ABSTRACT
EU chemicals legislation requires the use of all available information for hazard and risk assessment before new tests on vertebrates are proposed or conducted. In this context, extrapolation approaches for avoiding chronic fish testing on the basis of existing data have been explored. Simple linear relationships and interspecies sensitivity ratios between Daphnia and fish were calculated and acute-to-chronic relationships and ratios were calculated for fish, taking into consideration the mode of action. The best fitted relationships for the prediction of chronic fish toxicity are obtained based on acute fish and Daphnia data. Chemicals acting by unspecific reactivity and non-polar narcosis give the strongest acute and chronic Daphnia-to-chronic fish relationship. With acute fish data, strong relationships are obtained for all mode of action. Daphnia was found to be more sensitive than fish to several aniline derivatives and pesticides acting through cholinesterase inhibition, and less sensitive than fish to known endocrine disruptors. Extreme (i.e. <1 or >100) interspecies sensitivity ratios were mainly evident for chemicals acting by polar narcosis and specific reactivity. The safety factor of 100 commonly applied in environmental risk assessment does not seem to be equally protective for each mode of action.
1. Introduction
Several pieces of EU chemicals legislation (REACH, Biocidal Products Regulation, Plant Protection Products Regulation) may require long-term toxicity data on fish (EC Citation2006, Citation2013; EU Citation2012), i.e. when the outcome of the acute testing indicates a risk, or if long-term exposure is expected. However, the generation of such information comes at great cost to industry and implies the use of numerous fish (EC Citation2013; Scholz et al. Citation2013). On the other hand, European horizontal policy also requires to limit animal testing (EU Citation2010) and, for example, in the context of REACH, animal tests should be undertaken only as a last resort (EC Citation2006).
Thus, it is encouraged to use alternative methods as well as all available information for hazard and risk assessment (RA) before proposing or conducting new tests on animals. Nevertheless, so far, there are very few models to predict chronic fish toxicity, most of them being based on quantitative structure activity relationship (QSAR), such as ECOSAR™ (Mayo-Bean et al. Citation2012) or QSARCHE (Austin and Eadsforth Citation2014; Claeys et al. Citation2013).
Moreover, regulatory aquatic RA schemes require toxicity testing of chemicals on a limited number of laboratory species, which makes interspecies extrapolation a fundamental tenet of regulatory RA. In this effort to limit testing and to use already existing data, interspecies extrapolations have been used in environmental RA by applying safety factors (SFs), and they may play an increasing role in the future (Celander et al. Citation2011). One example is the use of SF applied to toxicological data to derive the predicted no-effect concentration (PNEC) for aquatic toxicity. These factors are intended to account for interspecies differences in sensitivity, extrapolation from acute to chronic effects, the physicochemical complexity of natural water versus laboratory test media, and the complexity of the ecosystem versus single-species laboratory tests. The choice of the factor (10, 50, 100 or 1000) depends on the quality and quantity of the available data (ECHA Citation2008). Species extrapolation is based on the hypothesis that some biological pathways are conserved among species, as opposed to direct comparisons of apical responses alone via species sensitivity distribution (Perkins et al. Citation2013).
Differences in species sensitivity regarding acute aquatic toxicity are well described (Hoekzema et al. Citation2006; Hutchinson et al. Citation2003; Jeram et al. Citation2005; Tebby et al. Citation2011; Weyers et al. Citation2000). To address the question of whether it is possible to predict acute toxicity in fish from non-vertebrate species, Netzeva, Pavan, and Worth (Citation2008) reviewed several quantitative activity--activity relationships between species, the most relevant relationship for acute fish toxicity being between Daphnia and rainbow trout (n = 360), with an r2 value of 0.67. More recent studies have confirmed the good correlation between acute fish and Daphnia toxicity data, especially for organothiophosphates (0.74 < r2 < 0.94, n = 9) and (benzo)triazoles (r2 = 0.87, n = 40) (Cassani et al. Citation2013; Kar and Roy Citation2010; Zhang et al. Citation2010; Zvinavashe et al. Citation2009). The correlation depends on both the bio-uptake process and the mode of action (MOA) of the chemical (Zhang et al. Citation2010), as well as its physicochemical properties (Tebby et al. Citation2011). The US EPA has also developed a specific tool, the Interspecies Correlation Estimation (ICE) tool (implemented as Web-ICE; Raimondo, Vivian, and Barron Citation2010) to predict acute toxicity to 12 different fish species, including bluegill, fathead minnow, sheepshead minnow, rainbow trout, and common carp, on the basis of Daphnia magna toxicity. The strongest correlation found by this model is between D. magna and bluegill (r2 = 0.62, n = 290) (US-EPA Citation2013). However, there is little work on the potential to predict chronic fish toxicity from Daphnia data.
There is a general agreement that predictive toxicology requires classification of chemical by chemical class or MOA, and reliable models for filling data gaps on properties and effects of environmental contaminants are mostly mechanism based (Nendza and Wenzel Citation2006; Netzeva,Pavan, and Worth Citation2008). Besides basal toxicity which acts through non-specific mechanism common for all chemicals, such as the partitioning into non-aqueous phases (e.g. membranes), environmental contaminants may also be specifically toxic in different ways due to interactions at the biomolecular level. The physico-chemical properties and structures of the chemicals are crucial parameters in determining the transport to and the interactions with biomolecular targets (e.g. receptors, enzymes), and environmental contaminants with a common MOA are generally expected to have similar structures and/or properties.
The Verhaar classification of environmental pollutants classifies organics pollutants into four classes depending on the modes of action, according to a series of structural rules (Enoch et al. Citation2008; Verhaar, van Leeuwen, and Hermens Citation1992). The first class (class 1, MOA 1: narcosis or baseline toxicity) regroups chemicals that are not reactive when considering overall acute effect and that do not interact with specific receptors. Those chemicals act via the so-called baseline toxicity, or non-polar narcosis, with toxicity being dependent upon hydrophobicity. The second class (class 2, MOA 2: less inert compounds) regroups less inert chemicals that are slightly more toxic than predicted by baseline toxicity estimations, acting by the so-called polar-narcosis mechanism. The third class (class 3, MOA 3: unspecific reactivity) regroups reactive chemicals that display a significantly higher toxicity than predicted by hydrophobicity alone, by forming irreversible covalent bonds with a variety of structures commonly found in macromolecules. The fourth class (class 4, MOA 4: specifically acting chemicals) regroups specifically acting chemicals that act by binding at specific receptors in a non-covalent manner, i.e. neurotoxicants or chemicals acting via acetylcholinesterase inhibition. A fifth class has been ulteriorly added, grouping all the chemicals that could not be classified in classes 1–4. This classification scheme has been implemented in the Toxtree Software, a toxic hazard estimation by decision tree approach (Patlewicz et al. Citation2008; Sourceforge Citation2004) and later in the OECD QSAR Toolbox (OECD Citation2012). It is used for chemical categorisation purposes, for the prioritisation of chemicals for subsequent testing, and for helping in risk and hazard assessment by separating small-to-intermediate organic chemicals into distinct classes that can either be assigned a mode of action, or a quantitative relationship between the structure of the classified chemicals and their acute aquatic toxicity.
Regarding acute-to-chronic extrapolation, a methodology based on simple linear regression was developed to predict the PNEC of chemicals to fishes from acute toxicity data (Mayer et al. Citation1992). PNEC was found to be highly accurate in 92% of the time (within a factor of 2 of the limits of the maximum acceptable toxicant concentrations for lethality) when applied to a data base of 18 chemicals. Ahlers et al. (Citation2006) determined a median acute-to-chronic ratio (ACR, the ratio between the acute toxicity data and chronic toxicity data for a given chemical) of 10.5 for fish, well below the ACR safety factor of 100 as implied by the REACH guidance (ECHA Citation2008). However, they found that individual ACRs vary considerably and go up to 4400. Thus, an SF of 100 is not protective for all chemicals and trophic levels. The use of fixed ratios for the extrapolation from acute-to-chronic toxicity is problematic, because some chemicals show different MOA under short- and long-term conditions, or from one species to another.
In this context, the usefulness of fish acute-to-chronic toxicity relationships and interspecies extrapolations for predicting chronic fish toxicity have been explored, taking into consideration the Verhaar MOA classification scheme. Moreover, when several toxicological data (e.g. LC50, NOEC [No-observed effect concentration]) were available for a given chemical and endpoint, those relationships have been calculated using either the lowest value (LV; i.e. expressing the highest toxicity) or the geometric mean (GM) based on all the available toxicological data, in order to investigate the influence of those two approaches on the outcome. As a matter of fact, the use of the lowest toxicological value is usually recommended in environmental RA in the interest of being as protective as possible. This is especially important for the setting of PNEC, for example, when it is derived from a single toxicological value in a given species but aims to protect all the other species. However, the use of the GM, calculated across the full range of empirical data available rather than just the most sensitive value, might allow more stable and representative estimates of chronic fish toxicity by giving less weight to the extreme value.
2. Methodology
2.1. Extraction of data
2.1.1. Databases
Organic chemicals with chronic fish toxicity data were selected, and data sets including acute fish toxicity data as well as acute and chronic Daphnia toxicity data were extracted using the OECD QSAR Toolbox 3.2 (OECD Citation2012) for all the chemicals selected. All databases available within the OECD QSAR Toolbox were selected, i.e. the Aquatic ECETOC, the Aquatic OASIS, the Aquatic Japan MoE, the ECHA database and the US EPA database. The list of chemicals extracted and used for the calculations is given in Table S1 (Online Supplementary Material).
2.1.2. Species
D. magna was the only species retained for Daphnia spp., while for fish, preference was given to OECD freshwater fish species (zebrafish [Danio rerio], fathead minnow [Pimephales promelas], common carp [Cyprinus carpio], Japanese medaka [Oryzias latipes], guppy [Poecilia reticulata], bluegill [Lepomis macrochirus], rainbow trout [Onchorhynchus mykiss], three-spined stickleback [Gasterosteus aculeatus]) as well as the saltwater species sheepshead minnow (Cyprinodon variegatus). Data from other species of freshwater fish or from saltwater or estuarine species were considered only if there were no data available on the preferred species.
2.1.3. Endpoint and duration of exposure
The LC50 was chosen as the endpoint for acute toxicity tests. The duration of acute toxicity tests is typically 48 h for Daphnia and 96 h for fish. The acute effects taken into account in this study were immobilisation and mortality for Daphnia (EC50 and LC50), and mortality for fish (LC50). The NOEC was chosen as the endpoint for long-term toxicity tests, as this is the most frequently reported data for long-term toxicity tests. The minimum duration of exposure considered was 21 days for Daphnia; and 21, 28 days or more for fish. The chronic effects taken into account were mortality, reproduction, growth and development.
2.1.4. Quality check
Data were checked for duplicates (i.e. if the same data were extracted from several databases), and it was checked that the toxicological value was below the solubility limit of the substances. From the ECHA database, only data ranked Klimisch score 1 or 2 were considered (Klimisch, Andreae, and Tillman Citation1997), i.e. data considered as reliable without restriction (score 1) or reliable with restriction (score 2: meaning that the study might not totally comply with the specific test guideline but the test parameters are sufficiently documented to be scientifically acceptable).
2.2. Treatment of data prior to analysis
2.2.1. Lowest value and geometric mean approaches
The unit of the data extracted was checked, harmonised (mg/L) and transformed in mol/L. If there was more than one value per endpoint of interest for a given chemical, either the lowest toxicological value (LV) was identified or the GM was calculated for the given endpoint and used for the analysis. Both approaches (LV and GM) were applied when analysing the whole set of chemicals (i.e. irrespective of the MOA), whereas only the GM approach was applied to the chemicals subdivided according to their MOA.
2.2.2. Assignment of modes of action
The selected chemicals were profiled with the QSAR Toolbox and classified into the five MOA classes according to the Verhaar classification scheme. To complete the classification, they were further profiled with Toxtree and the post-processing filter encoded as a KNIME workflow (Ellison et al. Citation2015) that has recently been developed in order to improve the predictivity of the Toxtree software. In case of conflicting results or MOA 5 chemicals, other data from the literature has been used to attribute a final MOA (Barron, Lilavois, and Martin Citation2015; ECETOC Citation2007; Russom et al. Citation1997).
2.3. Analysis
A simple linear regression on log10-transformed data was used (GraphPad Prism 5) to obtain the interspecies relationships and the corresponding r2 values (measures of goodness-of-fit) for the whole set of chemicals or subsets of chemicals according to their MOA. The robust regression and outlier removal method was used to identify potential outliers (Motulsky and Brown Citation2006); the r2 given are the r2 after elimination of the outlier(s). For the interpretation of regression data, it was assumed that a regression coefficient r2 of >0.6 corresponds to a moderate correlation whereas a value of about 0.8 and more corresponds to a reliable prediction.
The interspecies sensitivity ratio (SR) for each chemical (SRacute = LC50Daphnia/NOECFish; SRch = NOECDaphnia/NOECFish) was also calculated to compare the respective sensitivity of fish and Daphnia, as well as the ACR for fish (ACRfish = LC50Fish/NOECFish), using the GM value. The statistical result was characterised by the median and 90 percentile value as well as by their minimum and maximum values. The distribution of the SR (<10; between 10 and 100; between 100 and 1000; >1000) were also analysed, taking the MOA into account.
3. Results and discussion
3.1. Interspecies extrapolation (quantitative activity–activity relationships)
3.1.1. Daphnid-to-chronic fish relationships – irrespective of the MOA
There were 246 substances with matched Daphnia acute data (LC50 48 h) and chronic fish data (NOEC >21 days) and 193 substances with matched Daphnia chronic data (NOEC – 21 days) and chronic fish data. The relationship (i.e. simple linear regression on log10-transformed data) was significant with both approaches (LV or GM; p < 0.0001). With both acute Daphnia and chronic Daphnia data, the goodness-of-fit was improved by using the GM instead of the LV (r2 of logged data = 0.64 and 0.60, respectively, for acute Daphnia data; 0.59 and 0.57, respectively, for the chronic Daphnia data) ().
Table 1. Linear relationships between Daphnia acute or chronic toxicity data and fish chronic toxicity data.
17β-Estradiol is the only outlier identified in the acute Daphnia-to-chronic fish relationship, with both approaches ( and (a)); and is also identified as an outlier in the chronic Daphnia data-to-chronic fish relationship (with both approaches), along with fenitrothion (GM approach only; and (b)).
Figure 1. Relationships between Daphnia 48 h LC50 (a) and Daphnia NOEC (b) and fish NOEC values with the geometric mean approach. The dashed lines represent the 95% confidence interval. Italics: name of the outliers.
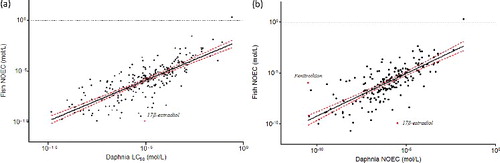
17β-Estradiol is a steroid and an estrogen sex hormone found in most vertebrates as well as many crustaceans, insects, fish, and other animal species. It is a MOA 2 chemical (i.e. polar narcotic) according to the Verhaar classification. It is a well-studied chemical characterised by a high quantity of data in the various databases (118 entries after extraction, 42 entries in our final database). The NOEC fish values reported display a high variability and range between 0.00001 and 0.001 mg/L. This can be due, amongst other factors, to the fact that the guidelines used for chronic fish tests cover different life stages. The impact of this variability is decreased by the use of the GM approach; nevertheless, 17β-estradiol remains an outlier due to its high toxicity to fish. Although being classified as MOA 2, 17β-estradiol acts primarily as an agonist of the estrogen receptor (ER) (Pinto, Estêvão, and Power Citation2014). The result of ER activation is a modulation of gene transcription and expression in ER-expressing cells which is the predominant mechanism by which 17β-estradiol mediates its biological effects. However, it also acts as an agonist of membrane ERs via which it can mediate a variety of rapid, non-genomic effects (Pinto, Estêvão, and Power Citation2014). Therefore, 17β-estradiol acts by a rather specific and non-narcotic MOA, at least when it comes to chronic toxicity; however, this is not captured in the Verhaar scheme, as this classification scheme is based on acute toxicity.
Fenitrothion is a phosphorothioate insecticide acting through acetyl cholinesterase inhibition. It appears as one of the most chronically toxic chemicals to Daphnia in our database, whilst having a medium toxicity to fish. However, there was only one Daphnia NOEC value available.
3.1.2. Daphnid-to-chronic fish relationships by MOA
To check whether or not the goodness-of-fit depends on the MOA, the linear regression was performed for each MOA class, using the GM approach. The results are shown in and (acute toxicity) and (chronic toxicity).
Figure 2. Relationships between Daphnia LC50 and fish NOEC values by MOA. Italics: name of the outliers; (a) all MOA; (b) MOA 1, r2 = 79; (c) MOA 3, r2 = 0.83.
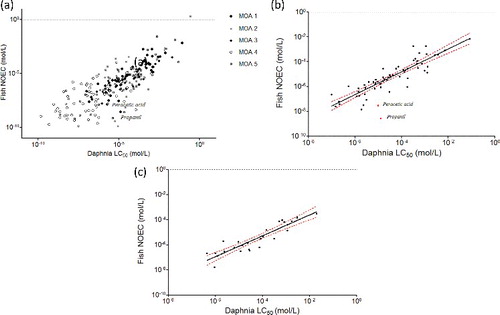
Figure 3. Relationships between Daphnia NOEC and fish NOEC values by MOA. Italics: name of the outliers; (a) all MOA; (b) MOA 1, r2 = 0.66; (c) MOA 3, r2 = 0.65.
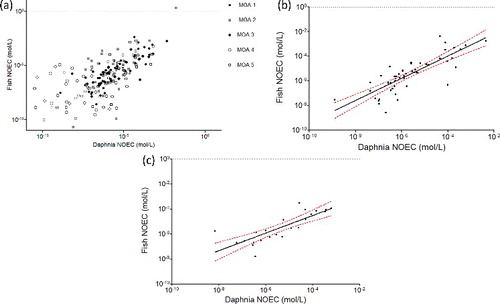
The relationships are highly significant (p ≤ 0.0001) for MOAs 1, 2, 3, and 5 chemicals. For MOA 4 chemicals, the relationships are not significant (p = 0.0004 and 0.4982, respectively, for the relationship based on acute Daphnia data and chronic Daphnia data). This means that chronic Daphnia toxicity would be a very poor predictor of chronic fish toxicity for MOA 4 chemicals. This makes sense as this is the group of chemicals with specific MOA which are not necessarily present in all taxa. It would therefore be interesting to divide further this MOA 4 group and to investigate the relationship of each specific mechanism (e.g. AchE inhibition, neurotoxicant, respiratory blocker, etc.).
In both cases, the r2 of logged data is higher for MOAs 1 and 3 (respectively, 0.79/0.83 for MOA 1/MOA 3 based on acute Daphnia data, (b,c), and 0.66/0.65 for MOA 1/MOA 3 based on chronic Daphnia data, (b,c)), and much lower for MOAs 2 and 4 chemicals (respectively, 0.36/0.22 for MOA 2/MOA 4 based on acute Daphnia data and 0.35/0.01 for MOA 2/MOA 4 based on chronic Daphnia data). The more reliable relationship is therefore obtained with acute Daphnia data, and not with chronic Daphnia data.
The r2 for MOA 5 chemicals is unexpectedly high for a class which is a mix of chemicals that could not be assigned to classes 1–4 (0.66 and 0.67, respectively, based on acute and chronic data). This could suggest that many MOA 1 or 3 chemicals might be present in the MOA 5 group knowing that the MOA 5 class is a mix of MOAs 1, 2, 3 and 4 chemicals that have not been identified as such.
Propanil and peracetic acid are identified as outliers in the relationship based on acute Daphnia data for MOA 1 chemicals. Propanil is a widely used contact herbicide which mainly acts against weeds by inhibiting their photosynthesis and CO2 fixation, and which shows a very high chronic toxicity toward fish compared to the other MOA 1 chemicals, which suggest a more specific mode of action than non-polar narcosis. Peracetic acid is used as an antimicrobial. Only one NOEC value for fish was available for this chemical, extracted from the ECHA database (0.0022 mg/L) representing a nominal value in the middle of the range of concentrations tested (0.2, 0.7, 2.2, 7.5, 22.4 µg peracetic acid/L).
3.1.3. Interspecies sensitivity ratio
The SRs of the chemicals have been calculated and their distribution is shown in (SRac = LC50Daphnia/NOECFish; SRch = NOECDaphnia/NOECFish). A ratio of >1 indicates that Daphnia are less sensitive compared to fish and a ratio of <1 indicates that Daphnia are more sensitive compared to fish. Quite expectedly, acute Daphnia LC50 values are generally higher than chronic fish NOEC values (81.6% of the chemicals), and usually within a factor 10 for 39% of the latter chemicals.
Figure 4. Sensitivity ratio derived with acute Daphnia data versus chronic fish data (black bar) and chronic Daphnia data versus chronic fish data (grey bar) in percentage of chemicals of the whole dataset; i.e. 34.7% of the chemicals demonstrate a higher chronic toxicity in Daphnia compared to NOEC fish and values range within a factor of 10; for 39% of the chemicals, the acute Daphnia LC50 indicates a lower sensitivity compared to chronic NOEC fish and values range within a factor of 10.
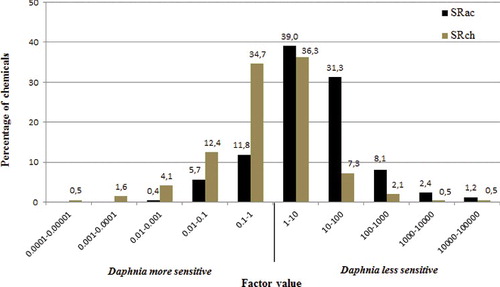
When using Daphnia NOEC values, the distribution is shifted to the left by a factor of 10, which suggests a medium factor of 10 between acute and chronic Daphnia toxicity data on the overall distribution. For 69.9% of the chemicals, the SRch is within a factor of 10; amongst which Daphnia is less sensitive for 35.7% of the chemicals, and more sensitive for 34.5% of the chemicals. As a whole, Daphnia is more sensitive for 53% of the chemicals, whereas fish is more sensitive for 47% of the chemicals. Therefore, none of these two trophic levels (i.e. fish and daphnids) seems to be more sensitive than the other in chronic testing, which is in line with some previous work (May and Hahn Citation2014).
In 90.7% of the cases, the chronic SRch is within a factor 100. For the remaining 9.3% corresponding to 18 chemicals, presented in , an extreme SRch (either <0.01 or >100) was calculated. These 18 chemicals are pharmaceuticals, pesticides, aromatic amines and hormones. They are mostly specifically acting chemicals (seven MOA 4) and polar narcotics (seven MOA 2), along with ibuprofen, classified as MOA 1, dimethyl disulphide, classified as MOA 3 and norethindrone and carbamazepine, classified as MOA 5 ().
Table 2. Substances with a high interspecies sensitivity ratio (SRch).
One of the chemicals for which Daphnia is less sensitive than fish is ibuprofen, a nonsteroidal anti-inflammatory pharmaceutical. Its pharmacological effects are believed to be due to inhibition of cyclooxygenase-2 (COX-2) which decreases the synthesis of prostaglandins involved in mediating inflammation, pain, fever, and swelling (Wishart et al. Citation2006). Norethindrone is a progestin hormone, whereas ethinyl estradiol and 17β-estradiol are estrogen hormones.
Amongst other chemicals for which Daphnia is far less sensitive than fish, there are the two pesticides chlorothalonil and imidacloprid. Clorothalonil is a broad-spectrum non-systemic fungicide also used as a wood protectant, pesticide, acaricide, and to control mold, mildew, bacteria and algae. It acts via the formation of substituted chlorothalonil-reduced glutathione derivatives in cells, which trigger inhibition of specific NAD thiol-dependent glycolytic and respiratory enzymes (Tillman, Siegel, and Long Citation1973). This action on basic glycolytic and respiratory pathways is conserved across biological taxa and toxicity is evident in multiple organisms.
Imidacloprid is a systemic neonicotinoid insecticide which acts as an insect neurotoxin, by blocking nicotinic acetylcholine receptors. Quite unexpectedly, it appears to be much more toxic to fish than to Daphnia. However, there was only one entry for chronic fish toxicity in the database, from the general literature. When going back to the original study, it appears that the NOEC corresponds to the concentration measured in the field when assessing the chronic endpoint 27 days after contamination of the field (1.1 μg/L) (Sanchez-Bayo and Goka Citation2005). As there was no effect, this quite low concentration appears as a NOEC, although other sources mention a much higher fish NOEC, i.e. the EFSA conclusion on imidacloprid retains a chronic NOEC value of 9.02 mg/L (EFSA Citation2014). This means that this high chronic SR is probably methodologically biased.
Regarding chemicals for which Daphnia is much more sensitive than fish, we found mainly pesticides acting through acetylcholinesterase inhibition and aromatic amines. Diazinon, disulfoton and methylparathion are organophosphate insecticides, whilst carbaryl is of the carbamate family, and fenitrothion is a phosphorothioate. All of them act through acetylcholinesterase inhibition. Carbamazepine is a pharmaceutical, an anticonvulsant, which works by decreasing nerve impulses that cause seizures and pain. Finally, aniline and its derivatives, p-methylaniline, 3-chloroaniline, 2-methylaniline and 3-amino-phenol show chronic sensitivity factors ranging between 0.001 and 0.01, which means that Daphnia is at least 100-fold more sensitive than fish for this group of chemicals.
It has to be highlighted that the Verhaar classification scheme is built on acute fish toxicity, and that chemicals are attributed to the four MOA classes based on structural characteristics. However, acute toxicity mechanisms are different from chronic toxicity mechanisms. This can explain why chemicals acting via endocrine disruption mechanisms are not identified as ‘specifically acting chemicals’ (MOA 4) but as, for example, MOA 2 in the case of ethinyl estradiol and 17β-estradiol. In the same way, aniline and its derivatives are classified as polar narcotics which are characterised by a slightly higher toxicity to fish than the baseline toxicity of MOA 1 chemicals, although its toxicity to Daphnia is much higher.
3.1.4. Sensitivity ratios and MOA
The distribution of the SRs according to their MOA () shows that 87% of the MOA 3 chemicals have a chronic SR for Daphnia/fish within a factor of 10, and 95.6% are within a factor of 100. Moreover, for the majority of the MOA 3 chemicals, Daphnia seems less sensitive than fish (60.9% of the MOA 3 chemicals have an SR between 1 and 10). Regarding MOA 1 chemicals, 76.9% of the chemicals have an SR within a factor 10, Daphnia being more sensitive than fish for 51.9% of them; and 98.1% of the MOA 1 chemicals have a chronic SR within a factor of 100.
Figure 5. Distribution of the chronic sensitivity ratios between Daphnia NOEC and fish NOEC according to their MOA.

For MOA 4, the SRs cover a broader range, i.e. only 64.7% of the MOA 4 chemicals have a chronic SR within a factor of 10, Daphnia being more sensitive for only 29.4% of the chemicals. 79.4% of these chemicals have a sensitive ratio within a factor of 100, which means that 20% of the MOA 4 chemicals have an extreme SR. MOA 2 chemicals also follow this pattern with only 58.4% of chemicals with an SR within a factor of 10 and 80.6% within a factor 100.
3.2. Acute-to-chronic fish data
3.2.1. Acute-to-chronic fish relationships – irrespective of the MOA
There were 240 substances with acute fish toxicity data and chronic fish data. The relationship was significant with both approaches (p < 0.0001), but as previously, the goodness-of-fit was improved by using the GM instead of the LV (r2 of logged data = 0.82 and 0.87, respectively, for the LV approach and the GM approach; ). Data are plotted in
Table 3. Linear relationships between acute fish data and chronic fish data.
Figure 6. Relationships between fish 96 h LC50 and fish NOEC values with the geometric mean approach. The dashed lines represent the 95% confidence interval. Italics: name of the outliers.
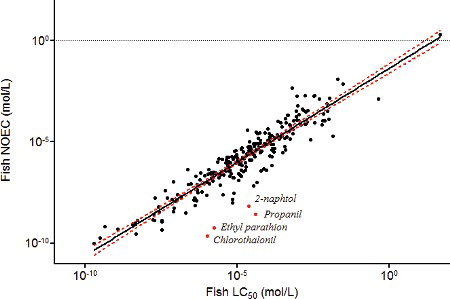
With either of the two approaches, propanil and chlorothalonil are identified as outliers because of higher chronic fish toxicity than expected when considering their acute fish toxicity, whereas the other outlier chemicals depend on the approach used (). This is in line with the previous results as propanil was already identified as an outlier in the acute Daphnia-to-chronic fish relationship due to its high chronic fish toxicity, and confirms the hypothesis of a specific chronic mode of action. According to Russom et al. (Citation1997), it is classified as an MOA 1 chemical acting as a non-polar narcotic in acute toxicity to fish. However, the OECD QSAR Toolbox identified this chemical as an MOA 5 chemical. Chlorothalonil, already mentioned for its extreme interspecies SR (Section 3.1.3), is classified as a specifically acting chemical (MOA 4).
With the GM approach, two other chemicals are identified as outliers because of a higher chronic toxicity to fish: 2-naphtol, a widely used intermediate for the production of dyes and other compounds (MOA 2), and ethyl parathion, an organothiophosphate pesticide (MOA 4).
3.2.2. Acute-to-chronic fish relationship by MOA
To check whether or not the goodness-of-fit depends on the MOA, the linear regression was performed for each MOA class. The results are presented in and plotted in . There were highly significant relationships (p < 0.0001) with high r2 (>0.80) between acute and chronic toxicity data for each MOA class. The highest r2 is obtained with MOA 3 and, more surprisingly, MOA 5 chemicals (r2 = 0.88; (b,c)).
Figure 7. Relationship between fish 96 h LC50 and fish NOEC values by MOA. The dashed lines represent the 95% confidence interval; (a) all MOA; (b) MOA 3, r2 = 0.88; (c) MOA 5, r2 = 0.88.
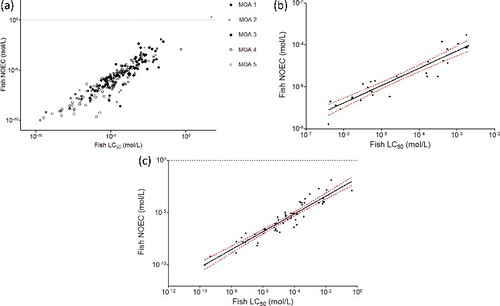
All the chemicals previously identified as outliers in the relationship based on the whole set of chemicals are also identified as outliers in their MOA groups: propanil (MOA 1), 2-napthol (MOA 2), ethylparathion, and chlorothalonil (MOA 4). In addition, in the MOA 1 chemicals group, three other chemicals are outliers: monoethanolamine (CAS No.: 141-43-5), genistein (CAS No.: 446-72-0) and n-methylisopropylamine (CAS No.: 4747-21-1).
Monoethanolamine is a primary amine used as feedstock in the production of detergents, emulsifiers, polishes, pharmaceuticals, corrosion inhibitors, or chemical intermediates. Only one NOEC value on fish was available for this chemical, and was extracted from the ECHA database (1.24 mg/L, O. latipes), along with four LC50 values (170, 349, 2070 and 2120 mg/L, on four different species: C. carpio, C. auratus, P. promelas and P. reticulata). The ECHA database also reported two values for n-methylisopropylamine: one LC50 96 h on D. rerio with mortality initiating from 43.3 mg/L (acute fish toxicity test, according to the OECD TG203, carried out in 2008; calculated LC50 of 48.5 mg/L) and one NOEC value >323 mg/L after 28 days of exposure (juvenile growth test carried out in 2011 according to the OECD TG215) on the same species. There was no explanation for the difference between those two values; but this explains the fact that this chemical appears as an outlier in the ACR relationship.
Genistein is a phytoestrogen and belongs to the category of isoflavones. Due to its structure similarity to 17β-estradiol, it has been shown to interact with animal and human ERs (Patisaul et al. Citation2002; Green Citation2015), but also to bind to and transactivate all three peroxisome proliferator-activated receptors (PPAR) isoforms, α, δ, and γ (Wang et al. Citation2014) and to possibly inhibit the DNA methyltransferase (Fang, Chen, and Yang Citation2007). This could explain the higher chronic toxicity for fish than expected. Only one LC50 value is reported for genistein (1.9 mg/L), along with five NOEC values coming from the same study (0.0013–0.0042 mg/L). All studies have been carried out with D. rerio.
3.2.3. Acute-to-chronic ratio
The average ACR for fish over the 240 chemicals was 141.8, but the median is 8.9, which means that there are extremely high values which influence this average. This is in line with some previous work reporting a median ACR for fish of 10.5 (Ahlers et al. Citation2006). Quite unexpectedly, for seven chemicals, the ACR was below 1 (but within a factor of 10, the lowest ACR being 0.15), which means that the chronic toxicity (NOEC) value in the database was higher for those chemicals than the acute toxicity value. This might be due to the fact that different endpoints and different species have been considered in the database; and that the variability between species and/or endpoints might overcome the ACR. Amongst these seven chemicals, three are MOA 1, two MOA 5, one MOA 4, and one MOA 2 (). Usually, MOA 4 chemicals are characterised by higher ACR due to their specific MOA; however, ziram, a pesticide, has an ACR of 0.54, according to our database. For ziram, two fish LC50 (0.0097 and 0.57 mg/L) and two NOEC values (0.101 and 0.189 mg/L) are available in our database. They have been extracted from the ECHA database and are considered reliable data.
Table 4. Name and mode of action (MOA) of chemicals with Acute to Chronic Ratio (ACR) < 1.
It was not possible to go back to the original reference of the pyridine data (MOA 2 chemical, ACR = 0.91); however, the very low toxicity (one LC50 value of 99.6 mg/L and one NOEC value of 110 mg/L) probably means that this higher NOEC is due to the experimental design.
Regarding flucythrinate (MOA 5, ACR = 0.67), a pyrethroid pesticide, two LC50 values of 0.0002 mg/L and one NOEC value of 0.0003 mg/L on two different species were extracted from the Aquatic ECETOC database. It was not possible to go back to the original studies. For octamethyltrisiloxane (MOA 5, ACR = 0.77), an ectoparasiticides drug, all data were extracted from the ECHA database: one LC50 value of 0.0194 mg/L on O. mykiss along with two NOEC values in the same range (0.027 mg/L on O. mykiss for growth, survival and hatching, and 0.021 mg/L for survival on P. promelas). We have some doubts on the value reported as LC50 since this value is also given as NOEC value of the same study.
Regarding diisopropylamine (MOA 1, ACR = 0.51), there were six LC50 values on four different species (37, 40, 42, 75, 196 and 798 mg/L), which is a rather broad and unusual range (a factor of 21 between the higher and the lower LC50 reported) and only one NOEC value of 187 mg/L. The highest LC50 of 798 mg/L and the NOEC value were obtained on the same species, G. aculeatus, which does not seem to be very sensitive to this chemical. All data were extracted from the ECHA database in which they were ranked as reliable with a Klimisch score of 1 or 2.
For 1,2-bromoethane (MOA 1, ACR = 0.54), one fish LC50 value (32.1 mg/L) and two NOEC values (5.81 and 9.62 mg/L for growth and mortality obtained with O. latipes) were extracted from the Aquatic ECETOC database. In addition to these data, the ECHA database contains two more LC50 values derived with O. mykiss (1.13 and 1.86 mg/L) and labelled with a Klimisch score of 1. This corresponds to a factor 28 between the lowest and the highest LC50 values.
The range of ACR is quite broad (up to 14,906) but 53.3% of the chemicals have an ACR below 10 and 92.9% have an ACR below 100 (). The maximum value of 14,906 is, therefore, far above the maximum ACR of 2450 found by Ahlers et al. (Citation2006). The 90th percentile value is 70.85. If we apply the Ahlers et al. (Citation2006) value of 30 to discriminate between low and high ACR, 190 chemicals (79.2%) have a low and 50 (20.8%) a high ACR.
The 17 chemicals with ACR above 100 are presented in . Ahlers et al. (Citation2006) previously found that MOA 1 was a good predictor that a substance would have a lower ACR, although other MOAs were not necessarily associated with higher ACRs; however, quite unexpectedly, six (35%) of these high ACR are MOA 1 chemicals, five (29%) are MOA 4 chemicals, three (17%) are MOA 2 chemicals and three (17%) are MOA 5 chemicals. The MOA 1 are genistein, monoethanolamine, dimethylamine, peracetic acid and diuron.
Table 5. Name and mode of action (MOA) of chemicals with acute-to-chronic ratio (ACR) >100.
Propanil, monoethanolamine and genistein were mentioned previously as they were identified as outliers in the MOA 1 acute fish-to-chronic fish relationship. Propanil has the highest ACR (14906). This is in line with previous work reporting an ACR of 18,100 (Kenaga Citation1982). This ACR is based on one LC50 entry (8.68 mg/L) and five NOEC entries from two studies, ranging from 0.0004 to 0.0012 mg/L. Other data sources, such as the US EPA in the Reregistration Eligibility Decision, retain a NOEC value of 0.0091 mg/L (236 days), and an LC50 of 2.3 mg/L. This would result in an ACR of 252 being still considered as a high ACR but much lower than the previous one derived from our database.
Dimethylamine is a secondary amine also characterised by high fish LC50 values (17, 118, 120, 210, 396 mg/L, derived with three different species, O. mykiss, D. rerio and P. reticulata). One NOEC value of 0.6 mg/L is reported for egg mortality, which is the only one considered in our analysis as it is the only precise NOEC value reported (the other NOEC values are reported as >10 and >20 mg/L). Therefore, the difference in sensitivity here might be due to the particular life stage considered for this study.
Regarding peracetic acid, nine LC50 values were reported in the ECHA database, ranging from 0.078 to 2 mg/L and derived with three different species (L. macrochirus, O. mykiss, D. rerio), whereas only one NOEC value (0.0022 mg/L; D. rerio) was reported. Diuron is an herbicide acting as photosynthesis inhibitor by blocking the plastoquinone binding site of photosystem II (Metz et al. Citation1986), classified as MOA 1 in the Verhaar classification scheme. Two LC50 values were reported for this chemical (14 and 14.7 mg/L, on P. promelas and O. mykiss, respectively) and five NOEC values (from 0.029 to 0.41 mg/L). The lowest values (0.0269 and 0.0334 mg/L) were extracted from the US EPA Ecotox database, from a single study carried out in 1987 on P. promelas. The highest value was extracted from the ECHA database (0.41 mg/L) from a study carried out in 1993 on O. mykiss.
Chlorothalonil (MOA 4), also identified as an outlier in the acute to chronic fish relationship, has the second highest ACR (4646). This high ACR is based on one fish LC50 (0.278 mg/L) and one NOEC value (0.00006 mg/L). This high chronic toxicity to fish, extracted from the ECOTOX US EPA database, was found in the literature (Teather, Jardine, and Gormley Citation2005), and is related to hatching survival of fertilised eggs after 36 days of exposure. However, when going back to the original study, it appears that 0.00006 mg/L was the only concentration tested (i.e. the concentration for which we start to observe an effect might be higher) and that the three duration schemes were 7 days, 3 weeks and 5 months and not 36 days. Therefore, this data might not be reliable.
The two chemicals with the next highest ACR have also been previously identified as outlier in the acute-to-chronic fish relationship: ethylparathion (MOA 4) and 2-naphthol (MOA 2). Only one NOEC value is reported for ethylparathion (0.17 μg/L, on growth), along with two LC50 values (0.5 and 0.51 mg/L). All these data come from the same study; however, it was not possible to access the original reference. Regarding 2-naphtol, only one LC50 and one NOEC value are available (3.46 and 0.001 mg/L, respectively), both extracted from the ECHA database. It was not possible to retrieve more details that could explain these high ACR.
3,4-Dichloroaniline (MOA 3, ACR = 430) is a data-rich chemical with 24 NOEC values, ranging from 0.0011 to 0.23 mg/L and derived with five different fish species and 20 LC50 values, ranging from 1.94 to 13.3 mg/L. It was not possible to go back to all of the original studies but high ACR (i.e. 1200) has already been reported for this chemical in the literature (Call et al. Citation1987).
Fonofos ACR (MOA 4, ACR = 468) is based on one LC50 value (1.09 mg/L on P. promelas) and one NOEC value (0.00233 mg/L on L. macrochirus) (Fairchild, Little, and Huckins Citation1992). In the same study, an LC50 of 5.3 μg/L (0.0053 mg/L) was reported for this organothiophosphate insecticide (L. macrochirus, 96 h) which was not included in our database. Regarding tert-butylphenol (MOA 2), the ACR of 521 is also based on only two data entries, one LC50 value (5.21 mg/L) and one NOEC value (0.01 mg/L), on the same species, P. promelas. The NOEC value comes from the ECHA database from an early life stage toxicity study (2008, ranked Klimisch score 1). The ECHA database also reports an LC50 value >1 mg/L.
Atrazine (MOA 5, ACR = 232) is another data-rich chemical with 12 NOEC values ranging from 0.0302 to 0.21 mg/L, on two different species (P. promelas and L. macrochirus) and different life stages (juvenile, larvae and adults). Two LC50 values are reported (20.5 and 18.8 mg/L) from two studies on two different species (O. mykiss and C. carpio). Atrazine is a triazine herbicide which acts by binding to the plastoquinone-binding protein in photosystem II. Atrazine has also been shown to have endocrine-disrupting effects (Mizota and Ueda Citation2006; Prossnitz, Arterburn, and Sklar Citation2007) including on wildlife (Hayes et al. Citation2003, Citation2002).
2,6-Dinitrotoluene (MOA 5, ACR = 193) is a nitroaromatic chemical used in the production of flexible polyurethane foams and as a plasticiser, deterrent coating and burn rate modifier in propellants. It is known to be toxic by converting haemoglobin into methaemoglobin and to be carcinogenic. Only two LC50 values (34 and 18.6 mg/L) and one NOEC value were reported (0.13 mg/L), for which it was not possible to go back to the original study.
3.2.4. Acute-to-chronic ratio and MOA
The distribution of the ACR according to MOA () shows that 100% of the MOA 3 chemicals have an ACR within a factor of 100, 56.7% within a factor of 10. 90.9% and 88.2% of the MOA 2 and MOA 4 chemicals, respectively, have an ACR within a factor of 100, of which 50% and 47% have an ACR within a factor of 10. However, only 85.5% of the MOA 1 chemicals are within an ACR of 100, with 53.2% chemicals with an ACR below 10.
Overall, these results show that an ACR of 100 when applied to acute fish toxicity data for substances with MOA 3 and 2, as defined by the Verhaar classification scheme, will protect against the chronic effects of industrial substances in, respectively, 100% and 90% of the cases. That is in line with some previous work that shows that an ACR of 100, as implied by the European guidance (CitationECHA 2008), is protective for >90% of the industrial chemicals (May and Hahn Citation2014). However, for MOA 4 and MOA 1 chemicals, an ACR of 100 would be sufficiently protective for only 88% and 85% of the chemicals, respectively.
4. Conclusions
Although the lowest value is usually used in environmental RA in the interest of being as protective as possible, it has been shown that the use of the GM, calculated across the full range of empirical data available, allows more stable and representative estimates of chronic fish toxicity, and improves the goodness-of-fit of the linear regressions, by decreasing the statistical influence of outliers.
Our results show that the best fitted relationships for predicting chronic fish toxicity are based on acute fish data (r2 = 0.87). Unexpectedly, acute Daphnia data (r2 = 0.64) gives a stronger relationship with chronic fish data than chronic Daphnia data (r2 = 0.59) when dealing with the whole set of chemicals regardless of the MOA. When considering the MOA according to the Verhaar scheme, MOA 3 and MOA 1 chemicals give the strongest Daphnia-to-chronic fish relationship. However, the relationships obtained with acute Daphnia data show a much better fit (r2 = 0.83 and 0.69 for MOA 3 and MOA 1, respectively) than the one obtained with chronic Daphnia data (r2 = 0.66 and 0.65 for MOA 1 and MOA 3, respectively). When considering acute fish data, all the MOAs give highly fitted relationships (r2 = 0.88 for MOA 3 and MOA 5 chemicals, 0.85 for MOA 4 chemicals and 0.83 for MOA 1 and MOA 2 chemicals).
Therefore, when acute fish toxicity data are available, they might give a reliable basis to extrapolate to chronic fish toxicity as a first tier assessment or within a weight of evidence approach. When no acute fish toxicity data are available, acute Daphnia toxicity data could be used as a surrogate at least for MOA 3 and MOA 1 chemicals. For MOA 4 chemicals, it has to be highlighted that this group brings together chemicals with highly specific toxicity and particular MOA; i.e. it considers as a whole chemicals acting by various and different MOAs such as neurotoxicants, AchE inhibitors and respiratory inhibitors. Moreover, the more specific is an MOA, the more uncertain will be the extrapolation of toxicity data to other species, especially if the mode of action is not conserved across taxa. This explains why the relationships obtained with MOA 4 are not significant and/or not well-fitted. It would be interesting to further breakdown this group to look at each specific MOA individually.
There is a correlation between chemicals with high ACR values or interspecies SR and the outliers identified in the above-mentioned relationships. When considering chemicals with a high interspecies SR, Daphnia being more sensitive than fish, several aniline derivatives and pesticides acting through cholinesterase inhibition were identified. When considering high interspecies SR chemicals for which Daphnia is less sensitive than fish, we found pesticides and known endocrine disruptors such as ethynil estradiol and 17β-estradiol.
Extreme interspecies SRs were mainly MOA 2, 4 and 5 chemicals. Regarding the ACR between acute and chronic fish toxicity data, around 50% of the chemicals of each MOA class have an ACR within a factor of 10, whereas 100%, 90.9%, 88.3% and 85.5% of the MOA 3, MOA 2, MOA 4 and MOA 1 chemicals, respectively, have an ACR within a factor of 100. Therefore, the SF of 100 commonly applied in environmental risk assessment does not seem to be equally protective for every MOA. The ACR can be used as a first tier approach; however, regression models provide more accurate, MOA-specific extrapolations from acute to chronic toxicity.
Thus, when acute toxicity data are available, regression equations seem to provide a reliable, MOA-specific means of extrapolation from acute to chronic toxicity data, and could be used as a first-tier approach in an integrated testing strategy in order to waive long-term toxicity testing on fish. The extrapolation of chronic fish toxicity can be based on acute fish toxicity data irrespective of MOA, as well as on acute Daphnia data, for MOA 3 and MOA 1 chemicals. MOA 5 chemicals are an heterogeneous group and need to be better characterised in terms of their MOA in order to develop additional MOA-based regression models.
In the future, new tools could help to strengthen extrapolation approaches. For example, adverse outcome pathways (AOPs) could help to identify where cross-species extrapolation is appropriate (Ankley et al. Citation2010; Burden et al. Citation2015) based on the conservation of the toxicological pathway between species. AOPs could also help in the grouping of chemicals based upon a common molecular initiating event (MIE) or other key event (KE) to refine and improve relationships by restricting them to more specific subsets of chemicals. For data-poor chemicals, another option would be the use of the threshold of toxicological concern (TTC) approach, which aims to establish a level of exposure for chemicals below which there would be no appreciable risk. This concept is well established for assessing human safety and has been reapplied for a wide range of structural classes. Use of the TTC in environmental safety is just beginning, and key questions focus on hazard extrapolation of diverse taxa across trophic levels, importance of mode of action, and whether safe concentrations for ecosystems estimated from acute or chronic toxicity data are equally useful and in what context (Belanger et al. Citation2015). A tiered approach in which each tier allows for greater accuracy of prediction could be based on the sequential use of the TTC, ACR and interspecies ratio values, and MOA-based regression models that generate chemical-specific predictions of chronic toxicity.
Database-S1.xlsx
Download MS Excel (66.2 KB)Disclosure statement
No potential conflict of interest was reported by the authors.
References
- Ahlers, J., C. Riedhammer, M. Vogliano, R.-U. Ebert, R. Kühne, and G. Schüürmann. 2006. “Acute to Chronic Ratios in Aquatic Toxicity-Variation Across Trophic Levels and Relationship with Chemical Structure.” Environmental Toxicology and Chemistry 25 (11): 2937–2945. doi:10.1897/05-701r.1.
- Ankley, G.T., R.S. Bennett, R.J. Erickson, D.J. Hoff, M.W. Hornung, R.D. Johnson, D.R. Mount, et al. 2010. “Adverse Outcome Pathways: A Conceptual Framework to Support Ecotoxicology Research and Risk Assessment.” Environmental Toxicology and Chemistry 29 (3): 730–741. doi:10.1002/etc.34
- Austin, T.J., and C.V. Eadsforth. 2014. “Development of a Chronic Fish Toxicity Model for Predicting Sub-Lethal NOEC Values for Non-Polar Narcotics.” SAR and QSAR in Environmental Research 25 (2): 147–160. doi:10.1080/1062936X.2013.871577
- Barron, M.G., C.R. Lilavois, and T.M. Martin. 2015. “MOAtox: A Comprehensive Mode of Action and Acute Aquatic Toxicity Database for Predictive Model Development.” Aquatic Toxicology (Amsterdam, Netherlands) 161C: 102–107. doi:10.1016/j.aquatox.2015.02.001
- Belanger, S.E., H. Sanderson, M.R. Embry, K. Coady, D. DeZwart, B.A. Farr, S. Gutsell, M. Halder, R. Sternberg, and P. Wilson. 2015. “It is Time to Develop Ecological Thresholds of Toxicological Concern to Assist Environmental Hazard Assessment.” Environmental Toxicology and Chemistry 34 (12): 2864–2869. doi:10.1002/etc.3132
- Burden, N., F. Sewell, M.E. Andersen, A. Boobis, J.K. Chipman, M.T.D. Cronin, T.H. Hutchinson, I. Kimber, and M. Whelan. 2015. “Adverse Outcome Pathways Can Drive Non-Animal Approaches for Safety Assessment.” Journal of Applied Toxicology 35 (9): 971–975. doi:10.1002/jat.3165
- Call, D.J., S.H. Poirier, M.L. Knuth, S.L. Harting, and C.A. Lindberg. 1987. “Toxicity of 3,4-Dichloroaniline to Fathead Minnows, Pimephales promelas, in Acute and Early Life-Stage Exposures.” Bulletin of Environmental Contamination and Toxicology 38 (2): 352–358.
- Cassani, S., S. Kovarich, E. Papa, P.P. Roy, L. van der Wal, and P. Gramatica. 2013. “Daphnia and Fish Toxicity of (Benzo)triazoles: Validated QSAR Models, and Interspecies Quantitative Activity-Activity Modelling.” Journal of Hazardous Materials 258–259: 50–60. doi:10.1016/j.jhazmat.2013.04.025
- Celander, M.C., J.V. Goldstone, N.D. Denslow, T. Iguchi, P. Kille, R.D. Meyerhoff, B.A. Smith, T.H. Hutchinson, and J.R. Wheeler. 2011. “Predictive Ecotoxicology Workshop - Species Extrapolation for the 21st Century.” Environmental Toxicology and Chemistry 30 (1): 52–63. doi:10.1002/etc.382
- Claeys, L., F. Iaccino, C.R. Janssen, P. Van Sprang, and F. Verdonck. 2013. “Development and Validation of a Quantitative Structure–Activity Relationship for Chronic Narcosis to Fish.” Environmental Toxicology and Chemistry/SETAC 32 (10): 2217–25. doi:10.1002/etc.2301
- EC (European Commission). 2006. “Regulation (EC) No 1907/2006 of the European Parliament and of the Council Concerning the Registration, Evaluation, Authorisation and Restriction of Chemicals (REACH)”. Official Journal of the European Union 3. OJ L396.
- EC (European Commission). 2013. “Seventh Report on the Statistics on the Number of Animals Used for Experimental and Other Scientific Purposes in the Member States of the European Union.” Official Journal of the European Union COM(2013)859 Final.
- ECETOC (European Centre For Ecotoxicology and Toxicology of Chemicals). 2007. Intelligent Testing Strategies in Ecotoxicology: Mode of Action Approach for Specifically Acting Chemicals. Technical Report No. 102. Brussels: ECETOC AISBL. ISSN-0773-0872-102.
- ECHA (European Chemicals Agency). 2008. “Characterisation of Dose [Concentration]–Response for Environment.” Chapter R.10 in Guidance on Information Requirements and Chemical Safety Assessment. Helsinki: European Chemicals Agency.
- EFSA (European Food Safety Authority). 2014. “Conclusion on the Peer Review of the Pesticide Risk Assessment for Aquatic Organisms for the Active Substance Imidacloprid.” EFSA Journal 12: 1–49. doi:10.2903/j.efsa.2014.3835
- Ellison, C.M., J.C. Madden, M.T.D. Cronin, and S.J. Enoch. 2015. “Investigation of the Verhaar Scheme for Predicting Acute Aquatic Toxicity: Improving Predictions Obtained from Toxtree Ver. 2.6.” Chemosphere 139: 146–154. doi:10.1016/j.chemosphere.2015.06.009
- Enoch, S.J., M. Hewitt, M.T.D. Cronin, S. Azam, and J.C. Madden. 2008. “Classification of Chemicals according to Mechanism of Aquatic Toxicity: An Evaluation of the Implementation of the Verhaar Scheme in Toxtree.” Chemosphere 73 (3): 243–248. doi:10.1016/j.chemosphere.2008.06.052
- EU (European Union). 2010. “Directive 2010/63/EU of the European Parliament and of the Council of 22 September 2010 on the Protection of Animals Used for Scientific Purposes.” Official Journal of the European Union. OJ L 276/33. doi:32010L0063
- EU (European Union). 2012. “Regulation (EU) No 528/2012 of the European Parliament and of the Council of 22 May 2012 Concerning the Making Available on the Market and Use of Biocidal Products.” Official Journal of the European Union. OJ L 167/1.
- Fairchild, J.F., E.E. Little, and J.N. Huckins. 1992. “Aquatic Hazard Assessment of the Organophosphate Insecticide Fonofos.” Archives of Environmental Contamination and Toxicology 22 (4): 375–379. doi:10.1007/BF00212556
- Fang, M., D. Chen, and C.S. Yang. 2007. “Dietary Polyphenols May Affect DNA Methylation.” The Journal of Nutrition 137 (1 Suppl): 223S–228S.
- Green, S.E. 2015. “In Vitro Comparison of Estrogenic Activities of Popular Women's Health Botanicals.” Master of Science Thesis, University of Illinois. doi:10.1017/CBO9781107415324.004
- Hayes, T., K. Haston, M. Tsui, A. Hoang, C. Haeffele, and A. Vonk. 2003. “Atrazine-Induced Hermaphroditism at 0.1 Ppb in American Leopard Frogs (Rana pipiens): Laboratory and Field Evidence.” Environmental Health Perspectives 111 (4): 568–575. doi:10.1289/ehp.5932
- Hayes, T., M. Tsui, K. Haston, C. Haeffele, A. Hoang, and A. Vonk. 2002. “Feminization of Male Frogs in the Wild.” Nature 419: 895–896.
- Hoekzema, C.C., A.J. Murk, B.J. Van de Waart, J.C.M. Van der Hoeven, and D.F. De Roode. 2006. “Alternative Approaches Can Greatly Reduce the Number of Fish Used for Acute Toxicity Testing.” Environmental Toxicology and Chemistry/SETAC 25 (5): 1322–1325. doi:10.1897/05-293R2.1
- Hutchinson, T.H., S. Barrett, M. Buzby, D. Constable, A. Hartmann, E. Hayes, D. Huggett, et al. 2003. “A Strategy to Reduce the Numbers of Fish Used in Acute Ecotoxicity Testing of Pharmaceuticals.” Environmental Toxicology and Chemistry/SETAC 22 (12): 3031–3036. doi:10.1897/02-558
- Jeram, S., J.M. Riego Sintes, M. Halder, J. Baraibar Fentanes, B. Sokull-Klüttgen, and T.H. Hutchinson. 2005. “A Strategy to Reduce the Use of Fish in Acute Ecotoxicity Testing of New Chemical Substances Notified in the European Union.” Regulatory Toxicology and Pharmacology 42 (2): 218–224. doi:10.1016/j.yrtph.2005.04.005
- Kar, S., and K. Roy. 2010. “First Report on Interspecies Quantitative Correlation of Ecotoxicity of Pharmaceuticals.” Chemosphere 81 (6): 738–747. doi:10.1016/j.chemosphere.2010.07.019
- Kenaga, E.E. 1982. “Predictability of Chronic Toxicity from Acute Toxicity of Chemicals in Fish and Aquatic Invertebrates.” Environmental Toxicology and Chemistry 1 (4): 347–358.
- Klimisch, H.J, M. Andreae, and U. Tillman. 1997. “A Systematic Approach for Evaluating the Quality of Experimental Toxicological and Ecotoxicological Data.” Regulatory Toxicology and Pharmacology 25 (1): 1–5.
- May, M., and S. Hahn. 2014. Comparison of Species Sensitivity of Daphnia and Fish in Acute and Chronic Testing - Report UBA-FB 27448. Hannover: Fraunhofer-Institut für Toxikologie und Experimentelle Medizin.
- Mayer, F.L., G.F. Krause, M.R. Ellersieck, and G. Lee. 1992. Statistical Approach to Predicting Chronic Toxicity of Chemicals to Fishes from Acute Toxicity Test Data – Report EPA/600/SR-92/091. Gulf Breeze, FL: United States Environmental Protection Agency.
- Mayo-Bean, K., K. Moran, B. Meylan, and P. Ranslow. 2012. ECOSAR Methodology Document. Washington, DC: US Environmental Protection Agency. https://www.epa.gov/sites/production/files/2015-09/documents/ecosartechfinal.pdf
- Metz, J.G., H.B. Pakrasi, M. Seibert, and C.J. Arntzer. 1986. “Evidence for a Dual Function of the Herbicide-Binding D1 Protein in Photosystem II.” FEBS Letters 205 (2): 269–274. doi:10.1016/0014-5793(86)80911-5
- Mizota, K., and H. Ueda. 2006. “Endocrine Disrupting Chemical Atrazine Causes Degranulation Through Gq/11 Protein-Coupled Neurosteroid Receptor in Mast Cells.” Toxicological Sciences 90 (2): 362–368. doi:10.1093/toxsci/kfj087
- Motulsky, H.J., and R.E. Brown. 2006. “Detecting Outliers When Fitting Data with Nonlinear Regression - A New Method Based on Robust Nonlinear Regression and the False Discovery Rate.” BMC Bioinformatics 7 (1): 1–20. doi:10.1186/1471-2105-7-123.
- Nendza, M., and A. Wenzel. 2006. “Discriminating Toxicant Classes by Mode of Action. 1. (Eco)toxicity Profiles.” Environmental Science and Pollution Research International 13 (3): 192–203.
- Netzeva, T.I., M. Pavan, and A.P. Worth. 2008. “Review of (Quantitative) Structure–Activity Relationships for Acute Aquatic Toxicity.” QSAR & Combinatorial Science 27 (1): 77–90. doi:10.1002/qsar.200710099
- OECD (Organisation for Economic Co-operation and Development) 2012. “QSAR Toolbox.” Software for Grouping Chemicals into Categoris and Filling Gaps in (Eco)toxicity Data Needs for Assessing the Hazards of Chemicals. https://www.qsartoolbox.org/home
- Patisaul, H.B., M. Melby, P.L. Whitten, and L.J. Young. 2002. “Genistein Affects ER Beta - but Not ER Alpha-Dependent Gene Expression in the Hypothalamus.” Endocrinology 143 (6): 2189–2197. doi:10.1210/endo.143.6.8843
- Patlewicz, G., N. Jeliazkova, R.J. Safford, A.P. Worth, and B. Aleksiev. 2008. “An Evaluation of the Implementation of the Cramer Classification Scheme in the Toxtree Software.” SAR and QSAR in Environmental Research 19 (5–6): 495–524. http://toxtree.sourceforge.net/
- Perkins, E.J., G.T. Ankley, K.M. Crofton, N. Garcia-reyero, C.A. Lalone, M.S. Johnson, J.E. Tietge, and D.L. Villeneuve. 2013. “Current Perspectives on the Use of Alternative Species in Human Health and Ecological Hazard Assessments.” Environmental Health Perspectives 121 (9): 1002–1010.
- Pinto, P.I.S., M.D. Estêvão, and D.M. Power. 2014. “Effects of Estrogens and Estrogenic Disrupting Compounds on Fish Mineralized Tissues.” Marine Drugs 12 (8): 4474–4494. doi:10.3390/md12084474
- Prossnitz, E.R.P., J.B. Arterburn, and L.A. Sklar. 2007. “GPR30: A G Protein-Coupled Receptor for Estrogen.” Molecular and Cellular Endocrinology 265–266: 138–142. doi:10.1016/j.mce.2006.12.010
- Raimondo, S., D. Vivian, and M. Barron. 2010. Web-Based Interspecies Correlation Estimation (Web-ICE) for Acute Toxicity: User Manual Version 3.1. Gulf Breeze, FL: US Environmental Protection Agency. A ccessed 30 August 2016. https://www.epa.gov/sites/production/files/documents/WebICE_User_manual.pdf
- Russom, C.L., S.P. Bradbury, S.J. Broderius, D.E. Hammermeister, and R.A. Drummond. 1997. “Predicting Modes of Toxic Action from Chemical Structure: Acute Toxicity in the Fathead Minnow (Pimephales promelas).” Environmental Toxicology and Chemistry 16 (5): 948–967.
- Sanchez-Bayo, F., and K. Goka. 2005. “Unexpected Effects of Zinc Pyrithione and Imidacloprid on Japanese Medaka Fish (Oryzias latipes).” Aquatic Toxicology 74 (4): 285–293. doi:10.1016/j.aquatox.2005.06.003
- Scholz, S., E. Sela, L. Blaha, T. Braunbeck, M. Galay-burgos, M. García-franco, N. Klüver, et al. 2013. “A European Perspective on Alternatives to Animal Testing for Environmental Hazard Identification and Risk Assessment.” Regulatory Toxicology and Pharmacology 67 (3): 506–530. doi:10.1016/j.yrtph.2013.10.003
- Sourceforge 2004. “Toxtree.” http://toxtree.sourceforge.net/
- Teather, K., C. Jardine, and K. Gormley. 2005. “Behavioral and Sex Ratio Modification of Japanese Medaka (Oryzias latipes) in Response to Environmentally Relevant Mixtures of Three Pesticides.” Environmental Toxicology 20 (1): 110–117. doi:10.1002/tox.20084
- Tebby, C., E. Mombelli, P. Pandard, and A.R.R. Péry. 2011. “Exploring an Ecotoxicity Database with the OECD (Q)SAR Toolbox and DRAGON Descriptors in Order to Prioritise Testing on Algae, Daphnids, and Fish.” Science of the Total Environment 409 (18): 3334–3343. doi:10.1016/j.scitotenv.2011.05.029
- Tillman, R.W., M.R. Siegel, and J.W. Long. 1973. “Mechanism of Action and Fate of the Fungicide Chlorothalonil (2,4,5,6-Tetrachloroisophthalonitrile) in Biological Systems: I. Reactions with Cells and Subcellular Components of Saccharomyces pastorianus.” Pesticide Biochemistry and Physiology 3 (2): 160–167. doi:10.1016/0048-3575(73)90100-4
- US-EPA (US Environmental Protection Agency) 2013. “Web-ICE v3.2.1, Interspecies Correlation Estimation.” Accessed 30 August 2016. https://www3.epa.gov/webice/webice/
- Verhaar, H.J.M., C.J. van Leeuwen, and J.L.M. Hermens. 1992. “Classifying Environmental Pollutants. 1: Structure–Activity Relationships for Prediction of Aquatic Toxicity.” Chemosphere 25 (4): 471–491.
- Wang, L., B. Waltenberger, E.M. Pferschy-Wenzig, M. Blunder, X. Liu, C. Malainer, T. Blazevic, et al. 2014. “Natural Product Agonists of Peroxisome Proliferator-Activated Receptor Gamma (PPARγ): A Review.” Biochemical Pharmacology 92 (1): 73–89. doi:10.1016/j.bcp.2014.07.018
- Weyers, A., B. Sokull-Klüttgen, J. Baraibar-Fentanes, and G. Vollmer. 2000. “Acute Toxicity Data: A Comprehensive Comparison of Results of Fish, Daphnia, and Algae Tests with New Substances Notified in the European Union.” Environmental Toxicology and Chemistry 19 (7): 1931–1933.
- Wishart, D.S., C. Knox, A.C. Guo, S. Shrivastava, M. Hassanali, P. Stothard, Z. Chang, and J. Woolsey. 2006. “DrugBank: A Comprehensive Resource for in Silico Drug Discovery and Exploration.” http://www.drugbank.ca/drugs/
- Zhang, X.J., H.W. Qin, L.M. Su, W.C. Qin, M.Y. Zou, L.X. Sheng, Y.H. Zhao, and M.H. Abraham. 2010. “Interspecies Correlations of Toxicity to Eight Aquatic Organisms: Theoretical Considerations.” Science of the Total Environment 408 (20): 4549–4555. doi:10.1016/j.scitotenv.2010.07.022
- Zvinavashe, E., T. Du, T. Griff, H.H. van den Berg, A.E. Soffers, J. Vervoort, A.J. Murk, and I.M. Rietjens. 2009. “Quantitative Structure–Activity Relationship Modeling of the Toxicity of Organothiophosphate Pesticides to Daphnia magna and Cyprinus carpio.” Chemosphere 75 (11): 1531–1538. doi:10.1016/j.chemosphere.2009.01.081