ABSTRACT
In the present study, the error estimation of Taylor series expansion method of moment (TEMOM) for particle population balance equation due to Brownian coagulation is proposed. The app-roximation introduced by Taylor series polynomials has two parts: one is the approximation of nonlinear collision kernel, and the other is the approximation of higher and fractional particle moment. The results show that the two kinds of errors have the same order in the original TEMOM model, which is related to the third derivative of collision kernel and the first four integer order particle moments, i.e., M0, M1, M2, and M3. Based on the lognormal size distribution assumption, the system errors are obtained for different TEMOM models due to Brownian coagulation; and all the errors are small and acceptable. The results have confirmed the validity of TEMOM model.
Copyright © 2016 American Association for Aerosol Research
EDITOR:
1. Introduction
In terms of continuous variables, the population balance equation (PBE) due to Brownian coagulation is written as[1] in which n(v,t)dv is the number of particles per unit spatial volume with particle volume from v to v + dv at time t; and β is the collision frequency function of coagulation. In theory, there exists a unique solution for chosen kernel function. However, the solution of PBE can only be solved for simple kernel functions, known as the constant (β ∼ 1), additive (β ∼ vi + vj), and multiplicative (β ∼ vivj) kernels, respectively. In most practical applications, the kernel takes on a significantly more complex form. For example, the free molecule kernel describes collision in a dilute gas-phase system as
[2] in which B1 = (3/4π)1/6(6kBT/ρp)1/2, and kB is the Boltzmann constant, T is the temperature; and ρp is the particle density. Generally, the coagulations that result from such physically realistic kernels are not solvable, and it is necessary to appeal to numerical method. The principal methods are the deterministic method of moment, sectional method, and the stochastic Monte Carlo method. Because of the relative simplicity of implementation and low computational costs, the moment method has become a powerful tool for investigating aerosol microphysical processes for many applications (Pratsinis Citation1988).
The kth-order moment Mk of particle size distribution is defined as follows:[3]
By multiplying both sides of PBE, Equation (Equation1[1] ), with vk and integrating over all particle sizes, a system of transport equations for Mk is obtained. In a spatially homogeneous system, the particle moments evolve in time due to the Brownian coagulation and can be expressed as follows:
[4]
Recently, Yu et al. (Citation2008) have proposed a moment-based approach, termed as the Taylor series expansion method of moment (TEMOM), to approximate PBE. This approach makes no prior assumption on the shape of particle size distribution; the derivation of TEMOM is completely based on Taylor series expansion; and the form of TEMOM is relatively simple. Therefore, TEMOM not only has advantages in the speed and accuracy of computation, it also provides a new way to analyze the characteristic of PBE theoretically. Based on the TEMOM model, Xie and Wang (Citation2013) and Xie (Citation2014, Citation2015) provide a series of asymptotic solutions of PBE for Brownian coagulation and agglomeration. Some analytical solutions of PBE are also proposed in the previous work, e.g. solution of PBE for Brownian coagulation in free molecule and continuum regime (Xie and He Citation2013; Yu et al. Citation2015a,b), solution of PBE for Brownian agglomeration in continuum regime (He et al. Citation2015; Yu et al. Citation2016).
It should be pointed out that TEMOM corresponds to a general perturbation theory approach, which is well known in the theoretical physics and requires very careful justification in each case. In the present study, as a basic problem of perturbation theory, the truncated error of TEMOM is estimated.
2. Error estimation
In mathematics, a Taylor series is a representation of a function as an infinite sum of terms that are calculated from the values of the function's derivatives at an expansion point (in TEMOM, the expansion point u = M1/M0 is the mean particle volume). In the derivation of the TEMOM model, Taylor series approximations are introduced twice, one is the approximation of nonlinear collision kernel function, and the other is the approximation of higher and fractional order moment.
2.1. The truncation error for the approximation of collision kernel
Without loss of generality, the Taylor series approximation for the collision kernel with bivariate can be expressed as follows:[5] where TP is the Taylor series polynomial, then its truncation error in the original TEMOM model of degree 2 (Yu et al. Citation2008) is
[6]
Taking the nonlinear collision kernel in the free molecule regime as example, difference between the collision kernel and its Taylor series approximation is shown in . It can be found that the maximum error occurs at the margin of the particle volume domain (vi > 0, vj > 0). In the contour plot of the relative error (error 1/β), all the contour lines are straight and perpendicular to the line vi = vj in the Cartesian coordinate system. Therefore, it is reasonable to simplify the truncation error estimation of collision kernel with bivariate into the ones with mono-variant. For a free molecule kernel, it is simplified as β ∼ v1/6. With the increasing number of Taylor series, the Taylor series polynomials are infinitely close to the original function in the region v > 0, which is shown in . This result reveals that the convergence of Taylor series is obvious, and the truncation error can be reduced as[7]
Figure 1. Difference between nonlinear collision kernel and its Taylor series approximations of degree 2 at expansion point u (particle mean volume): (a) the absolute error of collision kernel; (b) the contour plot for relative error of collision kernel.
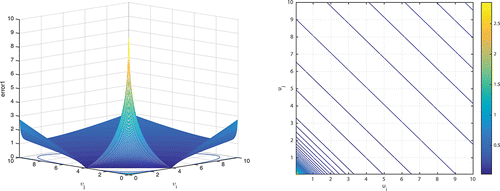
Figure 2. As the degree of Taylor polynomial rises, it approaches the correct functions; this image shows v1/6 and its Taylor approximations at the expansion point u (particle mean volume), polynomials of degrees 3, 5, 7, 9, 11, and 13.
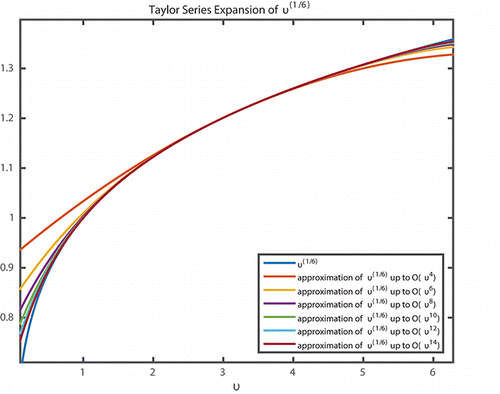
The truncation error is related to the third derivative of collision kernel and the first four integer order particle moments, i.e., M0, M1, M2, and M3.
2.2. The truncation error for the approximation of higher and fractional order moment
In the closure of higher and fractional order particle moment, vk can be expanded as follows:[9] and its corresponding moment is
[10]
Analogously, its truncation error in the original TEMOM model of degree 2 (Yu et al. Citation2008) is[11] where L2 is the maximum of the third derivative of vk in the range of particle volume v > 0. The truncation error of the approximation of higher and fraction order moment has the same order as that of the approximation of collision kernel.
To calculate the error of kth order particle moment, the particle size distribution (PSD) should be given in advance. Without loss of generality, assuming that PSD is a lognormal function,[12] in which the geometric average particle volume vg and standard deviation σ can be expressed in terms of the first three moments of the lognormal size distribution:
[13] where MC = M0M2/M12 is the dimensionless particle moment.
The comparison of Mk calculated by the first three integer order particle moment based on Equation (Equation10[10] ) with that based on Equation (Equation3
[3] ) is shown in . In the original TEMOM model (Yu et al. Citation2008), the maximum and minimum of k is 19/6 and –1/2, respectively. Generally, the values of Mk calculated by the two kinds of methods are in good agreement. shows the effect of parameters of lognormal size distribution on the relative error of Mk, which is defined as
[14]
Figure 3. Comparison of Mk based on Equation (Equation10[10] ) with that based on Equation (Equation3
[3] ) in terms of lognormal size distribution. The geometric average particle volume and standard deviation are vg = 0.1 and σ = 1.3445 (or MC = 2.2001), respectively.
![Figure 3. Comparison of Mk based on Equation (Equation10[10] ) with that based on Equation (Equation3[3] ) in terms of lognormal size distribution. The geometric average particle volume and standard deviation are vg = 0.1 and σ = 1.3445 (or MC = 2.2001), respectively.](/cms/asset/ff12d2a5-2320-4e09-a009-08c86abb2640/uast_a_1207058_f0003_oc.gif)
Figure 4. Effect of parameters of lognormal size distribution on the error of Mk. (a) Effect of geometric average particle volume on the error of of Mk with σ = 1.3445 (or MC = 2.2201); (b) effect of standard deviation on the error of Mk with vg = 0.1.
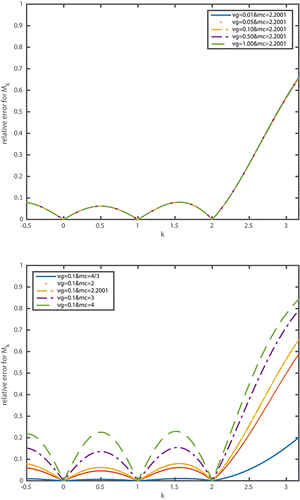
The relative error increases rapidly with the increase of k (k > 2). The results also show that the geometric average particle volume has a little influence on the error; but the effect of standard deviation on the error is significant, the greater the standard deviation, the greater the relative error. The reason can be found in the formula rearranged from Equation (Equation10[10] ) as
[15a] In addition, based on the assumption of lognormal size distribution, there is another approximation of Mk as (Pratsinis Citation1988)
[15b]
However, this method is only valid for smaller geometric average particle volume and standard deviation; for larger ones, the error is as large as that of TEMOM. It should be pointed out that the first three integer order moments (i.e., M0, M1, and M2) are calculated accurately.
2.3. System error of TEMOM model
Based on the above analysis, the system error of TEMOM model is[16] where L is a constant, which can be regarded as the maximum of the third derivative of
(Chen et al. Citation2014; Yu et al. Citation2015c). Since M0, M1, M2, and u are calculated accurately, the key point of error estimation is how to calculate the third order particle moment M3 and the third derivative of collision kernel. In the original TEMOM model, the third order particle moment is approximated with Equation (Equation15a
[15a] ) as follows:
[17]
Substituting it into Equation (Equation10[10] ), the calculation of Mk can be obtained as
[18]
which is a recursive iteration process. Therefore, the value of M3 and its error estimation should be obtained in another way. The best way to calculate M3 accurately is the definition of particle moment as Equation (Equation3[3] ). In the next section, the error estimation will be discussed with concrete examples.
3. Results and discussions
3.1. The error for simple kernels
For the constant, additive, and multiplicative kernels, the maximum of the three-order derivative of collision kernel is zero (L1 = 0); moreover, there is no need to close higher and fractional order moment with Taylor series polynomials. Therefore, the TEMOM is just the moment transformation of PBE, and their errors are all zero.
For a constant kernel (β ∼ 1), its TEMOM models are[19]
For additive kernel (β ∼ vi + vj), its TEMOM models are[20]
For multiplicative kernel (β ∼ vivj), its TEMOM models are[21]
These results are consistent with the previous analysis (Wattis Citation2006).
3.2. The error for Brownian agglomeration in the continuum regime
For the collision kernel in continuum regime,[22] where the constant B2 = 3kBT/2μ, and μ is the gas viscosity. The moment equation based on Equation (Equation4
[4] ) without approximation is
[23]
In this case, only the approximation of fractional order moment is needed to obtain the corresponding TEMOM (He et al. Citation2015) as[24]
The relative error defined as in Equation (Equation14[14] ) for M–aMa and M1–aM1+a in terms of lognormal size distribution with vg = 0.1 and MC = 2 is show in ; and their maximums are the same as 1.65%.
3.3. The error for Brownian coagulation in the free molecule regime
Similarly, the moment equation for Brownian coagulation in the free molecule regime without approximation is[25]
The growth rates of M0 and M2 (right-hand side of Equation (Equation25[25] )) are double integrals over the domain. Its corresponding TEMOM model is
[26]
In terms of lognormal size distribution with vg = 0.1 and σ = 1.3445 (or MC = 2.2001), the relative errors of growth rate for M0 and M2 are 2.65% and 0.98%, respectively. Due to the wide range of particle size (in this article, the particle volume ranges from 10−4 to 102 to obtain a more accurate error estimate), the double integrals for growth rates of M0 and M2 have high cost and low efficiency.
In previous studies (Xie and He Citation2014; Yu et al. Citation2015c), it has been revealed that different approximations of collision kernel using the same order of Taylor series and the same expansion point are almost equivalent. Therefore, the error of collision kernel expanded in part in the original TEMOM (Yu et al. Citation2008) is equivalent to that of the collision kernel expanded as a whole (Chen et al. 2014). Moreover, as mentioned in Section 2.1, the error estimation of collision kernel with bivariate can be simplified as the ones with mono-variant. Therefore, the system error of the TEMOM model can be approximated by the global error of collision kernel, and the influence of parameters of particle size distribution on the system error is reduced to influence of parameters on particle moment; this problem has been discussed in Section 2.2. In a free molecule regime, the relative errors of growth rates for M0 and M2 of the TEMOM model can be reduced to the ones of M1/6 and M7/6, which are 3.17% and 3.42%, respectively. From the point of view of errors, the original TEMOM (Yu et al. Citation2008) is more reasonable.
4. Conclusions
In the present study, the error estimation of TEMOM for population balance equation due to Brownian coagulation is proposed. The results show that the errors introduced by the approximation of nonlinear collision kernel and higher and fractional order particle moment have the same order as in the original TEMOM model, which is related to the first four integer order particle moments, i.e., M0, M1, M2, and M3. Based on the lognormal size distribution assumption, the system errors are obtained for different TEMOM models due to Brownian coagulation. For population balance equation with simple kernels, such as constant, additive, and multiplicative kernels, the system errors of TEMOM are zero; for the TEMOM model in the continuum regime, the relative errors of growth rates for M0 and M2 are the same; for the TEMOM model in the free molecule regime, the relative errors of growth rates for M0 and M2 are of little difference, but the errors are small and acceptable. The error estimations show that TEMOM is a promising tool to investigate particle population balance equation.
Funding
This work was supported by the National Natural Science Foundation of China with Grant Nos. 50806023 and 11572138.
References
- Chen, Z. L., Lin, J. Z., and Yu, M. Z. (2014). A Direct Expansion Method of Moments for Brownian Coagulation. Phys. Scripta, 89:125204.
- He, Q., Shchekin, A. K., and Xie, M. L. (2015). New Analytical TEMOM Solutions for a Class of Collision Kernels in the Theory of Brownian Coagulation. Physica A, 428:435–442.
- Pratsinis, S. E. (1988). Simultaneous Nucleation, Condensation, and Coagulation in Aerosol Reactor. J. Colloid Interface Sci., 124:416–427.
- Wattis, J. A. D. (2006). An Introduction to Mathematical Models of Coagulation-Fragmentation Processes: A Discrete Deterministic Mean-Field Approach. Physica D, 222:1–20.
- Xie, M. L., and Wang, L. P. (2013). Asymptotic Solution of Population Balance Equation Based on TEMOM Model. Chem. Eng. Sci., 94:79–83.
- Xie, M. L., and He, Q. (2013). Analytical Solution of TEMOM Model for Particle Population Balance Equation Due to Brownian Coagulation. J. Aerosol Sci., 66:24–30.
- Xie, M. L., and He, Q. (2014). The Fundamental Aspects of TEMOM Model for Particle Coagulation Due to Brownian Motion. Part I: In the Free Molecule Regimes. Int. J. Heat Mass Transfer, 70:1115–1120.
- Xie, M. L. (2014). Asymptotic Behavior of TEMOM Model for Particle Population Balance Equation Over the Entire Particle Size Regime. J. Aerosol Sci., 67:157–165.
- Xie, M. L. (2015). Asymptotic Solution of Moment Approximation of the Particle Population Balance Equation for Brownian Agglomeration. Aerosol Sci. Technol., 49:109–114.
- Yu, M. Z., Lin, J. Z., and Chan, T. L. (2008). A New Moment Method for Solving the Coagulation Equation for Particles in Brownian Motion. Aerosol Sci. Technol., 42:705–713.
- Yu, M. Z., Lin, J. Z., Cao, J. J., and Seipenbusch, M. (2015a). An Analytical Solution for the Population Balance Equation Suing a Moment Method. Particuology, 18:194–200.
- Yu, M. Z., Zhang, X. T., Jin, G. D., Lin, J. Z., and Seipenbusch, M. (2015b). A New Analytical Solution for Solving the Population Balance Equation in the Continuum Slip Regime. J. Aerosol Sci., 80:1–10.
- Yu, M. Z., Liu, Y. Y., Lin, J. Z., and Seipenbusch, M. (2015c). Generalized TEMOM Scheme for Solving the Population Balance Equation. Aerosol Sci. Technol., 49:1021–1036.
- Yu, M. Z., Liu, Y. Y., Jin, G. D., and Jin, H. H. (2016). A New Analytical Solution for Agglomerate Growth Undergoing Brownian Coagulation. Appl. Math. Model., 40:5497–5509.