Abstract
A new experimental technique has been developed to study the gas–particle partitioning behavior of primary organic aerosol (POA) emissions from combustion sources at atmospherically relevant concentrations. The technique involves slowly filling a Teflon chamber with a constant emission source. As aerosol concentrations increase inside the chamber, the gas–particle partitioning of semivolatile organics shifts to the particle phase, thus increasing the fuel-based POA emission factor. The technique allows characterization of partitioning under isothermal conditions and atmospherically relevant concentrations. The technique was evaluated using emissions from a small diesel engine; the measured changes in gas–particle partitioning agreed well with previously published data for this engine measured with a dilution sampler. The temperature dependence of the gas–particle partitioning was investigated by conducting experiments at three different temperatures (15°C, 26°C, and 33°C). Increasing organic aerosol concentration and decreasing temperature increased the fuel-based POA emission factor. The gas–particle partitioning data were fit using absorptive partitioning theory to determine the volatility distribution and enthalpy of vaporization (ΔH v) of the emissions. We have derived two fits; one using the volatility basis set approach and a second using a two-product model. Both fits are suitable for use in chemical transport models. These fits were tested using previously published thermodenuder data. Partitioning calculations predict that the gas–particle partitioning from POA emissions from this engine vary by about a factor of 4 across the atmospherically relevant range of temperature and organic aerosol concentrations. This underscores the semivolatile nature of POA emissions.
Copyright 2012 American Association for Aerosol Research
INTRODUCTION
Combustion systems emit a complex mixture of organic compounds that span a wide range of volatility (Hildemann et al. Citation1991; Schauer et al. Citation1999; Grieshop et al. Citation2009a). The primary organic aerosol (POA) is the fraction of these emissions that partition into the particle phase under atmospheric conditions. The semivolatile nature of POA complicates the definition of POA emission factors (Robinson et al. Citation2007, Citation2010).
Gas–particle partitioning of organic aerosol in the ambient atmosphere is considered to be an absorptive process (Pankow Citation1994a; Odum et al. Citation1996). Although the phase partitioning of individual organic compounds has been extensively studied, these data provide little insight into changes of bulk POA mass because the vast majority of the POA mass has not been speciated (Schauer et al. Citation1999). To address this problem, bulk partitioning data are often fit with partitioning theory to derive a set of lumped, surrogate compounds. This approach is widely used to treat secondary organic aerosol (SOA) partitioning (Odum et al. Citation1996; Griffin et al. Citation1999; Kalberer et al. Citation2000; Bian and Bowman Citation2002) and has more recently been applied to POA (Shrivastava et al. Citation2006; Grieshop et al. Citation2009a).
The gas–particle partitioning of POA has been measured with dilution samplers (Lipsky and Robinson Citation2006; Grieshop et al. Citation2009a), thermodenuders (Huffman et al. Citation2008, Citation2009; Grieshop et al. Citation2009a, Citation2009b, Citation2009c), and smog chambers (Grieshop et al. Citation2007, Citation2009a). For example, Lipsky and Robinson Citation(2006) used multiple dilution tunnels to dilute diesel exhaust and measure gas–particle partitioning at different concentrations. However, it is difficult to make measurements at atmospherically relevant concentrations with this method because of sampling artifacts and uncertain evaporation kinetics. A smog chamber-based dilution technique was developed by Grieshop et al. (Citation2007, Citation2009a) to better characterize the partitioning behavior at lower, more atmospherically relevant concentrations. However, wall losses limited the lowest OA concentrations that could be achieved. Thermodenuder data have also been used to reduce the uncertainty in the partitioning behavior in the lower concentration range and partitioning was measured down to organic aerosol concentrations (C OA) of 20 μg m−3 (Grieshop et al. Citation2009a). However, interpreting thermodenuder measurements is complicated by uncertainty in the enthalpy of vaporization (ΔH v) and the evaporation kinetics of the aerosol (An et al. Citation2007; Stanier et al. Citation2007; Cappa and Jimenez Citation2010; Saleh et al. Citation2011). Therefore, new techniques to measure POA partitioning over the full range of atmospheric conditions are needed.
The sensitivity of gas–particle partitioning to changes in temperature depends on ΔH v (Tsigaridis and Kanakidou Citation2003; Donahue et al. Citation2006). Individual organic compounds found in POA have ΔH v of 100 kJ mol−1 or higher (Bilde et al. Citation2003; Cappa et al. Citation2007; Epstein et al. Citation2010). However, experimental studies of SOA report much less temperature sensitivity, with effective ΔH v values of ∼30 kJ mol−1 (Offenburg et al. 2006; Pathak et al. Citation2007; Stanier et al. Citation2007). Therefore, chemical transport models such as CMAQ (Carlton et al. Citation2010) and GEOS-Chem (Chung and Seinfeld Citation2002; Pye and Seinfeld Citation2010) use similarly low ΔH v. Such low values are hard to theoretically justify (Donahue et al. Citation2006).
This paper describes a new experimental technique to characterize the gas–particle partitioning of POA emissions from combustion systems across a wide range of atmospherically relevant conditions. The technique is demonstrated using exhaust from a small diesel generator. It is then applied to quantify partitioning behavior at different temperatures. The data are analyzed using absorptive partitioning theory and a volatility basis set approach (Donahue et al. Citation2006) to derive the volatility distribution and effective enthalpy of vaporization (ΔH v) of the emissions.
MATERIALS AND METHODS
Experimental Approach and Instrumentation
Experiments were performed to quantify the gas–particle partitioning of POA emitted by a diesel generator (Yanmar L-A series air-cooled 1-cylinder, 4-cycle 6.6 HP) across a range of atmospherically relevant conditions. Experiments were conducted at medium (55–65%) load. At this load, the particle phase emissions from this engine are dominated by OC (Lipsky and Robinson Citation2005, Citation2006). The aerosol mass spectrum of the POA emitted from this engine is very similar to that of other diesel engines (Grieshop et al. Citation2009a).
The basic experiment involved injecting a series of small aliquots of exhaust into a 10-m3 temperature-controlled Teflon smog chamber (Welch Fluorocarbon, Dover, NH; ). The experimental design was motivated by traditional secondary OA yield experiments. However, instead of chemistry increasing the concentrations of semivolatile material, each aliquot of exhaust increased the aerosol concentrations inside the chamber, shifting gas–particle partitioning of semivolatile organics to the particle phase. Measuring the changes in partitioning over a range of concentrations (series of injections) creates a dataset that can be fit with absorptive partitioning theory to determine the volatility distribution of the emissions.
The chamber was initially filled with high-efficiency-particulate-air (HEPA)- and activated-carbon-filtered air. Exhaust was injected into the chamber through a heated inlet using an ejector dilutor (Dekati Ltd., DI1000). Both the inlet and air for the ejector dilutor were maintained at 150°C to minimize thermophoretic/condensational losses. When the hot exhaust entered the chamber, it was rapidly mixed with chamber air, which cooled the exhaust to the chamber temperature. Aliquots ranged in size from 60 to 300 L (0.6–3% of the chamber volume). The chamber was maintained at a constant temperature (±1°C of set point) throughout each experiment.
After each injection, the aerosol concentrations inside the chamber were allowed to mix before another aliquot was added to the chamber. This procedure was repeated so that the POA concentrations inside the chamber were systematically increased from around 1 to more than 100 μg m−3 (and in some cases >1000 μg m−3) over the course of an experiment. The experiments therefore span a range that includes the atmospherically relevant concentrations of OA (∼0.5–20 μg m−3) and superambient (plume) conditions. Spanning such a wide range of OA concentrations helps to both constrain partitioning at ambient conditions and describe the distribution of organic material more volatile than what is found in ambient particles that is often missed by traditional analyses (Robinson et al. Citation2007).
The diesel engine was warmed up for 30–60 min and then operated at a constant load during each experiment. We assume that the engine emissions were constant over the course of the experiment and that any changes in POA emission factor are due to changes in gas–particle partitioning caused by increasing aerosol concentrations in the chamber. This assumption is supported by two pieces of evidence. The engine-out CO2 concentration measured in the undiluted exhaust stream and the fuel-based black carbon emission factor were both constant (±10%) over the course of an individual experiment.
A suite of instruments was used to measure gas and particle concentrations inside the chamber including a scanning mobility particle sizer (SMPS, TSI Inc.), a quadrupole aerosol mass spectrometer (AMS, Aerodyne Research Inc.) (Jayne et al. Citation2000; Canagaratna et al. Citation2007), an Aethalometer (Magee Scientific, AE31), an in situ OC/EC analyzer (Sunset Laboratory Inc.), and a CO2 analyzer (Licor, LI820). The SMPS was located inside the temperature-controlled room to measure the aerosol size distribution at the same temperature as the chamber.
Dilution of the exhaust in the chamber was determined by measuring the background-corrected CO2 mixing ratio (Lipsky and Robinson Citation2005). The OA concentration measured by the AMS was determined using the fragmentation table of Allan et al. Citation(2004) and corrected for interference from gas-phase CO2. The total organic (aerosol + vapor) concentration was estimated using OC/EC analysis of samples collected on an undenuded quartz filter following the approach of Shrivastava et al. (Citation2006). The black carbon (BC) data obtained with the Aethalometer were corrected for particle loading using the method of Kirchstetter and Novakov (Citation2007).
Particle emissions data are reported as fuel-based emission factors (particle mass per unit mass of fuel burnt)
Wall Loss Corrections
Particle mass is lost to the walls during an experiment. The POA and BC concentrations were corrected for wall losses using the approach of Weitkamp et al. Citation(2007)
A key uncertainty is the loss of semivolatile organic vapors to the chamber walls (P wall). These losses are accounted for using Equation (2c). We consider two limiting cases (Weitkamp et al. Citation2007). When semivolatile vapors only partition the suspended particles, ω = 0, and P wall = 0 (i.e., there is no loss of semivolatile vapors to the wall). The second case assumes that the particles lost to the walls remain completely in equilibrium with the gas phase, ω = 1. P wall = 0 for the wall loss correction of BC.
The wall-loss-corrected POA concentration (C OA = C sus + C wall) is determined by first numerically integrating Equation (2a) to determine P sus, using the k w determined from fitting the measured decay of the BC concentration. P wall from Equation (2c) is substituted into Equation (2b) and solved to determine the aerosol mass lost on the chamber wall (C wall). The upper and lower limits on the wall-loss-corrected concentrations are considered by taking both ω = 0 and ω = 1 cases. Since the exhaust aliquots are successively added to the chamber, the suspended mass is generally much larger than the mass on the walls, which minimizes the difference between the ω = 0 and ω = 1 cases. This was a key motivation for the experimental design; it helps minimize the uncertainty associated with loss of semivolatile vapors to the walls, avoiding the potentially large uncertainties associated with in-chamber dilution (Grieshop et al. Citation2007, Citation2009a). The result is that there is little difference between the ω = 0 and ω = 1 cases; therefore, we only present the ω = 1 case here.
Fitting Methods
The experimental data were fit using absorptive partitioning theory to determine the volatility distribution and ΔH v of the emissions. The core analysis fits the data using the volatility basis set of Donahue et al. Citation(2006), which distributes the organic emissions across logarithmically spaced bins of effective saturation concentration, C* (μg m−3). We also fit the data using a two-product model similar to what is traditionally used to parameterize SOA chamber experiments (Odum et al. Citation1996; Griffin et al. Citation1999) in many models (Carlton et al. Citation2010; Pye and Seinfeld Citation2010).
Since the emissions are dominated by OC, gas–particle partitioning is assumed to be an absorptive process. Therefore, the mass fraction of organic mass in the condensed phase is (Pankow Citation1994b; Shrivastava et al. Citation2006; Donahue et al. Citation2006; Robinson et al. Citation2007)
The temperature sensitivity of C* is governed by the enthalpy of vaporization (ΔH v) via the Clausius–Clapeyron equation.
Equations (3) and (4) were fit to a large set of measured X p and C OA values from experiments conducted at different chamber temperatures to determine the volatility distribution and ΔH v of the emissions. C OA is the wall-loss-corrected AMS mass. X P is the ratio of C OA to the total organic emissions, C tot. Following the approach of Shrivastava et al. Citation(2006), C tot was estimated from the quartz filter OC measurements made with the in situ carbon analyzer after each aliquot became well-mixed inside the chamber. The filter collects the POA and some fraction of the semivolatile organic vapors as artifact (Lipsky and Robinson Citation2006). Although an imperfect measure of C tot, quartz filters are widely used to characterize POA emissions from combustion systems. An OM/OC factor of 1.2 was used to convert the quartz filter data to organic mass (Russell Citation2003).
We present two types of fits in this manuscript: a 6-bin basis set fit covering the C* range 10−2–103 μg m−3 (Donahue et al. Citation2006) and a two-product model fit. The C* values in the basis set fit are fixed, whereas in the two-product model they are free parameters. The volatility distribution (set of fi for the basis set fit or Ci* and fi for two-product model) and ΔH v were determined by simultaneously fitting data from experiments conducted at three temperatures (15°C, 26°C, 33°C).
Fitting was performed to determine the optimum volatility distribution (set of fi ) and ΔH v using a generalized reduced gradient (GRG2) algorithm that minimized
For both fits, two constraints were imposed on the fi values: 0.01 ≤ f i < 1 and the sum of the fi values was unity. For the basis set fit, we assumed that the ΔH v decreased linearly with the log of C* (Donahue et al. Citation2006; Grieshop et al. Citation2009a; Epstein et al. Citation2010) and that the slope of ΔH v(C*) would be less than or equal to that found for pure components in Epstein et al. Citation(2010). For the two-component fit, we assumed that ΔH v was constant.
RESULTS AND DISCUSSION
Evolving Organic Partitioning
Time series of measured aerosol and CO2 data from a typical experiment are shown in . plots wall-loss-corrected POA concentrations measured with the AMS, the wall-loss-corrected black carbon concentration measured with the Aethalometer, and the CO2 concentration. The alternating unshaded and shaded regions in the figure show when aliquots of exhaust were added to the chamber and subsequently allowed to equilibrate.
FIG. 2 (a) Time series of CO2 concentration and wall-loss-corrected black carbon and POA concentrations. (b) Wall-loss-corrected emission factors of black carbon and POA. Alternate shaded and unshaded regions show the individual exhaust injections. The open symbols indicate samples influenced by incomplete mixing while filled symbols indicate well-mixed data. The relatively constant black carbon emission factor indicates constant engine performance while the increase in POA EF is due to enhanced gas–particle partitioning with increasing concentration. (Color figure available online.)
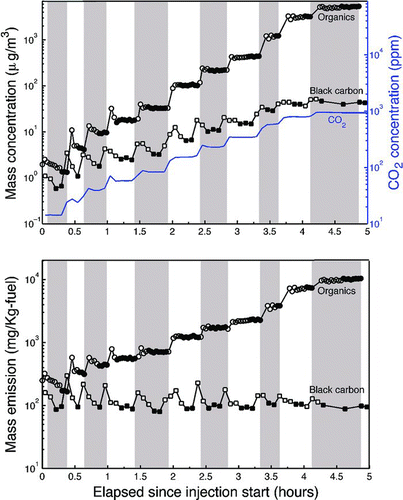
Each exhaust aliquot increased the CO2, POA, and BC concentrations inside the chamber. After each injection (intersection of shaded and unshaded regions), aerosol concentrations initially rose and then fell due to mixing, eventually reaching a steady state value. The mixing time scale was 5–10 min. Open symbols in are used to indicate the data influenced by incomplete mixing while the filled symbols indicate equilibrium concentrations. For some of the aliquots, it appears that the POA equilibrated faster than the BC; however, the AMS (1 min per sample) and Aethalometer (5 min per sample) had different temporal resolutions.
shows the time series for wall-loss-corrected BC and POA EF. As expected, the well-mixed BC EF were essentially constant, suggesting stable engine emissions. In contrast, the POA EF systematically increased, indicating that the increase in aerosol concentration associated with each aliquot of exhaust shifted gas–particle partitioning of semivolatile organics to the condensed phase.
presents the data on a partitioning plot, which is a scatter plot of the overall particle fraction (X p) versus C OA. X p is the ratio of C OA to the total (gas + particle; C tot) concentration of semivolatile material estimated from the quartz filter data. C OA (= C sus + C wall) is measured by the AMS, as described above.
FIG. 3 Partitioning plot showing change in particle fraction of semivolatile organic material (X p) as a function of wall-loss-corrected organic aerosol concentration (C OA). The experimental data from this study are compared with published data from the chamber dilution method of Grieshop et al. Citation(2009a) and dilution tunnel measurements of Shrivastava et al. Citation(2006) for the same diesel engine.
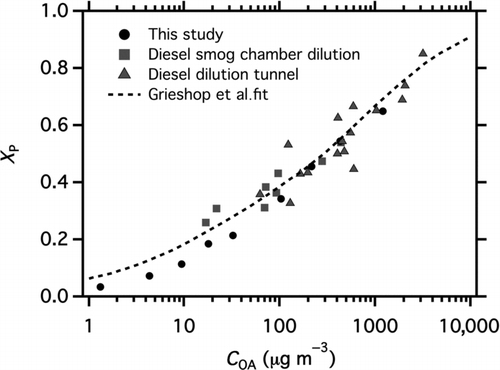
shows that, as expected from partitioning theory (Equation (3)), X p monotonically increases toward one with increasing C OA, reflecting changes in partitioning as organic material shifts from the gas to the particle phase at higher POA concentrations. The shape of the partitioning curve is governed by the volatility distribution of the organic emissions. also highlights an important advantage of the new experimental design, namely that it allows measurements to be made across the entire atmospherically relevant range of C OA down to 1 μg m−3. In contrast, previous measurements only reached a C OA of ∼20 μg m−3 due to issues with signal-to-noise ratios and/or wall losses (Grieshop et al. Citation2009a).
compares the new partitioning data collected by this study to previously published data for this engine measured with a dilution sampler (Shrivastava et al. Citation2006) and chamber dilution experiments (Grieshop et al. Citation2009a). There is good agreement between the new data and the previous measurements, with minor differences at low C OA (<40 μg m−3). We attribute these minor differences to uncertainty in defining C tot. also includes the partitioning curve calculated from the volatility distribution derived by Grieshop et al Citation(2009a) using previously published data. While the overall agreement between the new data and this fit is good; at C OA < 40 μg m−3, the Grieshop et al. volatility distribution somewhat overpredicts the X p values compared to the new measurements. In other words, the Grieshop et al. Citation(2009a) volatility distribution predicts that the emissions are modestly less volatile than our new data suggests; this difference is discussed later in the manuscript. In a following section, we derive a new volatility distribution that incorporates all of the available data, including the previously published dilution tunnel and smog chamber data, along with the new data measured in this study at multiple temperatures.
Temperature Sensitivity of Gas–Particle Partitioning
To investigate the effects of temperature on partitioning, the experiment was repeated at different chamber temperatures. shows partitioning plots of data measured at three atmospherically relevant temperatures: 15°C, 26°C, and 33°C. As expected, decreasing the temperature shifted the gas–particle partitioning to the condensed phase (increased X p) at a given C OA.
FIG. 4 Partitioning data measured at different temperatures (15°C, 26°C, 33°C). Curves show predicted partitioning calculated from the basis set fit (fi and ΔH v(C*)) presented in .
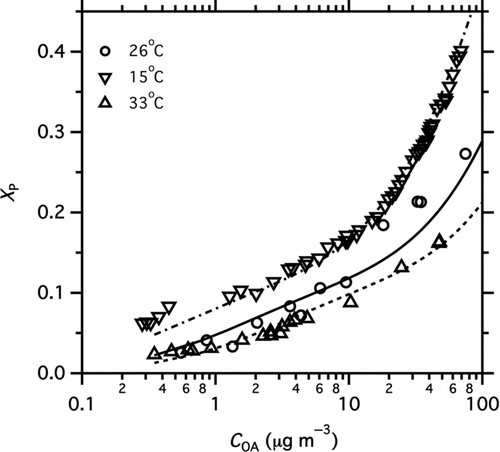
TABLE 1 Volatility distributions and ΔH v(C*) for the basis set and two-product model fits presented here, and the basis set fit from Grieshop et al. (2009a)
The average temperature sensitivity of the POA is −5.5% °C−1; it ranges from −3.5% °C−1 at 20 μg m−3 to −17.3% °C−1 at 1 μg m−3. The temperature sensitivity at 20 μg m−3 is similar to results from previous studies (Stanier et al. Citation2007; Grieshop et al. Citation2009a), but it is somewhat higher in the lower concentration range.
Fits to Absorptive Partitioning Theory
All of the data shown in and were fit simultaneously to determine the volatility distribution and enthalpies of vaporization for diesel exhaust. Two fits were performed: a 6-bin basis set fit and a two-product fit. The basis set fit is indicated by the lines in ; the two-product fit is shown in Figure S1. Both fits describe the data with high fidelity as indicated by the value obtained for the objective function (Equation (5)). The new parameterizations (fi 's and ΔH vap), along with the volatility distribution of Grieshop et al Citation(2009a), are listed in .
Since the experimental data at 15°C and 33°C were collected up to C OA ∼ 100 μg m−3, we were only able to constrain C* bins up to 103 μg m−3. C* = 10−2 μg m−3 was chosen as the lower limit. There is certainly organic material present with C* > 103 μg m−3, and perhaps material with C* < 10−2 μg m−3. However, the material in these C* bins cannot be constrained by our experimental data. Fortunately, the mass in these bins has little overall impact on X p over the atmospherically relevant range of C OA. Material in bins less volatile than C* = 10−2 μg m−3 will always exist in the condensed phase at ambient temperatures and C OA values. This material should be included in the C* = 10−2 μg m−3 bin in our fits. Material in bins more volatile than 103 μg m−3 exist almost exclusively in the vapor phase under ambient conditions. Even at the highest concentrations inside the chamber (C OA ∼ 103 μg m−3, an order of magnitude higher than very high atmospheric concentrations), organic material in the C* = 104 μg m−3 bin will only have 10% of its mass in the condensed phase.
The constrained best-fit ΔH v(C*) for the basis set parameterization was ΔH v(C*) = −11log(C*298 K) + 85 kJ mol−1. This slope is at the upper bound of the range considered by the fitting algorithm. Relaxing this constraint reveals that the absolute minimum of Equation (5) corresponds to somewhat steeper slopes (values less than −11), but that assigns very small and even negative ΔH v values to high C* bins. Therefore, these solutions were rejected as not physically realistic. The best-fit ΔH v(C*) for the two-product model was a constant ΔH v = 72 kJ mol−1. These values encompass the range of ΔH v values for individual compounds found in POA (Bilde et al. Citation2003; Cappa et al. Citation2007; Epstein et al. Citation2010). For example, C* values of high molecular weight n-alkanes, which are found in diesel exhaust, range from 61.5 kJ mol−1 for n-dodecane (C* ∼ 106μg m−3) (Majer and Svoboda Citation1985) to 80.8 kJ mol−1 for n-eicosane (C* ∼ 103 μg m−3) (Chirico et al. Citation1989) and 99.3 kJ mol−1 for n-octacosane (C* ∼ 101 μg m−3) (Chirico et al. Citation1989).
A number of other studies have also derived ΔH v values using thermodynamic data for individual compounds (Donahue et al. Citation2006; Grieshop et al. Citation2009a; Epstein et al. Citation2010) or by fitting gas–particle partitioning data (Offenberg et al. Citation2006; Pathak et al. Citation2007; Stanier et al. Citation2007). However, one cannot directly compare the different ΔH v values because the fitting procedure simultaneously determines both fi and ΔH v(C*). Therefore, the ΔH v values from this work (and other studies that fit data) are semiempirical. One cannot arbitrarily mix and match volatility distributions with ΔH v values from different studies.
Comparisons with Thermodenuder Data
Grieshop et al. Citation(2009a) characterized the volatility of the POA emissions from this engine using a thermodenuder. compares that thermodenuder data to predictions based on both of the new fits (basis set and two product) derived here. The thermodenuder data were not used to derive the volatility distributions presented above; therefore, it provides a strong test of the ability of the new fits to reproduce gas–particle partitioning data. In addition, the thermodenuder data were collected under different conditions (especially, temperature) so it tests the ability of the fits to extrapolate over a wider range of conditions.
FIG. 5 Comparison of thermodenuder data from Grieshop et al. Citation(2009a) to predictions based on volatility distributions derived in this study. Mass fraction remaining (MFR) is the fraction of POA that passes through the thermodenuder as a function of temperature. Volatility distributions and ΔH v(C*) values for the model predictions (curves) are listed in .
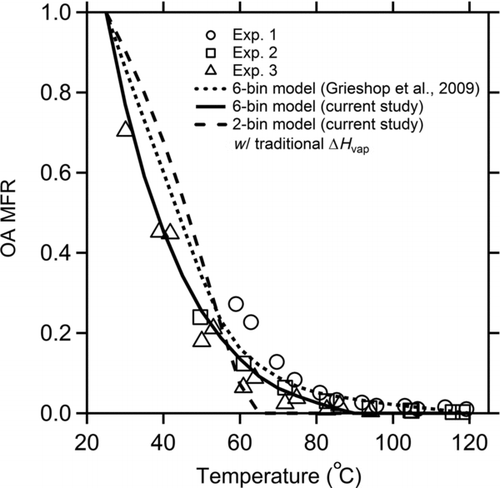
The thermodenuder data are presented as a thermogram- —POA mass fraction remaining (MFR) at different temperatures. The curves in were calculated using the model of Riipinen et al. Citation(2010) and the volatility distributions and ΔH v values listed in assuming an evaporation coefficient of 1. The model accounts for the dynamics of evaporation because equilibrium partitioning is not achieved in the thermodenuder.
The new basis set fit presented in , shown by the solid black line, passes right through the thermodenuder data. In comparison, the two-product fit does a relatively poor job of reproducing the thermodenuder data. It overpredicts the MFR for low temperatures and predicts that all of the OA evaporates by 65°C. Therefore, the basis set approach provides a more robust description of the gas–particle partitioning across a wider range of conditions than the two-product model. The thermodenuder data indicate that the two-product model is somewhat too volatile.
The new basis set fit even describes the thermodenuder data better than the fit of Grieshop et al. Citation(2009a). This seems surprising since the Grieshop et al. Citation(2009a) distribution was actually partially based on the thermodenuder data while the new fit was not. However, Grieshop et al. Citation(2009a) assumed that the aerosol reached equilibrium in the thermodenuder. If the aerosol does not reach equilibrium, then indicates that the Grieshop et al. Citation(2009a) distribution overestimates the amount of very low-volatility material in the emissions.
Atmospheric Implications
Current emission inventories and models assume that POA is nonvolatile, and that POA emission factors do not change with atmospheric dilution or temperature changes. Therefore, one goal of deriving volatility distributions for fresh emissions, such as diesel exhaust, is to enable models to account for the effects of temperature and ambient concentrations on POA emission factors (Robinson et al. Citation2010).
shows the predicted POA EF for the basis set fit across the entire atmospherically relevant range of C OA and temperature. The POA EF changes from 40 to 160 mg kg-fuel−1 when C OA concentrations vary between 1 and 20 μg m−3 and temperature varies between 0 and 30°C. These changes are only due to varying gas–particle partitioning, not varying emission rates. As expected, the POA emissions are highest at low-temperature, high-concentration conditions. The results in indicate that trying to represent the emissions from this engine with a traditional, static, nonvolatile POA EF will, in the best case (EF measured in the center of the atmospherically relevant space), create errors of at least a factor of 2 across the range of typical atmospheric conditions. Although this is a high-emitting engine, these sources also disproportionally contribute to urban pollution. The composition of the POA emissions from this engine is similar to that in the other engines (Grieshop et al. Citation2009a).
FIG. 6 (a) POA EF in mg kg-fuel−1 predicted by the basis set fit as a function of C OA and temperature. (b) Fractional difference (difference between two fits divided by mean expressed as a percent) between predicted ambient partitioning based on the basis set fit and two-product model shown in . The star indicates the point at which the emission factors are set to be equal to define C tot.
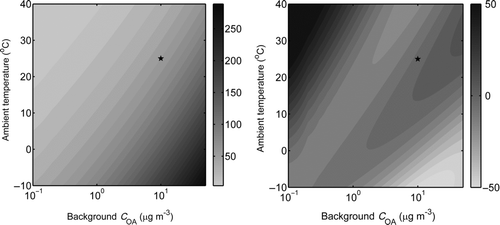
Although both the basis set and two-product fits accurately describe the experimental data ( and S1), raises concerns that the fits may diverge as one uses them to extrapolate away from the experimental data. This concern is examined in , which compares the POA emission factors predicted by the two fits across a wide range of atmospheric conditions. Across most of this range, the two predictions agree well, better than ±30%. Therefore, the wide variation shown in is not an artifact of a particular fit.
Figure S2 compares the basis set fit of Grieshop et al. Citation(2009a) to the new basis set fit derived here. As we have shown throughout this article, these two fits are similar, and both do a reasonable job of predicting the smog chamber and thermodenuder data. It is no surprise, therefore, that the two only exhibit minor differences (∼10%) at typical ambient conditions of temperature and C OA. This underscores that there are multiple combinations of fi and ΔH v that describe the experimental data in – (Cappa and Jimenez Citation2010).
CONCLUSIONS
A new experimental technique has been developed to systematically investigate gas–particle partitioning of POA emissions from combustion systems across atmospherically relevant conditions. The technique involves adding small aliquots of exhaust to a chamber while a source is operated at constant load. The data demonstrate that increasing aerosol concentrations cause the gas–particle partitioning of semivolatile POA to shift toward the particle phase. This technique has been evaluated using previously published data. By starting at low concentrations, the new technique avoids the problems of high particle losses associated with the in-chamber dilution technique developed by Grieshop et al. Citation(2007, Citation2009a). By allowing the vapors and particles to reach equilibration, the new technique avoids the uncertainties associated with evaporation kinetics that complicate interpretation of thermodenuder data (An et al. Citation2007; Stanier et al. Citation2007; Cappa and Jimenez Citation2010; Saleh et al. Citation2010). Finally, the technique avoids the signal-to-noise problems associated with filter measurements made on dilution samplers.
The new technique has been used to study the temperature dependence of gas–particle partitioning. The overall temperature sensitivity of the POA was found to be—5.5% °C−1; it ranged from −17.3% °C−1 at 1 μg m−3 to −3.5% °C−1 at 20 μg m−3. This means that the diurnal temperature variations will have a larger effect on gas–particle partitioning at atmospherically relevant concentrations than at the higher concentrations used in typical source characterization experiments. The enthalpy of vaporization of the POA has been determined using a set of experiments performed at different temperatures. For a basis set fit to the data, a linear dependence of ΔH v with C* with a slope of −11 kJ mol−1 decade−1 and a ΔH v of the C* = 1 μg m−3 bin of 85 kJ mol−1 accurately describes the measured data. A two-product model with a constant ΔH v of 72 kJ mol−1 can also describe the data. These relationships can be used in chemical transport models to predict the effects of temperature on gas–particle partitioning of POA.
uast_a_602761_sup_20270519.zip
Download Zip (92.6 KB)Acknowledgments
[Supplementary materials are available for this article. Go to the publisher's online edition of Aerosol Science and Technology to view the free supplementary files.]
Funding was provided by the U.S. Department of Defense Strategic Environmental Research and Development Program (SERDP) as part of project WP-1626 and by the EPA STAR program through the National Center for Environmental Research (NCER) under grand R833748. The views, opinions, and/or findings contained in this paper are those of the authors and should not be construed as an official position of the funding agency.
REFERENCES
- Allan , J. D. , Delia , A. E. , Coe , H. , Bower , K. N. , Alfarra , M. R. , Jimenez , J. L. , Middlebrook , A. M. , Drewnick , F. , Onasch , T. B. , Canagaratna , M. R. , Jayne , J. T. and Worsnop , D. R. 2004 . A Generalised Method for the Extraction of Chemically Resolved Mass Spectra from Aerodyne Aerosol Mass Spectrometer Data . J. Aerosol Sci. , 35 : 909 – 922 .
- An , W. J. , Pathak , R. K. , Lee , B.-H. and Pandis , S. N. 2007 . Aerosol Volatility Measurement Using an Improved Thermodenuder: Application to Secondary Organic Aerosol . J. Aerosol Sci. , 38 : 305 – 314 .
- Bian , F. and Bowman , F. M. 2002 . Theoretical Model for Lumping Multicomponent Secondary Organic Aerosol Mixtures . Environ. Sci. Technol. , 36 : 2491 – 2497 .
- Bilde , M. , Svenningsson , B. , Monster , J. and Rosensorn , T. 2003 . Even–Odd Alternation of Evaporation Rates and Vapor Pressures of C3–C9 Dicarboxylic Acid Aerosols . Environ. Sci. Technol , 37 : 1371 – 1378 .
- Canagaratna , M. R. , Jayne , J. T. , Jimenez , J. L. , Allan , J. D. , Alfarra , M. R. , Zhang , Q. , Onasch , T. B. , Drewnick , F. , Coe , H. , Middlebrook , A. , Delia , A. , Williams , L. R. , Trimborn , A. M. , Northway , M. J. , DeCarlo , P. F. , Kold , C. E. , Davidovits , P. and Worsnop , D. R. 2007 . Chemical and Microphysical Characterization of Ambient Aerosols with the Aerodyne Aerosol Mass Spectrometer . Mass Spectrom. Rev. , 26 : 185 – 222 .
- Cappa , C. D. and Jimenez , J. L. 2010 . Quantitative Estimates of the Volatility of Ambient Organic Aerosol . Atmos. Chem. Phys. , 10 : 5409 – 5424 .
- Cappa , C. D. , Lovejoy , E. R. and Ravishankara , A. R. 2007 . Determination of Evaporation Rates and Vapor Pressures of Very Low Volatility Compounds: A Study of the C-4, C-10, and C-12 Dicarboxylic Acids . J. Phys. Chem. A, , 111 : 3099 – 3109 .
- Carlton , A. G. , Bhave , P. V. , Napelenok , S. L. , Edney , E. O. , Sarwar , G. , Pinder , R. W. , Pouliot , G. A. and Houyoux , M. 2010 . Model Representation of Secondary Organic Aerosol in CMAQv4.7 . Environ. Sci. Technol. , 44 : 8553 – 8560 .
- Chirico , R. D. , Nguyen , A. , Steele , W. V. and Strube , M. M. 1989 . “ Vapor Pressure of n-Alkanes Revisited. New High-Precision Vapor Pressure Data on n-Decane, n-Eicosane, and n-Octacosane ” . In J. Chem. Eng. Data Vol. 34 , 149 – 156 .
- Chung , S. H. and Seinfeld , J. H. 2002 . Global Distribution and Climate Forcing of Carbonaceous Aerosols . J. Geophys. Res. Atmos. , 107 : 4407 doi: 10.1029/2001JD001397
- Donahue , N. M. , Robinson , A. L. , Stanier , C. O. and Pandis , S. N. 2006 . Coupled Partitioning, Dilution, and Chemical Aging of Semivolatile Organics . Environ. Sci. Technol. , 40 : 2635 – 2643 .
- Epstein , S. A. , Riipinen , I. and Donahue , N. M. 2010 . A Semiempirical Correlation Between Enthalpy of Vaporization and Saturation Concentration for Organic Aerosol . Environ. Sci. Technol. , 44 : 743 – 748 .
- Grieshop , A. P. , Donahue , N. M. and Robinson , A. L. 2007 . Is the Gas–Particle Partitioning in Alpha-Pinene Secondary Organic Aerosol Reversible? . Geophys. Res. Lett. , 34 : L14810 doi: 10.1029/2007GL029987
- Grieshop , A. P. , Donahue , N. M. and Robinson , A. L. 2009b . Laboratory Investigation of Photochemical Oxidation of Organic Aerosol from Wood Fires—Part 2: Analysis of Aerosol Mass Spectrometer Data . Atmos. Chem. Phys. , 9 : 2227 – 2240 .
- Grieshop , A. P. , Logue , J. M. , Donahue , N. M. and Robinson , A. L. 2009c . Laboratory Investigation of Photocehmical Oxidation of Organic Aerosol from Wood Fires—Part 1: Measurement and Simulation of Organic Aerosol Evolution . Atmos. Chem. Phys. , 9 : 1263 – 1277 .
- Grieshop , A. P. , Miracolo , M. A. , Donahue , N. M. and Robinson , A. L. 2009a . Constraining the Volatility Distribution and Gas-Particle Partitioning of Combustion Aerosols Using Isothermal Dilution and Thermodenuder Measurements . Environ. Sci. Technol. , 43 : 4750 – 4756 .
- Griffin , R. J. , Cocker , D. R. , Flagan , R. C. and Seinfeld , J. H. 1999 . Organic Aerosol Formation from the Oxidation of Biogenic Hydrocarbons . J. Geophys. Res. Atmos. , 104 : 3555 – 3567 .
- Hildemann , L. M. , Mazurek , M. A. , Cass , G. R. and Simoneit , B. R. T. 1991 . Quantitative Characterization of Urban Sources of Organic Aerosol by High-Resolution Gas Chromatography . Environ. Sci. Technol. , 25 : 1311 – 1325 .
- Huffman , J. A. , Docherty , K. S. , Aiken , A. C. , Cubison , M. J. , Ulbrich , I. M. , DeCarlo , P. F. , Sueper , D. , Jayne , J. T. , Worsnop , D. R. , Ziemann , P. J. and Jimenez , J. L. 2009 . Chemically-Resolved Aerosol Volatility Measurements from Two Megacity Field Studies . Atmos. Chem. Phys. , 9 : 7161 – 7182 .
- Huffman , J. A. , Ziemann , P. J. , Jayne , J. T. , Worsnop , D. R. and Jimenez , J. L. 2008 . Development and Characterization of a Fast-Stepping/Scanning Thermodenuder for Chemically-Resolved Aerosol Volatility Measurements . Aerosol Sci. Technol. , 42 : 395 – 407 .
- Jayne , J. T. , Leard , D. C. , Zhang , X. F. , Davidovits , P. , Smith , K. A. , Kolb , C. E. and Worsnop , D. R. 2000 . Development of an Aerosol Mass Spectrometer for Size and Composition Analysis of Submicron Particles . Aerosol Sci. Technol. , 33 : 49 – 70 .
- Kalberer , M. , Yu , J. , Cocker , D. R. , Flagan , R. C. and Seinfeld , J. H. 2000 . Aerosol Formation in the Cyclohexene–Ozone System . Environ. Sci. Technol. , 34 : 4894 – 4901 .
- Kirchstetter , T. W. and Novakov , T. 2007 . Controlled Generation of Black Carbon Particles from a Diffusion Flame and Applications in Evaluating Black Carbon Measurement Methods . Atmos. Environ. , 41 : 1874 – 1888 .
- Lipsky , E. M. and Robinson , A. L. 2005 . Design and Evaluation of a Portable Dilution Sampling System and the Effects of Residence Time on Mass Emission Rates . Aerosol Sci. Technol. , 39 : 542 – 553 .
- Lipsky , E. M. and Robinson , A. L. 2006 . Effects of Dilution on Fine Particle Mass and Partitioning of Semivolatile Organics in Diesel Exhaust and Wood Smoke . Environ. Sci. Technol. , 40 : 155 – 162 .
- Majer , V. and Svoboda , V. 1985 . Enthalpies of Vaporization of Organic Compounds: A Critical Review and Data Compilation , Oxford : Blackwell Scientific Publications .
- Odum , J. R. , Hoffman , T. , Bowman , F. M. , Collins , D. , Flagan , R. C. and Seinfeld , J. H. 1996 . Gas/Particle Partitioning and Secondary Organic Aerosol Yields . Environ. Sci. Technol. , 30 : 2580 – 2585 .
- Offenberg , J. H. , Kleindienst , T. E. , Jaoui , M. , Lewandowski , M. and Edney , E. O. 2006 . Thermal Properties of Secondary Organic Aerosol . Geophys. Res. Lett. , 33 : L03816
- Pankow , J. F. 1994a . An Absorption-Model of the Gas Aerosol Partitioning Involved in the Formation of Secondary Organic Aerosol . Atmos. Environ. , 28 : 189 – 193 .
- Pankow , J. F. 1994b . An Absorption-Model Gas–Particle Partitioning of Organic Compounds in the Atmosphere . Atmos. Environ. , 28 : 185 – 188 .
- Pathak , R. K. , Presto , A. A. , Lane , T. E. , Stanier , C. O. , Donahue , N. M. and Pandis , S. N. 2007 . Ozonolysis of Alpha-Pinene: Parameterization of Secondary Organic Aerosol Mass Fraction . Atmos. Chem. Phys. , 7 : 3811 – 3821 .
- Pye , H. O. T. and Seinfeld , J. H. 2010 . A Global Perspective on Aerosol from Low-Volatility Organic Compounds . Atmos. Chem. Phys. , 10 : 4377 – 4401 .
- Riipinen , I. , Pierce , J. R. , Donahue , N. M. and Pandis , S. N. 2010 . Equilibration Times of Organic Aerosol Inside Thermodenuders: Evaporation Kinetics Versus Thermodynamics . Atmos. Environ. , 44 : 597 – 607 .
- Robinson , A. L. , Donahue , N. M. , Shrivastava , M. K. , Weitkamp , E. A. , Sage , A. M. , Grieshop , A. P. , Lane , T. E. , Pierce , J. R. and Pandis , S. N. 2007 . Rethinking Organic Aerosols: Semivolatile Emissions and Photochemical Aging . Science , 315 : 1259 – 1262 .
- Robinson , A. L. , Grieshop , A. P. , Donahue , N. M. and Hunt , S. W. 2010 . Updating the Conceptual Model for Fine Particle Mass Emissions from Combustion Systems . J. Air Waste Manage. Assoc. , 60 : 1204 – 1222 .
- Russell , L. M. 2003 . Aerosol Organic-Mass-to-Organic-Carbon Ratio Measurements . Environ. Sci. Technol. , 37 : 2982 – 2987 .
- Saleh , R. , Shihadeh , A. and Khylstov , A. Y. 2011 . On Transport Phenomena and Equilibration Time Scales in Thermodenuders . Atmos. Meas. Tech. , 4 : 571 – 581 .
- Schauer , J. J. , Kleeman , M. J. , Cass , G. R. and Simoneit , B. R. T. 1999 . Measurement of Emissions from Air Pollution Sources. 2. C1 Through C30 Organic Compounds from Medium Duty Diesel Trucks . Environ. Sci. Technol. , 33 : 1578 – 1587 .
- Shrivastava , M. K. , Lipsky , E. M. , Stanier , C. O. and Robinson , A. L. 2006 . Modeling Semivolatile Organic Aerosol Mass Emissions from Combustion Systems . Environ. Sci. Technol. , 40 : 2671 – 2677 .
- Stanier , C. O. , Pathak , R. K. and Pandis , S. N. 2007 . Measurement of the Volatility of Aerosol from Alpha-Pinene Ozonolysis . Environ. Sci. Technol. , 41 : 2756 – 2763 .
- Tsigaridis , K. and Kanakidou , M. 2003 . Global Modelling of Secondary Organic Aerosol in the Troposphere: A Sensitivity Analysis . Atmos. Chem. Phys. , 3 : 1849 – 1869 .
- Weitkamp , E. A. , Sage , A. M. , Donahue , N. M. and Robinson , A. L. 2007 . Organic Aerosol Formation from Photochemical Oxidation of Diesel Exhaust in a Smog Chamber . Environ. Sci. Technol. , 41 : 6969 – 6975 .