ABSTRACT
In this article, a proof of concept of a new measurement instrument, differential diffusion analyzer (DDA), is established. The DDA enables the measurement of the size distribution of sub-10 nm aerosol particles, and it can also be used as a size classifier to separate a certain particle size from a size distribution for subsequent analysis. The developed technique is based on the diffusion separation of different size particles. Thus, the main advantage of the DDA compared to other methods is that particle charging is not required. Simulated and experimentally measured transmission efficiencies show that the diffusion-based differential size classification is a feasible concept, and moreover, shows that particle size is inversely proportional to the square root of the total flow rate.
Copyright © 2017 American Association for Aerosol Research
EDITOR:
1. Introduction
Aerosol particles in the sub-10 nm size range are of special interest in studies of new particle formation mechanisms of both natural and anthropogenic aerosols. Nucleation events frequently produce new particles in the atmosphere (Kulmala et al. Citation2004). Combustion sources, such as vehicle engines, produce particles within the same size range, formed either during exhaust gas cooling (Schneider et al. Citation2005) or already in the engine (Rönkkö et al. Citation2007). For both the natural and anthropogenic cases, the interest in particle diameter reaches down to cluster sizes, below 3 nm (Kulmala et al. Citation2013; Alanen et al. Citation2015). Thus, there is a need for new size fractionating instruments with a size range extending below 3 nm where the particle formation occurs (Kirkby et al. Citation2016).
Condensation particle counters (CPCs, see McMurry Citation2000) readily enable the number concentration measurement of particles larger than an instrument-specific lowest detectable size. Following the development work on, e.g., the working liquids (Okuyama et al. Citation1984, Iida et al. Citation2009, Fernandez de la Mora Citation2011), the lowest detectable size of recent commercial designs has reached well below 2 nm (Iida et al. Citation2009; Vanhanen et al. Citation2011). Particle size distribution can be measured with the CPC technique by using pulse height analysis (Saros et al. Citation1996) or a mixing type CPC with a variable mixing rate (Vanhanen et al. Citation2011). However, the particle growth activation and consequently the sizing are dependent on the particle material.
To facilitate less particle material-dependent size distribution measurement, a size classification instrument can be placed in series with a particle-sensing instrument, such as a CPC. The most widely used classification instrument is the differential mobility analyzer (DMA, Knutson and Whitby Citation1975) that can be used either in mobility stepping (Hoppel Citation1978) or scanning mode (Wang and Flagan Citation1990). The main advantage of the DMA is the high size resolution achievable. High size resolution can even be maintained down to 1 nm by improved DMA designs. Those interested in these developments are referred to Fernandez de la Mora (Citation2011) and the references therein. The fundamental limitation of this technique is that the particles need to be charged before entering the DMA and the charging process becomes inefficient for the smallest particles. The fraction of particles charged in the customary bipolar charger can be obtained from the treatment of Wiedensohler (Citation1998). The fraction of particles with a single charge of one polarity drops below 1% as the particle diameter goes below approximately 3 nm. The fraction also becomes somewhat dependent on the composition of the carrier gas (Steiner and Reischl Citation2012). Furthermore, the particle charging interrupts the size distribution measurement with the smallest particles as the mobility size of the ions escaping from a charger overlap with the size spectrum of the particles in the sub-3 nm size range (see, e.g., Steiner et al. Citation2014).
Another size classification instrument well suited for sub-10 nm particle sizes is the diffusion battery (see Knutson Citation1999). It was first combined with a CPC to measure the size of aerosol particles by Nolan and Guerrini (Citation1936). The idea of obtaining the size distribution was introduced by Pollak and Metnieks (Citation1957). The introduction of the wire screen type diffusion battery by Sinclair and Hoopes (Citation1975) and the theoretical scheme to predict its collection efficiency (Cheng and Yeh Citation1980) have enabled the construction of compact diffusion batteries. Typically, sample flow to a CPC is taken sequentially after increasing the number of diffusion stages (e.g., Sinclair et al. Citation1979). Aerosol penetration through the consequential stages results in integral, or cumulative, type of size distribution data. Differential data can, in principle, be obtained by subtracting the values of consecutive stages, but the sample aerosol needs to be very stable during the measurement sequence. Otherwise, the cumulative principle produces a noisy distribution, especially when a majority of the particles are larger than the limited classification range achievable.
To measure in real-time with the diffusion battery, Fierz et al. (Citation2002) combined the electrical detection of particles with the diffusion battery concept in a miniaturized diffusion size classifier. This principle produces differential data. However, because of the low resolution and high overlap of the diffusion stages, the data need in practice to be treated using an inversion algorithm (see Knutson Citation1999). The diffusion battery offers size classification according to a well-defined and relevant particle size concept. The drawbacks include rather limited size resolution and size range. Further, the cumulative classification principle does not facilitate sampling of particles of a limited size range.
In this work, we present a new differential diffusion analyzer (DDA). According to our knowledge, this is the first time diffusion has been utilized to achieve a differential size classification of airborne particles. First, we explore on theoretical basis the operation and the performance of the DDA. Second, we prove numerically and experimentally the feasibility of the DDA concept and study the scaling of the output particle size distribution and its width with flow rates.
2. Theoretical background and operation
2.1. Principle of operation
Einstein's celebrated work (Einstein Citation1905) related the Brownian motion to diffusion and the diffusion coefficient of a particle to its diameter. Using the currently prevalent notation, this relation can be expressed as[1] where
is the Boltzmann's constant,
is the temperature,
is the Cunningham slip correction,
is the gas viscosity, and
is the particle size. The equation effectively includes a definition for particle mobility diameter, giving a one-to-one relation between the diameter and the diffusion coefficient. The text below first operates with the diffusion coefficient.
Let us consider a textbook (e.g., Friedlander Citation2000) example of diffusion in one dimension: a particle sheet is initially (t = 0) released at x = 0. The concentration (#/m) at location at time
is given by the solution of the one-dimensional diffusion equation as
[2] where D is the diffusion coefficient, x is the location, t is the time, and
is the initial concentration profile of particles. An interesting feature of this solution is that at fixed position and time coordinates this function has a maximum for a certain diffusion coefficient value. The maximum is achieved at
[3]
shows the concentration as a function of the diffusion coefficient for = 1 cm and
= 10 s. The existence of this maximum can be applied to build a device that has a differential transfer function with respect to the particle diffusion coefficient. We call this device a differential diffusion analyzer (DDA).
Figure 1. The solution of a one-dimensional diffusion equation for x = 1 cm and t = 10 s. On the vertical axis is the concentration according to EquationEquation (2[2] ) and on the horizontal axis is the diffusion coefficient.
![Figure 1. The solution of a one-dimensional diffusion equation for x = 1 cm and t = 10 s. On the vertical axis is the concentration according to EquationEquation (2[2] ) and on the horizontal axis is the diffusion coefficient.](/cms/asset/83a6c343-d490-4c0d-a2aa-e19986fab378/uast_a_1367089_f0001_b.gif)
A one-dimensional DDA could be constructed as schematically presented in : a narrow sheet of particles is produced by a laminar sample aerosol flow through a narrow slit, sandwiched between two wide, laminar sheath gas flows. The time in EquationEquation (2
[2] ) is then dependent only on the distance traveled in the vertical (flow) direction, while
is directly the displacement of the particles in the horizontal (normal to the flow) direction. The flow system is completed with a flow for the classified particles and the excess flow. The classified particle flow can be extracted at a certain distance from the centerline or, as in the figure, from the wall limiting the flow.
It is apparent that the mechanical construction and the flow system are in principle similar to a DMA, but the scaling is different, as the residence time in the classification zone needs to be longer than in the case of electrical classification of same-sized particles. The DDA can be realized also using cylindrical symmetry. In fact, a normal DMA can be used with zero electric field. The popular design where sample flow is introduced at the outer wall of the annular space is however not the optimal solution with respect to transmission efficiency. This is because the Brownian motion is a stochastic phenomenon, and it spreads out particles radially in both directions when a DMA is used as a DDA. Consequently, particles that diffuse toward the outer wall and deposit on it are lost from the sample flow.
2.2. Theoretical transfer function
A better understanding on the capabilities of the DDA can be obtained by building a more accurate model that predicts the transfer function with realistic boundary conditions. The equation is[4] and the related boundary conditions are of the form:
[5] where
is the concentration,
and
are the locations of the DDA walls, which serve as a perfect sink for particles,
and
are the edges of the polydisperse flow,
is the diffusion coefficient of each particle size, and
is the initial concentration profile. illustrates the model and its parameters. The initial concentration is 1 in the polydisperse flow region and 0 in the sheath flow.
Figure 3. Illustration of the numerical model and its boundary conditions presented in EquationEquations (4[4] )–(7).
![Figure 3. Illustration of the numerical model and its boundary conditions presented in EquationEquations (4[4] )–(7).](/cms/asset/e23f33b2-c7c8-491f-b882-86b8dd495fcd/uast_a_1367089_f0003_b.gif)
The transfer function for each particle size was calculated numerically by integrating the concentration over the sample outflow:[6] where
is the transfer or kernel function,
,
, and
are the integration limits presented in , and
is the average residence time of the particles in the DDA (classifier volume divided with the total volumetric flow rate). Moreover, the size-classified sample flows are extracted from both walls to increase the instrument transmission efficiency, and the coefficient of two in front of the right-hand side of EquationEquation (6
[6] ) follows from the symmetry of the case. The integration limits
and
were calculated
[7] where W is the width of the DDA,
is the sample out flow (the sum of two sample out flows),
is the total flow, and the sheath flow is equal to the excess flow. In the latter equation, a laminar flow with uniform velocity profile and a balance of the sheath and the excess flows are assumed.
presents the numerically solved transfer functions for a planar DDA with different sample to sheath flow ratios. The residence time is same (= 3.3 s) in all the solved cases and the sample to sheath flow ratio was varied from 0.2 to 0.9. For comparison, the collected fraction onto a stage of a screen-type diffusion battery (Dubtsov et al. Citation2017) and the equilibrium charge distribution for singly charged particles are also shown (Wiedensohler Citation1998). Theoretical curves show that the flow ratio determines the width and the maximum value of the transmission function. Smaller the flow ratio, the narrower is the transmitted particle size range and the transmission efficiency. Compared to the deposited fraction function of a screen-type diffusion battery, the transmission efficiency curve of the DDA is more narrow for flow ratios up to 0.9. In the case of a planar DDA, the limiting flow ratio is 1 because all the sheath flow is then aspirated to the sample outflow. Flow ratios above unity produce a ‘‘tail’’ for the larger particle sizes and the shape of the DDA transmission efficiency function approaches the deposited fraction of the diffusion battery stage. As already mentioned, the residence time is constant for all flow ratios shown in . The transmitted particle size increases with increasing flow ratio because the diffusion distance between the sample in and the sample out flows shortens with the increasing sample to sheath flow ratio.
Figure 4. (a) Theoretical DDA transmission functions for different sample to sheath flow ratios (solid lines), the charge fraction of singly charged particles in equilibrium (dashed line, Wiedensohler Citation1998), and the collected particle fraction for the second stage of a two-stage screen diffusion battery (gray line, Dubtsov et al. Citation2017). Numbers adjacent to curves represent the ratio of the sample to sheath flow. (b) Theoretical transmission functions for different flow ratios as a function of the diffusion coefficient for the DDA and a DMA. The DMA dimensions correspond to the TSI's Nano DMA, and the DDA parameters used in the model were the same as in Figure (a). The DMA transmission efficiency was calculated according to the theory presented by Stolzenburg (Citation1988).
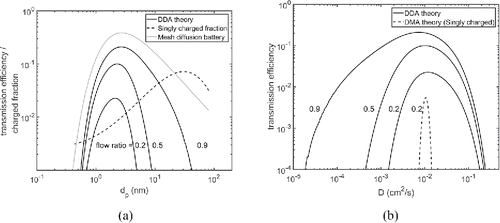
The transmission efficiency of the planar DDA depends on the chosen flow ratio and is a trade-off between the instrument size resolution and the transmission efficiency. Theoretically, the transmitted particle size can be selected independently of the instrument transmission efficiency because the flow ratio determines the diffusion distance between the sheath and the sample flows, and consequently, the maximum transmission efficiency. For the flow ratio of 0.5, that is, the sheath flow is twice the sample flow rate, the maximum transmission value is approximately three times higher than the equilibrium charge level at 10 nm and estimated to be 10 times higher at 2.7 nm. For the flow ratio of 0.2, the transmission efficiency of the DDA is equal with the DMA-based techniques at 6 nm, as the charging efficiency ultimately limits the achievable transmission efficiency of the DMA. Thus, the DDA has potential to provide significant improvement in the transmission efficiency compared to the DMA technique where particles have to be charged prior to the size classification and in the size resolution when compared to diffusion batteries in the sub-10 nm size range.
presents the DDA transmission efficiency curves shown in as a function of the diffusion coefficient and the transmission efficiency of a nanoparticle optimized DMA is also shown (TSI's Nano DMA, 15 lpm sheath flow, 3 lpm sample flow, voltage 100 V). The DMA transmission efficiency is the overall transmission efficiency of the DMA and the neutralizer. The DMA transmission was modeled according to Stolzenburg (Citation1988), and the bipolar charging probability of singly charged particles was calculated according to Wiedensohler (Citation1998). The figure shows that the resolution of the DDA is modest compared to that of an optimized DMA, however, it also shows that the transmission efficiencies above the particle charging limit can be attained with the DDA.
2.3. Proof of concept
2.3.1 Instrument
To prove the DDA concept, we used the TSI's Long DMA as a DDA (Model 3071A, inner radius 0.937 cm, outer radius 1.958 cm, and length of the classification region 44.44 cm). The central electrode was grounded to the housing to obviate any electric field between the inner and outer electrodes. The sheath and sample flow rates were varied to change the penetrating particle size, and the flows were set by mass flow controllers in a closed loop. The TSI long DMA was selected because it was readily available and it produces the required relatively long residence times without using unreasonably low flow rates. However, there are also known issues with nanoparticle penetration (e.g., Kousaka et al. Citation1986); the inlet part and the electrical insulation at the end of the DMA classifying section both introduce large nanoparticle losses.
2.3.2 CFD simulations
The transmission functions of the DDA were simulated with the ANSYS Fluent 17.1, computational fluid dynamics (CFD) software. Because the device Reynolds numbers lay in the range of 10–100, the flow was assumed to be laminar. A two-dimensional axisymmetric model was employed, and aerosol concentration was modeled in an Eulerian-fashion by solving the scalar transport equation for each particle size separately, according to the following equations:[8] where
is the particle concentration of the particle size i, and
is the diffusion coefficient calculated with the Stokes–Einstein relation (Einstein Citation1905). Furthermore, the particle sizes were modeled by altering the diffusion coefficient of the transported scalar, and the boundary condition was zero concentration at the walls. Thus, the walls served as a perfect sink for aerosol particles. Only diffusive and convective mass transport mechanisms were modeled.
The computation grid consisted of tetrahedral and hexagonal elements, and in order to fully resolve the concentration in the boundary layers at the solid walls, the smallest element size in the grid was set to 10 µm. The grid independence of the results was also assured by adapting the grid several times to the wall boundaries until the grid independence was reached.
shows a simulated concentration profile for 2, 5, and 25 nm particles in the DDA for a sheath flow rate of 0.96 lpm and a sample flow rate of 0.32 lpm. In addition, the dashed lines inside the contour plots indicate the last streamline that ends up onto the sample flow. shows the tendency of the 2 nm particles to diffuse to the walls of the inlet, resulting in a negligible portion that ends up in the sample outlet. shows that a large portion of the 5 nm particles are also lost to the walls in the DDA, but it also points out that a significant portion of particles diffuses beyond the sample flow limiting streamline, and thus ends up into the sample outlet. Besides the particle wall losses, particle concentration reduces also because of the dilution taking place when the particles migrate over the initially particle-free sheath flow.
Figure 5. Concentration profiles in the half-cross section of the DDA for 2 nm (a), 5 nm (b), and 25 nm (c) particles. The sample flow rate is 0.32 lpm and sheath flow rate is 0.96 lpm. The concentration is normalized to the unity at the inlet.
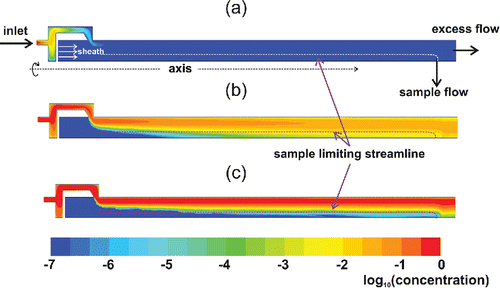
shows that for 25 nm particles, the residence time in the DDA classification regime is too short for them to reach the sample outlet. Based on , we conclude that diffusion separation is possible with a cylindrical DMA, and that the transmission efficiency function is differential for the sample to sheath flow rate ratio of 1/3.
2.4. Experimental tests
shows a schematic illustration of the calibration setup of the DDA. Monodisperse 2–20 nm silver particles were generated with the evaporation–condensation method (Scheibel and Porstendörfer Citation1983, Harra et al. Citation2012). Moreover, the particles were charged with a corona charger (Arffman et al. Citation2014) and subsequently classified with a NanoDMA (model 3085, TSI Inc., USA), and thereafter conducted to the DDA. Particles could be optionally directed through a Krypton-neutralizer and an electrostatic precipitator (ESP) before entering the DDA to remove all charged particles. The controlling particle transfer mechanism inside the DMA was diffusion, as the DMA central electrode was grounded to the outer cylinder. The transmission function of the DDA was measured with a particle size magnifier (PSM, covers both the Airmodus’ PSM and the A20 CPC), and for larger particles with the TSI's 3025 CPC. The transmission function was calculated from the ratio of the particle concentrations before and after the DDA.
2.5. Measured and simulated transmission functions
shows the measured and CFD simulated transmission as a function of the particle size for the sheath and sample flow rates of 0.48 lpm and 0.16 lpm, respectively. The transmission is reduced by the particle losses in the tubings before and after the DDA, and thus, approximately 20 cm piece of tube was added to the CFD simulations after the DDA. However, this mainly affects the peak height of the simulated transmission curve. The maximum transmission was found at a particle size of approximately 7.8 nm, which was also predicted by the simulations. The maximum transmission is low, because, especially the inlet of the used DDA is very effective trap for the nanoparticles.
Figure 7. Measured and CFD simulated transmission efficiency of the DDA for the sheath flow of 0.48 lpm and sample flow of 0.16 lpm.
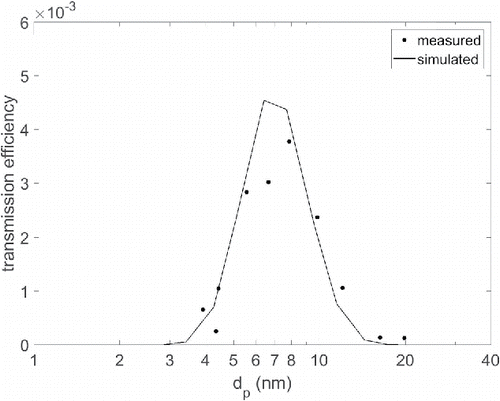
shows the scaling of the output particle size distribution as a function of the flow rates. The dots represent measured and the lines simulated values. These parameters were obtained by fitting a log-normal size distribution to the measurement data. shows the geometric mean diameter (GMD) of the output particle size distribution as a function of the total flow rate (sheath + sample flow). Results indicate that the output particle size is inversely proportional to the square root of the total flow rate. It was also confirmed that the electrical charge of the particles has no effect on the output particle size by measuring singly charged and absolute neutral particles.
Figure 8. (a) Geometric mean diameter (GMD) of the output particle size distribution from the DDA as a function of the total flow rate (for Qsample/Qsheat = 0.33). (b) Geometric standard deviation (GSD) of the output particle size distribution as a function of the aerosol to sheath flow ratio. (c) Transmission efficiency as a function of the sample to sheath flow ratio. Different symbols represent the charge state of particles and the solid and the dashed lines represent the CFD and analytical model results, respectively. The analytical model was calculated based on Equation (3).
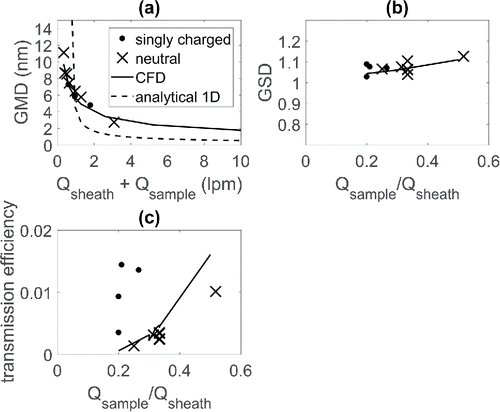
and shows the geometric standard deviation and the transmission efficiency as a function of the sample to sheath flow ratio. Simulated and experimental results of show that the width of the transmission function increases slightly with increasing flow ratio, as expected. shows that the transmission efficiency maximum value increases with increasing flow ratio as was also expected, but surprisingly, there is a significant difference between the charged and the neutral particles. The particle concentration in the measurement was rather low and, thus we do not expect that the space charge phenomena could have significant effect on the transmission efficiency. Instead, the explanation could be that there is an electrically insulating part in the DMA that is seen by the particles at the inlet or the outlet. This insulator surface can be charged by the deposited particles, or by some other processes, creating an electrostatic repulsion between the particles and the insulator, and therefore reduce particle losses. Measured transmission efficiency of uncharged particles and the simulated values were quite close to each other, although, there was some deviation in the results.
As shown in , the maximum transmission was found to be quite modest for the DDA when a conventional DMA was used for the diffusion classification, only 0.1%–1.4%. However, we estimate that much higher transmission efficiencies can be achieved by designing an instrument that is optimized for diffusion classification. The future instruments should be designed to minimize losses and maximize separation for sub-5 nm particles; thus, the transmission efficiency of the DDA would become more competitive with the DMA, as the particle charging probability with the existing chargers is usually very low (see Hernandez-Sierra et al. Citation2003; Alonso and Huang Citation2015). Besides the transmission efficiency, the uncertainty in the charging process in this size range is one of the biggest obstacles in the electrical mobility spectrometry, as discussed in a review by Li et al. (Citation2015).
3. Summary and discussion
In this study, we devised and tested a conceptually new instrument called the differential diffusion analyzer (DDA). We showed that the DDA has a differential transmission efficiency function with respect to particle size; according to our knowledge, this is the first time a differential airborne particle size classification has been realized with a diffusion-based instrument. The DDA can be used in a similar fashion as a DMA to size classify particles, or, when combined with a particle counter, to measure the particle size distribution. The DDA classifies particles according to their diffusion coefficient that has a well-defined and unambiguous connection to the mobility particle size. A great advantage of this technique is that electrically neutral particles can be classified. Thus, there is no need for a charger/neutralizer or a high voltage source, and consequently the whole construction can be kept extremely simple.
The one-dimensional diffusion equation reveals the presence of a maximum in the transmission with respect to the particle diffusivity for the fixed spatial and temporal coordinates. This maximum makes it possible to design an instrument that can differentially classify particles according to their diffusion coefficient, which can be used to infer particle size calculated via the Stokes–Einstein equation. Furthermore, we showed that in the sub-10-nm size range, higher transmission efficiency is attainable in a planar DDA than in an electrical mobility-based classification. For example, at 2 nm, the theoretical maximum penetration of a planar DDA was found to be 10% (for sample to sheath flow ratio of 0.5), while the DMA is limited to transmission efficiencies less than 1% due to the low particle charging probability.
We proved the concept of a differential diffusion analyzer both experimentally and by numerical simulation by operating an existing DMA (the TSI model 3071A) with reduced flow rates as a DDA. Moreover, the measured and CFD simulated transmission functions for the TSI's 3071A DMA showed how the output particle size varies with the total flow rate. We found that when the total flow rate was varied from 0.3 to 3.1 lpm, the output particle size varied from 11 to 3 nm, correspondingly. The aerosol-to-sheath flow ratio was maintained at 0.33. We also found that when the aerosol-to-sheath flow ratio was varied in the range of 0.2–0.5, the GSD of the output particle size distribution varied in the range of 1.03–1.13, and the particle transmission efficiency peak value in the range of 0.1%–1.4%. Although, the transmission efficiency was found to be quite modest when a cylindrical DMA was used for the diffusion classification, the peak transmission efficiency was found to be comparable to that of electrical size classification because of the low charging probability in the size range of 1–5 nm. Moreover, in the DDA technique, uncertainties related to charging efficiency are not present, which makes it an attractive option when small nanoparticles or molecular clusters are in question. The TSI long column, cylindrical DMA is not an optimal solution when classifying particles by diffusion because a large portion of the particles are lost to the outer or inner walls. This can be circumvented by designing the instrument in a way that is more optimal for diffusion classification.
References
- Alanen, J., Saukko, E., Lehtoranta, K., Murtonen, T., Timonen, H., Hillamo, R., Karjalainen, P., Kuuluvainen, H., Harra, J., Keskinen, J., and Rönkkö, T. (2015). The Formation and Physical Properties of the Particle Emissions From a Natural Gas Engine. Fuel, 162:155–161.
- Alonso, M., and Huang, C. H. (2015). High-Efficiency Electrical Charger for Nanoparticles. J. Nanopart. Res., 17:332.
- Arffman, A., Yli-Ojanperä, J., Kalliokoski, J., Harra, J., Pirjola, L., Karjalainen, P., Rönkkö, T., and Keskinen, J. (2014). High-Resolution Low-Pressure Cascade Impactor. J. Aerosol Sci., 78:97–109.
- Chen, D. R., Pui, D. Y. H., Hummes, D., Fissan, H., Quant, F. R., and Sem, G. J. (1998). Design and Evaluation of a Nanometer Aerosol Differential Mobility Analyzer (Nano-DMA). J. Aerosol Sci., 29:497–509.
- Cheng, Y. S. (2011). Instruments and Samplers Based on Diffusional Separation, in Aerosol Measurement: Principles, Techniques, and Applications, P. Kulkarni, P. A. Baron, and K. Willeke, eds., John Wiley & Sons, New York, pp. 365–379.
- Cheng, Y. S., and Yeh, H. C. (1980). Theory of a Screen-Type Diffusion Battery. J. Aerosol Sci., 11:313–320.
- Dubtsov, S., Ovchinnikova, T., Valiulin, S., Chen, X., Manninen, H. E., Aalto, P. P., and Petäjä, T. (2017). Laboratory Verification of Aerosol Diffusion Spectrometer and the Application to Ambient Measurements of New Particle Formation. J. Aerosol Sci., 105:10–23.
- Einstein, A. (1905). Über die von der molekulakinetischen Theorie der Wärme geforderte Bewegung von in ruhenden Flüssigkeiten suspendierten Teilchen. Ann. Phys., 17:549–560.
- Fernandez de la Mora, J. (2011). Electrical Classification and Condensation detection of Sub-3 nm Aerosols, in Aerosol Measurement: Principles, Techniques, and Applications, P. Kulkarni, P. A. Baron, and K. Willeke, eds., John Wiley & Sons, New York, pp. 697–721.
- Fierz, M., Scherrer, L., and Burtscher, H. (2002). Real-Time Measurement of Aerosol Size Distributions with an Electrical Diffusion Battery. J. Aerosol Sci., 33(7):1049–1060.
- Friedlander, S. K. (2000). Smoke, Dust, and Haze: Fundamentals of Aerosol Dynamics, 2nd ed. Oxford University Press, New York, pp. 30–31.
- Harra, J., Mäkitalo, J., Siikanen, R., Virkki, M., Genty, G., Kobayashi, T., Kauranen, M., and Mäkelä, J. M. (2012). Size-Controlled Aerosol Synthesis of Silver Nanoparticles for Plasmonic Materials. J. Nanopart. Res., 14(6):870.
- Hernandez-Sierra, A., Alguacil, F. J., and Alonso, M. (2003). Unipolar Charging of Nanometer Aerosol Particles in a Corona Ionizer. J. Aerosol Sci., 34(6):733–744.
- Hoppel, W. A. (1978). Determination of the Aerosol Size Distribution from the Mobility Distribution of the Charged Fraction of Aerosols. J. Aerosol Sci., 9:41–54.
- Iida, K., Stolzenburg, M. R., and McMurry, P. H. (2009). Effect of Working Fluid on Sub-2 nm Particle Detection with a Laminar Flow Ultrafine Condensation Particle Counter. Aerosol Sci. Technol., 43(1):81–96.
- Kirkby, J., Duplissy, J., Sengupta, K., Frege, C., Gordon, H., Williamson, C., Heinritzi, M., Simon, M., Yan, C., Almeida, J., Tröstl, J., Nieminen, T., Ortega, I. K., Wagner, R., Adamov, A., Amorim, A., Bernhammer, A.-K., Bianchi, F., Breitenlechner, M., Brilke, S., Chen, X., Craven, J., Dias, A., Ehrhart, S., Flagan, R. C., Franchin, A., Fuchs, C., Guida, R., Hakala, J., Hoyle, C. R., Jokinen, T., Junninen, H., Kangasluoma, J., Kim, J., Krapf, M., Kürten, A., Laaksonen, A., Lehtipalo, K., Makhmutov, V., Mathot, S., Molteni, U., Onnela, A., Peräkylä, O., Piel, F., Petäjä, T., Praplan, A. P., Pringle, K., Rap, A., Richards, N. A. D., Riipinen, I., Rissanen, M. P., Rondo, L., Sarnela, N., Schobesberger, S., Scott, C. S., Seinfeld, J. H., Sipilä, M., Steiner, G., Stozhkov, Y., Stratmann, F., Tomé, A., Virtanen, A., Vogel, A. L., Wagner, A. C., Wagner, P. E., Weingartner, E., Wimmer, D., Winkler, P. M., Ye, P., Zhang, X., Hansel, A., Dommen, J., Donahue, N. M., Worsnop, D. R., Baltensperger, U., Kulmala, M., Carslaw, K. S., and Curtius, J. (2016). Ion-Induced Nucleation of Pure Biogenic Particles. Nature, 533:521–526.
- Knutson, E. O. (1999). History of Diffusion Batteries in Aerosol Measurements. Aerosol Sci. Technol., 31(2–3):83–128.
- Knutson, E. O., and Whitby, K. T. (1975). Aerosol Classification by Electric Mobility: Apparatus, Theory, and Applications. J. Aerosol Sci., 6(6):443–451.
- Kousaka, Y., Okuyama, K., Adachi, M., and Mimura, T. (1986). Effect of Brownian Diffusion on Electrical Classification of Ultrafine Aerosol Particles in Differential Mobility Analyzer. J. Chem. Eng. Jpn., 19(5):401–407.
- Kulkarni, P., Baron, P. A., Willeke, K., Dhaniyala, S., Fierz, M., Keskinen, J., and Marjamäki, M. (2011). Instruments Based on Electrical Detection of Aerosols, in Aerosol Measurement: Principles, Techniques, and Applications, P. Kulkarni, P. A. Baron, and K. Willeke, eds., John Wiley & Sons, New York, p. 398.
- Kulmala, M., Kontkanen, J., Junninen, H., Lehtipalo, K., Manninen, H. E., Nieminen, T., Petäjä, T., Sipilä, M., Schobesberger, S., Rantala, P., Franchin, A., Jokinen, T., Järvinen, E., Äijälä, M., Kangasluoma, J., Hakala, J., Aalto, P. P., Paasonen, P., Mikkilä, J., Vanhanen, J., Aalto, J., Hakola, H., Makkonen, U., Ruuskanen, T., Mauldin, R. L. III, Duplissy, J., Vehkamäki, H., Bäck, J., Kortelainen, A., Riipinen, I., Kúrten, T., Johnston, M. V., Smith, J. N., Ehn, M., Mentel, T. F., Lehtinen, K. E. J., Laaksonen, A., Kerminen, V.-M., and Worsnop, D. (2013). Direct Observations of Atmospheric Aerosol Nucleation. Science, 22:911–912.
- Kulmala, M., Vehkamäki, H., Petäjä, T., Dal Maso, M., Lauri, A., Kerminen, V.-M., Birmili, W., and McMurry, P. H. (2004). Formation and Growth Rates of Ultrafine Atmospheric Particles: A Review of Observations. J. Aerosol Sci., 35(2):143–176.
- Li, Q., Jiang, J., and Hao, J. (2015). A Review of Aerosol Nanoparticle Formation from Ions. KONA Powder Part. J., 32:57–74.
- McMurry, P. (2000). The History of Condensation Nucleus Counters. Aerosol Sci. Technol., 33(4):297–322.
- Nolan, J. J., and Guerrini, V. H. (1936). The Determination of the Mass and Size of Atmospheric Condensation Nuclei. T. Faraday Soc., 32:1175–1181.
- Okuyama, K., Kousaka, Y., and Motouchi, T. (1984). Condensational Growth of Ultrafine Aerosol-Particles in a New Particle-Size Magnifier. Aerosol Sci. Technol., 3(4):353–366.
- Pollak, L. W. and Metnieks, A. L. (1957). On the Determination of the Diffusion Coefficient of Heterogeneous Aerosols by the Dynamic Method. Geofisica Pura e Applicata., 37:183–190.
- Reischl, G. P., Mäkelä, J. M., Karch, R., and Necid, J. (1996). Bipolar Charging of Ultrafine Particles in the Size Range Below 10 nm. J. Aerosol Sci., 27(6):931–949.
- Rönkkö, T., Virtanen, A., Kannosto, J., Keskinen, J., Lappi, M., and Pirjola, L. (2007). Nucleation Mode Particles with Non-Volatile Core in the Exhaust of a Heavy Duty Diesel Vehicle. Environ. Sci. Technol., 41(18):6384–6389.
- Saros, M. T., Weber, R. J., Marti, J. J., and McMurry, P. H. (1996). Ultrafine Aerosol Measurement Using a Condensation Nucleus Counter with Pulse Height Analysis. Aerosol Sci. Technol., 25(2):200–213.
- Scheibel, H. G., and Porstendörfer, J. (1983). Generation of Monodisperse Ag- and NaCl-Aerosols with Particle Diameters Between 2 and 300 nm. J. Aerosol Sci., 14(2):113–126.
- Schneider, J., Hock, N., Weimer, S., Borrmann, S., Kirchner, U., Vogt, R., and Scheer, V. (2005). Nucleation Particles in Diesel Exhaust: Composition Inferred from in situ Mass Spectrometric Analysis. Environ. Sci. Technol., 39:6153–6161.
- Sinclair, D., Countess, R. J., Liu, B. Y. H., and Pui, D. Y. H. (1979). Automatic Analysis of Submicron Aerosols, in Aerosol Measurement, D. A. Lundgren, et al., eds. University of Florida Press, Gainsville, FL, pp. 544–563.
- Sinclair, D., and Hoopes, G. S. (1975). A Novel Form of Diffusion Battery. Am. Ind. Hyg. Assoc. J., 36:39–42.
- Steiner, G., Jokinen, T., Junninen, H., Sipilä, M., Petäjä, T., Worsnop, D., Reischl, G. P., and Kulmala, M. (2014). High-Resolution Mobility and Mass Spectrometry of Negative Ions Produced in a 241Am Aerosol Charger. Aerosol Sci. Technol., 48(3):261–270.
- Steiner, G., and Reischl, G. P. (2012). The Effect of Carrier Gas Contaminants on the Charging Probability of Aerosols Under Bipolar Charging Conditions. J. Aerosol Sci., 54:21–31.
- Stolzenburg, M. R. (1988). An Ultrafine Aerosol Size Distribution Measuring System. Ph.D. dissertation, Department of Mechanical Engineering, University of Minesota.
- Vanhanen, J., Mikkilä, J., Lehtipalo, K., Sipilä, M., Manninen, H. E., Siivola, E., Petäjä, T., and Kulmala, M. (2011). Particle Size Magnifier for Nano-CN Detection. Aerosol Sci. Technol., 45(4):533–542.
- Wang, S. C., and Flagan, R. C. (1990). Scanning Electrical Mobility Spectrometer. Aerosol Sci. Technol., 13(2):230–240.
- Wiedensohler, A. (1998). An Approximation of the Bipolar Charge Distribution for Particles in the Submicron Size Range. J. Aerosol Sci., 19:387–389.