Abstract
Purpose
To investigate the performance of a narrow-scope knowledge-based RapidPlan (RP) model, for optimisation of intensity-modulated proton therapy (IMPT) plans applied to patients with locally advanced carcinoma in the gastroesophageal junction.
Methods
A cohort of 60 patients was retrospectively selected; 45 were used to ‘train’ a dose-volume histogram predictive model; the remaining 15 provided independent validation. The performance of the RP model was benchmarked against manual optimisation. Quantitative assessment was based on several dose-volume metrics.
Results
Manual and RP-optimised IMPT plans resulted dosimetrically similar, and the planning dose-volume objectives were met for all structures. Concerning the validation set, the comparison of the manual vs RP-based plans, respectively, showed for the target (PTV): the homogeneity index was 6.3 ± 2.2 vs 5.9 ± 1.2, and V98% was 89.3 ± 2.9 vs 91.4 ± 2.2% (this was 97.2 ± 1.9 vs 98.8 ± 1.1 for the CTV). Regarding the organs at risk, no significant differences were reported for the combined lungs, the whole heart, the left anterior descending artery, the kidneys, the spleen and the spinal canal. The D0.1 cm3 for the left ventricle resulted in 40.3 ± 3.4 vs 39.7 ± 4.3 Gy(RBE). The mean dose to the liver was 3.4 ± 1.3 vs 3.6 ± 1.5 Gy(RBE).
Conclusion
A narrow-scope knowledge-based RP model was trained and validated for IMPT delivery in locally advanced cancer of the gastroesophageal junction. The results demonstrate that RP can create models for effective IMPT. Furthermore, the equivalence between manual interactive and unattended RP-based optimisation could be displayed. The data also showed a high correlation between predicted and achieved doses in support of the valuable predictive power of the RP method.
Introduction
Adenocarcinomas of the oesophagus are among the tumour diseases with the fastest growing incidence [Citation1,Citation2]. In the case of locally advanced tumours, the trimodal approach consisting of chemotherapy, radiotherapy and surgery, has been established as a standard of care in the curative setting. However, whether distal adenocarcinomas and carcinomas of the gastroesophageal junction should not be treated with perioperative chemotherapy, but instead only with neoadjuvant radiochemotherapy, is currently unclear and critically discussed [Citation3–5].
The anatomical position of the oesophagus, near to several organs at risk such as the lungs, heart and stomach, suggests that especially carcinomas of the gastroesophageal junction could profit of proton beam therapy (PBT). Previously published dosimetric comparisons of PBT and intensity-modulated radiation therapy (IMRT) have shown a significant improvement in reducing radiation exposure of organs at risk (OAR) in the PBT group [Citation6–10]. Especially heart and lung doses were significantly lower in the context of proton irradiation. Regarding this, we recently demonstrated in an in-silico study, that, for cancers of the gastroesophageal junction, the use of intensity-modulated proton therapy (IMPT) had beneficial aspects over volumetric modulated arc therapy (VMAT) with photons [Citation11] also for the abdominal organs. One of the first randomised studies with the question if dosimetric advantages of PBT for locally advanced oesophageal cancer translates to improved clinical outcomes compared with IMRT was recently published [Citation12]. The results showed a significant reduction of radiation therapy-induced toxicities in favour of PBT. However, for PBT to become established in the long term, it must be demonstrated in future randomised studies that the known dosimetric advantages are also associated with improvements in treatment outcome [Citation13].
In this context, the development of tools aiming to streamline and enhance the quality of treatment planning is also needed for PBT. In particular, the process of dose optimisation, a potentially challenging task with manual planning, might benefit from the introduction of some degree of automation. A possible approach, which has already been tested and established for VMAT and IMRT, is given by the use of RapidPlan (RP, Varian Medical Systems, Palo Alto, California, USA), a machine-learning-based and semi-automated planning method [Citation14–16]. The conceptual aim of RP is to generate individualised optimisation objectives based on the prediction of the achievable dose distribution for any given patient and beam geometry. In fact, the core of RP is the use of a mathematical prediction model for dose-volume histograms. The RP models shall be trained on adequately selected and qualitatively well-prepared treatment plans with an adequate pooling of the population to be modelled. First fundamental works on the application of RP for protons was published by Delaney [Citation17,Citation18] where the specificity of RP for protons was outlined in comparison to the photon case, and early tests were performed on head and neck patients. Cozzi [Citation19] recently developed and validated an RP model for IMPT plans in patients with advanced hepatocellular carcinomas and compared these plans with VMAT plans. To prove the validity and reliability of the RP method for protons, it is necessary to further test it for a large number of different tumour entities, similar to the established use for photon plans.
A second key aspect of RP is that, given the generation of individualised optimisation objectives based on the earlier clinical experience, should enable the possibility to execute, in an un-attended manner, the inverse optimisation phase. In fact, RP generates the individual constraints at the lower side of the uncertainty band of the predicted DVH. For this reason, if a proper match between the model applied and the prospective patient is done, the automated process should result in clinically acceptable and high-quality plans (with the freedom to interactively further improve the optimisation process if deemed necessary for any reason).
The efficacy of the use of this kind of knowledge-based planning method relies on: (i) the quality of the data used for the training, (ii) the regression applied to build the predictive models, and (iii) the consistency between the new case and the population used for the training (i.e., the generalisation power of the model).
The RP implementation is agnostic to some aspects of the treatment planning, in particular to the technique (e.g., single field or multiple field optimisation) and the strategy applied for the compensation/management of organs motion (e.g., robust optimisation or gating). Although these are fundamental aspects for the quality of the plans and the safety of the delivery, this independence property makes RP versatile and applicable in a wide variety of cases. From a comparative study point of view, it is only necessary to ‘apply’ the same strategy to both manual and automated datasets and train the models on a consistent set of plans.
The present study aimed to provide further evidence of the feasibility of the RP model for proton planning by applying it to complex target volume structures such as those found in locally advanced carcinomas of the gastroesophageal junction. The performance of the RP model was benchmarked against manual optimisation.
Materials and methods
Patient selection, contouring, and dose prescription
The computed tomography (CT) images and structure sets of 60 patients were used for this in-silico investigation. All patients initially signed authorisation forms allowing the use of their data for further research. All patients presented with advanced (cT3cNx cM0) adenocarcinoma of the gastroesophageal junction. The gross tumour volume (GTV) was identified on the extent of the disease using the initial PET/CT scan, endoscopy report and CT scan. Contouring was performed according to institutional and consensus-based guidelines [Citation20] on free-breathing planning CT scans. The entire oesophageal wall, including any disease that extended through the wall, was contoured as the GTV as well as any PET/CT-avid or enlarged lymph nodes. The clinical target volume (CTV) included the peri-oesophageal and mediastinal lymph nodes as well as the submucosal spread along the oesophagus (corresponding to a 3–4 cm expansion on the GTV superiorly and inferiorly and a 1.0–1.5 cm radial expansion). A planning target volume (PTV) was generated, adding 0.7 cm isotropically to the CTV for consistency with earlier studies and reporting purposes. The lungs, the whole heart with its structures (atrial and ventricular left and right chambers and the coronaries), the oesophagus, the liver, the kidneys, the spleen, the stomach, the bowels and the spinal canal were contoured and considered as OARs.
The dose prescription was 41.4 Gy (RBE) in 23 daily fractions as in the clinical routine.
For reporting purposes only, all the plans were normalised to 100% as the mean dose to the PTV; the dose-volume objectives for the target volumes were: mean dose 41.4 Gy(RBE) to the GTV, CTV and PTV. For the GTV and CTV a minimum coverage of V98%≥98%. For the PTV the aims were used for reporting only and considered useful in case of plan optimisation done on this structure. The coverage required for the PTV was V98%≥90% and V95%≥95%. For all target structures, the near-to-maximum dose D1% was to be minimised as much as possible. For the OARs, the planning aims were: for the lungs: mean dose ≤12 Gy, V20Gy≤20%; for the heart: mean dose ≤10Gy and V30Gy≤10%; for the liver mean dose ≤15Gy; for the kidneys mean dose ≤15Gy and V20Gy≤32%. For all the other OARs, the aim was to minimise the dose (mean or near-to-maximum) as much as achievable without compromising the coverage of the target).
Treatment planning
The Eclipse treatment planning system (Varian Medical Systems, Palo Alto, USA) with the clinically released version 16.0 was used for all the patients and algorithms.
IMPT plans were created using pencil beam spot scanning from the ProBeam proton system (Varian Medical Systems, Palo Alto, USA). The dose distribution was optimised using the fluence-based nonlinear universal Proton Optimiser (NUPO) [Citation21]. Spot spacing was 0.425 of the energy-dependent in-air full-width half-maximum spot size at the isocenter. The multifield simultaneous spot optimisation method was selected for all plans. The Proton Convolution Superposition algorithm was used for the final dose calculation with a grid of 2.5 × 2.5 × 2.5 mm3. A constant relative biological effectiveness (RBE) of 1.1 was applied.
All patients in the study were planned with a class solution geometry defined by two posterior oblique fields with gantry angles set to 150° and 220°. Concerning the plan optimisation, in addition to the dose-volume objectives needed by the inverse planning engine, the robust optimisation method was enabled for the CTV to account for setup and range uncertainties considering ±3mm shifts in the isocentre along the x-y-z coordinates and ±3% in beam range. The 3 mm shifts are not to be intended as a proton specific margin to the CTV but the positioning uncertainty of it. The robust optimisation should result in plans minimising the trade-offs derived from the applied uncertainties.
The RapidPlan predictive model
The RP knowledge based planning engine consists of three main subsystems: (i) a model training environment, (ii) a dose volume histogram (DVH) prediction environment, and (iii) the generation of personalised dose-volume constraints for the plan optimisation. The first is devoted to the data modelling of the plan and patient datasets and the training of the predictive model for each OAR. The second component aims to estimate the dose distribution as the DVH achievable for a certain new patient, based on the application of the predictive model. The third element derives from the estimated DVH and from a set of customisable rules associated with the model, the actual dose-volume constraints to use for the dose plan optimisation.
For each OAR structure, RP for protons partitions the volumes into three sub-regions: (1) the out-of-field region where the geometry-based expected dose (GED) is approximately zero, (2) the in-field region where GED is non-negligible, and there is no overlap with the targets and (3) the target overlap region is the part of the volume that is also inside any structure labelled as a target. The regions 1 and 3 are, for different reasons, characterised by little modulation, and the estimation of the DVH can be modelled with simple average and standard deviation from the training dataset. On the contrary, the in-field region is the structure where high modulation occurs, and fine predictions are needed. The GED accounts for the position and distribution of an OAR with respect to the targets and impacts on the achievable dose distributions once a field geometry is defined. The core of RP is to parametrise the GED and achievable DVH employing principal component analysis and fit a model usable for prospective cases [Citation17,Citation18]. An explanatory diagram of the PCA process is presented in the complementary materials (Supplementary Figure 1). The model so achieved can be used then to predict a probable DVH for any prospective patient and any OAR included in the model itself. Further details about the model training can be found in [Citation19].
In parallel to the training of the DVH prediction model, the RP system associate to a model a set of optimisation objectives per each of the OARs included. The actual prospective objectives for any new patient will be derived from the list in the model and adapted to the DVH predictions. The type of objectives available is upper, mean, generalised equivalent uniform dose (gEUD) and line-type. For each of these, the defining parameters (e.g., the volume or dose values) and the priorities can be set explicitly (generating in this way a kind of rigid template) or left to the prediction engine to place them below the inferior limit of the uncertainty band of the estimated DVH (the ‘generate’ mode). The latter approach allows RP to introduce the maximum of flexibility and individualisation in the optimisation constraints. Line-type objectives are available in RP-based optimisation to limit the dose in a given structure for all volume levels with the same ‘strength’ in each region. Supplementary Figure 2 illustrates the logic of the objectives creation made by RP.
In our study, the mean, upper, gEUD and line-type model generated objectives and priorities were defined as described in . The list of objectives included in any model would be editable (to be fine-tuned or to add/remove objectives) by authorised users and adjustable according to institutional or protocol related requirements.
Table 1. CTV, PTV and OARs objectives implementation in the RapidPlan model.
The RP model of this study was defined and trained on a set of manually optimised IMPT plans, similarly to what described in [Citation19]. The training was performed on a cohort of 45 patients manually optimised by experienced planners and assessed according to the dose-volume planning aims of the study and the principle of dose minimisation to all the structures while maintaining the target coverage [Citation11]. To be included in the training set, a plan was carefully scrutinised by expert planners and clinicians to meet the aims of the study and to avoid sub-optimality of the dose distributions. In this manner, the variance present in the patient population is primarily due to the presence of varying target volumes (and organs at risk) in the population and not to deficiencies in the plan quality.
The remaining 15 patients of the investigated cohort, not used for the model training, were selected for independent validation tests (open-loop validation). For each patient in the validation cohort, two plans were designed for manual, and RP based IMPT. During manual optimisation, all the dose-volume objectives and the time spent with optimisation were possibly adjustable while the beam geometry was not tuneable. On the contrary, in the validation phase, no manual fine-tuning of the generated objectives was performed, and the optimisation was run in an unattended manner. This allowed the appraisal of the intrinsic performance of RP; further interactive tuning of the parameters might be advisable (if deemed necessary) in the routine clinical practice.
Quantitative assessment of dose-volume metrics
Quantitative metrics were derived from the DVH and included the mean dose and a variety of Dx and Vx parameters with Dx representing the minimum dose that covers an x fraction of volume (in % or cm3) and Vx representing the volume receiving at least an x level of dose (in % or Gy(RBE)). All parameters could be expressed either in absolute (Gy(RBE) or cm3) or relative (%) terms. The average DVHs were computed, for each structure and each cohort, with a dose binning resolution of 0.02 Gy(RBE). All the doses in the study are reported as Gy (RBE), i.e., in Cobalt Grey equivalent (corrected for the RBE factor).
The Wilcoxon matched-pairs signed-rank test was applied to evaluate the significance of the observed differences per each couple of plans. The threshold for statistical significance was p < .05.
Results
To visualise and quantify the efficacy of the model, the predicted and achieved mean doses for the various OARs were compared after full optimisation and dose computation. shows the scatter plot of this investigation and includes both the training (circles) and the validation (triangles) sets. The linear regression fit parameters, with a regression coefficient of 0.954 and 0.956, respectively, demonstrated how precisely the predicted doses correspond to the achieved values.
Figure 1. Scatter plot of the predicted versus achieved mean doses for the various OARs. The graphs include both training (squares) and validation (triangles) sets.
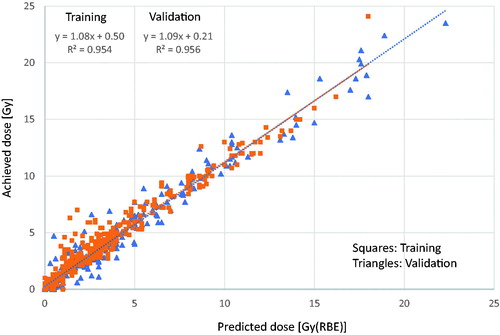
To provide a glance to the inter-patient variability of the training cohort, the distribution of the DVH for the primary OARs is visualised in Supplementary Figure 3 in the complementary materials, similar trends were observed for the remaining OARs not shown for space reasons. For some structures, the distribution of the DVH shown in that figure presented a wider range (e.g., for the stomach or the spleen) compared to, e.g., the heart or the lungs. This variability in the plans used for the training was not due to sub-optimality of the chosen plans but due to different organ’s filling as well as due to the different position of the organ to the target volumes.
shows the average DVHs for the manual and RP based IMPT plans for the target volumes and the various OARs for the validation cohort. From a qualitative perspective, the RP reproduced the manual plans in a very consistent manner without the presence of any significant deviation between the two datasets.
Figure 2. Average dose-volume histograms (solid lines) and one standard deviation range (dotted lines) for the entire validation cohort for all the techniques for the gross tumour volume (GTV), the clinical and planning target volumes (CTV and PTV) and the main organs at risk. Data are shown for the manually optimised intensity-modulated proton therapy plans (IMPT) and the plans optimised with RapidPlan (IMPT_RP).
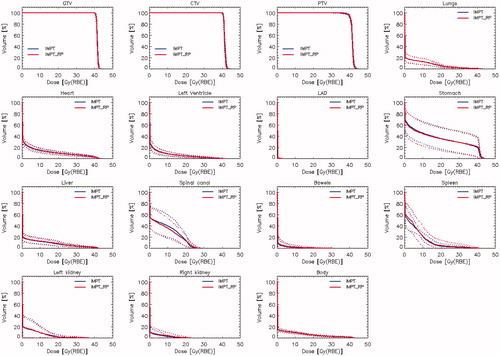
and summarise the quantitative analysis of the dose-volume metrics derived from the individual DVH from each patient. The data are reported as mean values for the manual plans (for the training and validation groups) and the RP-based automated plans in the open-loop experiment (validation set only). The clinical planning goals are reported for each parameter; the interpatient variance is reported as one standard deviation of the mean. The statistical significance is reported only if ≤0.1 and for the validation set only. As anticipated from the qualitative graphs, no clinically remarkable difference was measured among the three sets of plans.
Table 2. Summary of the planning objectives and average results (uncertainty expressed as 1 standard deviation) for the gross target volume (GTV), the clinical target volume (CTV) and for the planning target volume (PTV).
Table 3. Summary of the planning objectives and average results (uncertainty expressed as 1 standard deviation) for the main organs at risk investigated in the study).
Discussion
In this report, we summarised the results of the training and validation of an RP knowledge-based model applied to IMPT planning for locally advanced carcinoma of the gastroesophageal junction. The potential benefits of PBT for oesophageal cancer have already been presented in several publications [Citation6–10]. This study aimed to expand the evidence about the potential role of RP in PBT proton therapy planning for cancer of the gastroesophageal junction after earlier investigations pioneered its use for head and neck or liver cancer [Citation17–19]. No comparison was reported in the current study for photon-based volumetric modulated arc therapy since this topic was addressed in the earlier investigation [Citation11] and the IMPT plans demonstrated to outperform photons in many metrics.
The use of RP for PBT can bring several advantages. One of the most important is the improvement of planning effectiveness with more consistent results among different planners and patients. This can be particularly important in everyday clinical practice and the planning of IMPT if the tumour entity to be treated presents a challenge in terms of sparing the surrounding organs due to its anatomical location. Concerning this aspect, the selected patient and tumour collective is especially well suited to this question. Due to the anatomic location of the oesophagus within proximity to several organs at risk, the irradiation of such carcinomas is potentially challenging. However, the data of this study showed that the tolerance levels applied were achievable ().
One of the scopes of the use of RP is to improve the harmonisation of the plan quality in a cohort of patients and to partially automate the process of the optimisation. Both objectives were met as suggested by the results. The first is confirmed, e.g., by the small inter-patient variance of the dose-volume metrics and the high correlation between predicted and achieved doses. The second is confirmed by the fact that the RP based plans were optimised and computed in an entirely unattended manner (while manual planning required interactive trial and error to set the appropriate ideal objectives) with highly consistent results. As a caveat, we shall note that the harmonisation aim is somehow prone to the characteristics of the population under investigation. Significant variations of the target volumes among the patients, and, similarly, in the geometrical relation between targets and OARs can have an impact on the plan quality harmonisation power of the automated process.
Concerning the performance of the RP prediction engine, the data of the study showed a mild tendency of higher achieved mean doses compared to the predicted ().
This result suggests that some fine-tuning of either the optimisation priorities or of the dose-volume constraints should be done interactively for some patients to reduce this discrepancy. Otherwise, the definition of stronger priorities for the OARs should be implemented in the algorithm for a completely un-attended usage. From the other side, this finding is a direct consequence of the study design aiming to investigate the performance of the RP model when executed in an entirely un-attended manner. Since, generally speaking, the RP engine as per its design, does not pretend to achieve the absolute best plans (e.g., the generated constraints line are located slightly below the one standard deviation uncertainty band of the predicted DVH as shown in Supplementary Figure 2) the performance of the system could be improved with some additional tool. One solution might be given by the use of post-optimisation multi-criteria tuning of the plans which could facilitate the balance between competing objectives. In summary, the observed modest defect in performance might be mitigated with either stronger priority or with additional tunings.
For the study, the multi-field optimisation approach was adopted. It is essential to clarify that RP is somehow agnostic to the optimisation engine and delivery approach chosen, provided that this is based on inverse planning. For this reason, RP could also apply to single field optimisation with a uniform dose (SFO or SFUD). SFO could be a reasonable approach in the case of oesophageal cancer given the tumour localisation and the dose prescription and constraints. It could be seen as a valuable alternative to robust optimisation in the presence of organ motion. We opted not to include SFO in the present study to keep the focus on the role of RP rather than adding a second layer of comparison between IMPT and SFO which might be the scope of further investigations. For a proper use, RP model should be trained and used prospectively on a consistent choice of technique.
As a caveat, we shall mention that the plans in the study were all normalised to the mean dose to the PTV. Although this is not entirely appropriate when robust optimisation is applied (in this case normalisation should be applied to the CTV), the decision was made based on (i) the difference in scaling between CTV and PTV is marginal (); (ii) RP is agnostic to the origin of the training and to the methods applied for the prospective plans (i.e., if optimised with robust methods or not) and (iii) we opted to leave open the option (not advisable) to use the model also without robust optimisation. In such a case, the inclusion of PTV would have been necessary. Finally, normalisation to the PTV might facilitate comparison with earlier literature and with eventual data from photon therapy.
Some further limiting factors in the study should be considered. In the first instance, the relatively small size of the training cohort might impact on the predictive power for some of the organs at risk, given the large variance in the distribution of the DVH data (). This variance was anyhow related to the presence of variable targets in the training population, reflecting the reality of the clinical domain and not due to suboptimality of the plans chosen for the training which were carefully scrutinised before their selection. Relatively to the sample size, the RP system requires only a minimum of 20 entries to train the model on any given organ at risk. Nevertheless, the good predictive power of the model was demonstrated in the comparison between the predicted and achieved doses illustrated in .
Secondly, the scope of the model was kept intentionally ‘narrow’, i.e., limited to advanced cancer in the gastroesophageal junction. The training of a broad scope model, inclusive of the middle and upper tracts of the oesophagus might be advisable. Still, it would require a substantially larger cohort to properly sample all the anatomical structures and conditions. Further investigation in this direction is envisaged.
The selection of the gastroesophageal junction district also influenced the choice of the beam arrangement with two posterior oblique fields. While this arrangement is anatomically natural, no investigation was performed to appraise the potential benefit derived from some fine-tuning of the beam angles. In the earlier study for liver [Citation19], we demonstrated the minor role played by small variations in the beam arrangement. Significant deviations in the gantry angle from what used in the model training would induce, at least, sub-optimality in the plan optimisation. This because the RP modelling relies primarily on the relation between beam geometry and target-OARs positions. If a broader scope model would be considered, possibly substantially different beam directions might be selected (e.g., with anterior-oblique incidence) and this would require a further increase of the training set to account for all the contributing factors.
Another factor to be considered with care is the uncertainty associated with the segmentation of some of the organs at risk involved in the treatment. In particular, all the heart structures are hard to segment due to image quality and motion artefacts. In contrast, the abdominal structures like the stomach or the bowels are affected by a vast (daily) variation due to different filling conditions and internal organs movement. This problem was not directly addressed in the study, and the model training was performed under the assumption that a proper segmentation of the structures was performed. The performance of RP on planning CT datasets was therefore assessed as the primary scope of the study. The management of daily variations would fall in the broad area of motion control and of treatment adaptation which is fundamental but is independent of the use of automated planning tools like RP. In fact, RP is agnostic also to the motion management mitigation strategy applied and, in the frame of a comparative assessment between manual and automated planning, the relevant fact is to adopt the same strategy (if any) in both cases. This was done in the study with the same robust optimisation method. Concerning motion management, appropriate 4 D delivery methods such as breath-hold or gating for the respiratory induced and/or abdominal compression should be considered; more sophisticated approaches (like rescanning) could further mitigate the interplay problems due to organs motion while only plan adaptation could account for substantial anatomical changes. As mentioned above, SFO could be a valuable alternative in terms of robustness and the mitigation of inter-play effects. As an example, Mondlane [Citation22] showed that SFO, using beam specific PTVs, along with robust optimisation methods could bring about a potentially relevant reduction in the probability of normal tissue complication (for the kidneys) in patients with gastric cancer. The investigation of the entire motion management arena is fundamental before clinical adoption of PBT, but it is not directly impacting on the use of automated optimisation engines like RP. As a first-order mitigation approach, the study implemented the concept of robust optimisation, applied to the CTV, with both range and positioning uncertainties accounted for. Robustly optimised plans were used as input to the model training.
Conclusion
A narrow-scope knowledge-based RP model was trained and validated for IMPT planning in locally advanced cancers of the gastroesophageal junction. The results demonstrate that RP can create models for effective IMPT. The results showed the equivalence between manual interactive and unattended RP-based optimisation. Furthermore, our presented data revealed a high correlation between predicted and achieved doses in support of the valuable predictive power of the RP method.
Supplemental Material
Download MS Word (1.4 MB)Disclosure statement
L. Cozzi acts as Scientific Advisor to Varian Medical Systems and is Clinical Research Scientist at Humanitas Cancer Center. All other co-authors declare that they have no conflict interests.
References
- Ferlay J, Steliarova-Foucher E, Lortet-Tieulent J, et al. Cancer incidence and mortality patterns in Europe: estimates for 40 countries in 2012. Eur J Cancer. 2013;49(6):1374–1403.
- Castro C, Bosetti C, Malvezzi M, et al. Patterns and trends in esophageal cancer mortality and incidence in Europe (1980–2011) and predictions to 2015. Ann Oncol. 2014;25(1):283–290.
- Lordick F, Mariette C, Haustermans K, ESMO Guidelines Committee, et al. Oesophageal cancer: ESMO Clinical Practice Guidelines for diagnosis, treatment and follow-up. Ann Oncol. 2016;27(suppl 5):v50–v7.
- Hoeppner J, Lordick F, Brunner T, et al. ESOPEC: prospective randomized controlled multicenter phase III trial comparing perioperative chemotherapy (FLOT protocol) to neoadjuvant chemoradiation (CROSS protocol) in patients with adenocarcinoma of the esophagus (NCT02509286). BMC Cancer. 2016;16:503.
- Reynolds JV, Preston SR, O'Neill B, et al. ICORG 10-14: NEOadjuvant trial in adenocarcinoma of the oEsophagus and oesophagoGastric junction International Study (Neo-AEGIS). BMC Cancer. 2017;17(1):401.
- Warren S, Partridge M, Bolsi A, et al. An analysis of plan robustness for esophageal tumors: comparing volumetric modulated arc therapy plans and spot scanning proton planning. Int J Radiat Oncol Biol Phys. 2016;95(1):199–207.
- Liu C, Bhangoo RS, Sio TT, et al. Dosimetric comparison of distal esophageal carcinoma plans for patients treated with small-spot intensity-modulated proton versus volumetric-modulated arc therapies. J Appl Clin Med Phys. 2019;20(7):15–27.
- Xi M, Xu C, Liao Z, et al. Comparative outcomes after definitive chemoradiotherapy using proton beam therapy versus intensity modulated radiation therapy for esophageal cancer: a retrospective, single-institutional analysis. Int J Radiat Oncol Biol Phys. 2017;99(3):667–676.
- Warren S, Hurt CN, Crosby T, et al. Potential of proton therapy to reduce acute hematologic toxicity in concurrent chemoradiation therapy for esophageal cancer. Int J Radiat Oncol Biol Phys. 2017;99(3):729–737.
- Hirano Y, Onozawa M, Hojo H, et al. Dosimetric comparison between proton beam therapy and photon radiation therapy for locally advanced esophageal squamous cell carcinoma. Radiat Oncol. 2018;13(1):23.
- Celik E, Baus W, Baues C, et al. Volumetric modulated arc therapy versus intensity-modulated proton therapy in neoadjuvant irradiation of locally advanced oesophageal cancer. Radiat Oncol. 2020;15(1):120.
- Lin S, Hobbs B, Verma V, et al. Randomized phase IIB trial of proton beam therapy versus intensity-modulated radiation therapy for locally advanced esophageal cancer. J Clin Oncol. 2020;38(14):1569–1579.
- Verma V, Mishra MV, Mehta MP. A systematic review of the cost and cost-effectiveness studies of proton radiotherapy. Cancer. 2016;122(10):1483–1501.
- Fogliata A, Nicolini G, Bourgier C, et al. Performance of a knowledge-based model for optimization of volumetric modulated arc therapy plans for single and bilateral breast irradiation. PLoS One. 2015; 10(12):e0145137.
- Fogliata A, Wang PM, Belosi F, et al. Assessment of a model based optimization engine for volumetric modulated arc therapy for patients with advanced hepatocellular cancer. Radiat Oncol. 2014;9:236.
- Tol J, Delaney A, Dahele M, et al. Evaluation of a knowledge-based planning solution for head and neck cancer. Int J Radiat Oncol Biol Phys. 2015;91(3):612–620.
- Delaney A, Dahele M, Tol J, et al. Using a knowledge-based planning solution to select patients for proton therapy. Radiother Oncol. 2017;124(2):263–270.
- Delaney A, Verbakel W, Lindberg J, et al. Evaluation of an automated proton planning solution. Cureus. 2018;10:e3696.
- Cozzi L, Vanderstraeten R, Fogliata A, et al. The role of a knowledge based dose–volume histogram predictive model in the optimisation of intensity-modulated proton plans for hepatocellular carcinoma patients. Strahlenther Onkol. 2020.DOI:10.1007/s00066-020-01664-2
- Wu A, Bosch W, Chang D, et al. Expert consensus contouring guidelines for intensity modulated radiation therapy in esophageal and gastroesophageal junction cancer. Int J Radiat Biol Oncol Phys. 2015;92(4):911–920.
- Nocedal J, Wright S. Numerical optimisation. 2nd ed. Springer; 2006.
- Mondlane G, Ureba A, Gubanksi M, et al. Estimation of risk of normal tissue toxicity following gastric cancer radiotherapy with photon or scanned proton beams. Anticancer Res. 2018;38:2619–2625.