 mg/L, respectively). LFW was alkaline (mean pH 7.63). The conductivity was significantly different between the two wetlands in that the value for LFW (mean EC 1016.40 µS/cm) was nearly three times than that of HLHW (mean EC 299.36 µS/cm). The TSI revealed that the study regions were in light eutrophic (LFW) to meso-eutrophic (HLHW) states during the study periods ().
Table 2. Mean and standard deviation of physical chemistry factors in two wetlands during the study period.
Phytoplankton functional groups pattern
In this study, a total of 175 algae species were identified. The 175 algae species belong to the following groups: Chlorophyceae (45), Bacillariophyceae (66), Zygnematophyceae (12), Cyanobacteria (30), Euglenophyceae (12), Xanthophyceae (2), Cryptophyceae (4), Dinophyceae (3), and Raphidophyceae (1). The dominant algae species belonged to Bacillariophyta and Chlorophyta. Furthermore, Synedra, Nitzschia, and Scenedesmus were dominant in LFW while Navicula, Trachelomonas, and Gomphonema were dominant in HLHW. The abundance in LFW (1363.7 × 104 ind./L) was significantly higher than that in HLHW (148.7 × 104 ind./L) ().
Figure 2. Spatial and temporal variation of total phytoplankton abundance in each wetland and hydrological period during the study period (LF: Longfeng wetland, HLWH: Hulanhe wetland).
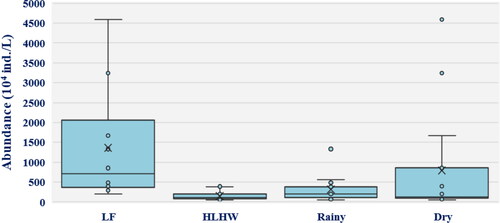
A total of 14 FGs were identified. The functional groups that best characterized the phytoplankton community in LFW were C (mean 20.8 × 104 ind./L), D (12.5 × 104 ind./L), J (31.8 × 104 ind./L), and (Y mean 29.0 × 104 ind./L) (, ). Species that are adapted to eutrophic, turbid and lentic habits were represented by the FGs C and D, consisting of Aulacoseira spp., Synedra spp., and Nitzschia spp. Notably, FGs J is composed of species such as Scenedesmus spp. and Coelastrum spp that are adapted to shallow enriched lakes and can tolerate high trophic states. HLHW was dominated by FGs MP (mean 5.2 × 104 ind./L), X1 (3.9 × 104 ind./L), and J (1.3 × 104 ind./L) ().
Figure 3. Spatial and temporal variation of the relative proportion of the different functional approaches (in abundance) in two wetlands during the study period (LF-R: sample station of Longfeng wetland in Rainy season, LF-D: sample station of Longfeng wetland in Dry season, HLHW-R: sample station of Hulanhe wetland in Rainy season, HLHW-D: sample station of Hulanhe wetland in Dry season).
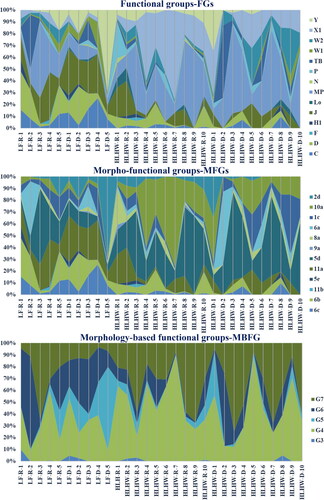
Figure 4. First and second axes of the RDA based on the abundance of the functional groups (FGs) (a and b), Morpho-functional groups (MFGs) (c and d) and Morphology-based functional groups (MBFGs) (e and f) on the Longfeng wetland and Hulanhe wetland.
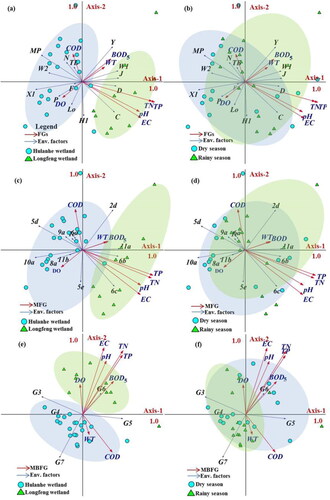
Table 3. Relative proportion of the descriptors species and their respective functional groups in two wetlands during study period.
Morpho-functional groups (MFGs) were represented by 12 groups. In LFW, we found that phytoplankton community was constituted by non-filament colonies (11a, mean 31.7 × 104 ind./L), represented by Pandorina spp., small (unicellular) flagellates (2d, mean 29.0 × 104 ind./L), composed of Cryptomons ovata, and large Pennates diatom (6 b, mean 12.4 × 104 ind./L and 6c, mean 20.8 × 104 ind./L), composed of Nizschia spp. and Synedra spp. In HLHW, we observed that small colonies without flagella colonies Cyanobacteria (5d, mean 5.5 × 104 ind./L), composed of Chroococcus spp.; filaments chlorophytes (10a, mean 3.92 × 104 ind./L), composed by Chlorella spp.; and non-filament colonies (11a, mean 1.3 × 104 ind./L) were key components in phytoplankton community ().
Morphology-based functional groups (MBFG) were represented by five groups, which varied in prevalence. In LFW, G4 and G5 occurred in the same proportions (41.2 × 104 ind./L and 42.6 × 104 ind./L, respectively). MBFG G4, composed of Scenedesmus spp. and Coelastrum spp., and associated with medium-sized phytoplankton from eutrophic shallow lakes. MBFG G5, composed of Cryptomons spp., was associated with unicellular flagellates of size medium to large. G6, composed of Synedra and Nitzschia, was another representative group (36.6 × 104 ind./L). It was associated with non-flagellated organisms with siliceous exoskeletons. These three groups contributed more than 88% of the total abundance. In HLHW, G4 (mean 7.0 × 104 ind./L) and G7 (mean 5.5 × 104 ind./L) co-contribute more than 85% of the total abundance. MBFG G7, composed of Scenedesmus, was associated with large mucilaginous colonies.
Phytoplankton functional groups associate with environment heterogeneity
The RDA for the functional groups (FGs) explained 41.7% of the total variability in the phytoplankton community composition (). The first two canonical axes together represented 65.4% of the variance of the species-environment relationship (axis 1: 47.7; axis 2: 16.8%). Axis 1 was positively correlated with TP (0.8843) and TN (0.8747), and negatively with COD (–0.2184). Axis 2 was positively correlated with COD (0.6573), and negatively with pH (–0.099). The Monte Carlo permutation test indicated that TP (P < 0.002), TN (P < 0.002), EC (P < 0.002), and pH (P < 0.002) were the key factors influencing FGs ecological distribution (). A higher level of TP was associated with higher J, C, and D. The RDA for the morpho-functional groups (MFGs) explained 41.5% of the total variability in the phytoplankton community composition. The first two canonical axes together represented 64.4% of the variance of the species-environment relationship (axis 1: 46.3; axis 2: 18.1%). Axis 1 was positively correlated with TN (0.9116) and TP (0.9097), and negatively with EC (0.8428) and pH (0.7772). Axis 2 was positively correlated with COD (0.7687), and negatively with TP (–0.0897). The Monte Carlo permutation test indicated that TP (P < 0.002), TN (P < 0.002), EC (P < 0.002), and pH (P < 0.002) were the key factors in MFGs ecological distribution. A higher level of TP was associated with higher 11a, 6 b, and 6c. The RDA for the morphology-based functional groups (MBFGs) explained 43.5% of the total variability in the phytoplankton community composition. The first two canonical axes together represented 90.6% of the species-environment relationship (axis 1: 58.7; axis 2: 31.9%). Axis 1 was positively correlated with TN (0.8064), TP (0.8064), EC (0.7148), and pH (0.6393). Axis 2 was positively correlated with pH (0.3770). The Monte Carlo permutation test indicated that TP (P < 0.002), TN (P < 0.004), EC (P < 0.006), and pH (P < 0.004) were the key factors associated with MBFG ecological distribution ().
Table 4. Summary statistics of Redundancy analysis in FGs, MFGs and MBFG.
Table 5. Results of the Monte Carlo test permutation test in FGs, MFGs and MBFG.
Discussion
The goal of this study was to evaluate the capacities of three functional classification approaches to characterizing phytoplankton response to environmental heterogeneity. In two wetlands, we found that MBFG had the strongest relationship with the environmental factors (43.5% of the total variability in the phytoplankton community composition). In addition, we found that the spatial distribution pattern of phytoplankton was closely linked to the TP, TN, EC, and pH. In response to our research questions, the results showed that (1) MBFG had the highest capacity for characterizing phytoplankton response to environmental heterogeneity in NE China wetlands and (2) TP, TN, EC, and pH were the most important environmental factors in the structuring of the phytoplankton community in the NE China wetlands. The high capacity of MBFG to characterize phytoplankton response to environmental heterogeneity indicates that this approach can be used for routine monitoring of water quality in NE China wetlands. Additional advantages—such as the relative simplicity—included ecological information for their application.
In RDA, the site score equals the weighted sum of the species scores (Lu et al. Citation2020). Therefore, the position of one sampling station in the ordination diagram represents the weights of all species at the site with respect to the two axes. Stations in the LFW were located in the positive direction of axis 1, which are included in the arrows of TN and TP. Environmental factors (TN and TP) represent high nutrient concentrations in LFW (). The TSI indicated that almost all of the samples from the LFW were highly eutrophic (). Stations in the HLHW are grouped on the negative side of axis 1, indicating the relatively low trophic level in the area. The results of RDA showed that the station positions in the ordination diagram could clearly reflect the environmental gradient in all three functional classification approaches. This result suggests that the distribution pattern of three functional classification approaches is relevant for characterizing wetland phytoplankton response to environmental heterogeneity. Morphological traits were widely considered a signal for revealing the phytoplankton ecological characteristics because they were closely associated with the capacity to acquire resources and to remain in the water column similar to the flagellate that could promote the motile ability to capture lights (Bortolini et al. Citation2014, Naselli-Flores Citation2014). A functional classification proposed by Reynolds et al. (Citation2002) promoted the idea that morphological traits respond to environmental change (Reynolds et al. Citation2002). Previous studies have found that clear eutrophic signals can be identified from the FGs distribution in freshwater ecosystems (Oguz et al. Citation2020). For example, Çelik and Sevindik (2015) used FGs to evaluate eutrophication and the poor nutrition characteristics of water in Turkish reservoirs (Celik and Sevindik Citation2015). They suggested that the presence of J and D in FGs indicates that water is a feature of eutrophication. We found a significant positive correlation between TN, D, and J, suggesting that D and J are indicators for eutrophication in the LFW. The main functional group of phytoplankton in the study area, Y, was positively correlated with functional group D. Thus, our results suggest that functional group Y could also express eutrophic characteristics of NE China wetlands. Furthermore, RNA and TSI revealed that the mild eutrophication in the HLHW slightly increased the proportion of FGs X1. X1 was reported to be dominant in eutrophic reservoirs (Reynolds et al. Citation2002). This result further highlights that FGs can characterize environmental heterogeneity in wetlands in NE China. Furthermore, MFGs 2d and 11a, which were associated with higher values of TN, TP, and EC in LFW, were also recorded in the Amazonian floodplain, indicating the increased nutrient concentrations (Pereira Souza Lobo et al. Citation2018). These MFGs were also noted in the shallow lakes from the Pampa Plain (Fernandez et al. Citation2014). In addition, while MFGs 2d tolerate highly turbulent environments, MFGs 11a inhabited eutrophic conditions (Salmaso and Padisak Citation2007). The co-dominance of 2d and 11a during LFW indicates that the environmental conditions selected for groups of species that share similar adaptive characteristics. The RDA revealed that the MBFG approach had the highest total variability in the phytoplankton community composition (43.5%). In RDA, MBFGs G3, G4, and G7 were important in the HLWH, while G5 and G6 were predominant in LFW and were associated with the highest values of TN and TP. The G5 group, marked by a high level of electrical conductivity, was also characteristic of shallow lakes from the Amazonian floodplain (Pereira Souza Lobo et al. Citation2018). Izaguirre et al. (Citation2012) noted that dominance by G5 was an indicator for high concentrations of inorganic suspended solids (Izaguirre et al. Citation2012).
Phytoplankton is a principal component of and the most important primary producer in wetland ecosystems (Reynolds et al. Citation2002). The phytoplankton community distribution patterns in wetland ecosystems are determined mainly by richness and abundance (Zhang and Zang Citation2015). Most researchers agree that excess nitrogen and phosphorus inputs from anthropogenic activities have influenced phytoplankton richness and abundance (Yang et al. Citation2016). Some studies have shown that nitrogen and phosphorus levels are relatively high in the wetlands of NE China (Gong et al. Citation2010, Zhang and Zang Citation2015). Based on a comparison of survey results, 1364 × 104 ind./L (in LFW) abundances obtained in this present study were consist with those noted eutrophic wetland studies in NE China. For example, a study conducted in the Zhalong Wetland, located in the Songnen Plain, NE China, showed that the mean abundance of phytoplankton is 1318 × 104 ind./L. Another study conducted on rivers from Songnen Plain revealed that the mean abundance of phytoplankton in the Mudanjiang River is 257 × 104 ind./L. The nutrient concentrations Mudanjiang River was similar with HLHW and had likely phytoplankton abundance (Yu et al. Citation2012). Our results are consistent with previous studies where the phytoplankton abundance in the eutrophic conditions show a significant upward trend (Almanza et al. Citation2016). The uptake of nutrients is regarded as one of the key factors influencing phytoplankton behavior (Reynolds et al. Citation2002). Furthermore, the availability of nutrients is closely associated with phytoplankton ecological distribution (Salmaso and Padisak Citation2007). This can be seen in the LFW, where the dominant phytoplankton species, such as FG W1, J, C, and D, occurred in eutrophic conditions (). The RDA revealed that all three functional classification approaches were regulated by TN and TP. The t test showed that the TP concentration of LFW was significantly higher than HLHW. Another study found that the TP content in water can meet the needs of phytoplankton if it exceeds 0.03 mg L−1 (Reynolds et al. Citation2002). Prior study has shown that where phosphorus is 0.1 mg/L, Scenedesmus can accumulate lipids as high as 53% (Li et al. Citation2010). In our study, the phosphorus concentration in LFW averages 1.32 mg/L. High TP concentration promotes the growth of Scenedesmus (Group J). This finding suggests that the TP concentration is a key predictor for the life strategy of Group J. Prior studies have revealed that excessive input of TN into the water column will lead to species simplification, impair the evenness, and cause a sudden increase in abundance (algal bloom) (Reynolds Citation1994). In our study, the highest TN concentration was recorded in LFW, indicating eutrophic conditions there. The FGs J, D, X1, and W1—which are associated with mesotrophic to eutrophic conditions—were positively correlated with TN. The results suggest eutrophication caused by excess TN input improved the growth of phytoplankton. Our results demonstrated the importance of nutrient enrichment to phytoplankton assemblages. EC levels in natural freshwater should influence the growth of phytoplankton, and high concentrations of inorganic suspended solids might promote the growth of Trachelomonas and Synedra (Gogoi et al. Citation2021, Hasirci Mustak and Tezel Ersanli Citation2015). In our study, RDA showed that high EC could promote the growth and colonization of Synedra and Cyclotella. This finding is consistent with our previous benthic diatom community study during ice cover from the Lalin River (Lu et al. Citation2020). That study showed that the relative abundance of Synedra greatly increased with increasing EC concentration. Synedra could serve as a signal of eutrophication in rivers (Masouras et al. Citation2021). Most studies have concluded that low pH has led to decreased functional diversity in the Brunei River estuary (Landsberg Citation2002). Our study showed that pH was closely related to the dominant species that inhabited eutrophic conditions. This suggests that neutral to alkali pH with high nutrient levels contributed to the growth and colonization of pioneer species in the wetlands of NE China.
Conclusions
In this study, we used three functional classification approaches to describe the phytoplankton community response to environmental heterogeneity. Our results showed that all three functional classification approaches (FGs, MFGs, and MBFG) could characterize the phytoplankton community response to environmental heterogeneity. In addition, we found that MBFG had the highest cumulative percentage variance of species composition. Therefore, we suggest using MBFG as a routine tool to characterize environmental heterogeneity. Ultimately, TN, TP, EC, and pH were key predictors for the phytoplankton community ecological distribution pattern in NE China wetlands.
Acknowledgements
We thank Miss Nan Song for sample collection. We thank LetPub (www.letpub.com) for its linguistic assistance during the preparation of this manuscript.
Disclosure statement
No potential conflict of interest was reported by the authors.
Data availability statement
The processed data required to reproduce these findings cannot be shared at this time as the data also forms part of an ongoing study.
Additional information
Funding
Notes on contributors
Xinxin Lu
Dr. Xinxin Lu is research associate of College of Life Science and Technology of Harbin Normal University. His research currently focuses on theory of plankton metacommunity assembly, water quality assessment and biodiversity.
Fengyang Sui
Dr. Fengyang Sui work in College of Life Science and Technology of Harbin Normal University, her study focuses on the Physiology.
Yan Liu
Yan Liu and Yawen Fan are Professor of College of Life Science and Technology of Harbin Normal University. Their research focuses on diatom taxonomy.
Yawen Fan
Yan Liu and Yawen Fan are Professor of College of Life Science and Technology of Harbin Normal University. Their research focuses on diatom taxonomy.
References
- Abonyi A, Leitao M, Stankovic I, Borics G, Varbiro G, Padisak J. 2014. A large river (River Loire, France) survey to compare phytoplankton functional approaches: Do they display river zones in similar ways? Ecol Indic. 46:11–22.
- Almanza V, Parra O, Bicudo CEDM, Gonzalez MA, Lopez M, Urrutia R. 2016. Phytoplankton blooms and changes in the phytoplankton community structure in three eutrophic shallow lakes of Central Chile. Gayana Bot. 73(2):191–205.
- Amorim CA, Valenca CR, de Moura-Falcao RH, AdN M. 2019. Seasonal variations of morpho-functional phytoplankton groups influence the top-down control of a cladoceran in a tropical hypereutrophic lake. Aquat Ecol. 53(3):453–464.
- Anonymous. 2011. Freshwater Algal Flora of the British Isles: An Identification Guide to Freshwater and Terrestrial Algae. In: John DM, Whitton BA, Brook AJ, editors. 2nd ed. p. 1–5.
- Bortolini JC, Bueno NC. 2017. Temporal dynamics of phytoplankton using the morphology-based functional approach in a subtropical river. Braz J Bot. 40(3):741–748.
- Bortolini JC, Rodrigues LC, Jati S, Train S. 2014. Phytoplankton functional and morphological groups as indicators of environmental variability in a lateral channel of the Upper Paraná River floodplain. Acta Limnol Bras. 26(1):98–108. 2014–03
- Bunn SE, Arthington AH. 2002. Basic principles and ecological consequences of altered flow regimes for aquatic biodiversity. Environ Manage. 30(4):492–507.
- Celik K, Sevindik TO. 2015. The phytoplankton functional group concept provides a reliable basis for ecological status estimation in the Caygoren Reservoir (Turkey). Turk J Bot. 39:588–598.
- Chengxue M, Hongxian Y, Shuaijun C. 2011. The community dynamics of phytoplankton with different degrees of human impact in cold water region, a case study in northeast China. 2011 International Symposium on Water Resource and Environmental Protection (ISWREP). 2011:p. 2311–2313.
- Dudgeon D, Arthington AH, Gessner MO, Kawabata ZI, Knowler DJ, Leveque C, Naiman RJ, Prieur-Richard AH, Soto D, Stiassny MLJ, et al. 2006. Freshwater biodiversity: importance, threats, status and conservation challenges. Biol Rev Camb Philos Soc. 81(2):163–182.
- Fernandez C, Caceres EJ, Parodi ER. 2014. Phytoplankton Development in a Highly Eutrophic man-made Lake From the Pampa plain of Argentina-a functional Approach. Int J Environ Res. Win. 8:1–14.
- Gogoi P, Kumari S, Sarkar UK, Lianthuamluaia L, Puthiyottil M, Bhattacharjya BK, Das BK. 2021. Dynamics of phytoplankton community in seasonally open and closed wetlands in the Teesta-Torsa basin. India, and management implications for sustainable utilization. Environ Monitoring Assessment. 193:810.
- Gong P, Niu ZG, Cheng XA, Zhao KY, Zhou DM, Guo JH, Liang L, Wang XF, Li DD, Huang HB, et al. 2010. China's wetland change (1990-2000) determined by remote sensing. Sci China Earth Sci. 53(7):1036–1042.
- Gu NT, Song QB, Yang XL, Yu XB, Li XM, Li G. 2020. Fluorescence characteristics and biodegradability of dissolved organic matter (DOM) leached from non-point sources in southeastern China. Environ Pollut. 258:113807.
- Hasirci Mustak S, Tezel Ersanli E. 2015. Spatial and temporal characterization of the physicochemical parameters and phytoplankton assemblages in Dodurga Reservoir (Sinop, Turkey). Turk J Bot. 39:547–554.
- Izaguirre I, Allende L, Escaray R, Bustingorry J, Perez G, Tell G. 2012. Comparison of morpho-functional phytoplankton classifications in human-impacted shallow lakes with different stable states. Hydrobiologia. 698(1):203–216.
- Ji ZH, Long ZW, Zhang Y, Wang YK, Qi XY, Xia XH, Pei YS. 2021. Enrichment differences and source apportionment of nutrients, stable isotopes, and trace metal elements in sediments of complex and fragmented wetland systems. Environ Pollut. 289:117852.
- Jin Y, Yu R, Zhang Z, Zhang Q, Li M, Cao Z, Wu L, Hao Y. 2020. Spatiotemporal variability of phytoplankton functional groups in a shallow eutrophic lake from cold, arid regions. Environ Monit Assess. 192:371.
- Lai J. 2013. Canoco 5: a new version of an ecological multivariate data ordination program. Biodiversity Sci. 21:765–768.
- Landsberg JH. 2002. The effects of harmful algal blooms on aquatic organisms. Rev Fisheries Sci. 10(2):113–390.
- Li X, Hu HY, Gan K, Sun YX. 2010. Effects of different nitrogen and phosphorus concentrations on the growth, nutrient uptake, and lipid accumulation of a freshwater microalga Scenedesmus sp. Bioresour Technol. 101(14):5494–5500.
- Liu JY, Zhang ZX, Xu XL, Kuang WH, Zhou WC, Zhang SW, Li RD, Yan CZ, Yu DS, Wu SX, et al. 2010. Spatial patterns and driving forces of land use change in China during the early 21st century. J Geogr Sci. 20(4):483–494. Aug
- Lu X, Liu Y, Fan Y. 2020. Diatom taxonomic composition as a biological indicator of the ecological health and status of a River Basin under agricultural influence. Water. 12(7):2067.
- Masouras A, Karaouzas I, Dimitriou E, Tsirtsis G, Smeti E. 2021. Benthic diatoms in river biomonitoring-present and future perspectives within the water framework directive. Water. 13(4):478.
- Naselli-Flores L. 2014. Morphological analysis of phytoplankton as a tool to assess ecological state of aquatic ecosystems: the case of Lake Arancio, Sicily, Italy. IW. 4(1):15–26.
- Oguz A, Akcaalan R, Koker L, Gurevin C, Dorak Z, Albay M. 2020. Driving factors affecting the phytoplankton functional groups in a deep alkaline lake. Turk J Bot. 44(6):633–646.
- Olofsson P, Foody GM, Herold M, Stehman SV, Woodcock CE, Wulder MA. 2014. Good practices for estimating area and assessing accuracy of land change. Remote Sens Environ. 148:42–57.
- Pereira Souza Lobo MTM, Nogueira IS, Sgarbi LF, Kraus CN, Bomfim EO, Garnier J, Marques DM, Bonnet M-P. 2018. Morphology-based functional groups as the best tool to characterize shallow lake-dwelling phytoplankton on an Amazonian floodplain. Ecol Indic. 95:579–588.
- Puglisi L, Adamo MC, Baldaccini NE. 2005. Man-induced habitat changes and sensitive species: a GIS approach to the Eurasian Bittern (Botaurus stellaris) distribution in a Mediterranean wetland. Biodivers Conserv. 14(8):1909–1922.
- Reid AJ, Carlson AK, Creed IF, Eliason EJ, Gell PA, Johnson PTJ, Kidd KA, MacCormack TJ, Olden JD, Ormerod SJ, et al. 2019. Emerging threats and persistent conservation challenges for freshwater biodiversity. Biol Rev Camb Philos Soc. 94(3):849–873.
- Reynolds CS, Huszar V, Kruk C, Naselli-Flores L, Melo S. 2002. Towards a functional classification of the freshwater phytoplankton. J Plankton Res. 24(5):417–428.
- Reynolds CS. 1994. The long, the short and the stalled: On the attributes of phytoplankton selected by physical mixing in lakes and rivers. Hydrobiologia. 289(1–3):9–21.
- Salmaso N, Padisak J. 2007. Morpho-functional groups and phytoplankton development in two deep lakes (Lake Garda, Italy and Lake Stechlin, Germany). Hydrobiologia. 578(1):97–112.
- Sim DZH, Mowe MAD, Song Y, Lu J, Tan HTW, Mitrovic SM, Roelke DL, Yeo DCJ. 2021. Tropical macrophytes promote phytoplankton community shifts in lake mesocosms: relevance for lake restoration in warm climates. Hydrobiologia. 848(20):4861–4884.
- Utermöhl H. 1958. Zur Vervollkommnung der quantitativen Phytoplankton-Methodik. SIL Communications, 1953-1996. 9(1):1–38.
- Wang M. 2002. Evaluate method and classification standard on lake eutrophication. Environ Monit China. 18:47–49.
- Wang XD, Zang SY. 2014. Distribution characteristics and ecological risk assessment of toxic heavy metals and metalloid in surface water of lakes in Daqing Heilongjiang Province, China. Ecotoxicology. 23(4):609–617.
- Wilson JR, Wilkerson FP, Blaser SB, Nielsen KJ. 2021. Phytoplankton community structure in a seasonal low-inflow estuary adjacent to coastal upwelling (Drakes Estero, CA, USA). Estuaries Coasts. 44(3):769–787.
- Xiao Q, Zong YT, Lu SG. 2015. Assessment of heavy metal pollution and human health risk in urban soils of steel industrial city (Anshan), Liaoning, Northeast China. Ecotoxicol Environ Safety. 120:377–385.
- Yang B, Jiang Y-J, He W, Liu W-X, Kong X-Z, Jorgensen SE, Xu F-L. 2016. The tempo-spatial variations of phytoplankton diversities and their correlation with trophic state levels in a large eutrophic Chinese lake. Ecol Indic. 66:153–162.
- Yu HX, Wu JH, Ma CX, Qin XB. 2012. Seasonal dynamics of phytoplankton functional groups and its relationship with the environment in river: a case study in northeast China. J Freshwater Ecol. 27(3):429–441.
- Zedler JB, Kercher S. 2005. Wetland resources: Status, trends, ecosystem services, and restorability. Annu Rev Environ Resour. 30(1):39–74.
- Zhang NN, Zang SY. 2015. Characteristics of phytoplankton distribution for assessment of water quality in the Zhalong Wetland, China. Int J Environ Sci Technol. 12(11):3657–3664.
- Zhao YW, Dong BQ, Li ZM, Cheng GH, Zhou LQ. 2018. Dynamic simulation of a duckweed-dominated wetland in north China based on a system dynamics model. Ecol Indic. 92:268–277.
- Zhong Q, Xue B, Abu Noman M, Wei Y, Liu H, Liu H, Zheng L, Jing H, Sun J. 2021. Effect of river plume on phytoplankton community structure in Zhujiang River estuary. J Ocean Limnol. 39(2):550–565.