Abstract
Background/Purpose
The current study aimed to investigate the correlation between tumor-infiltrating lymphocytes (TILs) and immunotherapy efficacy in patients with advanced non-small cell lung cancer (NSCLC).
Materials and methods
Eighty-nine patients with advanced NSCLC who received immune checkpoint inhibitors (ICIs) monotherapy were retrospectively enrolled in this study. The density of TILs in paraffin-embedded pathological tissues taken before receiving ICIs was quantitatively analyzed by immunohistochemical staining. The density of TILs was treated as a dichotomous variable using the median as the cutoff value. The Kaplan–Meier analysis was used to assess survival differences between groups. Univariate and multivariate Cox analyses were applied to screen out independent prognostic factors and further construct a nomogram prediction model to predict survival.
Results
Survival analysis showed that CD8+ TILs, CD4+ TILs, and IFN-γ+ Th1 were significant positive indicators for predicting progression-free survival (PFS) and overall survival (OS) (p < 0.05), whereas Foxp3+ Treg were a significant negative predictor (p < 0.05). The predictive role of IL-4+ Th2 was not apparent in this study and requires further investigation and exploration (p > 0.05). The nomogram prediction model exhibited good discriminative ability, with C-index values of 0.723 (95% CI 0.682-0.764) and 0.793 (95% CI, 0.738-0.848) in the training cohort and validation cohort, respectively. The AUC values indicated that the nomogram prediction model had high predictive value and the calibration curve presented good prediction accuracy.
Conclusions
TILs could predict the efficacy of immunotherapy and may become a promising predictor.
Introduction
Immune checkpoint inhibitors (ICIs) targeting programmed cell death-1/programmed cell death ligand-1 (PD-1/PD-L1) or the cytotoxic T lymphocyte antigen-4 (CTLA-4) pathway have shown sustained clinical responses in the treatment of multiple advanced cancers, especially in advanced non-small cell lung cancer (NSCLC) [Citation1]. Among multiple approved immune modulators, PD-1/PD-L1 blockade therapy has significantly improved the durable response rate and prolonged long-term survival with manageable adverse effects in monotherapy and combination therapy for patients with advanced NSCLC [Citation2,Citation3]. PD-1 is mainly expressed on activated T cells and inhibits T-cell activation through binding to the PD-1 ligands, PD-L1 and PD-L2. PD-L1, the primary ligand for PD-1, is expressed in various normal tissues and malignant cells [Citation4]. The expression of PD-L1 is upregulated on tumor cells in the presence of interferon-γ (IFN-γ) and other cytokines in chronic inflammation or infection. Upregulated PD-L1 binds PD-1 on T cells, leading to the development of T-cell exhaustion [Citation5]. ICIs blocking PD-1/PD-L1 interaction remarkably rescue the function of these exhausted T cells and enhance anti-tumor immunity. Therefore, the PD-L1 expression, determined by immunohistochemical methods, has been extensively studied as a predictor of the patients’ response to PD-1/PD-L1 blockade therapy and has proven to be a valid biomarker. However, strong responses to immunotherapy in some patients with low levels of PD-L1 expression complicate the application of PD-L1 as a predictive biomarker of exclusion [Citation6,Citation7]. In addition, tumor mutation burden (TMB), a measure of the number of mutations in a tumor, is emerging as a potential biomarker. The more mutations, the more neo-antigens, and the greater the chance that one or more of those neo-antigens will generate immunogenicity and trigger T-cell responses [Citation8]. Accumulating evidence supports that tumor types with high TMB will be more responsive to immunotherapy regimens [Citation9]. Although the US Food and Drug Administration (FDA) granted tissue-agnostic accelerated approval for pembrolizumab in TMB ≥10 mutations/Mb solid tumors in 2020 [Citation10], diagnostic tests to evaluate TMB are being developed and refined, and further prospective validation studies are required for the development of TMB as a clinical predictive biomarker.
Tumor-infiltrating lymphocytes (TILs) have conferred a predictive impact and represent a promising predictive biomarker that needs further exploration and validation before use [Citation11]. Among different tumor-infiltrating immune cells, including leucocytes, macrophages, dendritic cells, and mast cells, TILs are lymphocytes with a higher specific immunological response against tumor cells [Citation12]. The major component of the tumor microenvironment (TME) is represented by TILs containing different fractions of CD4+ (helper) and CD8+ (cytotoxic) T lymphocytes. Studies have suggested that CD8+ T lymphocytes play an essential role in anti-tumor immunity and can potentially be a valuable biomarker for predicting the efficacy of ICIs [Citation13]. However, the role of CD4+ T lymphocytes and their subsets in response to PD-1/PD-L1 blockade therapy is to be investigated in detail, with no apparent correlation established yet [Citation14]. Therefore, our study will further explore the correlation between TILs and the efficacy of immunotherapy to provide better guidance for its application in treatment of NSCLC.
Materials and methods
Participants
This study retrospectively analyzed advanced NSCLC patients hospitalized in Shandong Cancer Hospital, who received ICIs monotherapy from September 2019 to June 2021, and were followed up until February 2022. The inclusion criteria of patients were as follows: 1) pathological diagnosis of advanced NSCLC (stage III or IV) or recurrence and metastasis, and without epidermal growth factor receptor (EGFR) mutation and anaplastic lymphoma kinase (ALK) rearrangement; 2) had never previously received immunotherapy or radiotherapy; 3) received at least two cycles of immune monotherapy; 4) aged from 18 to 85 years old with the Eastern Co-operative Oncology Group (ECOG) performance status (PS) score of 0–2; 5) the presence of evaluable lesions on imaging according to the Response Evaluation Criteria In Solid Tumors version 1.1. The exclusion criteria were as follows: 1) female patients with a pregnancy before or after inclusion in the study; 2) patients with brain metastasis symptoms or more than three brain metastases; 3) patients having systemic diseases requiring hormone or other immunosuppressant treatment; 4) meningeal metastasis. We finally included 89 cases and divided them into a training cohort and a validation cohort in a ratio of 7:3 (Supplementary Figure 1). Immunohistochemical staining and other methods were used to analyze the infiltration density of TILs in paraffin-embedded pathological tissue samples of enrolled patients before receiving immunotherapy.
Immunohistochemistry analysis
The pretreatment biopsy specimens for all enrolled patients were retrospectively collected. Sections of 3.5 µm thickness were cut from formalin-fixed paraffin-embedded tissues. The pathologist confirmed the presence of NSCLC by hematoxylin and eosin (H&E) staining. H&E stained sections were generated from selected primary tumor blocks with representative tumor regions. Slides were then stained using an automated slide stainer for CD4 and CD8 and counter-stained with hematoxylin. These stained slides were automatically scanned using a Tissue Imaging Cytometry System and then viewed using the Aperio ImageScope analysis system. Finally, the immune cell infiltration density within the tumor regions was quantitatively analyzed using the ImageJ software. IFN-γ/CD4, IL-4/CD4, and Foxp3/CD4 double staining were performed using an automated slide stainer. For double staining targets IFN-γ, IL-4, and Foxp3 were stained by DAB and the color was brown, while CD4 was stained by basic fuchsin and the color was red. Two pathologists of intermediate grade and above independently interpreted the double-staining results.
Assessment
Overall survival (OS) was defined as the time from randomization to death from any cause. Progression-free survival (PFS) was defined as the time from randomization to disease progression or death for any reason. Response evaluation, including complete response (CR), partial response (PR), stable disease (SD), and progressive disease (PD), was performed according to the Response Evaluation Criteria in Solid Tumors version 1.1. The independent and centralized evaluation of treatment response was conducted by two radiologists using a blind method.
The TILs are defined as lymphocytes within and around cancer cells. TILs were quantified as counts of cells positive for each marker within each square millimeter and divided into high expression (Thigh) versus low expression (Tlow) groups by a median cutoff point to analyze the correlation between the expression levels of each class of TILs and the survival benefit of patients after ICI monotherapy.
Statistical analysis
The Kaplan–Meier method was used to estimate OS and PFS, while log-rank tests were used to assess the differences in PFS and OS between groups. Multiple comparisons were made to analyze statistical differences between groups. The chi-squared test and Fisher’s exact test were used for the correlation analysis of categorical variables. The Cox regression model was applied to analyze the hazard ratio (HR). A nomogram prediction model was constructed to evaluate the predictive value of biomarkers. Receiver operating characteristic (ROC) curves were analyzed, and the area under the curves (AUC) was calculated to assess the prediction accuracy. The discriminatory power of the model was assessed by the concordance index (C-index). Finally, calibration curves were plotted to evaluate and verify the performance of the nomogram. SPSS version 23.0 was used for all statistical analyses, and the plots were generated using the R statistical software 4.2 and GraphPad Prism 9.0. p < 0.05 was considered statistically significant.
Results
Patient characteristics
We ultimately included 89 patients with advanced NSCLC who received ICIs monotherapy for subsequent analysis. Among them, 62 patients were divided into training cohorts, and 27 patients were divided into validation cohorts. There were no significant differences in baseline characteristics such as age, gender, smoking history, and ECOG PS score between the training cohort and validation cohort. In the training cohort, 13 (21%) patients received immunotherapy in the first line of treatment, 33 (53.2%) patients in the second line, and 16 (25.8%) patients in the third line and beyond. The median PFS of all patients was 5.5 months, and the median OS was 10.3 months. The other baseline characteristics of the patients are shown in .
Table 1. Patient demographics and baseline characteristics.
Quantification of TILs in the training cohort
We detected the infiltration density of CD8+ TILs and CD4+ TILs in paraffin-embedded pathological tissues. The expression level was measured by the number of positive lymphocytes per square millimeter. The study populations were divided into the CD8+ Thigh and CD8+ Tlow groups as well as the CD4+ Thigh and CD4+ Tlow groups, with the respective medians 475/mm2 for CD8+ and 300.5/mm2 for CD4+ cells as the cutoff values.
We also focused on the correlation between CD4+ T cell subsets and the efficacy and prognosis of immunotherapy. The CD4+ T cell subsets mainly include T helper cell 1 (Th1), T helper cell 2 (Th2), and regulatory T lymphocytes (Treg). Th1 primarily secrete IFN-γ, interleukin-2 (IL-2), and tumor necrosis factor-α (TNF-α), while the Th2 cells secrete interleukin-4 (IL-4), interleukin-5 (IL-5), and interleukin-6 (IL-6). Further, the forkhead/winged helix transcription factor (Foxp3) is well recognized as a specific marker of Treg cells. Consequently, we considered CD4+ IFN-γ+ to represent Th1, CD4+ IL-4+ to represent Th2, and CD4+ Foxp3+ to represent Treg. Patients were similarly divided into the high- or low-expression groups using the median expression as the cutoff value (Supplementary Table 1). The representative pictures of high and low TILs expression in each category are shown in .
Figure 1. Representative pictures of TILs infiltration density by immunohistochemical staining (×200). CD8+ TILs with high (a) and low (b) infiltration density. CD4+ TILs with high (c) and low (d) infiltration density. IFN-γ+ Th1 with high (e) and low (f) infiltration density. IL-4+ Th2 with high (g) and low (h) infiltration density. Foxp3+ Treg with high (i) and low (j) infiltration density.
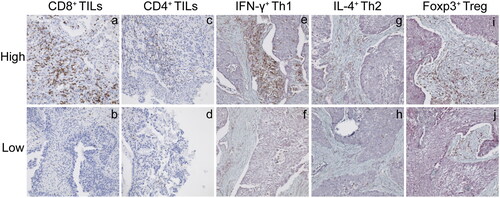
The correlation between TILs expression and clinicopathological characteristics
We analyzed and compared the clinical and pathological characteristics, including age, gender, smoking history, ECOG PS score, pathological type, disease stage, the timing of immunotherapy, and type of ICIs among patients distributed in high and low TILs (CD8+ TILs, CD4+ TILs, IFN-γ+ Th1, IL4+ Th2, and Foxp3+ Treg) groups. The results showed no significant differences in age, gender, smoking history, ECOG PS score, pathological type, and other characteristics between patients in the high and low expression groups (Supplementary Table 2).
Predictive significance of TILs for the efficacy of immunotherapy
The median PFS was significantly longer in the CD8+ Thigh group than in the CD8+ Tlow group (6.8 vs. 4.3 months; p = 0.039; HR: 0.59; 95% CI: 0.35-1.01). Similarly, the median OS of the CD8+ Thigh group was also better than that of the CD8+ Tlow group (12.6 vs. 9.8 months; p = 0.015; HR: 0.53; 95% CI: 0.30-0.92) (Supplementary Figure 2(a–b)). A similar trend was observed in the CD4+ Thigh group with a significantly longer median PFS (6.5 vs. 4.2 months; p = 0.014; HR: 0.54; 95% CI: 0.31–0.93) and median OS (12.9 vs. 8.7 months; p < 0.01; HR: 0.41; 95% CI: 0.23–0.73) than that of the CD4+ Tlow group (Supplementary Figure 2(c–d)). These results suggest that patients with high infiltration density of CD8+ TILs and CD4+ TILs have a better prognosis after immunotherapy.
In the CD4+ TILs subset analysis, the median PFS was significantly longer in the Th1high group than in the Th1low group (6.3 vs. 4.3 months; p = 0.049; HR: 0.60; 95% CI: 0.36–1.03), as was the median OS in the Th1high and Th1lowgroups (12.9 vs. 8.9 months; p < 0.01; HR: 0.46; 95% CI: 0.26–0.80) (Supplementary Figure 3(a–b)). These results suggest that high levels of IFN-γ+ Th1 can be used as a biological indicator of a good response to immunotherapy. Although the median PFS (6.1 vs. 4.5 months; p = 0.373) and median OS (12.3 vs. 9.3 months; p = 0.062) of the Th2high group was higher than that of the Th2low group (Supplementary Figure 3(c-d)), there was no significant difference between the two groups. Therefore, although patients with higher IL-4+ Th2 expression tended to have better outcomes with immunotherapy, IL-4+ Th2 could not be considered an independent factor for predicting the efficacy of immunotherapy. Further, in the Treghigh and Treglow groups, the median PFS was significantly lower in the Treghigh group (5.3 vs. 6.5 months; p = 0.021; HR: 1.78; 95% CI: 1.04–3.01) (Supplementary Figure 3(e)). The median OS was also significantly lower in the Treghigh group than in the Treglow group (10.1 vs. 12.6 months; p = 0.014; HR: 1.86; 95% CI: 1.07–3.25) (Supplementary Figure 3(f)). These results indicate that high numbers of Foxp3+ Treg are a negative predictor of the patient’s response to ICIs.
Nomogram construction and validation
Univariate and multivariate Cox analyses revealed that the timing of immunotherapy and the density of CD8+ TILs and Foxp3+ Treg could be potential predictive markers for the outcome of immunotherapy (p < 0.05) (). We then constructed a nomogram prediction model based on the above independent risk factors to predict 6-, 9-, and 12-month OS (). The C-indices of the training cohort and validation cohort were 0.723 (95% CI: 0.682–0.764) and 0.793 (95% CI, 0.738–0.848), respectively. The AUC of the training cohort and validation cohort demonstrated that the nomogram had good accuracy and discrimination (). Further, the calibration curves of the training cohort and validation cohort showed that the predicted values were consistent with the observed values, indicating that the nomogram prediction model had good prediction accuracy ().
Discussion
Currently, the sensitivity and response to immunotherapy vary because of individual differences between patients with advanced NSCLC. There are ICIs exhibiting promising efficacy in the treatment of NSCLC that is currently approved by the FDA as first-line therapy for advanced or metastatic NSCLC, consolidation therapy after radiotherapy and chemotherapy in patients with unresectable locally advanced NSCLC, and adjuvant therapy after surgical resection and chemotherapy in patients with respectable NSCLC. However, resistance to ICIs in lung cancer is also a common problem, either when patients are insensitive to their efficacy or when rapid disease progression occurs after a transient response [Citation15]. Although PD-L1 and TMB are predictive of response to immunotherapy in the clinic, accurately predicting which patients will respond to ICIs is still tricky [Citation16]. Therefore, it is necessary to continue to search for suitable biomarkers to predict the efficacy of immunotherapy and thus identify the patients who are responsive to ICIs.
Characterizing the immune infiltrate could facilitate the evaluation of the treatment efficacy of immunotherapy. Many studies suggest that immune-inflamed tumors, which have a higher density of CD8+ TILs than immune-desert tumors, can elicit a potent immune response [Citation17,Citation18]. In the present study, we confirmed that patients with a high infiltrate density of CD8+ TILs had a better prognosis than patients with a low infiltrate density (p < 0.05). The CD4+ TILs also have indispensable functions in anti-tumor immune responses. However, due to the phenotypic and functional heterogeneity of CD4+ T cells, different subsets are expected to play different and even opposing roles in the tumor environment [Citation19,Citation20]. This study found that patients with high expression of IFN-γ+ Th1 have stronger responses to ICIs (p < 0.05), which may be closely related to the fact that IFN-γ can induce an increase in PD-L1 levels on the surface of macrophages and tumor cells [Citation21]. In parallel, Ayers et al. found that the TME of tumors responsive to pembrolizumab treatment had a common feature of active IFN-γ signaling [Citation21], consistent with the findings herein. In contrast to the well-defined roles of Th1 cells in cancer immunity, the functions of IL-4-producing Th2 cells are more ambiguous. In the study, although patients with high IL-4+ Th2 expression had some benefits in immunotherapy, there was no significant difference (p > 0.05). According to Bretscher’s Th2-skewing hypothesis, successful anti-tumor immunity is mediated by Th1 and CD8+ T cells. In contrast, the universal mechanism of tumor escape involves a substantial Th2 component in the anti-tumor immune response. This hypothesis was proposed on the basis that the Th1 and Th2 differentiation pathways are known to antagonize each other [Citation22,Citation23]. However, because of the limitations of analytical techniques and specimen number, further studies are still needed to explore the relationship between the two. The presence of Treg cells in the TME severely hinders ICIs from exerting their anti-tumor effect. The results of this study confirm that patients with low Foxp3+ Treg infiltration density respond significantly better to immunotherapy than patients with high infiltration density (p < 0.05). Treg cells are thought to promote cancer development by suppressing CD8+ T cell function [Citation24,Citation25]. Besides, Foxp3+ Treg can significantly upregulate the expression of surface proteins PD-1, Tim-3, CTLA-4, and LAG-3, resulting in a highly immunosuppressive phenotype [Citation26,Citation27].
The univariate and multivariate Cox analyses revealed that CD8+ TILs, Foxp3+ Treg, and the timing of immunotherapy were independent prognostic factors (p < 0.05). To be better able to guide the application of immunotherapy in the clinic, we also constructed a nomogram prediction model based on this analysis. The prediction model exhibits good discrimination and prediction accuracy in both the training cohort and validation cohort.
Our study demonstrates the impact of TILs on the efficacy of immunotherapy from multiple aspects. However, this study has certain limitations, including the retrospective study design and the imbalance in clinical characteristics between groups that can create inherent biases. The study results may also be biased due to the influence of sample size and sample specificity. Further, TILs subpopulation analysis techniques include immunohistochemistry or flow cytometry, both of which have limitations. However, TILs play a vital role in the tumor immune microenvironment, and our research further confirms their predictive role in the efficacy of patients undergoing immunotherapy and their excellent potential as an emerging immunotherapy biomarker. Besides, current studies suggest that the predictive power of any single biomarker is limited. Constructing an integrated prediction model combining multiple biomarkers and monitoring techniques is perhaps the best solution to the impediments of ICIs’ efficacy prediction. We believe that TILs will be an integral part of a comprehensive prediction model in the future.
Ethical approval
This study was conducted in accordance with the Declaration of Helsinki and was approved by the Institutional Review Board/Ethics Committee of Shandong Cancer Hospital and Institute (Reference No. SDTHEC201905001). Written informed consent was acquired from each patient prior to sample collection.
Authors’ contributions
Wenjie Zhang and Sumei Li contributed equally to this work.
Supplemental Material
Download PDF (473.8 KB)Acknowledgments
We would like to thank all the participants who contributed to this article.
Disclosure statement
No potential conflict of interest was reported by the author(s).
Data availability statement
The datasets used and/or analyzed during the current study are available from the corresponding author upon reasonable request.
Correction Statement
This article was originally published with errors, which have now been corrected in the online version. Please see Correction (http://dx.doi.org/10.1080/0284186X.2023.2257429)
Additional information
Funding
References
- Mamdani H, Matosevic S, Khalid AB, et al. Immunotherapy in lung cancer: current landscape and future directions. Front Immunol. 2022;13:823618. doi: 10.3389/fimmu.2022.823618.
- Melosky B, Juergens R, Hirsh V, et al. Amplifying outcomes: checkpoint inhibitor combinations in first-line non-small cell lung cancer. Oncologist. 2020;25(1):64–77. doi: 10.1634/theoncologist.2019-0027.
- Qiao M, Jiang T, Ren S, et al. Combination strategies on the basis of immune checkpoint inhibitors in non-small-cell lung cancer: where do we stand? Clin Lung Cancer. 2018;19(1):1–11. doi: 10.1016/j.cllc.2017.06.005.
- Badalamenti G, Fanale D, Incorvaia L, et al. Role of tumor-infiltrating lymphocytes in patients with solid tumors: can a drop dig a stone? Cell Immunol. 2019;343:103753. doi: 10.1016/j.cellimm.2018.01.013.
- Karachaliou N, Gonzalez-Cao M, Crespo G, et al. Interferon gamma, an important marker of response to immune checkpoint blockade in non-small cell lung cancer and melanoma patients. Ther Adv Med Oncol. 2018;10:1758834017749748. doi: 10.1177/1758834017749748.
- Mazzaschi G, Madeddu D, Falco A, et al. Low PD-1 expression in cytotoxic CD8(+) tumor-infiltrating lymphocytes confers an immune-privileged tissue microenvironment in NSCLC with a prognostic and predictive value. Clin Cancer Res. 2018;24(2):407–419. doi: 10.1158/1078-0432.Ccr-17-2156.
- Remon J, Facchinetti F, Besse B. The efficacy of immune checkpoint inhibitors in thoracic malignancies. Eur Respir Rev. 2021; 30(162):200387. doi: 10.1183/16000617.0387-2020.
- Jardim DL, Goodman A, de Melo Gagliato D, et al. The challenges of tumor mutational burden as an immunotherapy biomarker. Cancer Cell. 2021;39(2):154–173. doi: 10.1016/j.ccell.2020.10.001.
- Gibney GT, Weiner LM, Atkins MB. Predictive biomarkers for checkpoint inhibitor-based immunotherapy. Lancet Oncol. 2016; 17(12):e542–e551. doi: 10.1016/s1470-2045(16)30406-5.
- Chan TA, Yarchoan M, Jaffee E, et al. Development of tumor mutation burden as an immunotherapy biomarker: utility for the oncology clinic. Ann Oncol. 2019;30(1):44–56. doi: 10.1093/annonc/mdy495.
- Zhang L, Chen Y, Wang H, et al. Massive PD-L1 and CD8 double positive TILs characterize an immunosuppressive microenvironment with high mutational burden in lung cancer. J Immunother Cancer. 2021;9(6):e002356. doi: 10.1136/jitc-2021-002356.
- Pang Z, Chen X, Wang Y, et al. Comprehensive analyses of the heterogeneity and prognostic significance of tumor-infiltrating immune cells in non-small-cell lung cancer: development and validation of an individualized prognostic model. Int Immunopharmacol. 2020;86:106744. doi: 10.1016/j.intimp.2020.106744.
- Ameratunga M, Asadi K, Lin X, et al. PD-L1 and tumor infiltrating lymphocytes as prognostic markers in resected NSCLC. PLOS One. 2016;11(4):e0153954. doi: 10.1371/journal.pone.0153954.
- Hu-Lieskovan S, Lisberg A, Zaretsky JM, et al. Tumor characteristics associated with benefit from pembrolizumab in advanced non-small cell lung cancer. Clin Cancer Res. 2019;25(16):5061–5068. doi: 10.1158/1078-0432.Ccr-18-4275.
- Zhang F, Bai H, Gao R, et al. Dynamics of peripheral T cell clones during PD-1 blockade in non-small cell lung cancer. Cancer Immunol Immunother. 2020;69(12):2599–2611. doi: 10.1007/s00262-020-02642-4.
- Bie F, Tian H, Sun N, et al. Research progress of anti-PD-1/PD-L1 immunotherapy related mechanisms and predictive biomarkers in NSCLC. Front Oncol. 2022;12:769124. doi: 10.3389/fonc.2022.769124.
- Yu Y, Zeng D, Ou Q, et al. Association of survival and immune-related biomarkers with immunotherapy in patients with non-small cell lung cancer: a meta-analysis and individual patient-level analysis. JAMA Netw Open. 2019;2(7):e196879. doi: 10.1001/jamanetworkopen.2019.6879.
- Durgeau A, Virk Y, Corgnac S, et al. Recent advances in targeting CD8 T-cell immunity for more effective cancer immunotherapy. Front Immunol. 2018;9:14. doi: 10.3389/fimmu.2018.00014.
- González-Navajas JM, Elkord E, Lee J. Editorial: CD4(+) T cells in cancer immunotherapies. Front Immunol. 2021;12:737615. doi: 10.3389/fimmu.2021.737615.
- Oja AE, Piet B, van der Zwan D, et al. Functional heterogeneity of CD4(+) tumor-infiltrating lymphocytes with a resident memory phenotype in NSCLC. Front Immunol. 2018;9:2654. doi: 10.3389/fimmu.2018.02654.
- Ayers M, Lunceford J, Nebozhyn M, et al. IFN-γ-related mRNA profile predicts clinical response to PD-1 blockade. J Clin Invest. 2017;127(8):2930–2940. doi: 10.1172/jci91190.
- Frafjord A, Buer L, Hammarström C, et al. The immune landscape of human primary lung tumors is Th2 skewed. Front Immunol. 2021;12:764596. doi: 10.3389/fimmu.2021.764596.
- Gao S, Hsu TW, Li MO. Immunity beyond cancer cells: perspective from tumor tissue. Trends Cancer. 2021; 7(11):1010–1019. doi: 10.1016/j.trecan.2021.06.007.
- Ganesan AP, Johansson M, Ruffell B, et al. Tumor-infiltrating regulatory T cells inhibit endogenous cytotoxic T cell responses to lung adenocarcinoma. J Immunol. 2013;191(4):2009–2017. doi: 10.4049/jimmunol.1301317.
- Hu X, Gu Y, Zhao S, et al. Elevated circulating CD4(+)CD25(-)Foxp3(+) regulatory T cells in patients with nonsmall cell lung cancer. Cancer Biother Radiopharm. 2019;34(5):325–333. doi: 10.1089/cbr.2018.2672.
- Whiteside TL. FOXP3+ treg as a therapeutic target for promoting anti-tumor immunity. Expert Opin Ther Targets. 2018; 22(4):353–363. doi: 10.1080/14728222.2018.1451514.
- Alemohammad H, Najafzadeh B, Asadzadeh Z, et al. The importance of immune checkpoints in immune monitoring: a future paradigm shift in the treatment of cancer. Biomed Pharmacother. 2022;146:112516. doi: 10.1016/j.biopha.2021.112516.