ABSTRACT
Dimethylamine (DMA) has a stabilizing effect on sulfuric acid (SA) clusters, and the SA and DMA molecules and clusters likely play important roles in both aerosol particle formation and growth in the atmosphere. We use the monodisperse particle growth model for acid-base chemistry in nanoparticle growth (MABNAG) together with direct and indirect observations from the CLOUD4 and CLOUD7 experiments in the cosmics leaving outdoor droplets (CLOUD) chamber at CERN to investigate the size and composition evolution of freshly formed particles consisting of SA, DMA, and water as they grow to 20 nm in dry diameter. Hygroscopic growth factors are measured using a nano-hygroscopicity tandem differential mobility analyzer (nano-HTDMA), which combined with simulations of particle water uptake using the thermodynamic extended-aerosol inorganics model (E-AIM) constrain the chemical composition. MABNAG predicts a particle-phase ratio between DMA and SA molecules of 1.1–1.3 for a 2 nm particle and DMA gas-phase mixing ratios between 3.5 and 80 pptv. These ratios agree well with observations by an atmospheric-pressure interface time-of-flight (APi-TOF) mass spectrometer. Simulations with MABNAG, direct observations of the composition of clusters <2 nm, and indirect observations of the particle composition indicate that the acidity of the nucleated particles decreases as they grow from ∼1 to 20 nm. However, MABNAG predicts less acidic particles than suggested by the indirect estimates at 10 nm diameter using the nano-HTDMA measurements, and less acidic particles than observed by a thermal desorption chemical ionization mass spectrometer (TDCIMS) at 10–30 nm. Possible explanations for these discrepancies are discussed.
Copyright © 2016 American Association for Aerosol Research
EDITOR:
1. Introduction
Atmospheric aerosol particles affect Earth's energy balance directly by scattering and absorbing solar radiation, and indirectly when being activated as cloud condensation nuclei (CCN) or ice nuclei (IN) in clouds, thereby modifying cloud radiative properties. According to the Intergovernmental Panel on Climate Change (IPCC), the radiative forcing associated with the indirect effect of anthropogenic aerosols has the largest uncertainty of all forcing mechanisms for the industrial period (IPCC Citation2013).
Aerosol particles can form directly in the atmosphere by nucleation and may thereafter grow by condensation of vapors with low enough volatility to undergo gas-to-particle conversion, or by the condensation of semi-volatile species that subsequently react in particles to form low volatility compounds. Particles reaching diameters larger than 40–120 nm may activate as CCN, depending on their physico-chemical properties and the ambient water supersaturation (Dusek et al. Citation2006). High condensational growth rates enhance the climatic impact of the nucleated particles, because coagulation acts to reduce the number concentration of the particles while they grow. Although highly uncertain, model simulations suggest that almost half of the global CCN (activated at 0.2% supersaturation) could originate from nucleation of aerosol particles (Merikanto et al. Citation2007). It should be noted, however, that this number is sensitive to what is assumed about the growth of newly formed particles.
Formation of aerosol particles in many places has been observed to be strongly dependent on the gas phase sulfuric acid (SA) concentration (Weber et al. Citation1995, Citation1997). Formation rates predicted by binary SA-water nucleation theory (Kulmala et al. Citation1998; Vehkamaki et al., Citation2002) are generally several orders of magnitude too low to explain formation rates observed in the atmospheric boundary layer (Spracklen et al. Citation2005). However, particle formation involving SA is enhanced by the presence of basic compounds such as ammonia (Ball et al. Citation1999), which have a stabilizing effect on the SA clusters. Kirkby et al. (Citation2011) observed formation rates enhanced by up to a factor of 1000 when ammonia was present at 100 pptv compared to binary SA-water nucleation. Despite this large enhancement due to the presence of ammonia, the authors concluded that atmospheric concentrations of ammonia and SA are too low to account for observed boundary layer new particle formation rates.
Similar to ammonia, amines are basic compounds that have a stabilizing effect on SA clusters and could therefore play an important role in new particle formation. Mäkelä et al. (Citation2001) observed in the boreal forest of Hyytiälä in Finland that aerosol particles were enriched with dimethylamine (DMA) during new particle formation events compared to periods with no events. Furthermore, during new particle formation events in Tecamac, Mexico, and in Hyytiälä, aminium ions accounted for 47% and 23%, respectively, of the positive ions in 8–10 nm particles, indicating that aminium salts may play important roles in both particle formation and early growth (Smith et al. Citation2010). Based on quantum chemical calculations, DMA is likely to be much more efficient than ammonia in stabilizing SA clusters (Kurtén et al. Citation2008; Loukonen et al. Citation2010). These results are in line with observations from the cosmics leaving outdoor droplets (CLOUD) chamber in CERN by Almeida et al. (Citation2013), who found that DMA above 3 pptv can enhance particle formation rates by more than a factor of 1000 compared with ammonia, resulting in formation rates similar to those observed in the atmospheric boundary layer. Furthermore, Jen et al. (Citation2014) observed that DMA is more efficient than methylamine and trimethylamine in helping the production of stable SA dimers. Similar observations were made by Yu et al. (Citation2012).
Ions are thought to enhance particle formation rates to some extent, because ions have a stabilizing effect on molecular clusters (Yu and Turco Citation2000). In the CLOUD chamber, ion concentrations similar to those occurring at ground level in the atmosphere enhanced the formation rates by a factor of between 2 and more than 10 when ammonia and sulfuric acid were present in the chamber at atmospherically relevant concentrations (Kirkby et al. Citation2011). However, during experiments focusing on SA-DMA nucleation, the ion-induced contribution was small, reflecting the high stability of electrically neutral SA-DMA clusters (Almeida et al. Citation2013). This finding is consistent with cluster population simulations using quantum-chemistry-based cluster evaporation rates predicting a minor contribution of ions to SA-DMA nucleation (Olenius et al. Citation2013). Measurements of charged clusters smaller than ∼2 nm inside the CLOUD chamber using an atmospheric-pressure interface time-of-flight (APi-TOF) mass spectrometer indicated that clusters containing three or more SA molecules had a steady-state SA to DMA molecular ratio of close to 1:1 during ion-induced SA-DMA nucleation (Almeida et al. Citation2013; Bianchi et al. Citation2014). Similar ratios were later reported for neutral clusters smaller than ∼2 nm based on measurements with a chemical ionization (CI)-APi-TOF (Kürten et al. Citation2014). These findings are consistent with quantum chemical calculations (Ortega et al. Citation2012, Citation2014).
Anthropogenic emissions of sulfur dioxide have decreased considerably during the last decades over Europe and North America, and are predicted to decrease globally during the next decades (Lamarque et al. Citation2011; Amann et al. Citation2012). Therefore, decreasing new particle formation has been predicted over the next decades over Europe (Ahlm et al. Citation2013) and globally (Makkonen et al. Citation2012). An important issue, however, is that amine scrubbing is likely to become the dominant technology for CO2 capture from fossil-fueled power plants (Nielsen et al. Citation2012). Therefore, amine emissions may increase in the future, which could have important implications for new particle formation with potential climate effects. This highlights the need for better understanding of the emissions, atmospheric processing, and losses of amines as well as their accurate representation in, e.g., air quality and climate models. Indeed, increasing effort has been made during recent years to improve the scientific understanding of sources, concentrations, and thermodynamic properties of atmospheric amines and their mixtures with common aerosol constituents (Ge et al. Citation2011a,Citationb).
Taking into consideration the experimental and theoretical results discussed above for SA-DMA nucleation, it is clear that DMA, and possibly also other amines, may play important roles in the formation of new particles in the atmosphere. However, the nucleated particles need to grow to CCN size before they can have a climate effect. Therefore, it is important to understand the SA-DMA system not only when it comes to nucleation and cluster growth, but also when it comes to evolution of particle composition and size during growth up to CCN size. The fact that instruments like the APi-TOF and CI-APi-TOF only detect clusters up to ∼2 nm makes thermodynamic modeling an important (and often the only) tool when estimating how the particle composition changes in the diameter range between 2 and 10 nm—especially since direct quantum chemical calculations for particles larger than ∼2 nm are also prohibitive (Henschel et al. Citation2014). For particles with diameters between 10 and 40 nm, only a few instruments are capable of chemical composition measurements at ambient levels in the atmosphere (Smith et al. Citation2004; Zordan et al. Citation2010). The goals of this study, focusing on the SA-DMA-water system, are to (1) investigate the evolution of size and composition of freshly formed particles as they grow from ∼2 nm up to more than 20 nm as predicted by state-of-the-art models combining thermodynamics and kinetics; (2) compare direct and indirect observations of the composition evolution to predictions by a thermodynamic particle growth model; and (3) investigate the roles of different kinetic and thermodynamic processes contributing to >4 nm nanoparticle growth in the CLOUD chamber.
2. Methods
2.1. Experimental
The experiments in this study were carried out during the CLOUD4 (June–July 2011) and CLOUD7 (October–December 2012) campaigns in the CLOUD chamber located at CERN (Kirkby et al. Citation2011; Almeida et al. Citation2013; Duplissy et al. Citation2016). Temperature and relative humidity in the chamber during the investigated experiments were 278 K and 38%, respectively. The CLOUD chamber can be exposed to a 3.5 GeV/c secondary pion (π+) beam from the CERN Proton Synchrotron providing the opportunity to investigate impacts of galactic cosmic rays (Duplissy et al. Citation2010). In addition, an electrical clearing field of 20 kV m−1 can be applied that effectively sweeps out all background ions produced by galactic cosmic rays.
A nano-hygroscopicity tandem differential mobility analyzer (nano-HTDMA) system was used to investigate hygroscopic growth of nanoparticles formed by ternary SA-DMA-water nucleation events (see Kim et al. Citation2016 for details). The hygroscopic growth factor (HGF) is here defined as the ratio of the particle diameter measured in the wet differential mobility analyzer (DMAwet) at ∼90% (±2%) RH to the diameter selected in the dry one (DMAdry) at 4.5 ± 2% RH. The HGFs were calculated for particles with dry diameters of 10, 15, or 20 nm for each experiment.
Direct measurements of the molecular composition for 10–30 nm volume median diameter particles were performed with a thermal desorption chemical ionization mass spectrometer (TDCIMS; Smith et al. Citation2004; Lawler et al. Citationin review). Ammonia and amines were quantified using positive ion detection with solvated hydronium reagent ion, and sulfate was quantified using hydrated superoxide reagent ion. The instrument was calibrated during the experiments by both direct addition of ammonium sulfate solution to the platinum wire as well as by sampling laboratory-generated ammonium sulfate nanoparticles. Calibrations were also performed to quantify instrument response to dimethylamine (Lawler et al. Citationin review).
An APi-TOF built by Tofwerk AG (Thun, Switzerland) and Aerodyne Research Inc. was used to measure the mass spectra for ion clusters smaller than ∼2.1 nm mobility diameter, corresponding to 3300 Th when using the procedure described by Ehn et al. (Citation2011) for conversion from mass measurements to mobility equivalent diameter of the ions. A description of the APi-TOF can be found in Junninen et al. (Citation2010), and the operation of the APi-TOF is described in detail by Schobesberger et al. (Citation2013). Note that no ionization of the sample was performed for these measurements, thus only ions charged in the CLOUD chamber were detected. Hence, the APi-TOF measurements were performed only when the electric clearing field was off with ion pairs being produced inside the CLOUD chamber.
Aerosol particle size distributions were measured with a nano-Scanning Mobility Particle Sizer (nano-SMPS). Aerosols were selected using a TSI type short differential mobility analyzer measuring from 4 to 80 nm. The particle concentration behind the mobility analyzer was measured with a modified TSI 3772 CPC with a cut-off of 5.6 nm and a detection threshold of 3.1 nm. Growth rates were determined based on the 50% rise of a size bin (Lehtipalo et al. Citation2016). The temporal evolution of a size bin was fitted with a least-square-fitted Gaussian peak (Lehtinen and Kulmala Citation2003; Kulmala et al. Citation2012) yielding the times when the concentration reached its maximum (mode) and 50% of the mode (the 50% rise time is equivalent to the full width at half maximum of the Gaussian peak). To calculate the growth rate, we plotted the diameters corresponding to the 50% rise time against time. Because the temporal evolution of the considered size showed a linear behavior, we applied a linear fit with the slope representing the growth rate. Calculating growth rates based on modal growth yielded similar growth rates.
The gas phase SA monomer concentration was measured with a chemical ionization mass spectrometer (CIMS; Kürten et al. Citation2011; Rondo et al. Citation2016). Gas phase dimethylamine and ammonia concentrations were measured with an ion chromatograph (Praplan et al. Citation2012).
2.2. Numerical models
2.2.1. Thermodynamic equilibrium modeling
The Extended-Aerosol Inorganics Model (E-AIM) (http://www.aim.env.uea.ac.uk; Clegg et al. Citation1992; Wexler and Clegg Citation2002; Clegg and Seinfeld Citation2006a,Citationb) was used to calculate the thermodynamic equilibrium between the gas and particle phases. No solid formation occurred in E-AIM for the investigated systems under the prevailing conditions, implying the relevant equilibrium to be that between the gas and aqueous phases.
The condensation and evaporation of a vapor depend on the equilibrium vapor pressure (peq), and thus on particle phase chemistry and particle size (Seinfeld and Pandis Citation2006):[1] where γi is the activity coefficient, Xi, and Xj are molar fractions of the condensing species i and the other compounds j in the particle, and psat,i is the saturation vapor pressure above pure liquid i. The exponential term in Equation (Equation1
[1] ) is the size-dependent Kelvin term where σ is surface tension of the solution, and
is the partial molar volume of i in the liquid. The condensing species that we consider are SA, DMA, water, and ammonia. For simplicity, we approximate the partial molar volumes by the molar mass of species i divided by the particle density. According to performed sensitivity tests, this approximation introduces an error of less than 17% in the predicted particle composition (base to SA ratio).
In E-AIM, the activity coefficients of water and ions are calculated based on the Pitzer, Simonson, and Clegg equations (Clegg et al. Citation1992). We chose the group contribution method UNIFAC as the activity model for the neutral form of dimethylamine in E-AIM. In the aqueous phase, acid deprotonation and base protonation are taken into account. The density of the aqueous solution in the model is described by a parameterization obtained from measurements of densities of aqueous solutions of dimethyl aminium sulfate (Clegg et al. Citation2013). Surface tension in the model is described by a composition-dependent parameterization obtained from measurements of the SA-DMA-water system by Hyvärinen et al. (Citation2004). The size-dependence of the surface tension is only taken into account through the potential dependence that composition has on particle size, otherwise the capillarity assumption is assumed to hold true (see Henschel et al. Citation2014 for a discussion on the validity of this assumption for the studied system).
To estimate the composition of a particle of a certain size, the equilibrium vapor pressures obtained from E-AIM were corrected for the Kelvin effect by multiplying with the Kelvin term in Equation (Equation1[1] ) in an iterative manner.
2.2.2. Kinetic condensation modeling
The evolution of particle size and composition for the SA-DMA-water system was investigated using the monodisperse particle growth model for acid-base chemistry in nanoparticle growth (MABNAG; Yli-Juuti et al. Citation2013). Vapor concentrations and initial particle size and composition are given as inputs to MABNAG. The model predicts how particle size and composition change with time. Condensation of SA is calculated based on its mass flux in the gas phase according to Nieminen et al. (Citation2010):[2]
[3]
In these equations, d is the diameter, M is the molar mass of SA, R is the gas constant, T is the temperature, is the thermal speed, p is the partial pressure of SA, and peq is the equilibrium vapor pressure of SA, which in this study in MABNAG was set to zero. Subscripts p and v refer to the particle and SA vapor, respectively. Furthermore, ϵ is a correction factor to describe the situation also in the continuum regime, Kn is the Knudsen number, and βm is the transition regime correction factor for mass transport determined according to Fuchs and Sutugin (Citation1970) and Lehtinen and Kulmala (Citation2003).
In MABNAG, water and DMA are, contrary to SA, assumed to be in equilibrium between the gas and particle phases, and these equilibria are calculated by E-AIM for each time step (Section 2.2.1). The equilibrium vapor pressures determined by E-AIM are corrected for the Kelvin effect according to Equation (Equation1[1] ). In the original version of MABNAG described by Yli-Juuti et al. (Citation2013), particle density and surface tension were assumed to stay constant. However, in the updated version of MABNAG used in this study, these parameters are assumed to change with particle composition (Section 2.2.1). This means that changes in particle density and surface tension are now taken into account in the iteration of the Kelvin effect in MABNAG.
The aerosol pH is calculated in MABNAG through the following equation (Stumm and Morgan Citation1996):[4] where
represent the hydrogen ion activity coefficient and the aqueous mole fraction, respectively, which come as output from E-AIM.
To test the impact of self-coagulation during the growth events discussed in Section 3.2, we extended MABNAG to include coagulation between newly formed particles. Since MABNAG is a monodisperse model, we assumed coagulation to be monodisperse, thus assuming all particles to have the same particle size. This means that we predict a lower limit of the contribution from coagulation to the growth, because the coagulation coefficient increases when the ratio of the particle diameters for two coagulating particles increases (Seinfeld and Pandis Citation2006). This version of MABNAG is MABNAGcoag. The coagulation coefficients were defined according to Table 13.1 in Seinfeld and Pandis (Citation2006). Note that coagulation will not affect the predicted particle composition at a certain diameter because DMA is assumed to equilibrate at each time step in the model.
To estimate the contribution of cluster collisions during the growth events, we developed a cluster version of MABNAG. We calculated a time-independent concentration distribution of SA-DMA clusters using the Atmospheric Cluster Dynamics Code (ACDC) model (McGrath et al. Citation2012). We consider only clusters with up to four SA and four DMA molecules, for which the quantum chemical data used here to calculate cluster evaporation rates were available. Water molecules were not included in the cluster population simulations due to the lack of quantum chemical data for all hydrated clusters. The cluster version of MABNAG calculates the mass flux of sulfuric acid into the particle by calculating the coagulation rates of clusters onto the particle in every time step. A gas and particle phase equilibrium is assumed to hold for DMA similarly to the original version of the model. This version of MABNAG is MABNAGcluster.
3. Results and discussion
3.1. Evolution of the particle composition between 2 and 20 nm
Particles were formed in the CLOUD chamber by ternary SA-DMA-water nucleation (Almeida et al. Citation2013) followed by growth to more than 20 nm in dry diameter. Hygroscopic growth factors were determined for 10, 15, and 20 nm dry diameter particles based on measurements with the nano-HTDMA (Kim et al. Citation2016), while during most periods the TDCIMS did not size-select particles, but rather allowed the particle size distribution in the chamber to determine the range of sampled particle size.
shows how the HGFs changed with particle size for six different 5–8 h long experiments with the π+ beam turned off and the clearing field turned on, i.e., neutral experiments with no ions in the chamber. The gas phase DMA concentration varied between 20 and 45 pptv during these experiments, whereas the SA monomer concentration varied between 5 × 106 and 3 × 107 cm−3. As seen in , HGFs were highest for the 10 nm particles, being between 1.47 and 1.52, and lower for the 15 nm (1.38–1.42) and 20 nm (1.38–1.46) particles. Decreasing HGFs as the particles grow is contrary expectations for particles of identical composition. Due to a decreasing Kelvin effect with increasing particle size (Equation (Equation1[1] )), the HGF would increase with increasing particle size if the particle composition were constant during growth. This has been observed in several studies, e.g., Lewis (Citation2006), Biskos et al. (Citation2009), and Park et al. (Citation2009). Thus, because the HGF did not increase with increasing particle size, the particles must have become less hygroscopic as they grew.
Table 1. Observed HGFs for different DMA and SA gas phase concentrations in the CLOUD chamber (Kim et al. Citation2016), and particle phase base/acid ratios given as input to the simulations resulting in a predicted water uptake agreeing with the observed HGFs for 10, 15, and 20 nm dry diameter particles.
TDCIMS observations of base to acid ion ratio were conducted for particles larger than about 10 nm during these experiments. To achieve sufficient signal, the TDCIMS sample time was 30 min for these experiments, and there was no size-selection of the sampled particles, resulting in a range of particle diameters sampled. The TDCIMS measurements consistently show particle base-to-acid ratios a factor of 5 to 10 times lower than pH-neutral ammonium sulfate calibration aerosol. The observed base-to-acid ratio was in the range of 0.07–0.45 for the experiments described here (Lawler et al. Citationin review). Ammonium was always a significant contributor to the observed base fraction of the particles, typically present at levels greater than or comparable to DMA.
A series of simulations of aerosol particle water uptake were performed using E-AIM in order to investigate what DMA to SA ratio in the particle phase results in a water uptake matching the HGFs observed by the nano-HTDMA for 10, 15, and 20 nm particles. In these model simulations, evaporation of SA and dimethylamine was turned off in E-AIM, meaning that these two compounds only existed in the particle phase in the simulations. Thus, the aerosol water uptake was predicted by E-AIM for a fixed base-to-acid ratio in the particle phase given as input to the model together with the RH observed inside DMAwet during the specific experiment. The Kelvin effect was taken into account externally for 10, 15, and 20 nm particles.
As an example of these simulations, shows observed HGFs for 10 nm (dashed line) and 20 nm (dotted line) dry diameter particles during the experiment on the first row of , together with predicted HGFs at an RH of 90% for some different base/SA molar ratios in the particle phase. In , the observed HGFs are 1.47 and 1.38 for the 10 nm and 20 nm particles, respectively. As discussed above and seen in , larger HGFs are predicted for the 20 nm particles (circles) than for the 10 nm particles (stars) for a specific base/SA molar ratio in the particle phase due to a decreasing Kelvin effect with increasing size. The HGFs are also predicted to decrease as the base/SA molar ratio increases because DMA is less hygroscopic than SA. The best agreement between the simulation and the nano-HTDMA observations occurs for a base/SA ratio of ∼0.4 and ∼1.4 for the 10 and 20 nm particles, respectively. Thus, given the observed HGFs and the simulated water uptake with E-AIM, the 10 nm particles had a lower base/SA ratio than the 20 nm particles, which had a base/SA ratio between those of dimethyl aminium bisulfate (one base molecule per SA molecule) and dimethyl aminium sulfate (two base molecules per SA molecule). In summary, the results of these model simulations indicate that the base/acid ratio of the particles is predicted to increase during growth.
Figure 1. HGFs predicted by E-AIM for 10 and 20 nm dry diameter particles (stars, circles, squares, and triangles) and observed with nano-HTDMA (dashed and dotted lines) for the first experiment in with RH = 90 ± 2% in DMAwet. Each point (star, circle, square, or triangle) represents one simulation with the particle composition given on the x-axis. The error bars show the uncertainty in HGF due to uncertainty in RH in DMAwet.
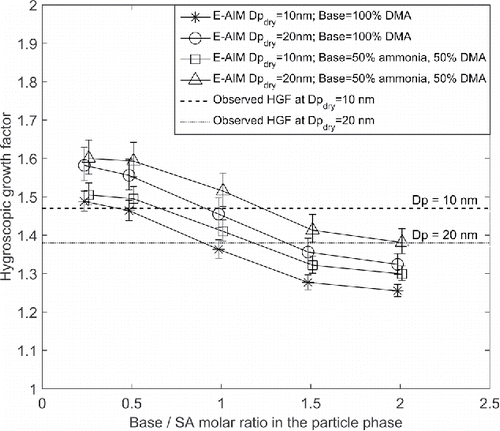
Measurements by TDCIMS and the ion chromatograph indicated some contamination by ammonia in the particle and gas phases during these new particle formation events (Lawler et al. Citationin review). To get an idea of how sensitive the water uptake is to ammonia contamination, we repeated the set of model simulations under the assumption that the base in the particle phase consisted of 50% DMA and 50% ammonia, for the 10 nm (squares) and 20 nm (triangles) particles. As seen in , this modification results in somewhat higher predictions of the HGFs. The best agreement between the nano-HTDMA observations and simulations assuming that the particles contained ammonia occurs at base/SA molar ratios of ∼0.7 and ∼2 for the 10 and 20 nm particles, respectively. Thus, the 20 nm particles are now predicted to contain two base molecules per acid molecule.
By repeating the procedure discussed above, and presented in , for each of the experiments in , the particle composition could be predicted for each of these experiments. The resulting particle composition using this method for the 10, 15, and 20 nm particles, when assuming 0 or 50% ammonia as base in the particle phase, is shown in . For each of the experiments, the base/SA ratio is predicted to increase as the particles grow from 10 to 20 nm, as mentioned previously. The increase in particle base/SA ratio during growth is also illustrated in as arithmetic means of the predicted base/SA ratios in at 10, 15, and 20 nm (black x-marks) with the error bars representing the full range of values. The DMA/SA ratios measured with the TDCIMS overlap with the base/SA ranges determined from the nano-HTDMA observations combined with E-AIM simulations at 10 nm but are otherwise lower ().
Figure 2. Evolution of the particle chemical composition (a) based on: (Equation1[1] ) observations of positive and negative cluster composition using APi-TOF; (Equation2
[2] ) MABNAG simulations of the evolution of the chemical composition under the observed gas phase concentrations in the chamber; (Equation3
[3] ) E-AIM simulations of hygroscopic growth factors and comparisons to nano-HTDMA data; and direct observations by the TDCIMS. (b) The evolution of the aerosol pH as simulated by MABNAG. Solid and dashed lines in (a) and (b) represent simulations with assumed NH3 concentrations of 0 and 20 pptv, respectively. The temperature in the chamber was 278 K. The RH in the chamber was 38% and in the humidified DMA 90 ± 2%. The presented MABNAG simulations were run for chamber conditions of temperature and RH.
![Figure 2. Evolution of the particle chemical composition (a) based on: (Equation1[1] ) observations of positive and negative cluster composition using APi-TOF; (Equation2[2] ) MABNAG simulations of the evolution of the chemical composition under the observed gas phase concentrations in the chamber; (Equation3[3] ) E-AIM simulations of hygroscopic growth factors and comparisons to nano-HTDMA data; and direct observations by the TDCIMS. (b) The evolution of the aerosol pH as simulated by MABNAG. Solid and dashed lines in (a) and (b) represent simulations with assumed NH3 concentrations of 0 and 20 pptv, respectively. The temperature in the chamber was 278 K. The RH in the chamber was 38% and in the humidified DMA 90 ± 2%. The presented MABNAG simulations were run for chamber conditions of temperature and RH.](/cms/asset/83842724-b743-4ef0-803a-5e2b67404e4e/uast_a_1223268_f0002_c.gif)
At diameters below 2 nm, the evolution of the steady-state composition during growth was investigated using measurements by the APi-TOF that provided the evolution of the cluster composition from ∼1 to ∼2 nm dry diameter (). The negative (stars) and positive (pluses) clusters start out at a base/SA molar ratio of zero and one, respectively. The formation of SA-DMA clusters is not the focus of this study, but has been investigated in detail by Almeida et al. (Citation2013), Kürten et al. (Citation2014), and Bianchi et al. (Citation2014). According to these studies, the first step in the formation of the negatively charged clusters is the formation of the SA dimer (H2SO4· HSO4−), explaining why the negative clusters start out at a base/SA ratio of zero in . Once the most abundant trimer has formed, consisting of three SA molecules and two DMA molecules (3,2), each condensing acid molecule can be stabilized by one additional DMA molecule, resulting in clusters with a base/SA ratio close to that of dimethyl aminium bisulfate. Beyond (7,6) clusters, increased uptake of DMA resulted in a base/SA molar ratio slightly larger than one (Almeida et al. Citation2013). The growth of the positive clusters started out from a protonated DMA ion but was otherwise observed to proceed by pairwise additions of SA and DMA molecules similar to the growth of the negative clusters (Almeida et al. Citation2013).
includes the evolution of the steady-state composition of the negative and positive clusters as they grow in size, measured by the APi-TOF during both CLOUD4 (black) and CLOUD7 (cyan and orange). The CLOUD4 cluster compositions in represent the average evolution of the cluster composition for negative and positive clusters between ∼1 and ∼2 nm for a large number of experiments with DMA gas phase concentrations between 3.5 and 80 pptv, with the mean being equal to 26 pptv, and SA monomer concentrations between 7.5 × 105 and 5.7 × 106 cm−3. The cluster compositions representing CLOUD7 are based on two individual experiments with DMA gas phase concentrations at either 18 pptv (cyan) or 72 pptv (orange), values close to the DMA gas phase concentrations during the nano-HTDMA and TDCIMS observations (), which were also part of CLOUD7. The SA monomer concentrations during these two events were at 1.2 × 107 and 1.1 × 107 cm−3, respectively. Whereas the HGF and chemical composition measurements were performed without ions in the chamber, the APi-TOF measurements were performed with the clearing field turned off because the APi-TOF can only detect charged clusters. However, cluster population dynamics simulations with the Atmospheric Cluster Dynamics Code (ACDC) model suggest that the evolution of the neutral cluster composition evolves in the same way as charged clusters, with pairwise addition of SA and DMA molecules at smaller cluster sizes (Olenius et al., Citation2013; Almeida et al. Citation2013). This is in agreement with observations of neutral clusters with CI-APi-TOF by Kürten et al. (Citation2014). The difference between CLOUD4 and CLOUD7 in base/SA molar ratio for clusters measured with the APi-TOF is relatively small, in particular for the negative clusters (). The positively charged clusters in CLOUD4 have slightly higher base/SA molar ratios than those observed during the two experiments in CLOUD7. The same APi-TOF was used in CLOUD4 and CLOUD7 for the measurements of negative ions whereas two different APi-TOFs were used for the measurements of positive ions, explaining why there is a larger difference between CLOUD4 and CLOUD7 for positive clusters. However, the overall trend for the observed cluster base/SA molar ratio is, regardless of charge of the cluster, to increase as the clusters grow toward 2 nm.
The APi-TOF thus covers the ∼1–2 nm dry diameter interval, whereas the nano-HTDMA together with E-AIM simulations provide indirect information of the particle composition at 10, 15, and 20 nm, and TDCIMS provides direct observations of composition in the 10–30 nm diameter range. To complement and connect these observations, we performed a set of model simulations using MABNAG. The simulations were performed with input gas phase DMA concentrations of 3.5 and 80 pptv, which were the upper and lower limits of the DMA concentration during the CLOUD4 APi-TOF measurements, and 10 and 40 pptv, values close to the observed DMA concentrations during the HGF measurements. The input SA gas phase concentration was set to 107 cm−3 in all these simulations. The SA concentration, however, does not affect the predicted particle composition at a certain diameter because the model assumes that both DMA and water vapor are in equilibrium with the particle phase in each time step.
As an example of these simulations, shows how the mole fractions of SA, DMA, and water are predicted to change as a particle grows between 2 and 26 nm in diameter for an input DMA gas phase concentration of 40 pptv. Due to a decreasing Kelvin effect with increasing particle size, the mole fraction of water increases as the particle grows. This results in an increasing surface tension and a decreasing particle density with increasing particle size (), since water has a higher surface tension and lower density than SA and DMA. The increasing surface tension and decreasing particle density make the Kelvin term larger than it would be if these parameters remained constant at their initial values (Equation (Equation1[1] )). This means that the rate at which the Kelvin effect for water decreases with increasing particle size will be somewhat reduced considering variable surface tension and particle density. As seen in , the SA and DMA mole fractions both decrease as the particle grows due to the increasing water mole fraction. However, the DMA mole fraction decreases more slowly than the SA mole fraction, resulting in an increasing DMA/SA molar ratio with increasing particle size (green line in ). This is due to a decreasing Kelvin effect for DMA with increasing particle size. The changes in mass fractions of SA and DMA as the particle grows () are much smaller than the corresponding changes in mole fractions. The SA mass fraction is larger than the DMA mass fraction as a result of SA having a larger molar mass than DMA. This means that condensation of sulfuric acid dominates the condensational growth, although the contribution of DMA to the particle dry size somewhat increases as the particle grows following from a decreasing Kelvin effect with increasing particle diameter. Due to the low molar mass of water, the contribution of water vapor to the growth is relatively low in these simulations representing CLOUD chamber conditions with a relative humidity of 38%.
Figure 3. MABNAG simulation with the DMA gas phase concentration being equal to 40 pptv. The plots show change during growth for (a) molar fractions in the particle phase of SA, DMA, and water; (b) surface tension and particle density; (c) mass fraction in the particle phase.
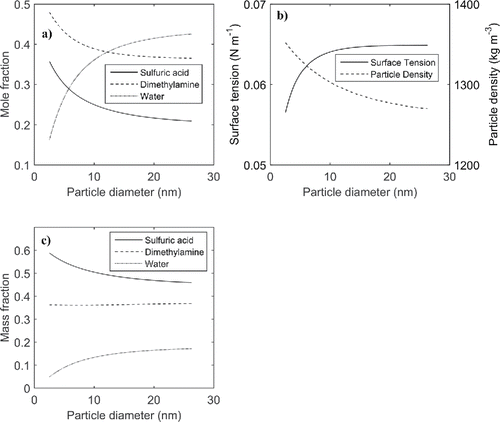
As seen in , the predicted particle-phase base/SA molar ratio from these simulations with MABNAG (solid lines) increases with increasing input DMA gas phase concentration. However, the base/SA ratio dependency on the DMA gas-phase concentration is predicted to decrease with decreasing particle size. At a dry diameter of 20 nm, the simulation with an input DMA gas phase concentration of 3.5 pptv gives a predicted base/SA molar ratio of less than 1.4, whereas the simulation with an input DMA gas phase concentration of 80 pptv gives a base/SA ratio of almost 2. At 2 nm, however, the difference between base/SA ratios predicted at 3.5 pptv and 80 pptv DMA is minor; the predicted base/SA ratio is ∼1.25 for 3.5 pptv DMA and ∼1.45 at 80 pptv DMA. Interestingly, the predicted base/SA ratios at 2 nm are, for all the different input gas phase concentrations between 3.5 and 80 pptv, relatively close to the base/SA ratio observed by the APi-TOF at 2 nm. This indicates that the DMA was, if not completely, at least close to being in equilibrium between the gas and particle phases at 2 nm, since this equilibrium is assumed to prevail in MABNAG. Furthermore, it suggests that thermodynamic modeling with E-AIM seems to be a reasonable approach for representing the composition of particles as small as ∼2 nm as long as the composition-dependence of the Kelvin effect (including the composition-dependence of density and surface tension) is accounted for—and at least until more powerful computational chemistry-based approaches become available. These results are generally in line with those by Henschel et al. (Citation2014) who presented a comparison of quantum chemical and thermodynamic calculations on the hydration of SA+DMA clusters. Another trend seen in for DMA gas phase concentrations of 40 pptv or higher is a predicted increase in base/SA ratio of the particles as they grow from 2 to 20 nm. For gas phase DMA concentrations of 10 pptv or lower, the corresponding ratio is predicted to only slightly increase as the particles grow up to 20 nm. The aerosol pH is predicted to increase for all the simulations (), indicating that the acidity of the particles decreases during growth. The predicted pH values presented in are close to those estimated by Weber et al. (Citation2016) for aqueous particles of ammonium bisulfate (pH ∼ 0) and ammonium sulfate (pH ∼ 3).
As mentioned in Section 3.1, measurements with the ion chromatograph indicated some ammonia contamination in the chamber with typical concentrations of ∼20 pptv. To investigate the impact of ammonia on predictions of the particle base/acid ratio, additional simulations were run with MABNAG assuming 20 pptv of ammonia in the gas phase. These simulations predicted a particle phase DMA/ammonia mole fraction much larger than one for DMA gas phase concentrations of 40 or 80 pptv, close to one for a DMA gas phase concentration of 10 pptv, and lower than one for gas phase concentration of 3.5 pptv (not shown). As seen in , including ammonia slightly increases the predicted base/SA ratio (), and the aerosol pH (), in particular for the simulations with low DMA gas phase concentrations. Decreasing acidity during particle growth is predicted by the model also when taking ammonia contamination into account ().
MABNAG simulations assuming that DMA is in equilibrium between the gas- and particle phases thus seem to agree closely with observations by the APi-TOF at 2 nm. Furthermore, the MABNAG simulations as well as the observations by the APi-TOF suggest that the particle acidity decreases with increasing particle size. Moreover, the evolution of the composition between 10 and 20 nm based on predictions of water uptake by E-AIM and comparisons to nano-HTDMA data follows the same trend in terms of acidity. At 20 nm dry diameter, the particle compositions predicted by MABNAG are within the range of the particle compositions resulting from the HGF observations combined with E-AIM simulations. However, the TDCIMS measurements suggest a much higher acidity at 20 nm. At 10–15 nm dry diameter, both direct observations with TDCIMS and hygroscopic growth analysis suggest a lower base/SA molar ratio than predicted by MABNAG. The temperature outside the chamber was 20°C whereas the temperature inside the chamber was 5°C implying an increasing temperature as the air flows from the chamber to the nano-HTDMA and TDCIMS. This means that some DMA could evaporate in the sampling line as a result of the higher temperature, and thereby also lower RH, outside the chamber. A simulation was performed using a test version of MABNAG to estimate the upper limit of the impact of evaporation in the sampling line on the DMA to SA ratio assuming a concentration of 40 pptv gas-phase DMA in the chamber and no gas-phase DMA present in the sampling line. In this test version of MABNAG, DMA partitioning between the gas and particle phases was treated kinetically. This simulation indicated a reduction in DMA to SA ratio by 15% and 17% for 10 nm and 20 nm diameter particles, respectively. These predicted reductions in DMA to SA ratio are lower than the 53% discrepancy between MABNAG and the upper limit of estimates based on the combined nano-HTDMA and E-AIM approach. The TDCIMS observations for 10 and 20 nm particles could be impacted by further evaporation during the period between their collection and analysis, but they show only a modestly higher discrepancy with MABNAG (69% and 74%, respectively) than the HTDMA-based approach. Thus, evaporation of DMA in the sampling line can only explain a minor fraction of the discrepancies in DMA to SA ratio.
Another potential factor that could contribute to the lower acidity predicted by MABNAG, compared to observations, is the assumption in MABNAG that DMA is in instant equilibrium between the gas and particle phases, which could result in an overprediction of DMA uptake by the particles in the chamber. However, predictions of DMA to SA ratios using the test version of MABNAG with kinetic condensation/evaporation of DMA shows a negligible difference compared to the predictions using the default version of MABNAG.
Other potential explanations for the discrepancy in particle acidity include the possibility that there might be aspects, e.g., particle size-dependencies, of the particle-phase neutralization reactions and the phase state (Chan and Chan Citation2013; Cheng et al. Citation2015) that we currently do not understand, and that are not taken into account in MABNAG. There may also exist unexpected nonlinear effects in the surface tension or density parameterizations and thus in the Kelvin effect not accounted for by MABNAG. Furthermore, there are uncertainties in the thermodynamics of dimethyl aminium sulfate (DMAS) water uptake. Previous studies have suggested that DMAS would have equilibrium water content on molar basis similar to that of ammonium sulfate, and this is also assumed in E-AIM (Clegg et al. Citation2013). However, a recent study (Sauerwein et al. Citation2015) on water activities of bulk solutions found significantly higher equilibrium water content for DMAS compared to previous studies (Clegg et al. Citation2013). At 90% RH, the water content of DMAS aqueous solution (moles of water per moles of salt) measured by Sauerwein et al. (Citation2015) was more than 50% larger than that of ammonium sulfate. This suggests a significant underestimation of the hygroscopicity of DMAS in the thermodynamic calculations in the current study, which would lead to an underestimation also of the DMA/SA ratio derived from the HTDMA measurements. Assuming a higher hygroscopicity of DMAS in estimates of the particle composition would shift the HTDMA-derived DMA/SA ratios closer to those predicted using MABNAG. Equilibrium water content of DMAS aqueous solution at 90% RH according to Sauerwein et al. (Citation2015) would give approximately 47% larger HGF of bulk solution compared to the results in this study based on results by Clegg et al. (Citation2013). This increase in HGF would translate to an increase by approximately 69% in the κ value, which in turn would correspond to an increase by approximately 20%–50%, or even more, in the calculated DMAS fraction. It should be noted that these numbers are rough estimates as the calculation requires assumptions of, e.g., density of the DMAS aqueous solution (here estimated using E-AIM) and they are given here only to demonstrate the extent of effects of the possible uncertainties in the thermodynamic properties. Finally, there are uncertainties in the measurements as well (Lawler et al. Citationin review; Kim et al. Citation2016).
3.2. Growth rates and contribution from different processes
We estimated particle diameter growth rates by measuring particle number size distribution with a nano-SMPS and calculating the 50% rise of a size bin during new particle formation events in the chamber. shows the growth rates observed during six different experiments together with the average SA monomer concentration ranging between 5.1 × 106 and 1.2 × 107 cm−3, and the average DMA gas phase concentration ranging between 20 and 44 pptv in the different experiments. Gas phase ammonia was present only as a result of contamination with typical concentrations around 20 pptv. The growth rates were measured over different particle diameter intervals in different experiments, but within the range of 4–34 nm. In all these experiments, the π+ beam was turned off and the clearing field turned on implying ion-free conditions in the chamber. The growth rates in range from 2.4 to 11.5 nm h−1, generally increasing with increasing SA monomer concentration.
Table 2. Particle diameter interval, average gas phase concentration, and observed and predicted growth rates with MABNAG default version and with self-coagulation between particles included during six different experiments in ion-free conditions in the CLOUD chamber.
Condensational growth and resulting growth rates were also predicted based on simulations with MABNAG for each of the six experiments with model input taken from the observed average gas phase concentrations, temperature, and relative humidity (). The standard deviations of the gas phase concentrations during the growth events were 11%–34% of the average concentrations. The predicted growth rates resulting from these simulations are plotted against the observed growth rates in (circles and squares). Clearly, MABNAG substantially underpredicts the growth rates, implying that the condensation of SA monomers, gas phase DMA, and water vapor alone cannot explain the observed growth rates. Lehtipalo et al. (Citation2016) observed a large contribution from clusters containing SA and DMA to the growth for particles in the range of 1–3 nm. The SA molecules in these clusters are not measured by the CIMS since it only detects SA monomers. The CLOUD7 measurements show that in the presence of DMA, the SA monomers measured by the CIMS represent only a fraction of the total SA contained in the monomers and clusters, that is available for particle growth (Rondo et al. Citation2016). Therefore, to investigate the contribution of cluster collisions to the growth for the six experiments in , we ran the same simulations using the cluster version of the model MABNAGcluster (Section 2.2.2), thereby predicting growth rates resulting from both the condensation of monomers and collisions of clusters with up to four SA and four DMA molecules. Taking cluster collisions into account, however, only increased the growth rates by less than 10% (2%–10% increase), indicating that collisions of clusters containing SA and DMA may have contributed less to the growth for particles with a diameter of 4–34 nm than for the 2 nm particles investigated by Lehtipalo et al. (Citation2016). However, the MABNAGcluster calculations included only clusters up to the 4–4 cluster leaving out larger clusters, which could increase the cluster contributions to the growth.
Figure 4. Simulated versus observed growth rates using the default version of MABNAG (circles and squares) and MABNAGcoag with monodisperse coagulation included (crosses and triangles). Circles and crosses denote growth rates with initial size below 10 nm, and squares and triangles denote growth rates with initial size above 10 nm. The horizontal bars indicate the uncertainty in the observations.
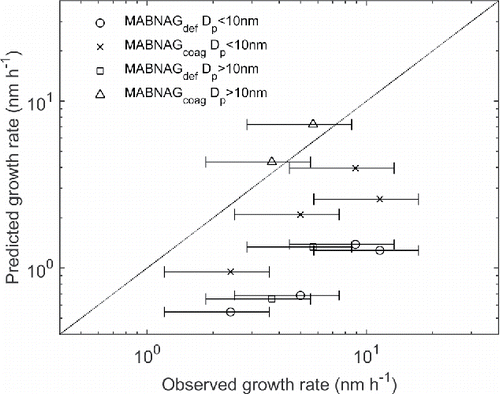
Another microphysical process that can contribute significantly to the growth is coagulation of aerosol particles (Kerminen and Kulmala Citation2002). The importance of coagulation increases with increasing particle number concentration. The number concentrations in the CLOUD chamber during these particle formation experiments were high, reaching 105 cm−3 in most of the events and 5 × 105 cm−3 in one of the events, suggesting that coagulation could potentially be important in these experiments. To get a rough estimate of how coagulation could affect the growth rates, we ran the same simulations using the coagulation version of the model MABNAGcoag—assuming that self-coagulation is the dominant coagulation process in the newly formed and growing particle population and thus yielding a lower limit for the importance of coagulation. As seen in , the growth rates get closer to the observed values when taking into account self-coagulation of aerosol particles. According to these simulations, coagulation between newly formed particles accounted for at least 43%–85% of the diameter growth. The two simulations with best agreement between simulation and observation occurred for the two growth events occurring at the largest particle diameters (12.9–19.1 nm and 16.0–34.0 nm) denoted with triangles in . The maximum coagulation coefficients for equally sized particles are reached at about 20 nm diameter (Seinfeld and Pandis Citation2006), and the underprediction due to the monodisperse assumption is less significant at the larger sizes (Hinds Citation1999).
4. Conclusions
We investigated the aerosol particle size and composition evolution of freshly formed aerosol particles consisting of SA, DMA, and water using (1) simulations with the particle growth model MABNAG, (2) observations of the cluster composition using APi-TOF in the CLOUD chamber, (3) observations of HGFs in the CLOUD chamber with a nano-HTDMA system combined with E-AIM simulations of water uptake, and (4) direct measurements of nano-particle composition by TDCIMS. In addition, we determined growth rates using nano-SMPS data. Here the key results and main conclusions are presented:
The simulations with MABNAG, assuming DMA is always in thermodynamic equilibrium between the gas and particle phases, agree relatively well with observations by the APi-TOF at a diameter of ∼2 nm in terms of molar ratio between DMA and SA molecules in the particle phase. According to the observations by the APi-TOF and the simulations with MABNAG, this ratio varies between 1 and 1.3 at 2 nm, and is relatively insensitive to variations in gas-phase DMA in the 3.5–80 pptv range with typical atmospheric concentrations of SA.
The MABNAG simulations, the direct observations by the APi-TOF, and the nano-HTDMA observations combined with E-AIM simulations, all suggest that the acidity of the nucleated particles decreases as the particles grow from ∼2 to 20 nm. According to the model simulations, this is a result of a decreasing Kelvin effect of DMA with increasing particle diameter, which increases particle phase DMA concentrations and thereby increases the amount of DMA available for particle phase acid–base reactions with sulfuric acid.
MABNAG predicts a lower particle phase acidity than suggested by the indirect estimates using the nano-HTDMA observations combined with E-AIM simulations at 10 nm diameter, and less acidic particles than observed by the TDCIMS at 10–30 nm. Evaporation of DMA in the sampling line can only explain a minor part of these discrepancies. Other potential explanations for the discrepancies in particle acidity include (1) that there might be aspects of the particle-phase neutralization reactions that we do not understand; (2) nonlinear effects in the surface tension or density parameterizations and thus in the Kelvin effect; (3) uncertainties in the phase of the particles; and (4) uncertainties in the hygroscopicity of dimethyl aminium sulfate; and (5) instrumental artefacts.
Growth rates in the 4–34 nm diameter range were measured with nano-SMPS and simulated with MABNAG. Sulfuric acid had the largest mass flux and thereby contributed most to the condensational growth. When taking only condensation of SA monomers, gas-phase DMA, and water vapor into account, MABNAG substantially underpredicted the growth rates. However, when self-coagulation was accounted for, the simulated growth rates were brought closer to the observed ones. Thus, self-coagulation between aerosol particles made a large contribution to the diameter growth rates (43%–85%) under the conditions in the CLOUD chamber in this study.
These results, together with those reported by Almeida et al. (Citation2013), Kürten et al. (Citation2014), Kim et al. (Citation2016), and Lehtipalo et al. (Citation2016), suggest that for simplified approaches required for, e.g., large-scale modeling purposes, it is reasonable to assume that particles formed by the nucleation of SA stabilized by DMA begin with a DMA/SA ratio of about 1. As the nucleated particles grow toward 10 nm, they evolve toward lower acidity depending on the ambient DMA concentration. This is similar to what was observed for the SA-ammonia system by Schobesberger et al. (Citation2015). However, between ∼10 and 20 nm, the discrepancies in base to SA ratio between the model predictions and the direct and indirect observations suggest that there might be factors involved that we do not fully understand, such as size-dependencies of the phase state or particle-phase neutralization reactions.
While our results give the first estimates of how the particle composition in the nucleating SA-DMA-water system might evolve, they also point at important questions to be answered by future studies. First, the apparent discrepancy between the predicted particle composition and the TDCIMS and nano-HTDMA results at 10–20 nm remains to be explained. Second, instead of simple SA-DMA-water systems, the atmospheric aerosol formation processes often include other molecules as well such as ammonia and oxidized organics (Bzdek et al. Citation2010) whose exact interactions with the DMA-containing system need to be quantified.
Acknowledgments
The authors would like to thank CERN for supporting CLOUD with important technical and financial resources, and for providing a particle beam from the CERN Proton Synchrotron. The authors also thank P. Carrie, L.-P. De Menezes, J. Dumollard, K. Ivanova, F. Josa, I. Krasin, R. Kristic, A. Laassiri, O. S. Maksumov, B. Marichy, H. Martinati, S. V. Mizin, R. Sitals, A. Wasem, and M. Wilhelmsson for their important contributions to the experiment. The authors thank the tofTools team for providing tools for mass spectrometry analysis. The authors would also like to thank Tinja Olenius for providing the cluster distributions from the ACDC model used in their MABNAG simulations to determine the contribution from cluster collisions to the growth.
Funding
This research has received funding from the EC Seventh Framework Programme (EG Marie Curie Initial Training Network “CLOUD-ITN,” MC-ITN “CLOUD-TRAIN” no. 316662), the European Union's Horizon 2020 research and innovation program under the Marie Sklodowska-Curiegrant agreement No 656994, U.S. Department of Energy (DE-SC0014469), the German Federal Ministry of Education and Research, the Swiss National Science Foundation, the Academy of Finland, the Finnish Funding Agency for Technology and Innovation, the Väisälä Foundation, the Nessling Foundation, the Strategic Funding from University of Eastern Finland, the Austrian Science Fund, the Portuguese Foundation for Science and Technology, the Swedish Research Council (grant 2011-5120), the Swedish Research Council FORMAS (grant 2015-748), the Presidium of the Russian Academy of Sciences and Russian Foundation for Basic Research, the U.S. National Science Foundation, and the Davidow Foundation.
References
- Ahlm, L., Julin, J., Fountoukis, C., Pandis, S. N., and Riipinen, I. (2013). Particle Number Concentrations Over Europe in 2030: The Role of Emissions and New Particle Formation. Atmos. Chem. Phys., 13:10271–10283.
- Almeida, J., Schobesberger, S., Kürten, A., Ortega, I. K., Kupiainen-Määttä, O., Praplan, A. P., Adamov, A., Amorim, A., Bianchi, F., Breitenlechner, M., David, A., Dommen, J., Donahue, N. M., Downard, A., Dunne, E., Duplissy, J., Ehrhart, S., Flagan, R. C., Franchin, A., Guida, R., Hakala, J., Hansel, A., Heinritzi, M., Henschel, H., Jokinen, T., Junninen, H., Kajos, M., Kangasluoma, J., Keskinen, H., Kupc, A., Kurtén, T., Kvashin, A. N., Laaksonen, A., Lehtipalo, K., Leiminger, M., Leppä, J., Loukonen, V., Makhmutov, V., Mathot, S., McGrath, M. J., Nieminen, T., Olenius, T., Onnela, A., Petäjä, T., Riccobono, F., Riipinen, I., Rissanen, M., Rondo, L., Ruuskanen, T., Santos, F. D., Sarnela, N., Schallhart, S., Schnitzhofer, R., Seinfeld, J. H., Simon, M., Sipilä, M., Stozhkov, Y., Stratmann, F., Tomé, A., Tröstl, J., Tsagkogeorgas, G., Vaattovaara, P., Viisanen, Y., Virtanen, A., Vrtala, A., Wagner, P. E., Weingartner, E., Wex, H., Williamson, C., Wimmer, D., Ye, P., Yli-Juuti, T., Carslaw, K. S., Kulmala, M., Curtius, J., Baltensperger, U., Worsnop, D. R., Vehkamäki, H., and Kirkby, J. (2013). Molecular Understanding of Sulphuric Acid-Amine Particle Nucleation in the Atmosphere. Nature, 502:359–363.
- Amann, M., Borken-Kleefeld, J., Cofala, J., Heyes, C., Klimont, Z., Rafaj, P., Purohit, P., Schöpp, W., and Winiwarter, W. (2012). Future Emissions of Air Pollutants in Europe. Current legislation baseline and the scope for further reductions. IIASA, TSAP Report #1, Version 1.0.
- Ball, S. M., Hanson, D. R., Eisele, F. L., and McMurry, P. H. (1999). Laboratory Studies of Particle Nucleation: Initial Results for H2SO4, H2O, and NH3 Vapors. J. Geophys. Res., 104:23709–23718.
- Bianchi, F., Praplan, A. P., Sarnela, N., Dommen, J., Kürten, A., Ortega, I. K., Schobesberger, S., Junninen, H., Simon, M., Tröstl, J., Jokinen, T., Sipilä, M., Adamov, A., Amorim, A., Almeida, J., Breitenlechner, M., Duplissy, J., Ehrhart, S., Flagan, R. C., Franchin, A., Hakala, J., Hansel, A., Heinritzi, M., Kangasluoma, J., Keskinen, H., Kim, J., Kirkby, J., Laaksonen, A., Lawler, M. J., Lehtipalo, K., Leiminger, M., Makhmutov, V., Mathot, S., Onnela, A., Petäjä, T., Riccobono, F., Rissanen, M. P., Rondo, L., Tomé, A., Virtanen, A., Viisanen, Y., Wiiliamson, C., Wimmer, D., Winkler, P. M., Ye, P., Curtius, J., Kulmala, M., Worsnop, D. R., Donahue, N. M., and Baltensperger, U. (2014). Insight into Acid-Base Nucleation Experiments by Comparison of the Chemical Composition of Positive, Negative, and Neutral Clusters. Environ. Sci. Technol., 48:13675–13684.
- Biskos, G., Buseck, P. R., and Martin, S. T. (2009). Hygroscopic Growth of Nucleation-Mode Sulfate Particles. Aerosol Sci., 40:338–347.
- Bzdek, B. R., Ridge, D. P., and Johnston, M. V. (2010). Size-Dependent Reactions of Ammonium Bisulfate Clusters with Dimethylamine. J. Phys. Chem., 114:11638–11644.
- Chan, L. P., and Chan, C. K. (2013). Role of the Aerosol Phase State in Ammonia/Amines Exchange Reactions. Environ. Sci. Technol., 47:5755–5762.
- Cheng, Y., Su, H., Koop, T., Mikhailov, E., and Pöschl, U. (2015). Size Dependence of Phase Transitions in Aerosol Nanoparticles. Nat. Commun., 6:5923. doi:10.1038/ncomms6923
- Clegg, S. L., Pitzer, K. S., and Brimblecombe, P. (1992). Thermodynamics of Multicomponent, Miscible, Ionic Solutions. 2. Mixtures Including Unsymmetrical Electrolytes. J. Phys. Chem., 96:9470–9479.
- Clegg, S. L., Qiu, C., and Zhang, R. (2013). The Deliquescence Behaviour, Solubilities, and Densities of Aqueous Solutions of Five Methyl- and Ethyl-Aminium Sulphate Salts. Atmos. Environ., 73:145–158.
- Clegg, S. L., and Seinfeld, J. H. (2006a). Thermodynamic Models of Aqueous Solutions Containing Inorganic Electrolytes and Dicarboxylic Acids at 298.15 K. 1. The acids as Non-Dissociating Components. J. Phys. Chem. A, 110:5692–5717.
- Clegg, S. L., and Seinfeld, J. H. (2006b). Thermodynamic Models of Aqueous Solutions Containing Inorganic Electrolytes and Dicarboxylic Acids at 298.15 K. 2. Systems Including Dissociation Equilibria. J. Phys. Chem. A, 110:5718–5734.
- Duplissy, J., Enghoff, M. B., Aplin, K. L., Arnold, F., Aufmhoff, H., Avngaard, M., Baltensperger, U., Bondo, T., Bingham, R., Carslaw, K., Curtius, J., David, A., Fastrup, B., Gagné, S., Hahn, F., Harrison, R. G., Kellett, B., Kirkby, J., Kulmala, M., Laakso, L., Laaksonen, A., Lillestol, E., Lockwood, M., Mäkelä, J., Makhmutov, V., Marsh, N. D., Nieminen, T., Onnela, A., Pedersen, E., Pedersen, J. O. P., Polny, J., Reichl, U., Seinfeld, J. H., Sipilä, M., Stozhkov, Y., Stratmann, F., Svensmark, H., Svensmark, J., Veenhof, R., Verheggen, B., Viisanen, Y., Wagner, P. E., Wehrle, G., Weingartner, E., Wex, H., Wilhelmsson, M., and Winkler, P. M. (2010). Results from the CERN Pilot CLOUD Experiment. Atmos. Chem. Phys., 10:1635–1647.
- Duplissy, J., Merikanto, J., Franchin, A., Tsagkogeorgas, G., Kangasluoma, J., Wimmer, D., Vuollekoski, H., Schoberberger, S., Lehtipalo, K., Flagan, R. C., Brus, D., Donahue, N. M., Vehkamäki, H., Almeida, J., Amorim, A., Barmet, P., Bianchi, F., Breitenlechner, M., Dunne, E. M., Guida, R., Henschel, H., Junninen, H., Kirkby, J., Kürten, A., Kupc, A., Määttänen, A., Makhmutov, V., Mathot, S., Nieminen, T., Onnela, A., Praplan, A. P., Riccobono, F., Rondo, L., Steiner, G., Tome, A., Walther, H., Baltensperger, U., Carslaw, K. S., Dommen, J., Hansel, A., Petäjä, T., Sipilä, M., Stratmann, F., Vrtala, A., Wagner, P. E., Worsnop, D. R., Curtius, J., and Kulmala, M. (2016). Effect of Ions on Sulfuric Acid-Water Binary Particle Formation: 2. Experimental Data and Comparison with QC-Normalized Classical Nucleation Theory. J. Geophys. Res. Atmos., 121:1725–1775. doi:10.1002/2015JD023539.
- Dusek, U., Frank, G. P., Hildebrandt, L., Curtius, J., Schneider, J., Walter, S., Chand, D., Drewnick, F., Hings, S., Jung, D., Borrmann, S., and Andreae, M. O. (2006). Size Matters More than Chemistry for Cloud-Nucleating Ability of Aerosol Particles. Science, 312:1375–1378. doi:10.1126/science.1125261.
- Ehn, M., Junninen, H., Schobesberger, S., Manninen, H. E., Franchin, A., Sipilä, M., Petäjä, T., Kerminen, V.-M., Tammet, H., Mirme, A., Mirme, S., Hõrrak, U., Kulmala, M., and Worsnop, D. R. (2011). An Instrumental Comparison of Mobility and Mass Measurements of Atmospheric Small Ions. Aerosol Sci. Technol., 45:522–532.
- Fuchs,, N. A., and Sutugin, A. G. (1970). Highly Dispersed Aerosols. Ann Arbor Science Publishers, London.
- Ge, X., Wexler, A. S., and Clegg, S. L. (2011a). Atmospheric Amines – Part I. A Review. Atmos. Environ., 45:524–546.
- Ge, X., Wexler, A. S., and Clegg, S. L. (2011b). Atmospheric Amines – Part II. Thermodynamic Properties and Gas/Particle Partitioning. Atmos. Environ., 45:524–546.
- Henschel, H., Acosta Navarro, J. C., Yli-Juuti, T., Kupiainen-Määttä, O., Olenius, T., Ortega, I. K., Clegg, S. L., Kurtén, T., Riipinen, I., and Vehkamäki, H. (2014). Hydration of Atmospherically Relevant Molecular Clusters: Computational Chemistry and Classical Thermodynamics. J. Phys. Chem., 118(14):2599–2611.
- Hinds, W. C. (1999). Aerosol Technology: Properties, Behavior, and Measurements of Airborne Particles. 2nd ed. Wiley-Interscience, the United States of America.
- Hyvärinen, A. P., Lihavainen, H., Hautio, K., Raatikainen, T., Viisanen, Y., and Laaksonen, A. (2004). Surface Tensions and Densities of Sulfuric Acid + Dimethylamine + Water Solutions. J. Chem. Eng. Data, 49:917–922.
- IPCC: Climate Change. (2013). The Physical Science Basis, in Contribution of Working Group I to the Fifth Assessment Report of the Intergovernmental Panel on Climate Change, T. F. Stocker, D. Qin, G. -K. Plattner, M. Tignor, S. K. Allen, J. Boschung, A. Nauels, Y. Xia, V. Bex, and P. M. Midgley, eds., Cambridge University Press, Cambridge, United Kingdom and New York, NY, USA, pp. 571–657.
- Jen, C. N., McMurry, P. H., and Hanson, D. R. (2014). Stabilization of Sulfuric Acid Dimers by Ammonia, Methylamine, Dimethylamine, and Trimethylamine. J. Geophys. Res., 119:7502–7514.
- Junninen, H., Ehn, M., Petäjä, T., Luosujärvi, L., Kotiaho, T., Kostiainen, R., Rohner, U., Gonin, M., Fuhrer, K., Kulmala, M., and Worsnop, D. R. (2010). A High-Resolution Mass Spectrometer to Measure Atmospheric Ion Composition. Atmos. Meas. Tech., 3:1039–1053, doi:10.5194/amt-3-1039-2010.
- Kerminen, V.–M., and Kulmala, M. (2002). Analytical Formulae Connecting the “Real” and the “Apparent” Nucleation Rate and the Nuclei Number Concentration for Atmospheric Nucleation Events. J. Aerosol Sci., 33:609–622.
- Keskinen, H., Virtanen, A., Joutsensaari, J., Tsagkogeorgas, G., Duplissy, J., Schobesberger, S., Gysel, M., Riccobono, F., Slowik, J. G., Bianchi, F., Yli-Juuti, T., Lehtipalo, K., Rondo, L., Breitenlechner, M., Kupc, A., Almeida, J., Amorim, A., Dunne, E. M., Downard, A. J., Ehrhart, S., Franchin, A., Kajos, M. K., Kirkby, J., Kürten, A., Nieminen, T., Makhmutov, V., Mathot, S., Miettinen, P., Onnela, A., Petäjä, T., Praplan, A., Santos, F. D., Schallhart, S., Sipilä, M., Stozhkov, Y., Tomé, A., Vaattovaara, P., Wimmer, D., Prevot, A., Dommen, J., Donahue, N. M., Flagan, R. C., Weingartner, E., Viisanen, Y., Riipinen, I., Hansel, A., Curtius, J., Kulmala, M., Worsnop, D. R., Baltensperger, U., Wex, H., Stratmann, F., and Laaksonen, A. (2013). Evolution of Particle Composition in CLOUD Nucleation Experiments. Atmos. Chem. Phys., 13:5587–5600.
- Kim, J., Ahlm, L., Yli-Juuti, T., Lawler, M., Keskinen, H., Tröstl, J., Schobesberger, S., Duplissy, J., Amorim, A., Bianchi, F., Donahue, N. M., Flagan, R. C., Hakala, J., Heinritzi, M., Jokinen, T., Kürten, A., Laaksonen, A., Lehtipalo, K., Miettinen, P., Petäjä, T., Rissanen, M. P., Rondo, L., Sengupta, K., Simon, M., Tomé, A., Williamson, C., Wimmer, D., Winkler, P. M., Ehrhart, S., Ye, P., Kirkby, J., Curtius, J., Kulmala, M., Lehtinen, K. E. J., Smith, J. N., Riipinen, I., and Virtanen, A. (2016). Hygroscopicity of Nanoparticles Produced from Homogeneous Nucleation in the CLOUD Experiments. Atmos. Chem. Phys., 16:293–304.
- Kirkby, J., Curtius, J., Almeida, J., Dunne, E., Duplissy, J., Ehrhart, S., Franchin, A., Gagné, S., Ickes, L., Kürten, A., Kupc, A., Metzger, A., Riccobono, F., Rondo, L., Schobesberger, S., Tsagkogeorgas, G., Wimmer, D., Amorim, A., Bianchi, F., Breitenlechner, M., David, A., Dommen, J., Downard, A., Ehn, M., Flagan, R. C., Haider, S., Hansel, A., Hauser, D., Jud, W., Junninen, H., Kreissl, F., Kvashin, A., Laaksonen, A., Lehtipalo, K., Lima, J., Lovejoy, E. R., Makhmutov, V., Mathot, S., Mikkilä, J., Minginette, P., Mogo, S., Nieminen, T., Onnela, A., Pereira, P., Petäjä, T., Schnitzhofer, R., Seinfeld, J. H., Sipilä, M., Stozhkov, Y., Stratmann, F., Tomé, A., Vanhanen, J., Viisanen, Y., Vrtala, A., Wagner, P. E., Walther, H., Weingartner, E., Wex, H., Winkler, P. M., Carslaw, K. S., Worsnop, D. R., Baltensperger, U., and Kulmala, M. (2011). Role of Sulphuric Acid, Ammonia and Galactic Cosmic Rays in Atmospheric Aerosol Nucleation. Nature, 476:429–433.
- Kulmala, M., Laaksonen, A., and Pirjola, L. (1998). Parameterizations for Sulfuric Acid/Water Nucleation Rates. J. Geophys. Res., 103(D7):8301–8307.
- Kulmala, M., Petäjä, T., Nieminen, T., Sipilä, M., Manninen, H. E., Lehtipalo, K., Dal Maso, M., Aalto, P. P., Junninen, H., Paasonen, P., Riipinen, I., Lehtinen, K. E. J., Laaksonen, A., and Kerminen, V.–M. (2012). Measurement of the Nucleation of Atmospheric Aerosol Particles. Nature Protocols, 7(9):1651–1667.
- Kupc, A., Amorim, A., Curtius, J., Danielczok, A., Duplissy, J., Ehrhart, S., Walther, H., Ickes, L., Kirkby, J., Kürten, A., Lima, J. M., Mathot, S., Minginette, P., Onnela, A., Rondo, L., and Wagner, P. E. (2011). A Fibre-Optic UV System for H2SO4 Production in Aerosol Chambers Causing Minimal Thermal Effects. J. Aerosol Sci., 42:532–543.
- Kürten, A., Jokinen, T., Simon, M., Sipilä, M., Sarnela, N., Junninen, H., Adamov, A., Almeida, J., Amorim, A., Bianchi, F., Breitenlechner, M., Dommen, J., Doanahue, N. M., Duplissy, J., Ehrhart, S., Flagan, R. C., Franchin, A., Hakala, J., Hansel, A., Heinritzi, M., Hutterli, M., Kangasluoma, J., Kirkby, J., Laaksonen, A., Lehtipalo, K., Leiminger, M., Makhmutov, V., Mathot, S., Onnela, A., Petäjä, T., Praplan, A. P., Riccobono, F., Rissanen, M. P., Rondo, L., Schobesberger, S., Seinfeld, J. H., Steiner, G., Tomé, A., Tröstl, J., Winkler, P. M., Williamson, C., Wimmer, D., Ye, P., Baltensperger, U., Carslaw, K. S., Kulmala, M., Worsnop, D. R., and Curtius, J. (2014). Neutral Molecular Cluster Formation of Sulphuric Acid-Dimethylamine Observed in Real Time Under Atmospheric Conditions. P. Natl. Acad. Sci. USA, 111:15019–15024, doi:10.1073/pnas.1404853111.
- Kürten, A., Rondo, L., Ehrhart, S., and Curtius, J. (2011). Performance of a Corona Ion Source for Measurement of Sulfuric Acid by Chemical Ionization Mass Spectrometry. Atmos. Meas. Tech., 4:437–443, doi: 10.5194/amt-4-437-2011.
- Kurtén, T., Loukonen, V., Vehkamäki, H., and Kulmala, M. (2008). Amines are Likely to Enhance Neutral and Ion-Induced Sulfuric Acid-Water Nucleation in the Atmosphere More Efficiently than Ammonia. Atmos. Chem. Phys., 8:4095–4103.
- Lamarque, J.-F., Kyle, G., Meinshausen, M., Riahi, K., Smith, S., van Vuuren, D., Conley, A., and Vitt, F. (2011). Global and Regional Evolution of Short-Lived Radiatively-Active Gases and Aerosols in the Representative Concentration Pathways. Climatic Change, 109:191–212.
- Lawler, M. J., Winkler, P. M., Kim, J., Ahlm, L., Tröstl, J., Praplan, A. P., Schobesberger, S., Kürten, A., Kirkby, J., Bianchi, F., Duplissy, J., Hansel, T., Jokinen, T., Keskinen, H., Lehtipalo, K., Leiminger, M., Petäjä, T., Rissanen, M., Rondo, L., Simon, M., Sipilä, M., Williamson, C., Wimmer, D., Riipinen, I., Virtanen, A., and Smith, J. N. (in review). Unexpectedly Acidic Nanoparticles Formed in Dimethylamine-Ammonia-Sulfuric Acid Nucleation Experiments at CLOUD. Atmos. Chem. Phys. Disc., doi:10.5194/acp-2016-361.
- Lehtinen, K. E. J., and Kulmala, M. (2003). A Model for Particle Formation and Growth in the Atmosphere with Molecular Resolution in Size. Atmos. Chem. Phys., 3:251–257, doi:10.5194/acp-3-251-2003.
- Lehtipalo, K., Rondo, L., Kontkanen, J., Schobesberger, S., Jokinen, T., Sarnela, N., Kürten, A., Ehrhart, S., Franchin, A., Nieminen, T., Riccobono, F., Sipilä, M., Yli-Juuti, T., Duplissy, J., Adamov, A., Ahlm, L., Almeida, J., Amorim, A., Bianchi, F., Breitenlechner, M., Dommen, J., Downard, A. J., Dunne, E. M., Flagan, R. C., Guida, R., Hakala, J., Hansel, A., Jud, W., Kangasluoma, J., Kerminen, V. –M., Keskinen, H., Kim, J., Kirkby, J., Kupc, A., Kupiainen-Määttä, O., Laaksonen, A., Lawler, M. J., Leiminger, M., Mathot, S., Olenius, T., Ortega, I. K., Onnela, A., Petäjä, T., Praplan, A., Rissanen, M. P., Ruuskanen, T., Santos, F. D., Schallhart, S., Schnitzhofer, R., Simon, M., Smith, J. N., Tröstl, J., Tsagkogeorgas, G., Tomé, A., Vaattovaara, P., Vehkamäki, H., Vrtala, A. E., Wagner, P. E., Williamson, C., Wimmer, D., Winkler, P. M., Virtanen, A., Donahue, N. M., Carslaw, K. S., Baltensperger, U., Riipinen, I., Curtius, J., Worsnop, D. R., and Kulmala, M. (2016). The Effect of Acid-Base Clustering and Ions on the Growth of Atmospheric Nano-Particles. Nat. Commun., 7:11594 doi:10.1038/ncomms11594.
- Lewis, E. R. (2006). The Effect of Surface Tension (Kelvin Effect) on the Equilibrium Radius of a Hygroscopic Aqueous Aerosol Particle. Aerosol Sci., 37:1605–1617.
- Loukonen, V., Kurtén, T., Ortega, I. K., Vehkamäki, H., Pádua, A. A. H., Sellegri, K., and Kulmala, M. (2010). Enhancing Effect of Dimethylamine in Sulfuric Acid Nucleation in the Presence of Water – A Computational Study. Atmos. Chem. Phys., 10:4961–4974.
- Mäkelä, J. M., Yli-Koivisto, S., Hiltunen, V., Seidl, W., Swietlicki, E., Teinilä, K., Sillanpää, M., Koponen, I. K., Paatero, J., Rosman, K., and Hämeri, K. (2001). Chemical Composition of Aerosol During Particle Formation Events in Boreal Forest. Tellus, 53B:380–393.
- Makkonen, R., Asmi, A., Kerminen, V.–M., Boy, M., Arneth, A., Hari, P., and Kulmala, M. (2012). Air Pollution Control and Decreasing New Particle Formation Lead to Strong Climate Warming. Atmos. Chem. Phys., 12:1515–1524.
- McGrath, M. J., Olenius, T., Ortega, I. K., Loukonen, V., Paasonen, P., Kurtén, T., Kulmala, M., and Vehkamäki, H. (2012). Atmospheric Cluster Dynamics Code: A Flexible Method for Solution of the Birth-Death Equations. Atmos. Chem. Phys., 12:2345–2355.
- Merikanto, J., Napari, I., Vehkamaki, H, Anttila, T., and Kulmala, M. (2007). New Parameterization of Sulfuric Acid-Ammonia-Water Ternary Nucleation Rates at Tropospheric Conditions. J. Geophys. Res., 112:doi:10.1029/2006JD007977.
- Nielsen, C. J., Herrmann, H., and Weller, C. (2012). Atmospheric Chemistry and Environmental Impact of the use of Amines in Carbon Capture and Storage (CCS). Chem. Soc. Rev., 41:6684–6704.
- Nieminen, T., Lehtinen, K. E. J., and Kulmala, M. (2010). Sub-10 nm Particle Growth by Vapor Condensation – Effects of Vapor Molecule Size and Particle Thermal Speed. Atmos. Chem. Phys., 10:9773–9779.
- Olenius, T., Kupiainen-Määttä, O., Ortega, I. K., Kurtén, T., and Vehkamäki, H. (2013). Free Energy Barrier in the Growth of Sulfuric Acid-Ammonia and Sulfuric Acid-Dimethylamine Clusters. J. Chem. Phys., 139: 084312. doi:10.1063/1.4819024.
- Ortega, I. K., Kupiainen, O., Kurtén, T., Olenius, T., Wilkman, O., McGrath, M. J., Loukonen, V., and Vehkamäki, H. (2012). From Quantum Chemical Formation Free Energies to Evaporation Rates. Atmos. Chem. Phys., 12:225–235.
- Ortega, I. K., Olenius, T., Kupiainen-Määttä, O., Loukonen, V., Kurtén, T., and Vehkamäki, H. (2014). Electrical Charging Changes the Composition of Sulfuric Acid-Ammonia/Dimethylamine Clusters. Atmos. Chem. Phys., 14:7995–8007.
- Park, K., Kim, J., and Miller, A. L. (2009). A Study of Size and Structure on Hygroscopicity of Nanoparticles using a Tandem Differential Mobility Analyzer and TEM. J. Nanopart. Res., 11:175–183.
- Praplan, A. P., Bianchi, F., Dommen, J., and Baltensperger, U. (2012). Dimethylamine and Ammonia Measurements with Ion Chromatography During the CLOUD4 Campaign. Atmos. Meas. Tech., 5:2161–2167.
- Reischl, G. P. (1991). Measurement of Ambient Aerosols by the Differential Mobility Analyzer Method: Concepts and Realization Criteria for the Size Range between 2 and 500 nm. Aerosol Sci. Technol., 14:5–24.
- Rondo, L., Ehrhart, S., Kürten, A., Leiminger, M., Simon, M., Sipilä, M., Jokinen, T., Sarnela, N., Junninen, H., Adamov, A., Almeida, J., Amorim, A., Bianchi, F., Breitenlechner, M., Duplissy, J., Franchin, A., Hakala, J., Hansel, A., Heinritzi, M., Hutterli, M., Kangasluoma, J., Kirkby, J., Lehtipalo, K., Makhmutov, V., Mathot, S., Onnela, A., Petäjä, T., Praplan, A. P., Rissanen, M. P., Schobesberger, S., Steiner, G., Stratmann, F., Tomé, A., Tröstl, J., Wagner, P. E., Williamson, Ch., Wimmer, D., Ye, P., Baltensperger, U., Carslaw, K. S., Kulmala, M., Worsnop, D. R., and Curtius, J. (2016). Effect of Dimethylamine on the Gas Phase Sulfuric Acid Concentration Measured by Chemical Ionization Mass Spectrometry (CIMS). J. Geophys. Res, 121:3036–3049.
- Sauerwein, M., Clegg, S. L., and Chan, C. K. (2015). Water Activities and Osmotic Coefficients of Aqueous Solutions of Five Alkyl-Aminium Sulfates and their Mixtures with H2SO4 at 25 °C. Aerosol Sci. Technol., 49:566–579.
- Schobesberger, S., Franchin, A., Bianchi, F., Rondo, L., Duplissy, J., Kürten, A., Ortega, I. K., Metzger, A., Schnitzhofer, R., Almeida, J., Amorim, A., Dommen, J., Dunne, E. M., Ehn, M., Gagné, S., Ickes, L., Junninen, H., Hansel, A., Kerminen, V. –M., Kirkby, J., Kupc, A., Laaksonen, A., Lehtipalo, K., Mathot, S., Onnela, A., Petäjä, T., Riccobono, F., Santos, F. D., Sipilä, M., Tomé, A., Tsagkogeorgas, G., Viisanen, Y., Wagner, P. E., Wimmer, D., Curtius, J., Donahue, N. M., Baltensperger, U., Kulmala, M., and Worsnop, D. R. (2015). On the Composition of Ammonia-Sulfuric Acid Ion Cluster During Aerosol Particle Formation. Atmos. Chem. Phys., 15:55–78.
- Schobesberger, S., Junninen, H., Bianchi, F., Lönn, G., Ehn, M., Lehtipalo, K., Dommen, J., Ehrhart, S., Ortega, I. K., Franchin, A., Nieminen, T., Riccobono, F., Hutterli, M., Duplissy, J., Almeida, J., Amorim, A., Breitenlechner, M., Downard, A. J., Dunne, E. M., Flagan, R. C., Kajos, M., Keskinen, H., Kirkby, J., Kupc, A., Kürten, A., Kurtén, T., Laaksonen, A., Mathot, S., Onnela, A., Praplan, A. P., Rondo, L., Santos, F. D., Schallhart, S., Schnitzhofer, R., Sipilä, M., Tomé, A., Tsagkogeorgas, G., Vehkamäki, H., Wimmer, D., Baltensperger, U., Carslaw, K. S., Curtius, J., Hansel, A., Petäjä, T., Kulmala, M., Donahue, N. M., and Worsnop, D. R. (2013). Molecular Understanding of Atmospheric Particle Formation from Sulfuric Acid and Large Oxidized Organic Molecules. Proc. Natl. Aca. Sci., 110:17223–17228.
- Seinfeld, J. H., and Pandis, S. N. (2006). Atmospheric Chemistry and Physics: From Air Pollution to Climate Change. 2nd ed. John Wiley & Sons, Inc., New Jersey.
- Smith, J. N., Barsanti, K. C., Friedli, H. R., Ehn, M., Kulmala, M., Collins, D. R., Scheckman, J. H., Williams, B. J., and McMurry, P. H. (2010). Observations of Aminium Salts in Atmospheric Nanoparticles and Possible Climatic Implications. PNAS, 107:6634–6639.
- Smith, J. N., Moore, K. F., McMurry, P. H., and Eisele, F. L. (2004). Atmospheric Measurements of sub-20 nm Diameter Particle Chemical Composition by Thermal Desorption Chemical Ionization Mass Spectrometry. Aerosol Sci. Technol., 38(2):100–110.
- Spracklen, D. V., Pringle, K. J., Carslaw, K. S., Chipperfield, M. P., and Mann, G. W. (2005). A Global off-line Model of Size-Resolved Aerosol Microphysics: I. Model Development and Prediction of Aerosol Properties. Atmos. Chem. Phys., 5:2227–2252.
- Stumm, W., and Morgan, J. J. (1996). Aquatic Chemistry: Chemical Equilibria and Rates in Natural Waters, Wiley, New York.
- Vehkamaki, H., Kulmala, M., Napari, I., Lehtinen, K. E. J., Timmreck, C., Noppel, M., and Laaksonen, A. (2002). An Improved Parameterization for Sulfuric Acid-Water Nucleation Rates for Tropospheric and Stratospheric Conditions. J. Geophys. Res., 107:doi:10.1029/2002JD002184.
- Voigtlander, J., Duplissy, J., Rondo, L., Kurten, A., and Stratmann, F. (2012). Numerical Simulations of Mixing Conditions and Aerosol Dynamics in the CERN CLOUD Chamber. Atmos. Chem. Phys., 12:2205–2214.
- Weber, R. J., Marti, J. J., McMurry, P. H., Eisele, F. L., Tanner, D. J., and Jefferson, A. (1997). Measurements of New Particle Formation and Ultrafine Particle Growth Rates at a Clean Continental Site. J. Geophys. Res., 102:4375–4385.
- Weber, R. J., McMurry, P. H., Eisele, F. L., and Tanner, D. J. (1995). Measurement of Expected Nucleation Precursor Species and 3–500 nm Diameter Particles at Mauna Loa Observatory, Hawaii. J. Atmos. Sci., 52:2242–2257.
- Weber, R. J., Guo, H., Russell, A. G., and Nenes, A. (2016). High Aerosol Acidity Despite Declining Atmospheric Sulfate Concentrations Over the Past 15 Years. Nat. Geosci., 9:282–286.
- Wexler, A. S., and Clegg, S. L. (2002). Atmospheric Aerosol Models for Systems Including the Ions H+, NH4+, Na+, SO42-, NO3-, Cl-, Br- and H2O. J. Geophys. Res., 107:doi:10.1029/2001JD000451.
- Yli-Juuti, T., Barsanti, K., Hildebrandt Ruiz, L., Kieloaho, A.–J., Makkonen, U., Petäjä, T., Ruuskanen, T., Kulmala, M., and Riipinen, I. (2013). Model for Acid-Base Chemistry in Nanoparticle Growth (MABNAG). Atmos. Chem. Phys., 13:12507–12524.
- Yu, F. Q., and Turco, R. P. (2000). Ultrafine Aerosol Formation Via Ion-Mediated Nucleation. Geophys. Res. Lett., 27:883–886.
- Yu, H., McGraw, R., and Lee, S.–H. (2012). Effects of Amines on Formation of sub-3 nm Particles and Their Subsequent Growth. Geophys. Res. Lett., 39:doi:10.1029/2011GL050099.
- Zordan, C. A., Pennington, M. R., and Johnston, M. V. (2010). Elemental Composition of Nanoparticles with the Nano Aerosol Mass Spectrometer. Anal. Chem., 82(19):8034–8038.