Abstract
Particle–vapor molecule (condensation) and particle–particle (coagulation) collision kernels are critically important parameters for the description of particle size distribution evolution in aerosols. We use mean first passage time calculations to find a dimensionless form of the collision kernel that applies in the limit where the ratio of the masses of colliding entities (aerosol particles or vapor molecules) to gas molecule mass, Z, approaches infinity, and the colliding entities are dilute in concentration. In these calculations, the motion of colliding entities is monitored with Langevin dynamics, and the collision kernel is inferred from the time required for collision. The presented analysis reveals that the dimensionless collisional kernel (H) is a function solely of the diffusive Knudsen number (KnD , the square root of the product of kT and the reduced mass divided by the product of the reduced friction factor and collision radius), and a number of commonly used expressions for the collision kernel also collapse to the functional form H(KnD ), irrespective of whether they were derived for Z → ∞ or Z → 0 conditions. The examined collision kernel expressions are further found to agree within 10% of our calculations as well as with each other across the entire KnD range. This result suggests that a single collision kernel can be used to describe all transition regime collision rates, i.e., both condensation and coagulation rates, with reasonable accuracy, and establishes that Langevin-equation-based mean first passage time calculations can serve as a simple approach to examine transition regime collision phenomena.
INTRODUCTION
The growth of particles in the gas phase by thermally driven collisions between particles (coagulation), particles and molecular clusters, and particles and vapor molecules (condensation/surface growth) plays a critical role in a number of aerosol processes, including new particle formation in the atmosphere (Kuang et al. Citation2009) and nanomaterials synthesis (Gurav et al. Citation1993; Swihart Citation2003). The behavior of these systems is largely governed by the collision rate, R (the number of collisions per unit time per unit volume of aerosol), the calculation of which is simplified by the fact that particles, clusters, and vapor molecules are typically found at low volume fractions in the gas phase. Under such dilute conditions, collisions can be modeled as two-body interactions wherein thermal motion drives collisions (Narsimhan and Ruckenstein Citation1985; Friedlander Citation2000), and the collision rate is given as:
Accurate calculation of collision rates thus requires accurate calculation of β. When the radius of at least one object is large relative to the mean persistence distance of the colliding entities (Rader Citation1985), the continuum approximation is satisfied and Smoluchowski's β applies (Chandrasekhar Citation1943; Given et al. Citation1997):
With few exceptions (Chernyak Citation1995; Gutsch et al. Citation1995; Heine and Pratsinis Citation2007; Azarov and Veshchunov Citation2010; Veshchunov Citation2010), there has been little recent focus on better understanding both Z → 0 and Z → ∞ thermally driven collision processes. Nonetheless, there are several reasons for further examination of the transition regime collision kernel. First, collision kernel expressions have been sparingly compared with results of Brownian dynamics simulations (Trzeciak et al. Citation2004; Madler et al. Citation2006; Heine and Pratsinis Citation2007), and when comparison has been performed (Nowakowski and Sitarski Citation1981; Narsimhan and Ruckenstein Citation1985), it has been limited to collisions between identical-sized particles or led to poor agreement with the unambiguous free molecular and continuum limiting expressions (Gutsch et al. Citation1995). With recent advances in computational capabilities, Brownian dynamics simulations can accurately predict thermally driven particle–particle collision rates (the Z → ∞ limit); hence, they should be able to provide a means for more detailed examination of collision kernel expressions than is possible with currently available experimental approaches. Second, various authors have used different definitions for the mean persistence distance of the colliding entities (Jeans Citation1954; Dahneke Citation1983; Adachi et al. Citation1985; Rader Citation1985; Sceats Citation1989; Gatti and Kortshagen Citation2008), and hence different definitions of the dimensionless parameter (i.e., the ratio of the persistence distance to the collision radius) used to determine whether a collision is continuum, free molecular, or transition regime process. We refer to this parameter as KnD , the diffusive Knudsen number (Loyalka Citation1976; Dahneke Citation1983; Rogak and Flagan Citation1992), which must approach zero in the continuum limit and approach ∞ in the free molecular limit. The lack of consensus on an appropriate definition of KnD has complicated comparisons between different collision rate expressions (Rader Citation1985), and therefore comparisons of available collision kernel expressions are sparsely performed. Finally, in examining collision processes, vapor molecule condensation has been examined in a distinctly different manner than has particle–particle coagulation. As noted above, the theoretical basis for this is clear; while aerosol particles are significantly more massive than gas molecules (Z ≫ 1), this need not be the case for vapor molecules. However, while the most commonly used collision kernel expression in condensation (the Fuchs–Sutugin kernel) derives from a Boltzmann equation solution in the limit of Z = 0, condensing vapor molecules or molecular clusters are often significantly more massive than the surrounding background gas (Li and Davis Citation1996), and for these species, the Z → ∞ collision kernel would be preferred. A single collision kernel should be able to describe both thermally driven condensation and coagulation in the dilute limit as Z → ∞, as the motion of all entities can be described in a similar manner. Furthermore, the extent to which Z influences the collision kernel can be better understood by comparing kernels inferred with the assumption Z = 0 to those inferred as Z → ∞.
Motivated by these issues, the purpose of this work is two-fold. First, we develop an expression for the collision kernel in the Z → ∞ limit, which converges to the correct continuum and free molecular expressions and, with an appropriate definition of KnD , can correctly predict collision kernels in the transition regime. For this purpose, we make use of mean first passage time calculations (Klein Citation1952; Narsimhan and Ruckenstein Citation1985), with the assumptions that the colliding entities are dilute in concentration and that the motion of colliding entities is accurately described by Langevin dynamics (Chandrasekhar Citation1943; Sitarski and Seinfeld Citation1977; Ermak and Buckholz Citation1980; Madler et al. Citation2006; Isella and Drossinos Citation2010). We further show that the transition regime collision kernel is best represented in a nondimensionalized form that can be predicted with Buckingham Π theorem (Buckingham Citation1914, 1915). The second goal of this study is to compare the mean first passage time derived collision kernel expression to previously developed Z → 0 and Z → ∞ expressions. In doing so, we show that all examined collision kernel expressions can also be written in this nondimensionalized form and that are all in good agreement with the results of first passage calculations, regardless of whether they were derived for low-mass vapor molecule condensation, coagulation, or using Fuchs’ limiting sphere approach.
MEAN FIRST PASSAGE TIME CALCULATIONS
Motion in the Transition Regime
To utilize mean first passage time calculations to determine the collision kernel, an appropriate model of the motion of colliding entities is necessary. This model is first described, followed by a description of the mean first passage time calculation procedure. We assume that for a dilute, hard-sphere, Z → ∞ entity surrounded by a homogenous bath gas under isothermal conditions, motion is well described by a Langevin equation (Chandrasekhar Citation1943), given as:


Use of the Langevin equation restricts the presented analysis to situations where the time required for entities to collide is significantly longer than the characteristic time of motion, thus allowing separation of the drag force and the fluctuating diffusion force acting on moving entities in the force balance equation. Fortunately, most realistic aerosol systems are sufficiently dilute in concentration for this criterion to hold valid. Solutions to Equation (4) were developed by Ermak and Buckholtz (1980), with which the velocity vector, , and position vector,
, at any time, t + Δt, can be predicted, provided that the velocity and position are known at the previous time, t, and that moving entity has a known mass and diffusion coefficient/friction factor. For hard-sphere entities, this solution can be expressed by the following equations:


Simulation Procedure
A number of previous studies have used the Langevin equation to examine transition regime collisions (Nowakowski and Sitarski Citation1981; Gutsch et al. Citation1995; Trzeciak et al. Citation2004; Heine and Pratsinis Citation2007; Isella and Drossinos Citation2010). For our calculations, we build upon the methods of Narsimhan and Ruckenstein (Citation1985), who were the first to note that in examining dilute systems, only the motion of two colliding entities need to be considered, as opposed to a large multientity computational domain. Here, however, we must be specific about the definition of a dilute system, as the simplification to monitoring two entities places a second condition on the presented analysis. In aerosol systems at thermal equilibrium, a large number of aerosol particles and vapor molecules are dispersed within a background gas. Assuming that initially there are no concentration gradients of particles or vapor molecules within this medium, the collision and subsequent sticking of two entities reduces the local particle/vapor molecule concentration, such that the probability of a collision occurring within the same region of space is reduced as compared with regions where a collision has not occurred. In a sufficiently dilute system, prior to any further collisions, aerosol particles and vapor molecules will again redisperse themselves via thermal motion such that no concentration gradients exist within the medium, hence the probability of collision is spatially uniform at all times. The simplification to monitor the motion of only two bodies applies only in this instance. Combined with the prior restriction arising from the use of the Langevin equation, the calculations utilized here thus only apply when the characteristic time of particle/vapor molecule motion is faster than the characteristic time for particle mixing, which in turn must be faster than the time for collision. Again, with the exception of highly concentrated aerosol systems (Heine and Pratsinis Citation2007), this assumption is reasonable for most systems studied experimentally. We also note that the assumption of homogenously distributed particles and vapor molecules differs from the assumption traditionally made in analyzing continuum regime collisions, i.e., that concentration gradients of particles and vapor molecules exist at steady state, driving the flux of particles and vapor molecules to a collector particle surface (Chandrasekhar Citation1943; Friedlander Citation2000). However, as shown by Veshchunov (Citation2010), in the continuum limit, analysis via the steady-state flux approach (the diffusion regime) and of dilute, homogenously distributed systems (the kinetic regime) lead to matching functional forms of the continuum regime collision kernel. For this reason, mean first passage time calculations and related Brownian Dynamics algorithms can and have been successfully employed to determine diffusion-limited reaction rates in the continuum regime (Klein Citation1952; Northrup et al. Citation1984, 1986; Douglas et al. Citation1994; Given et al. Citation1997) and are employed here for rate determination in the continuum, transition, and free molecular regimes.
Two types of mean first passage time calculations are performed in this work. First, collisions in which one entity is considerably smaller and faster than the other are examined, which represent collisions between a heavy vapor molecule or molecular cluster and a particle. In this case, the radius, mass, and friction factor of the particle are >> than that of the vapor molecule/cluster; thus, particle motion is negligible, and moving vapor molecule/cluster may be treated as a point mass. shows a simple depiction of this mean first passage time calculation, which we henceforth refer to as the single-entity calculation. A spherical particle of radius, ai
, is placed in the center of a square box with a side length of 50ai
and periodic boundary conditions. A single moving point mass of zero radius but nonzero fj
and mj
is also placed at a random location on the surface of the box, and a given a random velocity sampled from a Maxwell–Boltzmann distribution (Vincenti and Kruger Citation1975; at the specified temperature). To move the point mass in the simulation domain, we first nondimensionalize Equations (5a)–(5c) by defining Δτ = (fj
/mj
)Δt, , and
, where i denotes the immobile particle and j denotes the moving point mass, which gives:




The second set of mean first passage time calculations mimics particle–particle collisions (), in which the motion of both colliding entities is monitored. As two equations of motion are needed, we refer to this calculation as the two-entity calculation. Equations are again solved in a dimensionless fashion, with Δτ = (fij
/μij)Δt, , and
, where fij = fi fj
/(fi + fj
) is the reduced friction factor. This normalization gives the following two solutions for the motions of particles type i and type j:
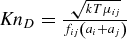
Identical convergence criteria are used for two-entity calculations as are for single-entity calculations, again with 500–1200 collisions necessary for each τ mean calculation and 10 τ mean calculations used for each reported value.
RESULTS AND DISCUSSION
Nondimensionalized Collision Kernels
Nondimensionalization of the Ermak and Buckholtz solution suggests that the dimensionless collision kernel, H, is dependent upon KnD for the single-entity system and upon KnD , θm , and θf for the two-entity system. With dimensional analysis, we can gain further insight into the correct functional form for the transition regime collision kernel. From Equations (2) and (3), it is apparent that the collision kernel when a single entity of negligible radius is moving depends upon the thermal energy, kT; the moving entity friction factor, fj ; the stationary particle radius, ai ; and the moving entity mass, mj (note fi → ∞, aj → 0, and mi → ∞ in the single-entity limit). These five variables form two dimensionless groups, which can be used to fully describe transition regime collisions: H and KnD (as defined for single-entity collisions), which are the nondimensionalizations of β and kT, respectively. The traditional normalization of β/βc (Fuchs Citation1964; Loyalka Citation1976; Narsimhan and Ruckenstein Citation1985; Veshchunov Citation2010) can also follow from dimensional analysis, where βc is the continuum collision kernel (Equation (2)) and the second dimensionless number is again KnD , but in this system it is the nondimensionalization of mj . A final approach to scale the collision process is to normalize β by βFM , the free molecular collision rate (Equation (3)), leading to KnD as a nondimensionalization of the friction factor. Here, we elect to use H for the dimensionless collision kernel as opposed to β/βc or β/βFM as nondimensionalization of the Langevin equations allows for H to be directly determined from mean first passage time calculations, and in both the continuum and free molecular regime, thermal energy drives collisions; hence, β and kT are the most appropriate nonrepeating variables in describing the collision process. H can be expressed in terms of the aforementioned variables as:
For collision processes with two moving entities, from Equations (2) and (3), β is found to be a function of kT, fi , fj , mi , mj , ai , and aj . The description of a system of eight variables should require five dimensionless groups. However, in Equations (2) and (3), the following groupings are found: fi and fj as fij , mi and mj as μij , and ai and aj as ai + aj . This again reduces the system to five variables, which can be described by H and KnD , where H is redefined as:
Figure 2 The nondimensionalized collision rate, H, for (a) single-entity and (b) two-entity mean first passage simulations as a function of KnD . Gray lines denote the expected limits as KnD → 0 and KnD → ∞. (c) A zoomed in plot of H(KnD ) in the region 0.05< KnD < 5. Closed symbols: single-entity mean first passage time calculations. Open symbols: two-entity mean first passage time calculations.
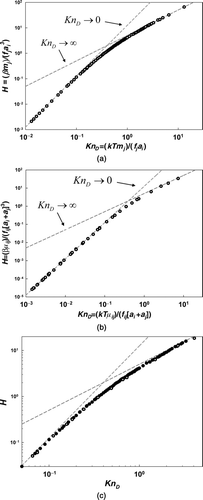
Mean First Passage Time Results
Mean first passage time simulations are performed at varying KnD for single entities and at varying KnD , θm , and θf for two moving entities. Calculated H values are shown at varying KnD for single- and two-entity systems in and b, respectively. Also shown in both figures are the low and high Knudsen number limiting expressions for H (Equations (11a) and (11b), dashed gray lines). Tables of results from mean first passage time calculations are given in the supplementary information. In performing two-entity calculations, we have used only θm and θf values that are realistic for collisions between entities of similar density in the continuum, transition, and free molecular regimes. This leads to a correlation between the examined θm and θf values, and although this places a restriction on the presented results, in realistic systems dominated by collision growth in which the motion of both colliding entities is important, the colliding entities typically have a similar density (i.e., collisions between a massive small particle and much less massive larger particle are rare). From both figures it is apparent that (1) H is a single valued function of KnD for both types of collisions (with further direct evidence provided in the supplementary information) and (2), at low and high Knudsen numbers, both shown data sets converge to the appropriate KnD → 0 and KnD → ∞ limits, respectively. This is in contrast with the earlier related study of Gutsch et al (1995), in which mean first passage time calculations grossly underpredicted both the continuum and free molecular collision kernels. How this underpediction arose is unclear, though it is perhaps due to an overly large time step used in order to minimize the time necessary for computation.
shows a zoomed in plot about the Knudsen number range from 0.05 to 5, with results from both single-entity (closed circles) and two-entity (open circles) calculations. Although several calculated two-entity results are slightly (∼3%) lower than single-entity results near KnD ≈ 1, the two sets of results collapse to a single curve remarkably well. Overall, these results support predictions made from dimensional analysis, namely, there is a single curve, H(KnD ), to describe collisions between dilute concentration, transition regime entities in the Z → ∞ limit.
Table 1 A summary of previous developed collision kernels for transition regime condensation and coagulation, expressed in the form H(KnD )
Comparison to Existing Kernels
Earlier studies of transition regime collisions via Langevin simulations suffered from high statistical variability in the obtained results or made use of a limited number of calculated values (Nowakowski and Sitarski Citation1981; Narsimhan and Ruckenstein Citation1985; Gutsch et al. Citation1995). Due to the small time steps used in calculations as well as the strict convergence criteria employed, the results obtained in this study do not suffer from the same degree statistical variability as prior work (though some random variations in results from Brownian Dynamics are inevitable). Therefore, we may consider results from mean first passage time calculations to be “in silico” derived data for comparison with existing collision kernels. While there are a number of collision kernels derived elsewhere, we choose to examine the following, which are used numerously in investigations of collision-governed systems: (a) the Fuchs–Sutugin (1970) expression for the condensation collision kernel in the limit Z = 0, (b) Loyalka's condensation collision kernel in the limit Z = 0 (Loyalka Citation1973), (c) Fuchs's expression (1964) for the coagulation kernel for which Z → ∞, (d) Dahneke's (1983) expression for the coagulation collision kernel with Z → ∞, (e) the recently developed collision kernel from Veshchunov (Citation2010) with Z → ∞, and (f) the hard-sphere limit of the collision kernel from Fuchs's diffusion charging theory (Fuchs Citation1963), which utilizes the limiting sphere concept. For appropriate comparison, each of these expressions must be recast in terms of H and KnD , which is shown in . We note that in rewriting these expressions, for any entity i, the diffusion coefficient in the original expression is replaced with kT/fi . This leads to fi as a function of Z in several expressions (as Di depends on Z for vapor molecules), but isolates all Z dependencies within the friction factor, which is an input to dimensionless collision kernel expressions. If present in expressions developed for coagulation collision kernels, the quantity 6πηai /Cc (Kni ) is also replaced with fi , where η is the dynamic viscosity of the surrounding gaseous medium. Cc (Kni ) is the empirical Cunningham (Citation1910) correction factor, which is a function of Kni , the momentum Knudsen number (Friedlander Citation2000) for entity i, as opposed to the diffusive Knudsen number, which applies to mass transfer processes and is dependent upon fi . Finally, in the nondimensionalization of Fuchs’ diffusion charging theory, the mean free path of the diffusing entity is taken to be (1.329/fi )(kTmi )1/2 (Adachi et al. Citation1985) and the expression given by Natanson (Citation1960) is used to determine the limiting sphere radius. With these definitions, all six examined collision kernel expressions appropriately collapse into the form H(KnD ), allowing for comparison to mean first passage time calculations. The Fuchs–Sutugin (“a” in ), Loyalka's (“b” in ), and Dahneke's expression (“d” in ) all fit the general form of:
Figure 3 Percent deviation of previously developed expressions for the collision kernel as a function of KnD . (a) Fuchs–Sutugin's condensation kernel, (b) Loyalka's condensation kernel, (c) Fuchs's coagulation kernel, (d) Dahneke's coagulation kernel, (e) Veshchunov's coagulation kernel, (f) the hard-sphere limit of Fuchs collision kernel, and (g) the regression Equation (14). Closed symbols: single-entity mean first passage time calculations. Open symbols: two-entity mean first passage time calculations. Black guide lines are marked at /±5% deviation, and gray guidelines are marked at ±10% deviation.
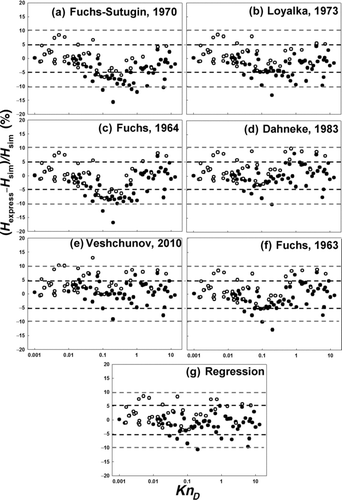
Figure 4 Comparison of six selected transition regime collision kernels (two derived for condensation, three for coagulation, and the limiting sphere model) to the collision kernel based on regression analysis of mean first passage time calculations. (Color figure available online.)
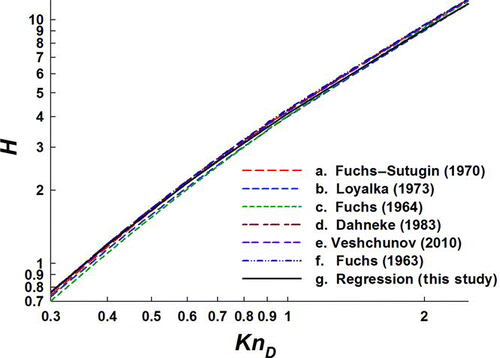
In addition to this comparison, we use mean first passage time calculations to construct a nondimensionalized collision kernel which may be used to predict particle–particle collision rates and, based on the good agreement with the Fuchs–Sutugin and Loyalka curves, also particle–vapor molecule collision rate with reasonable accuracy. Following Veshchunov (Citation2010), we propose a four parameter fit of the form:
Overall, through comparison of mean first passage time calculations to existing collision kernel expressions, we find that all examined expressions, regardless of the limits imposed during their derivation, are within several percent of mean first passage derived values and of one another across the entire diffusive Knudsen number range. On the basis of the comparisons made by Veshchunov (Citation2010), this excellent agreement is somewhat anticipated. Nonetheless, that mean first passage time calculations give rise to a similar collision kernel expression as do other approaches further suggests that a single collision kernel expression can be used to describe collision processes at all Z (both condensation and coagulation) across the entire KnD range, with an accuracy suitable for examination of almost all dilute aerosol systems.
As all examined collision kernel expressions are in excellent agreement with one another, we make no claim that the collision kernel expression inferred here is superior to others; rather, it is only introduced for appropriate comparison. We do note, however, that prior examinations of transition regime collisions have either required solution of the Boltzmann equation or unique mathematical insight into the collision process. For this reason, an understanding of particle collision processes outside of the continuum and free molecular limits is often difficult to gain for beginning practitioners in aerosol science. Unlike prior examinations, the approach employed here requires only prior knowledge of dimensional analysis (taught at the undergraduate level in most engineering curricula) as well as an understanding of force balances (such as the Langevin equation of motion). We therefore suggest that for pedagogical purposes, the mean first passage time approach to collision kernel development be widely utilized. Further, mean first passage time calculations can be applied to examine collisions with nonspherical particles much more readily than prior approaches, and via first passage analysis nonspherical particle collision examination is underway in our laboratory (Gopalakrishnan et al. Citation2011).
CONCLUSIONS
Mean first passage time calculations coupled with dimensional analysis are used to study transition regime collisions. On the basis of this work, we conclude the following:
1. | For hard-sphere, dilute entities whose motion can be described by the Langevin equation, the dimensionless collision kernel, H, can be determined solely as a function of the diffusive Knudsen number, KnD . Both H and KnD can be derived from nondimensionalization of the Langevin-based first passage time calculations, as well as by use of traditional dimensional analysis. | ||||
2. | A number of existing expressions for the collision kernel also collapse into the dimensionless functional form H(KnD ), and are compared with mean first passage time results. The good agreement between all examined kernels and our calculations strongly suggests that a single dimensionless collision kernel can be used to reliably infer both condensation and coagulation rates. Along these lines, a four parameter fit is developed from first passage time calculations for the collision kernel. | ||||
3. | The determined collision kernel applies rigorously only to hard-sphere, Z → ∞, entities in the dilute limit, whose friction factors (diffusion coefficients) do not vary spatially. Electrostatic and van der Waals forces often have an influence in gas-phase systems (Fuchs Citation1963; Sceats Citation1989; Huang et al. Citation1991; Filippov and Burtscher Citation1994) and may be included in analysis in future work. Such forces can be easily incorporated into the Langevin equation, and with forces included dimensional analysis will lead to the dimensionless collision kernel as a function not only of the diffusive Knudsen number, but also on additional dimensionless numbers that represent the magnitude of the potential interactions. It is also important to note that the determined function H(KnD ) is not influenced by the functional form of the friction factor [e.g., it may be inferred from the Stokes–Millikan equation (Larriba et al. Citation2011) or from Chapman–Enskog based diffusion coefficients], though friction factors must be known in order to determine β at a given H. | ||||
4. | While our findings indeed suggest that the influence of Z on the dimensionless collision kernel is small, the approach utilized here is vastly simpler than Boltzmann-equation-based approaches and results in an approximation to the true form of H(KnD ). More exact analysis, however, particularly of low-mass vapor molecule and particle collisions under nonisothermal conditions is still required to fully describe transition regime collisions. |
uast_a_601775_sup_20187883.zip
Download Zip (54.6 KB)Acknowledgments
The authors thank Professor Peter McMurry for numerous discussions on mass transfer processes in the transition regime. We also thank two anonymous referees for their thought-provoking comments on this work, as well as the Minnesota Supercomputing Institute (MSI) for providing the high performance computing hardware used in mean first passage time calculations. Partial support for this work was provided by National Science Foundation grants NSF-BES-0646507 and NSF-CHE-1011810.
[Supplementary materials are available for this article. Go to the publisher's online edition of Aerosol Science and Technology to view the free supplementary files.]
REFERENCES
- Adachi , M. , Kousaka , Y. and Okuyama , K. 1985 . Unipolar and Bipolar Diffusion Charging of Ultrafine Aerosol-Particles . J. Aerosol Sci. , 16 ( 2 ) : 109 – 123 .
- Azarov , I. B. and Veshchunov , M. S. 2010 . Development of the New Approach to the Brownian Coagulation Theory: Transition Regime . J. Eng. Thermophys. , 19 ( 3 ) : 128 – 137 .
- Brock , J. R. 1966 . Diffusion to Particles in the Near Free Molecular Regime . J. Colloid Interf. Sci. , 22 : 513 – 516 .
- Buckingham , E. 1914 . On Physical Similar Systems; Illustrations of the Use of Dimensional Equations . Phys. Rev. , 4 : 345 – 376 .
- Buckingham , E. 1915 . Model Experiments and the Forms of Empirical Equations . Trans. ASME , 37 : 263 – 296 .
- Chandrasekhar , S. 1943 . Stochastic Problems in Physics and Astronomy . Rev. Mod. Phys. , 15 : 1 – 89 .
- Chatterjee , A. , Kerker , M. and Cooke , D. D. 1975 . Brownian Coagulation of Aerosols in the Transition Regime . J. Colloid Interf. Sci. , 53 : 71 – 82 .
- Chernyak , V. 1995 . The Kinetic Theory of Droplet Evaporation . J. Aerosol Sci. , 26 : 873 – 885 .
- Cunningham , E. 1910 . On the Velocity of Steady Fall of Spherical Particles Through Fluid Medium . Proc. Roy. Soc. London Ser. A. , 83 ( 563 ) : 357 – 365 .
- Dahneke , B. E. 1983 . “ Simple Kinetic Theory of Brownian Diffusion in Vapors and Aerosols ” . In Theory of Dispersed Multiphase Flow , Edited by: Meyer , R. E. 97 – 133 . New York : Academic Press .
- Davis , E. J. and Ray , A. K. 1978 . Submicron Droplet Evaporation in the Continuum and Non-Continuum Regimes . J. Aerosol Sci. , 9 : 411 – 422 .
- Douglas , J. F. , Zhou , H. X. and Hubbard , J. B. 1994 . Hydrodynamic Friction and the Capacitance of Arbitrarily-Shaped Objects . Phys. Rev. E , 49 ( 6 ) : 5319 – 5337 .
- Ermak , D. L. and Buckholz , H. 1980 . Numerical-Integration of the Langevin Equation—Monte-Carlo Simulation . J. Comput. Phys. , 35 ( 2 ) : 169 – 182 .
- Filippov , A. V. and Burtscher , H. 1994 . Bipolar Charging of Aerosol-Particles in Electropositive Gas of High-Purity . Aerosol Sci. Technol. , 21 ( 1 ) : 37 – 45 .
- Friedlander , S. K. 2000 . Smoke, Dust, and Haze , New York : Oxford University Press .
- Fuchs , N. A. 1934 . Zur Theorie der Koagulation . Z. Phys. Chemie , 171 : 199 – 208 .
- Fuchs , N. A. 1959 . Evaporation and Droplet Growth in Gaseous Media , London : Pergamon Press .
- Fuchs , N. A. 1963 . On the Stationary Charge Distribution on Aerosol Particles in a Bipolar Ionic Atmosphere . Geofis. Pura Appl. , 51 : 185 – 193 .
- Fuchs , N. A. 1964 . The Mechanics of Aerosols , New York : Macmillan .
- Fuchs , N. A. and Sutugin , A. G. 1970 . Highly Dispersed Aerosols , Ann Arbor : Ann Arbor Science Publishers .
- Gatti , M. and Kortshagen , U. 2008 . Analytical Model of Particle Charging in Plasmas Over a Wide Range of Collisionality . Phys. Rev. E , 78 ( 4 ) : 046402
- Given , J. A. , Hubbard , J. B. and Douglas , J. F. 1997 . A First-Passage Algorithm for the Hydrodynamic Friction and Diffusion-Limited Reaction Rate of Macromolecules . J. Chem. Phys. , 106 ( 9 ) : 3761 – 3771 .
- Gopalakrishnan , R. , Thajudeen , T. and Hogan , C. J. 2011 . Collision Limited Reaction Rates for Arbitrarily Shaped Particles Across the Entire Diffusive Knudsen Number Range . J. Chem. Physics ,
- Gurav , A. , Kodas , T. , Pluym , T. and Xiong , Y. 1993 . Aerosol Processing of Materials . Aerosol Sci. Technol. , 19 ( 4 ) : 411 – 452 .
- Gutsch , A. , Pratsinis , S. E. and Loffler , F. 1995 . Agglomerate Structure and Growth-Rate by Trajectory Calculations of Monomer-Cluster Collisions . J. Aerosol Sci. , 26 ( 2 ) : 187 – 199 .
- Heine , M. C. and Pratsinis , S. E. 2007 . Brownian Coagulation at High Concentration . Langmuir , 23 ( 19 ) : 9882 – 9890 .
- Huang , D. D. , Seinfeld , J. H. and Okuyama , K. 1991 . Image Potential Between a Charged-Particle and an Uncharged Particle in Aerosol Coagulation Enhancement in All Size Regimes and Interplay with Vanderwaals Forces . J. Colloid Interf. Sci. , 141 ( 1 ) : 191 – 198 .
- Isella , L. and Drossinos , Y. 2010 . Langevin Agglomeration of Nanoparticles Interacting via a Central Potential . Phys. Rev. E , 82 : 011404
- Jeans , J. 1954 . The Dynamical Theory of Gases , New York : Dover .
- Kim , D. S. , Park , S. H. , Song , Y. M. , Kim , D. H. and Lee , K. W. 2003 . Brownian Coagulation of Polydisperse Aerosols in the Transition Regime . J. Aerosol Sci. , 34 : 859 – 868 .
- Klein , G. 1952 . Mean First-Passage Times of Brownian Motion and Related Problems . Proc. Roy. Soc. London Ser. A , 211 : 431 – 443 .
- Kuang , C. , McMurry , P. H. and McCormick , A. V. 2009 . Determination of Cloud Condensation Nuclei Production from Measured New Particle Formation Events . Geophys. Res. Lett. , 36 : L09822
- Larriba , C. , Hogan , C. J. , Attoui , M. , Borrajo , R. , Fernandez-Garcia , J. and Fernandez de la Mora , J. 2011 . The Mobility-Volume Relationship Below 3.0 nm Examined by Tandem Mobility-Mass Measurement . Aerosol Sci. Technol. , 45 : 453 – 467 .
- Li , W. and Davis , E. J. 1996 . Aerosol Evaporation in the Transition Regime . Aerosol Sci. Technol. , 25 : 11 – 21 .
- Loyalka , S. K. 1973 . Condensation on a Spherical Droplet . J. Chem. Physics. , 58 : 354 – 356 .
- Loyalka , S. K. 1976 . Brownian Coagulation of Aerosols . J. Colloid Interf. Sci. , 57 : 578 – 579 .
- Loyalka , S. K. 1982 . Condensation on a Spherical Droplet, II . J. Colloid Interf. Sci. , 87 : 216 – 224 .
- Madler , L. , Lall , A. A. and Friedlander , S. K. 2006 . One-Step Aerosol Synthesis of Nanoparticle Agglomerate Films: Simulation of Film Porosity and Thickness . Nanotechnology , 17 : 4783 – 4795 .
- Narsimhan , G. and Ruckenstein , E. 1985 . Monte-Carlo Simulation of Brownian Coagulation over the Entire Range of Particle Sizes from near Molecular to Colloidal—Connection Between Collision Efficiency and Interparticle Forces . J. Colloid Interf. Sci. , 107 ( 1 ) : 174 – 193 .
- Natanson , G. 1960 . On the Theory of Charging of Amicroscopic Aerosol Particles Resulting from the Capture of Gaseous Ions . Sov. Phys. Tech. Phys. , 5 : 538 – 551 .
- Northrup , S. H. , Allison , S. A. and Mccammon , J. A. 1984 . Brownian Dynamics Simulation of Diffusion-Influenced Bimolecular Reactions . J. Chem. Phys. , 80 ( 4 ) : 1517 – 1526 .
- Northrup , S. H. , Curvin , M. S. , Allison , S. A. and Mccammon , J. A. 1986 . Optimization of Brownian Dynamics Methods for Diffusion-Influenced Rate-Constant Calculations . J. Chem. Phys. , 84 ( 4 ) : 2196 – 2203 .
- Nowakowski , B. and Sitarski , M. 1981 . Brownian Coagulation of Aerosol Particles by Monte Carlo Simulation . J. Colloid Interf. Sci. , 83 : 614 – 622 .
- Rader , D. J. 1985 . Application of the Tandem Differential Mobility Analyzer to Studies of Droplet Evaporation and Growth. Ph.D. thesis , Minneapolis, MN : Department of Mechanical Engineering, University of Minnesota .
- Ray , A. K. , Davis , E. J. and Ravindran , P. 1979 . “ Determination of Ultra-Low Vapor Pressures by Submicron Droplet Evaporation ” . In J. Chem. Phys. Vol. 71 , 582 – 587 .
- Rogak , S. N. and Flagan , R. C. 1992 . Coagulation of Aerosol Agglomerates in the Transition Regime . J. Colloid Interf. Sci. , 151 ( 1 ) : 203 – 224 .
- Sahni , D. C. 1966 . The Effect of a Black Sphere on the Flux Distribution in an Infinite Moderator . J. Nucl. Energy , 20 : 915 – 920 .
- Sahni , D. C. 1983 . An Exact Solution of Fokker–Planck Equation and Brownian Coagulation in the Transition Regime . J. Colloid Interf. Sci. , 91 : 418 – 429 .
- Sceats , M. G. 1989 . Brownian Coagulation in Aerosols—The Role of Long Range Forces . J. Colloid Interf. Sci. , 129 : 105 – 112 .
- Seaver , A. E. 1984 . Closed Form Equations for the Evaporation Rate and Droplet Size of Knudsen Droplets . Aerosol Sci. Technol. , 3 : 177 – 185 .
- Shon , S.-N. , Kasper , G. and Shaw , D. T. 1980 . An Experimental Study of Brownian Coagulation in the Transition Regime . J. Colloid Interf. Sci. , 73 : 233 – 243 .
- Sitarski , M. and Nowakowski , B. 1979 . Condensation Rate of a Trace Vapor on Knudsen Aerosol from the Solution of the Boltzmann Equation . J. Colloid Interf. Sci. , 72 : 113 – 122 .
- Sitarski , M. and Seinfeld , J. H. 1977 . Brownian Coagulation in the Transition Regime . J. Colloid Interf. Sci. , 61 : 261 – 271 .
- Swihart , M. T. 2003 . Vapor-Phase Synthesis of Nanoparticles . Curr. Opin. Colloid Interface Sci. , 8 : 127 – 133 .
- Szymanski , W. W. , Majerowicz , A. and Wagner , P. E. 1989 . Measurement of Brownian Coagulation in Monodispersed and Bidispersed Liquid Aerosols . Aerosol Sci. Technol. , 11 : 1 – 10 .
- Trzeciak , T. M. , Podgorski , A. and Marijnissen , J. C. M. 2004 . Langevin Dynamics Simulation of Aerosol Coagulation in Highly Concentrated Systems . Inz. Chem. Procesowa , 25 : 1741 – 1746 .
- Veshchunov , M. S. 2010 . A New Approach to the Brownian Coagulation Theory . J. Aerosol Sci. , 41 : 895 – 910 .
- Vincenti , W. G. and Kruger , C. H. 1975 . Introduction to Physical Gas Dynamics , Huntington, NY : Krieger .
- Wagner , P. E. and Kerker , M. 1977 . Brownian Coagulation of Aerosols in Rarified Gases . J. Chem. Phys. , 66 : 638 – 646 .