Abstract
In this article, we use local indicators of spatial association (LISA) and other spatial analysis techniques to analyze the distribution of centers with high employment density within metropolitan areas. We examine the 359 metropolitan areas across the United States at three points in time (1990, 2000, and 2010) to provide a spatio-temporal panoramic of urban spatial structure. Our analysis highlights three key findings. (1) The monocentric structure persists in a majority of metropolitan areas: 56.5% in 1990, 64.1% in 2000, and 57.7% in 2010. (2) The pattern of employment centers remains stable for most metropolitan areas: the number of centers remained the same for 74.9% of metropolitan areas between 1990 and 2000 and for 85.2% between 2000 and 2010. (3) Compared with monocentric metropolitan areas, polycentric metros are larger and more dense, with higher per-capita incomes and lower poverty rates.
Introduction
Urban economics and urban geography have long been shaped by theoretical and empirical debates over monocentricity and polycentricity, regarding the internal structure of metropolitan regions and the number of centers with high employment density. Theoretical debates can be traced all the way back to von Thunen’s (Citation1826) “isolated state” model, which portrayed a monocentric market with concentric rings where agricultural land uses with the highest transport costs and highest revenues were found in the rings closest to the center. This is the essence of the monocentric “bid-rent” model, which was refined and adapted to replace rural farmers with urban commuters: Alonso (Citation1964), Mills (Citation1972), and Muth (Citation1969) extended the basic model to account for land-use competition between industrial production, commercial and retail activities, and residential areas. The monocentric urban model centered on a single Central Business District (CBD) dominated urban analysis for decades and was definitively integrated into a unified economic framework by Fujita (Citation1989). The a priori assumption of the CBD reinforced the simplicity and formal analytical elegance of the monocentric model as a dominant paradigm in the development of urban economic theory in the latter half of the twentieth century.
Yet the validity of the monocentric approach can be questioned from alternative perspectives. In theoretical terms, Wheaton (Citation1979), Griffith (Citation1981), and Berry and Kim (Citation1993) have challenged key assumptions of the classical model formulations. On the other hand (and more decisively from those fields concerned with public policy) the undeniable empirical experience of urbanism challenged the hegemony of Alonso monocentricity: many cities evolved into structures with multiple employment centers, exposing the stark contrast between monocentric model simplicity and the more complex continencies of a sophisticated, often confusing reality. There is an extensive empirical literature on polycentric employment structures, which we will analyze later in this study: key contributions were showcased in the special issues of Regional Science and Urban Economics (1991) on “Causes and Consequences of Changing Urban Form” and in Geographical Analysis on “The Multimodal Metropolis.”
In this context, Fujita and Ogawa (Citation1982) developed a theoretical model of urban policentricity with the possibility of multiple equilibria. The key assumption involves the insight that the benefits from interactions between firms is a negative exponential function of distance (in contrast to the traditional assumption of a linear relation). Subsequent inquiry (such as that of Fujita & Krugman, Citation1995) consolidated a major stream of the literature now recognized as the New Economic Geography. One of the fundamental limitations of the monocentric model—its exogenous logic premised on an a priori supposition of a single CBD—is now superceded. Current models (monocentric as well as polycentric) deduce employment centers endogenously, based on the behaviors of agents or circumstances of urban form (see White, Citation1999 for a review and synthesis).
All of these theoretical considerations are necessary to provide a framework for our purposes in this article; but this is not a theoretical contribution. Rather, we hope to inform the debate on monocentricity and polycentricity in empirical terms. There is an emerging consensus that above a certain size threshold, modern metropolitan areas become polycentric—and that most metropolitan areas in developed countries are in this category. Put simply, the monocentric model—previously so important in mainstream urban theory—might have become obsolete when dealing with the real world. In this article we evaluate this proposition with rigorous empirical tests for metropolitan areas in the United States. Our analysis yields three main results. First, monocentricity retains a substantial influence on the intraurban structure of many metropolitan areas. Second, polycentric metropolitan areas are larger and more dense and have higher per capita incomes with lower poverty rates compared with monocentric equivalents. Third, there is no clear evolutionary trend toward polycentricity between 1990 and 2010: employment centers proliferated in some metropolitan areas and declined in number elsewhere—but remained constant in most metropolitan regions.
The article is structured as follows. In the next section we present a survey of the most recent trends defining intraurban structures in modern cities. In the third section we offer an overview of the empirical literature on the measurement of centers. Our methodology is described in the fourth section. The fifth section is devoted to the study area and data. In the sixth and longest section we give a detailed account of the results of our empirical analysis. The final section summarizes the results and implications for urban theory.
Polycentricity … and beyond
Modern cities exhibit great complexity in their internal structure; like living beings, cities evolve over time, sometimes remarkably quickly. Many different factors influence this process: demographic, social, economic, geographic, political, cultural (and others more directly related to the postulates of the New Economic Geography, such as circular causation, inertia or path dependence; see Lee, Citation2007 and García-López & Muñiz, Citation2010). In this section we draw on the geography and urban studies literature to describe the most significant changes experienced by US metropolitan areas in the last quarter century. This literature survey helps contextualize the results of our empirical investigation amidst the debates on monocentricity and polycentricity.
There is a general consensus that in recent decades a phenomenon of intrametropolitan employment decentralization has emergedFootnote1: the traditional CBD has lost importance as an employment center in relative terms and sometimes also in absolute terms (Shearmur, Coffey, Dube, & Barbonne, Citation2007). Where this employment deconcentration has led, however, is a question where agreement is more difficult to reach.
One school of thought, inspired by Garreau’s (Citation1991) work on edge cities, portrays the erosion of monocentricity leading to the formation of multiple employment subcenters. This “suburban downtown” phenomenon can be defined as the process by which employment leaves the CBD and recentralizes in an orderly and compact fashion in new poles or nodes that constitute a polycentric structure—with fairly clear boundaries separating distinct edge cities. Garreau’s (Citation1991) criteria identified 45 downtowns, 119 edge cities, and 73 emerging edge cities in 35 metropolitan areas across the United States.
An opposing view is offered by Gordon and Richardson (Citation1996), who analyze the city so frequently cited as the supreme instance of polycentricity—Los Angeles. Between 1970 and 1990, the decentralization of employment did not lead to polycentricity, but rather a pattern of generalized dispersion (Gordon & Richardson, Citation1996).Footnote2 In a similar analysis of Atlanta, Fujii and Hartshorn (Citation1995) identify a pattern of “scatteration.” Furthermore, Lang (Citation2003) coins the term “edgeless city” as an opposite of the edge cities, to describe an emergent metropolitan form of highly dispersed employment patterns. In this school of thought, polycentricity is merely an intermediate, transitory phase between a historically inherited monocentricity and a future pattern beyond the present configuration of multiple centers.Footnote3
Which of these two approaches is most realistic from a theoretical point of view? There are arguments in favor of both. To simplify a multi-faceted debate, everything depends on the trade-offs between the intensity of agglomeration economies versus the ubiquity of automobile transport and advanced communications technologies. High agglomeration economies, in which distance and face-to-face contact remain relevant, favor an ordered structure where subcenters arise endogenously to generate a polycentric pattern. By contrast, transportation and communication innovations can make economies of agglomeration available across an entire metropolitan area: economic agents can be scattered across locations where they enjoy agglomeration economies while incurring lower congestion costs and lower land and housing prices. The result is a more unstructured and scattered pattern of employment. Ultimately, these trade-offs are region- and industry-specific, insofar as economies of agglomeration and new transport and communications advances are contingent upon industrial sector and regional context (Lee, Citation2007).
The empirical literature highlights this contingency. In the metropolitan area of Barcelona, Muñiz and Garcia-López (Citation2010) find evidence that physical proximity matters for knowledge-intensive activities, creating a pattern of organized growth in subcenters between 1991 and 2001. A similar set of findings that support polycentricity and the importance of face-to-face contacts is provided by analyses of Vienna’s service sector in 2006 (Helbich, Citation2012; Helbich & Leitner, Citation2010). García-López and Muñiz (Citation2010) also deduce a nuanced polycentric pattern for employment in Barcelona in 1986 and 2001. In contrast, Lang, Sanchez, and Oner’s (Citation2009) analysis of 2005 data confirms the “edgeless” character of 13 large US metropolitan regions, where commercial office space development exhibits a seemly random scattering across the metropolitan fabric.
Empirical measurement of employment centers
Given its importance to the theoretical essence of urban economics, the literature on employment centers is extensive and remarkably wide in scope. While we have already highlighted some of the main theoretical milestones in the literature, in this section we focus on one particular issue: the empirical identification of urban employment centers. This issue remains a contested domain in the literature, with no clear consensus. Below, we review the main techniques in use and present a case for a method that incorporates exploratory spatial data analysis (ESDA).
Although there is no single, clear definition of an employment center, there is widespread agreement on general characteristics: as McMillen and Smith (Citation2003) emphasize, a center is defined as an area with “significantly higher” employment densities compared to surrounding areas. It is also generally recognized that an employment center will be sufficiently large to have a significant effect on the overall spatial structure of the urban area, leading to local increases in population density, land prices, and/or housing prices. Finally, Giuliano and Small (Citation1991) point out that the areas making up the center need to be contiguous. Beyond these areas of conceptual consensus, however, there is no agreement on which measurements and methodologies should be used for the empirical detection of employment centers; many alternative criteria are used, each with their own strengths and weaknesses. A brief (and far from comprehensive) review highlights four main approaches.
The most intuitive and popular approach is the method devised by Giuliano and Small (Citation1991). Examining the case of Los Angeles, they propose the following definition: a continuous set of zones, each with a density score above a cutoff D, that together have at least E total employment and for which all immediately adjacent zones outside the subcenter have density below D. The peak of the center is then defined as the area within the cluster with the highest density. Once the cutoff is chosen, it is straightforward to recognize which areas constitute a center, and which ones do not. The problem, however, is a priori: defining a density level is very subjective, and as Giuliano and Small (Citation1991) demonstrate, the final result (and number of centers identified) depends critically on the threshold used. Another drawback of the method is that cutoffs appropriate for one city may not be relevant for another—complicating efforts to compare the findings of different case studies.
A second approach, exemplified in the work of Craig and Ng (Citation2001), employs spline quantiles to estimate density functions of the distance to the CBD and to identify centers as areas of rising density. Although this method does not require an arbitrary or subjective cutoff, the method is only valid when the CBD is known ex ante, which implies the prerequisite of relevant local contextual knowledge. Additionally, as McMillen (Citation2001) observes, the procedure is really detecting rings of high density rather than actual centers, and thus is best suited for monocentric cities.
A third method, illustrated by McMillen (Citation2001), McMillen and Smith (Citation2003), and McMillen (Citation2004), involves a two-stage approach based on locally weighted regression; in the first stage, a density surface is created to be used in the second stage as a benchmark to analyze the signs of residuals in order to pick as a center those significantly positive. The advantage of this method is that it involves less subjectivity than the cutoff approach, since the decision of which areas are defined as centers depends on the statistical significance of the residuals. However, as Griffith and Wong (Citation2007) point out, it requires the assumption that employment density follows some general pattern from which centers positively deviate.
A fourth and more recent strand of the literature entails the use of ESDA and local measures of spatial autocorrelation to identify centers. The approach was first used by Paez, Uchida, and Miyamoto (Citation2001), and other examples include Baumont, Ertur, and Le Gallo (Citation2004), Riguelle, Thomas, and Verhetsel (Citation2007), Griffith and Wong (Citation2007), and Rodríguez-Gámez and Dallerba (Citation2012). These explicitly spatial techniques have been used in other fields to detect statistically significant spatial clusters or hot-spots; when applied to the study of employment centers, the approach is effective and successful for identifying centers. Furthermore, it may be used in ways that do not require qualitative local knowledge or a priori assumptions about the distribution of local employment.
It is in this context of the literature that our contribution is situated. We aim to provide empirical insight in order to inform the debates on monocentricity and polycentricity. To do this, we have made four key methodological decisions. The first is temporal; instead of the conventional approach of choosing a single point in time, we analyze data for 1990, 2000, and 2010 to determine whether the trend is toward greater polycentricity. Second, instead of the traditional focus on one or a few metropolitan areas,Footnote4 we consider all 359 metropolitan statistical areas (MSAs) defined in the United States at the end of the twentieth century. The third decision relates to the spatial scale of data aggregation used as a basic unit of reference; this issue is far from trivial, of course, given the importance of the well-known modifiable area unit problem (MAUP) (Openshaw & Taylor, Citation1981).Footnote5 With such considerations in mind, in this study we take the census tract as the basic unit of reference. In our judgment, this offers two important advantages: census tracts are generally designed to remain as stable as possible over time (a crucial issue when more than one time period is studied), and tracts can be readily linked with economic data that allow us to go beyond the simple monocentricity/polycentricity dichotomy, to develop more meaningful metropolitan classifications.
The fourth and final decision is the specific analytical procedure. Given that we consider an entire national metropolitan system, it is infeasible to use methods requiring detailed local knowledge. An objective technique is required to maintain direct comparisons while identifying differentiating elements of each metropolitan region. With these requirements in mind, we found the ESDA family of methods to be particularly well suited for our purposes; in particular, the local indicators of spatial association (LISA) technique is valuable for identifying employment centers within each MSA.
Methods
To analyze the evolution of polycentricity in the United States, we draw on advances in ESDA—in particular, the local analytical strengths of LISA—and define a small number of simple rules in order to distill a large volume of data into empirical measures of urban employment centers.
The main purpose of ESDA is to explore phenomena in which space is particularly relevant but where traditional econometric techniques are unlikely to detect meaningful spatial patterns. There are two main types of ESDA tools: global and local. The former give an overall estimate of the presence of spatial autocorrelation over a study area, while the latter are employed to detect heterogeneity and local deviations from the broader, general trends of global indicators. Typical uses of local ESDA tools include the analysis of spatial clusters, hot-spots, and outlier detection. Given the focus of our study, we employ the local version of ESDA to detect foci of significantly high values of employment density. Although several variants of local indicators are in use, we adopt Anselin’s (Citation1995) LISA techniques, which construct local spatial statistics to measure “significant spatial autocorrelation for each location.” The method calculates a local version of the traditional Moran’s I autocorrelation statistic; this is now a widely used and robust approach that allows our results to be compared with others in the literature.Footnote6
Positive values of Ii indicate the presence of positive spatial autocorrelation (of either high or low values) and are used to identify clusters; negative values indicate negative autocorrelation—high values surrounded by low values, or vice versa—and thus identify outlier observations. In order to test for statistical significance, we take the conditional permutation approach, in which each observation i is held fixed and the neighboring values are permutated several times (9,999 in this case) following a spatially random process. The statistic is computed for each of such permutations, enabling the construction of an empirical distribution from which pseudo p-values can be obtained to impute significance.
The local Moran’s I distinguishes between positive and negative spatial autocorrelation; if an observation exhibits a positive value, however, it is not possible to discern whether it is a case of a high value surrounded by high values (hereafter, “HH”) or a low value surrounded by low values (LL). In our particular case, this distinction is crucial in order to identify areas of high employment density (see Griffith & Wong, Citation2007 for a discussion of this issue). For this purpose, we use another ESDA tool, the Moran’s scatterplot (Anselin, Citation1996). This method graphs the variable of interest on the horizontal axis, while presenting on the vertical axis the variable’s spatial lag—an average of the neighboring values for each observation. This allows us to observe what type of relationship each observation has with its neighbors and to distinguish between HH and LL values (which clearly appear in separate quadrants of the scatterplot).
In this study, we develop a set of simple rules to automate the process of selecting areas of high employment density as centers. We consider an area of the city an employment center when it meets the following two requirements:
Its local spatial autocorrelation statistic attains significance at the 10% level.Footnote7
It represents a cluster of high values (HH) or an outlier of high values among low ones (HL).
These two criteria identify all spatial units that qualify to be considered employment centers; we then perform a contiguity test, considering as a single center all those zones that meet the specified conditions and are contiguous. Thus our operational definition (similar to Guillain, Le Gallo, & Boiteux-Orain, Citation2006), is:
An employment center is a contiguous set of spatial units within an urban region, conditional on each spatial unit exhibiting a spatial concentration of high employment density that is significant at the p < 0.10 level.
This conceptualization reflects the key elements of the methodological literature review presented earlier: the approach seeks spots of high employment density and considers contiguous qualifying areas together as integrated entities—but because it is based on simple statistical criteria, it does not require extensive local knowledge to formulate relevant cutoffs. Moreover, since it does not depend on distances to a pre-existing CBD, it is not vulnerable to the implicit bias toward ring-shaped agglomerations; it makes no a priori assumptions regarding the spatial pattern of employment distribution. Since there is no consideration of land prices or population densities, it can be applied to very different contexts. The data requirements are conceptually simple (employment densities at an intraurban level), facilitating empirical analysis across very large databases.
Another crucial decision involves the definition of the spatial weights matrix on which the LISAs are based. This is an N × N matrix W used to formalize the spatial relationships between census tracts within each MSA. Every matrix cell wij signifies the degree of spatial relation between observations i and j, with the general convention of diagonal elements wij = 0. We adopt the well-established, widely used “queen contiguity” criterion, which considers as spatial neighbors any observations that share any length of border (including vertices). Contiguous neighbors are assigned wij = 1, while all remaining cells of W are set to zero; all rows of W are standardized in order to sum to 1. The spatial lag of variable y, Wy, thus becomes the average value of y in the vicinity of each observation.
The methods outlined thus far relate to the detection of high-density employment centers; in our analysis below, however, it will become clear that we can divide the 359 metropolitan areas across the United States into a number of distinctive categories, based on the number of centers in consecutive decades. We can then examine the levels of other relevant economic agglomeration indicators across different metropolitan categories; to measure statistical significance of the cross-category differences, we use the technique known as random labeling (Rey & Sastre-Gutiérrez, Citation2010). This produces pseudo-significance levels based on the empirical distribution obtained through a simulation in which the generated samples are obtained by reshuffling the values of original, observed differences. Given two samples sa and sb of length na and nb, we want to test whether the statistic T (e.g., the mean) shows a statistically equal value. The null hypothesis then is:
First, all elements from both groups are pooled. The procedure generates several samples (in this case, 99,999 which, in turn, implies a minimum possible p-value of 1/100,000 = 0.00001) by randomly reshuffling the pool of values. Each reshuffling creates two groups sa* and sb* of the same size as the originals (na and nb), with each iteration obtaining differences in the statistics from those groups (Ta* and Tb*). This produces an empirical distribution against which we can test the difference of the original two statistics (Ta and Tb) and generate pseudo p-values by applying the following formula:
where Ki is a function that takes the value 1 if the difference between the generated statistics in the i-th permutation is equal to or greater than the observed one, and 0 otherwise.
Taken together, these features make our method suitable for the analysis of a large number of cities at different time periods with a consistent procedure that facilitates comparative evaluation. The methods extract patterns that occur across urban regions, without implicitly privileging thresholds that are particular to only certain types of cities. This is made possible at the cost of abstracting the idea of an urban center from local, contextual considerations. This may seem to be an oversimplification, given the focus of much of the literature on employment centers: most of the studies of polycentricity and urban spatial structure usually only consider one (Craig & Ng, Citation2001; Giuliano & Small, Citation1991) or very few (McMillen, Citation2001) urban regions, and usually only one point in time. Yet the aim of the present study is to complement (rather than challenge or supplant) existing work—to provide a broad overview of the phenomenon of polycentricity in a large world urban region over a 20-year period. With this purpose in mind, we believe our methodological trade-off between local contextual detail versus wider scope and scalability is worthwhile and justified.
Study area and data
We have chosen the United States as the study area for several reasons. It remains the world’s largest economy (according to the IMF, in 2012 its GDP constituted 22.5% of the worldwide total), and excellent data are available for its cities. It is the most closely studied area in the literature devoted to the identification of employment centers; this facilitates comparability with our analysis.
Our analysis focuses on the evolution of urban employment centers from 1990 to 2000 to 2010. To operationalize our analytical procedures, data are required for two types of spatial units: one that measures a broad functional urban region and another disaggregate scale appropriate for the conceptualization of local employment centers. For the former we use MSAs as defined in the June 2003 demarcations issued by the Office of Management and Budget (US Bureau of the Census, Citation2003); metropolitan areas are defined by composite measures of population density and commuting patterns, thus representing well the concept of a regional labor market. For the disaggregate sub-area units, we use census tracts, which are usually delineated to encompass residential populations of approximately 6,000; tract delineations are intended to produce areas that are socioeconomically homogeneous and as stable as possible over time. This decision implies that we can only detect employment centers at any scale the same area or larger than a census tract; without information on the spatial distribution of employment within a tract, we cannot consider this in the delineation of employment centers. This problem can be overcome with, for instance, point-pattern analysis applied to microdata at the firm level (e.g., Helbich, Citation2012), but these data are not available for the three time periods considered. In addition, we believe the scale at which the tract operates is small enough to allow us to capture the main structure present in most urban regions. Both MSAs and tracts offer the advantage of extensive data availability for the years considered. Our data set is comprised of 359 MSAs and more than 52,000 tracts per year ().
Table 1. Data set summary (number of observations).
Two additional data requirements are noteworthy. First, representations of the geographical shape and configuration of MSAs and tracts are extracted from the National Historical GIS (NHGIS) (Minnesota Population Center, Citation2004). Polygon areas and contiguity relationships are also calculated from this source with the use of PySAL (Rey & Anselin, Citation2007). Second, the geographical units are linked to several socioeconomic measures essential for evaluating theories of urban polycentricity. Population, income, and poverty data are obtained from the NHGIS. Employment data by place of work for 1990 and 2000 come from the Census 2000 Special Tabulation Product 64 (stp64), which provides detailed tract-to-tract commuting flows for all metropolitan areas; we consider only urban tracts and sum over inflows to obtain employment totals at the tract level. This product is not available for 2010, however, so we rely on the Census Transportation Planning Products (CTPP) 5-year small-area data, which is based on 2006–2010 American Community Survey (ACS) estimates that provide employment counts at the tract level for 2010.Footnote8
Results: US metropolitan areas in 1990, 2000, and 2010
Evolution of employment centers
We begin our exploration of the results with a series of tabulations of the configuration of employment centers across each of the 359 metropolitan areas. Since the methodology is designed to be consistent and objective across metropolitan areas, while incorporating local spatial structure through the LISA approach, the results provide a robust, comparable view of spatial structure over a 20-year period of major economic and spatial restructuring. A double entry matrix is used (see –) to distinguish changes over decade intervals—the beginning year in rows, the ending year in columns—showing the three possible categories into which a metropolitan region may be classified: (1) no statistically significant center, (2) monocentric, and (3) polycentric.
Table 2. Evolution of polycentricity, 1990–2000 (number of MSAs).
Table 3. Evolution of polycentricity, 2000–2010 (number of MSAs).
Table 4. Evolution of polycentricity, 1990–2010 (number of MSAs).
Four key findings are immediately apparent. First, most metropolitan regions exhibit no change in their status over the time periods studied: stability prevails. Most MSAs fall on the diagonal, representing 74.9% of all metropolitan areas in the 1990–2000 period and 85.2% in the 2000–2010 period; over the longer 20-year interval, this share drops to 71.9%. Second, the number of metropolitan areas with no statistically significant centers dropped markedly in the 1990s (from 19 to 2), while increasingly slightly the next decade (from 2 to 4). Third, there is modest evidence of polycentric evolution. We can view the elements of the matrix above the diagonal as a shift to a greater number of centers, while in contrast those below the diagonal signify movement toward fewer centers.Footnote9 In the 1990s, 13.4% of all MSAs were above the diagonal, while 11.7% were below; in the next decade, 10.0% of all MSAs were above the diagonal, while 4.73% were below. Over the entire 20-year period, MSAs moving toward more centers—the ones above the diagonal—comprised 17.5% of all metropolitan areas, versus 10.6% of MSAs moving toward fewer centers. Additional evidence of a modest evolution of polycentricity (not shown in the tables) is the slight but steady increase in the average number of centers per metropolitan area: from 2.25 in 1990 to 2.28 in 2000 to 2.53 in 2010. Fourth, despite this slight move toward polycentric structure, in general the classical, traditional configuration of monocentricity endures. Much of the urban sub-centers literature has involved researchers choosing one or a few case study metropolitan areas that, a priori, are believed to provide a rich setting in which to examine polycentricity; but when we examine all of the metropolitan areas across the United States, monocentricity dominates. In 1990, 56.5% of all metropolitan areas retain monocentric employment structures. This share rises to 64.1% in 2000, and slips back to 57.7% in 2010.
What are the geographies of these trends? Are there discernable regional patterns in the locations of those metropolitan areas with declining numbers of centers and of those with increasing evidence of polycentricity? To explore these questions, we performed overall spatial autocorrelation tests with Moran’s I (Moran, Citation1948), calculated for the number of centers per MSA for each year, and the difference in the number of centers from 1990 to 2000 and from 2000 to 2010. For this step, a spatial weights matrix is defined on the basis of distances between MSA centroids. We select the widely used k nearest neighbors rule; we experimented with several values to ensure stability of the main findings and thus present here only the results of k = 9. Results indicate that, in the five cases, the null hypothesis of no spatial autocorrelation is rejected at p < 0.05, with the sole exception of the 1990–2000 period; the overall Moran’s I is positive, indicating that similar values tend to cluster together.
The evidence of regional geographical autocorrelation in the spatial structure of metropolitan areas invites further scrutiny. We therefore performed an analysis of local spatial autocorrelation, using the same indicators as for detecting employment centers but now in the national context—where the units are MSAs rather than census tracts. An individual test is performed on each MSA to determine (a) whether the spatial correlation with its neighbors is statistically significant, and (b) if so, the nature of the relationship: if the MSA has a high value of the variable under investigation, surrounded by other MSAs with high values, the result will be a “high-high” classification (other possibilities are high-low, low-high, or low-low). This procedure helps to detect whether trends toward polycentricity—and the overall balance between monocentric and polycentric urban structures—exhibit systematic, statistically significant regional regularities. The analysis was undertaken for the four comparisons that attained statistical significance in the previous step: the number of centers in 1990, 2000, and 2010, as well as the change in the number of centers from 2000 to 2010. Since the results of the first three periods are almost identical, we present the results for the intermediate year 2000 and the change in the number of centers from 2000 to 2010 (see and ).
Figure 1. Regional spatial autocorrelation of metropolitan employment centers, 2000.
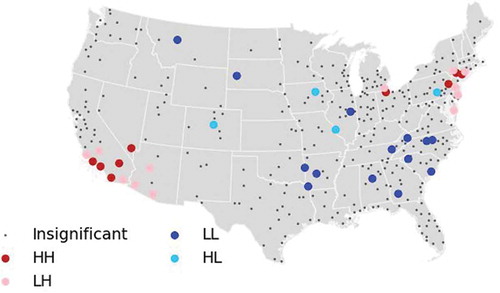
Several findings emerge from the regional spatial analysis of employment centers in 2000 (). Forty of the 359 metropolitan areas (11.1%) exhibit spatially statistically significant distinctions. Nine metropolitan areas attain significance as high-high clusters in terms of the number of centers: four in California, one each in Nevada, Ohio, and Pennsylvania, and two in New York. Fourteen metropolitan areas exhibit regional autocorrelation as low-low—clusters of MSAs with few centers—and 11 of these are in the Southeast. In general, three broad regional complexes can be discerned: (1) polycentric MSAs mixed with nearby metros with fewer centers, in the Southwest; (2) a second mixture of high-high and low-high metros in the Northeast, from New England to Ohio; and (3) low-low metros with few employment centers in the Southeast, from Virginia through the Carolinas, Georgia, and Alabama, as well as Tennessee and Arkansas.
Analyzing the change in the number of centers between 2000 to 2010 () highlights 40 metropolitan areas with statistically significant proximity effects. The evidence indicates that evolution toward fewer employment centers is proceeding in three regions: (1) New Hampshire, Massachusetts, and New Jersey, (2) Indiana and Illinois, and (3) Texas. In contrast, all seven high-high results signifying spatial clustering of metropolitan areas with increasing numbers of centers are in the Southwest, in Arizona, Nevada, and Southern California.
Figure 2. Change in employment centers, 2000–2010.
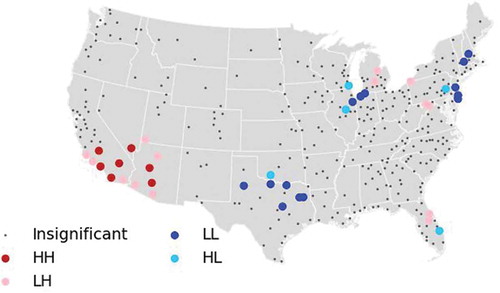
Overall, metropolitan areas with multiple employment centers—and with increases in the number of centers—are associated with Southern California, Nevada, and Arizona. The Southeast is marked by de-centered metropolitan structures. The Great Lakes and New England regions have a dual character: on the one hand, their metropolitan areas have comparatively higher numbers of centers in 2000; on the other hand, the trend in the last decade has been toward fewer centers. De-centralized employment growth is also remarkably pronounced in Texas.
City characterization
For any metropolitan area where employment centers have been delineated, there are nine possible categories in each decade’s comparison of changes (see –). It is thus possible and insightful to take the analysis a step further. It is a simple matter to calculate the average value of several geographic and economic indicators for metropolitan areas within each of the nine categories. Thus, for instance, we can determine whether polycentric cities are more dense than monocentric metros or whether income per capita grows with the number of centers in metropolitan areas. We examine cross-category variation in four variables measured at the metropolitan scale—total population, employment density, income per capita, and the percentage of the population with incomes below the federally defined poverty rate—that are central to urban economic theory. Theoretical debates on “optimum” city size can be traced to the seminal work of Henderson (Citation1974), and the positive correlation between density and productivity is today a widely understood stylized fact, based largely on the work of Ciccone and Hall (Citation1996). Theoretical work on economies of agglomeration (see Fujita & Thisse, Citation2002 for a review) predicts higher incomes and lower poverty rates for polycentric urban regions.
We analyzed the mean values for our chosen indicators for each of the nine categories based on changes between 2000 and 2010 (results for the 1990–2000 period are very similar). The random labeling technique is used to assess differences from the global average for each indicator, yielding pseudo p-values to estimate statistical significance; subindexes, + and −, denote group averages significantly above and below the global mean (). Results are presented for the mean values of the variables in the year 2000; tables based on 1990 and 2010 values are substantively identical.
Table 5. Metropolitan population.
The average metropolitan area in 2000 had a population just over 640 thousand. For metropolitan areas without statistically significant employment centers either in 2000 or in 2010 (one MSA—see ), the population is just under 58,000. Populations increase systematically as we proceed down the diagonalFootnote10 of , indicating a direct and monotonic relationship between size and number of centers. Metropolitan areas that remain polycentric have average populations over 1.54 million, versus 197 thousand for metros that remain monocentric.
Metropolitan job density (measured in total employment divided by total area in square kilometers) also exhibits a systematic, increasing trend as we proceed down the matrix diagonal (). Employment densities for monocentric cities are significantly below the global average, while that for polycentric cities is significantly above average. Metropolitan areas with multiple centers in both years have a higher average employment density than any other of the nine categories.
Table 6. Metropolitan employment density.
Income per capita is no less important than size and density (). Once again, the mean values increase systematically and monotonically as we move down the matrix diagonal. Monocentric metropolitan areas have significantly lower per capita incomes compared with the global mean, while metropolitan areas that were polycentric at the beginning and end of the decade have the highest per capita incomes.
Table 7. Metropolitan per capita income.
Finally, poverty rates () diverge between monocentric and polycentric metropolitan areas. Metropolitan areas which began and ended the decade with monocentric structure have poverty rates that are significantly higher than the global mean; consistently monocentric metropolitan areas have poverty rates significantly below the global average.
Table 8. Metropolitan percentage of population below the poverty line.
Overall, metropolitan areas with a consistently polycentric employment center between 2000 and 2010 were significantly larger, more dense, with higher per capita incomes, and lower poverty rates compared to monocentric metropolitan regions.
Conclusions
In this empirical study of urban economic agglomeration, our goal has been to analyze the extent and evolution of polycentric and monocentric employment structures. We sought to provide the most extensive view of metropolitan employment structure possible, by analyzing some 359 metropolitan regions for the period between 1990 and 2010. Moving beyond the common tendency in the literature to focus on one or a handful of metropolitan areas, we developed a simple yet explicitly spatial method for detecting employment centers—incorporating LISA—that captures variations in urban structure without requiring extensive qualitative local knowledge.
Our analysis yields three fundamental results. First, monocentric metropolitan regions remain the most prevalent, accounting for 56.5% of all metropolitan areas in 1990, 64.1% in 2000, and 57.7% in 2010. This finding contrasts with the general consensus of the literature on employment centers, which emphasizes the polycentric nature of contemporary urbanization. Yet this is only an apparent paradox; from a theoretical (Fujita & Ogawa, Citation1982) and empirical perspective, a certain size threshold must be attained for an urban region to become polycentric. Thus the stylized fact correlating population with the number of employment centers (McMillen & Smith, Citation2003), coupled with the fact that most empirical studies focus on one or a few large metropolitan areas, sustains a widespread illusion of pervasive polycentricity. Our extensive analysis of 359 metropolitan areas includes a wide range of large, medium, and small metropolitan areasFootnote11—and most of the small and medium-sized areas retain a monocentric structure.
Second, our analysis indicates general continuity in metropolitan employment structure, with only modest changes. In the 1990s, 74.9% of all metropolitan areas retained the same number of employment centers, 13.4% saw an increase, and the remaining 11.7% experienced a reduction; in the next decade, the corresponding figures were 85.2%, 10.0%, and 4.73%. In light of these data, we cannot deduce any clear increase in polycentricity; rather, we find “a little bit of everything.” These results are in line with an emerging stream of the literature emphasizing that the evolution of urban structures is not a one-way process reducible to a single pattern or trajectory; different and sometimes opposing tendencies coexist and develop at the same time. Lee (Citation2007), for example, analyzes six US metropolitan areas from 1980 to 2000 and deduces that two of them produced job dispersion, polycentricity was strengthened in two others, and in the other two the CBD remained dominant. Such nonlinear and nonuniform evolution is also demonstrated in Shearmur et al.’s (Citation2007) analysis of three Canadian metropolitan areas between 1996 and 2001; their findings lead them to recommend that inquiry should look beyond the “edge/edgeless” or “polycentricity/scatteration” dichotomies, in order to acknowledge that these phenomena and others (chaos versus order) are occurring at the same time and in the same places. (Shearmur et al., Citation2007, p. 1733). Shearmur and Hutton (Citation2011) reach a similar conclusion in a study of five Canadian metropolitan areas from 1981 to Citation1996, finding patterns that are anything but homogeneous in terms of employment distribution and growth trajectories. All of these findings attest to the complexityFootnote12 noted earlier in the study of the trajectories of modern metropolitan regions.
Third, our classification of metropolitan areas according to the evolution of their internal structure reveals systematic differences in indicators of agglomeration economies. On average, polycentric metropolitan areas are larger, with higher employment densities, higher per capita incomes, and lower poverty rates when compared with monocentric metropolitan areas. These results are consistent with theories of the positive externalities of agglomeration; on average, the densest areas are the most productive, sustaining higher levels of economic output per worker (see Ciccone & Hall, Citation1996 for analysis of US data, and Ciccone, Citation2002 for European data) and thus generating higher per capita incomes and lower poverty rates. The mechanisms of this virtuous cycle—greater size and density creating still larger size and thus greater productivity and higher incomesFootnote13—were first noted by Marshall (Citation1890) and have been systematized and updated by Duranton and Puga (Citation2004). The microfoundations of positive externalities associated with size and density are driven by the urbanized efficiencies of sharing, matching, and learning processes. All of these results merit a working hypothesis that polycentric metropolitan growth is associated with higher levels of economic development; yet, beyond the scope of our analysis here, it remains to be determined the precise nature of the causality. Do more employment centers, all else constant, nurture greater economic growth? Or does economic development foster the proliferation of urban employment centers?
Two extensions of our work offer fruitful lines of inquiry. First, it is worth exploring the robustness of our conclusions if alternative methods are used to detect employment centers. Second, it is important to complement our “extensive” analysis with further “intensive” refinements. Our broad, extensive, and exploratory analysis provides key insights on metropolitan employment structure from a fully-representative sample of hundreds of metropolitan areas; yet formal, multivariate spatial-econometric analyses are required for in-depth analyses to disentangle the interrelations of economic development, size, and other factors on the changing internal spatial structure of metropolitan regions. It is encouraging indeed that some research along these lines is already yielding new insights (e.g., Arauzo-Carod & Viladecans-Marsal, Citation2009; García-López & Muñiz, Citation2013; Meijers & Burger, Citation2010).
Additional material
Download PDF (82.8 KB)Notes
1. Although the consensus exists, it is not unanimous: Forstall and Greene (Citation1997) find no clear support for either a concentration or a deconcentration hypothesis in Los Angeles between 1980 and 1990.
2. Leslie and Huallacháin (Citation2006) affirm that at present Phoenix is the typical example of a large, sprawling metropolitan area with very fast demographic growth.
3. It is worth noting that Gordon and Richardson (Citation1996) choose to begin the title of their analysis with “Beyond Polycentricity.”
4. McMillen and Smith (Citation2003) identified subcenters for 62 large urban areas in the United States. Another notable exception is Sarzynski, Galster, and Stack (Citation2013), who investigate spatial patterns of land use for 257 US metropolitan areas.
5. Of course, the best way to overcome the MAUP is to carry out a complete sensitivity analysis, taking into account all the possible different geographical units. This is a formidable task, which exceeds the aims of this article. In one of the sections below we use census tracts as the primary unit of analysis; in another section we examine variations across MSAs.
6. Alternatives to Moran’s I have been suggested in the literature and present interesting opportunities for future research. Of particular relevance are the APLE statistics put forth by Li, Calder Catherine, and Cressie (Citation2007, Citation2012). Given that the performance and robustness of Moran’s I (local and global) is already established and the main contribution of this article is not methodological but, rather, substantive, we decide to adhere to them.
7. It is important to notice that, among the techniques reviewed previously, one of the most conservative in terms of detecting urban employment centers is that using spatial autocorrelation methods. This is empirically supported by Arribas-Bel and Sanz-Gracia (Citation2013), where it can be seen that the number of employment centers in 2000 is considerably smaller when applying LISA compared with Giuliano and Small’s (Citation1991) method. Taking this fact into consideration, we have decided to adopt a 10% significance level, less strict than the conventional 5%.
9. It might appear surprising that, for the analyzed period, there exist MSAs in which the number of centers decreases. Nevertheless, this is not an entirely new result: Leslie (Citation2010) finds a similar phenomenon for Phoenix, Arizona, between 1995 and 2004.
10. For as well as –, while the values off the diagonal provide useful information, they are based on very small numbers of metropolitan areas—and should thus be interpreted with caution.
11. In 2000, the smallest MSA has 52,457 inhabitants. The average size is 640,335 people and the median size 222,581.
12. Batty (Citation2005) asserts that cities are complex systems, but not complicated, in line with the classic applications of this concept in disciplines such as biology, chemistry, or physics.
13. According to the classical theory of distribution and under conditions of perfect competition, each worker receives a salary equal to the monetary value of her marginal productivity.
References
- Alonso, William (1964). Location and land use: Towards a general theory of rent. Cambridge, MA: Harvard University Press.
- Anselin, Luc (1995). Local indicators of spatial association—LISA. Geographical Analysis, 27(2), 93–115.
- Anselin, Luc (1996). The Moran scatterplot as an ESDA tool to assess local instability in spatial association. In M. Fischer, H. Scholten, & D. Unwin (Eds.), Spatial analytical perspectives on GIS (pp. 111–125). London: Taylor and Francis.
- Arauzo-Carod, Josep-Maria, & Viladecans-Marsal, Elisabeth (2009). Industrial location at the intra-metropolitan level: The role of agglomeration economies. Regional Studies, 43, 545–558.
- Arribas-Bel, Daniel, & Sanz-Gracia, Fernando (2013). On the existence of agglomeration shadows at the metropolitan level. Mimeo.
- Batty, Michael (2005). Cities and complexity. The MIT Press.
- Baumont, Catherine, Ertur, Ce, & Le Gallo, Julie (2004). Spatial analysis of employment and population density: The case of the agglomeration of Dijon 1999. Geographical Analysis, 36(2), 146–176.
- Berry, Brian J. L., & Kim, Hak-Min (1993). Challenges to the monocentric model. Geographical Analysis, 25, 1–4.
- Ciccone, Antonio (2002). Agglomeration effects in Europe. European Economic Review, 46, 213–227.
- Ciccone, Antonio, & Hall, Robert E. (1996). Productivity and the density of economic activity. American Economic Review, 87, 54–70.
- Craig, Steven G., & Ng, Pin (2001). Using quantile smoothing splines to identify employment subcenters in a multicentric urban area. Journal of Urban Economics, 49(1), 100–120.
- Duranton, Gilles, & Puga, Diego (2004). Micro-foundations of urban agglomeration economies. In J. V. Henderson & J. F. Thisse (Eds.), Handbook of urban and regional economics (Vol. 4). Amsterdam: North-Holland.
- Forstall, Richard L., & Greene, Richard P. (1997). Defining job concentrations: The Los Angeles case. Urban Geography, 18(8), 705–739.
- Fujii, Tadashi, & Hartshorn, Truman A. (1995). The changing metropolitan structure of Atlanta, Georgia: Locations of functions and regional structure in a multinucleated urban area. Urban Geography, 16, 680–707.
- Fujita, Masahisa (1989). Urban economic theory: Land use and city size. Cambridge: Cambridge University Press.
- Fujita, Masahisa, & Krugman, Paul (1995). When is the economy monocentric? Von Thünen and Chamberlin unified. Regional Science and Urban Economics, 25(4), 505–528.
- Fujita, Masahisa, & Ogawa, Hideaki (1982). Multiple equilibria and structural transition of non-monocentric urban configurations. Regional Science and Urban Economics, 12(2), 161–196.
- Fujita, Masahisa, & Thisse, Jacques F. (2002). Economics of agglomeration: Cities, industrial location, and regional growth. Cambridge University Press.
- García-López, Miquel-Angel, & Muñiz, Ivan (2010). Employment decentralisation: Polycentricity or scatteration? The case of Barcelona. Urban Studies, 47(14), 3035–3056.
- García-López, Miquel-Angel, & Muñiz, Ivan (2013). Urban spatial structure, agglomeration economies, and economic growth in Barcelona: An intra-metropolitan perspective. Papers in Regional Science, 92(3), 515–534.
- Garreau, Joel (1991). Edge city: Life on the new frontier. New York, NY: Doubleday.
- Giuliano, Genevieve, & Small, Kenneth (1991). Subcenters in the Los Angeles region. Regional Science and Urban Economics, 21, 163–182.
- Gordon, Peter, & Richardson, Harry W. (1996). Beyond polycentricity: The dispersed metropolis, Los Angeles, 1970–1990. Journal of the American Planning Association, 62, 289–295.
- Griffith, Daniel (1981). Modelling urban population density in a multi-centered city. Journal of Urban Economics, 9(3), 298–310.
- Griffith, Daniel, & Wong, David (2007). Modeling population density across major US cities: A polycentric spatial regression approach. Journal of Geographical Systems, 9(1), 53–75.
- Guillain, Rachel, Le Gallo, Julie, & Boiteux-Orain, C. (2006). Changes in spatial and sectoral patterns of employment in Ile-De-France, 1978–97. Urban Studies, 43, 2075–2098.
- Helbich, Marco (2012). Beyond postsuburbia? Multifunctional service agglomeration in Vienna’s urban fringe. Journal of Economic and Social Geography, 103(1), 39–52.
- Helbich, Marco, & Leitner, Michael (2010). Postsuburban spatial evolution of Vienna’s urban fringe: Evidence from point process modeling. Urban Geography, 31(8), 1100–1117.
- Henderson, J. Vernon (1974). The sizes and types of cities. American Economic Review, 64, 640–656.
- Lang, Robert E. (2003). Edgeless cities: Exploring the elusive metropolis. Washington, DC: The Brookings Institution Press.
- Lang, Robert E., Sanchez, Thomas W., & Oner, Asli C. (2009). Beyond edge city: Office geography in the new metropolis. Urban Geography, 30(7), 726–755.
- Lee, Bumsoo (2007). “Edge” or “edgeless” cities? Urban spatial structure in U.S. metropolitan areas, 1980 to 2000. Journal of Regional Science, 47(3), 479–515.
- Leslie, Timothy F. (2010). Identification and differentiation of urban centers in phoenix through a multi-criteria Kernel-density approach. International Regional Science Review, 33(2), 205–235.
- Leslie, Timothy F., & Huallacháin, Brandon Ó. (2006). Polycentric phoenix. Economic Geography, 82(2), 167–192.
- Li, Hongfei, Calder Catherine, A., & Cressie, Noel (2007). Beyond Moran’s I: Testing for spatial dependence based on the spatial autoregressive model. Geographical Analysis, 39(4), 357–375.
- Li, Hongfei, Calder Catherine, A., & Cressie, Noel (2012). One-step estimation of spatial dependence parameters: Properties and extensions of the APLE statistic. Journal of Multivariate Analysis, 105(1), 68–84.
- Marshall, Alfred (1890). Principles of economics. London: MacMillan.
- McMillen, Daniel (2001). Nonparametric employment subcenter identification. Journal of Urban Economics, 50(3), 448–473.
- McMillen, Daniel (2004). Employment densities, spatial autocorrelation, and subcenters in large metropolitan areas. Journal of Regional Science, 44(2), 225–244.
- McMillen, Daniel, & Smith, Stephani C. (2003). The number of subcenters in large urban areas. Journal of Urban Economics, 53(3), 321–338.
- Meijers, Evert J., & Burger, Martijn J. (2010). Spatial structure and productivity in US metropolitan areas. Environment and Planning A, 42, 1383–1402.
- Mills, Edwin (1972). Studies in the structure of the urban economy. Baltimore, MD: The John Hopkins Press.
- Minnesota Population Center. (2004). National historical geographic information system. Pre-release version 0.1.50. Minneapolis: University of Minnesota. Retrieved from http://www.nhgis.org
- Moran, Alfred P. (1948). The interpretation of statistical maps. Journal of the Royal Statistical Society, Series B (Methodological), 10(2), 243–251.
- Muñiz, Ivan, & Garcia-López, Miquel-Angel (2010). The polycentric knowledge economy in Barcelona. Urban Geography, 31(6), 774–799.
- Muth, Richard F. (1969). Cities and housing: The spatial pattern of urban residential land use. Chicago, IL: University of Chicago Press.
- Openshaw, Stan, & Taylor, P. (1981). The modifiable areal unit problem. In N. Wrigley & R. J. Bennett (Eds.), Quantitative geography (pp. 60–70). Henley-on-Thames: Routledge and Kegan Paul.
- Paez, Antonio, Uchida, Takashi, & Miyamoto, Kazuaki (2001). Spatial association and heterogeneity issues in land price models. Urban Studies, 38(9), 1493–1508.
- Rey, Sergio, & Anselin, Luc (2007). Pysal, a Python library of spatial analytical methods. The Review of Regional Studies, 37(1), 5–27.
- Rey, Sergio J., & Sastre-Gutiérrez, Myrna L. (2010). Interregional inequality dynamics in Mexico. Technical report, GeoDa Center for Geospatial Analysis and Computation, Arizona State University.
- Riguelle, Francois, Thomas, Isabelle, & Verhetsel, Ann (2007). Measuring urban polycentrism: A European case study and its implications. Journal of Economic Geography, 7(2), 193–215.
- Rodríguez-Gámez, Liz, & Dallerba, Sandy (2012). Spatial distribution of employment in Hermosillo, 1999–2004. Urban Studies, 49(16), 3663–3678.
- Sarzynski, Andrea, Galster, George, & Stack, Lisa (2013). Evolving United States metropolitan land use patterns. Urban Geography, 35(1), 25–47.
- Shearmur, Richard, Coffey, William, Dube, Christian, & Barbonne, Remy (2007). Intrametropolitan employment structure: Polycentricity, scatteration, dispersal and chaos in Toronto, Montreal and Vancouver, 1996–2001. Urban Studies, 44(9), 1713–1738.
- Shearmur, Richard, & Hutton, Thomas (2011). Canada’s changing city-regions: The expanding metropolis. In L. S. Bourne, T. Hutton, R. Shearmur, & J. Simmons (Eds.), Canadian urban regions: Trajectories of growth and change (pp. 99–124). Toronto: Oxford University Press.
- US Bureau of the Census. (2003). Census geographic glossary. Retrieved from http://www.census.gov/geo/lv4help/cengeoglos.html
- Von Thunen, Johann H. (1826). Der isolierte Staat in Beziehung auf landtschaft under nationalokonomie (C. M. Wartenberg, Von Thunen’s Isolated State, Oxford, English Trans., 1966). Hamburg: Pergamon Press.
- Wheaton, William C. (1979). Monocentric models of urban land-use: Contributions and criticism. In P. Mieszkowski & M. Straszheim (Eds.), Current issues in urban economics. Baltimore, MD: John Hopkins Press.
- White, Michelle J. (1999). Urban areas with decentralized employment: Theory and empirical work. In E. S. Mills & P. Cheshire (Eds.), Handbook of regional and urban economics (Vol. 3). Amsterdam: North Holland.