Abstract
Introduction
The optimal launch and adoption of novel medicines require effective navigation of the commercial and regulatory landscape, including three key gatekeeper groups: regulators, payors, and prescribers. Traditionally, despite lacking generalisability, the pharmaceutical industry provides Phase III randomized controlled trial evidence to gatekeepers. Despite additional real-world evidence (RWE) improving the generalisability of evidence, the provision of RWE remains in its infancy. The industry may use a multiple stakeholder approach to RWE generation to address each group's priorities and expectations effectively.
Methods
The Jandhyala Method observed a consensus of expert opinion on the expected effects of supplementing randomised controlled trial evidence (RCTE) with RWE on the depth and time to maximal adoption. The study recruited participants from two of the three gatekeeper stakeholder groups (prescribers and payors) and their four aligned industry stakeholder groups (market access, commercial, medical affairs, and regulatory affairs) groups involved in the approval or adoption of investigational medicines.
Results
The observed consensus predicted a shortened time to maximal adoption by 22% and increased maximal adoption by 31% of a new medicine with additional RWE at launch. The suggested rationales for these effects varied across stakeholder groups and between specific effects. The results found the prescriber gatekeeper to be dominant in driving the predicted shortening of adoption. No such dominant subsystem was identified for the expected increase in adoption depth, although a potential rationale was the broader population observed in the supplementary RWE.
Conclusions
The proposed model provides an intuitive temporal framework within which subsystem logic can be further researched to identify and address stakeholder groups’ needs and weigh those needs. Preliminary results appear to support adopting a multiple stakeholder approach to RWE for augmenting the uptake of novel medicines.
Introduction
The adoption of novel medicines depends on effective navigation of the commercial and regulatory landscape, including engagement with three key gatekeeper groups: regulators, payors, and prescribers. However, approaches for engaging with these groups (both individually and collectively) remain poorly studied. Modelling and optimising engagement with these gatekeeper groups may lead to more patients receiving the benefits of new medicines sooner. Under systems theoryCitation1 the adoption of a medicine can be modelled as an open system that interacts with its environment. Within this system, the three gatekeepers exist as subsystems, each employing their own “black box” logic to inputs they receive to generate their output of a decision which, if favourable, allows the medicine to progress to the next subsystem in the series ().
Figure 1. The medicine adoption model. Abbreviations. PH–: non-pharmaceutical company stakeholder groups; PH+: pharmaceutical company stakeholder groups.
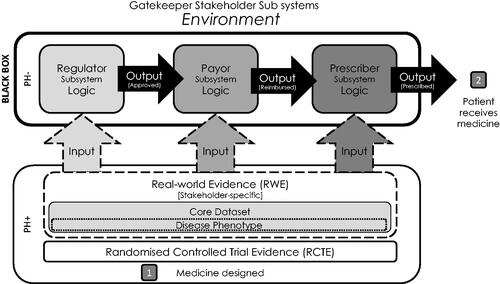
Regulatory bodies such as the European Medicines Agency (EMA) and the Food and Drug Administration (FDA) form the regulatory subsystemCitation2. Pharmaceutical companies applying for approval of a new medicine must provide them with suitable inputs in the form of evidence demonstrating the safety and efficacy of their novel medicine in its target disease populationsCitation3,Citation4. With sufficient evidence, the regulatory gatekeeper logic is satisfied, and authorisation is granted to market the medicine to prescribers and for revenue to be generated commercially.
Following this regulatory approval, the payor subsystem must be navigated. An appropriate engagement involves providing a suitable input of evidence to present a compelling logical case to the relevant payor bodies for the cost-effectiveness and ability of novel medicines to meet a clear clinical need in the specified therapeutic area. In addition, medicines must justify the reimbursement price requested.
Finally, the prescribers must be logically encouraged with appropriate evidential inputs to select the novel medicine over the others available to complete the sequence of gatekeeper events to achieve adoption in the medicine reaching the patient. These three gatekeepers base their decisions to approve the progression of the medicine on the inputs or evidence they are given. These gatekeepers, or stakeholders, have been formally examined for heterogeneity in their requirements from patient registries, a vehicle for delivering RWECitation5. The results justify a multiple stakeholder approach to generating RWE specifically targeted to their individual research questions and confirm a critical mismatch between the pharmaceutical company and gatekeeper stakeholders in these quality indicators of 54%Citation5.
Regulators consider evidence from Phase III randomised controlled clinical trials (RCTE) as the gold standard level of evidence to inform their decisionCitation6. However, the lack of generalisability of trial results to the broader population limits their utility for other stakeholders, especially in payor discussions. This limited generalisability is due to the highly selected patient populations they recruitCitation7 and the lack of neutralityCitation8 or accuracyCitation9 of endpoints they use when measuring changes in the disease phenotype on an intervention. Therefore, it is reasonable to assume that supplementing RCTE with evidence generated with real-world patients (RWE) could improve the overall body of evidenceCitation10. This RWE is optimally generated via patient registries, using gatekeeper stakeholder-relevant endpoints such as disease-specific instruments to measure disease severity and quality of lifeCitation9. From this multiple stakeholder perspective, recent research on the optimal configuration of such patient registries in rare diseases highlighted the importance of a core dataset and the need to engage with patients in the study designCitation5.
Several authors have suggested various definitions of RWE, some of which differ materially. In this study, RWE is the scientific knowledge generated on the level of the individual outside the confines of the clinical trials directive, answering research questions in a more generalised, real-world patient populationCitation11. Similarly, the expected effects of arriving at launch of a new medicine with RWE in addition to RCTE was defined as: an increase in the depth of maximal adoption and reduction in the time to maximal adoption, compared to a reference for RCTE alone.
The recently issued EMA guidance on the use of RWE in initial marketing authorisation approval applications, highlight the increasing recognition of the versatility and utility of this evidenceCitation12. However, the use of RWE in regulatory submissions is a new concept. Consequently, the benefits of RWE in addition to or in place of RCTE at the point of regulatory submission and during payor negotiations are currently unclearCitation13. Furthermore, due to the novelty of this approach, there appears to be a lack of information that could be used to inform whether supplementing clinical data with RWE is of any benefit to regulatory submissions or payor discussionsCitation13.
The patient remains the focus of all efforts involved in the adoption of new medicine. Therefore, any impacts of RWE in addition to RCTE on the overall number of patients treated or maximum adoption and the time to reach this peak would also be valuable in justifying the extra investment required in generating the RWE. In addition, detailed knowledge of the black box logic employed by the gatekeeper subsystems by which this influence is delivered would be invaluable for fine-tuning RWE generating strategies for maximal effect.
However, there is a shortage of evidence on this subject, and observed case data may take time to emerge. Therefore, generating a model based on the expert opinion of relevant representatives of pharmaceutical and non-pharmaceutical industry stakeholder groups (SHGs) on their expectations of an effect presents an initial hypothesis-generating approach to address this data gap.
This study used the Jandhyala method, a novel group awareness and consensus observing methodologyCitation14 differentiated from existing consensus-generating methodologies through its passive observation of consensus, efficient participant engagement profile, and critical metrics of participant awareness and item consensusCitation15.
Study objectives
This study has four objectives. To:
quantify the expected effects of supplementing RCTE with RWE on the depth of maximal adoption and time to maximal adoption.
identify the supporting rationale (SR) for any expected changes to the depth of maximal adoption and time to maximal adoption.
assess the extent to which different stakeholder groups proposed different supporting rationales, and
assess the extent to which different supporting rationales may apply to changes in the depth of maximal adoption and time to maximal adoption.
Methods
Jandhyala method
This method comprises two anonymised survey rounds, named “the Awareness Round (1)” and “the Consensus Round (2),” that are administered to identified subject-matter experts via the use of an online survey platformCitation14. Expert consensus is achieved by observing levels of awareness and subsequent consensus on the answers solicited by list-regenerating research questions via these two anonymised online surveys. In doing so, an awareness index and consensus index is calculated for each answer in the overall list of responses for each of the questions.
For this study, the Awareness Round (1) solicited free-text responses to open-ended questions, and results were anonymised, coded, and thematically analysed into items. An awareness index, which measures the frequency of an item as a factor of the most commonly occurring item in the study, was also calculated. Three researchers refined responses into mutually exclusive supporting rationales using content analysis and open codingCitation16,Citation17. The codes were attributed to the relevant participants' answers by one researcher before being confirmed by a second researcher.
Finally, the survey questions were constructed to verify the hypothesised expectation of an effect of launching with RWE in addition to RCTE, capture the size of the expected effect, and a list of the supportive rationale for the expected effect on the parameters of the depth of maximal adoption and time to maximal adoption.
The following questions were used in the survey:
In considering the adoption of a new medicine, from your experience, how much would you agree with the statement that:
Arrival at EMA/FDA approval with RWE and registration studies increases maximal adoption – maximal adoption is defined as the peak number of prescriptions.
If you have registered a degree of agreement, please explain how/why in the free text boxes.
Suppose a medicine launched with Phase III alone achieved a maximal adoption of 100 patients, on average. How many patients would you expect a medicine launched with both Phase III and RWE to achieve, on average, at its maximal adoption (e.g. 120 patients)?
In considering the adoption of a new medicine, in retrospect, how much would you agree with the statement that:
Arrival at EMA/FDA approval with RWE and registration studies shortens the time to maximal adoption – maximal adoption is defined as the peak number of prescriptions.
If you have registered a degree of agreement, please explain how/why in the free text boxes.
If a medicine launched with Phase III alone achieved a time to maximal adoption of 100 months, on average, how many months would you expect a medicine launched with Phase III + RWE to achieve its maximal adoption in comparison (e.g. 75 months).
All participants were provided ten free-text fields and asked to fill a minimum of three of them with their supporting rationales for the expected effect on each parameter. The participants who completed the first round participated in the Consensus Round (2) survey. In this round, participants rated their level of agreement with the inclusion of each supporting rationale arising from the Awareness Round (1) survey in a final and exhaustive list of grounds explaining the expected effect of launching a new medicine with RWE in addition to RCTE on the depth of maximal adoption and time to maximal adoption.
The participants scored their level of agreement with each supporting rationale on a 5-point Likert scale (1 – Strongly Disagree, 2 – Disagree, 3 – Neither Agree nor Disagree, 4 – Agree, 5 – Strongly Agree). A consensus index was generated from the Consensus Round (2) survey responses, this time focusing on the levels and proportions of agreement (agree or strongly agree ratings). Supporting rationales reaching a consensus level of >50% (CI > 0.5) were retained in the final list.
Study participants
This study recruited 47 participants between May 2019 and January 2020 from the author’s professional network and advertisements on professional social media networking sites. Potential participants needed to have had experience in RWE in rare diseases from the following industry (pharmaceutical) and non-industry (non-pharmaceutical) stakeholder groups (SHGs): Market Access experts (MAcc), Commercial experts (Comm), Medical Affairs experts (MAff), Regulatory Affairs experts (RAff), Prescribers (Pres), and Payors (Payo).
Non-pharmaceutical stakeholder participants comprised seven Payors and nine Prescribers. Pharmaceutical stakeholder participants included seven Commercial experts, six Market Access experts, 13 Medical Affairs experts, and five Regulatory Affairs experts.
Statistical analysis
Quantitative and qualitative statistical methods provided an estimate of the additive effect of RWE to RCTE on the depth of maximal adoption and time to maximal adoption. In doing so, the supporting rationales for each of the respective expected effect sizes were identified along with the heterogeneity among stakeholders’ supporting rationales and, finally, the degree of differentiation between the supporting rationales for two effects.
Quantitative analysis
Quantitative data solicited from participants regarding the expected effect size in the increase in the depth of maximal adoption (number of patients) and the decrease in the time to maximal adoption (number of months) were summarised using the median and inter-quartile range (IQR) for each stakeholder group. The reduction in the time to maximal adoption is provided as a decrease from a reference of 100 months for RCTE alone. The increase in maximal adoption was an increase from a reference value of 100 patients with RCTE alone. Consensus on supporting rationales for both effect sizes was also obtained.
The hypothesis that there is expected to be an increase in the depth of maximal adoption and a decrease in the time to maximal adoption in RWE combined with RCTE versus RCTE alone was tested using the Wilcoxon rank-sum test, based on values from participants of all stakeholder groups. In addition, the hypothesis of heterogeneity among stakeholder groups for an increase in the depth of maximal adoption and a decrease in time to maximal adoption was tested using the Kruskal-Wallis test. Finally, posthoc multiple comparisons were performed using Dunn's test to explore whether statistically significant heterogeneity among stakeholder groups existed.
The distributions of effect size in an increase in the depth of maximal adoption and a decrease in the time to maximal adoption among stakeholder groups are presented using box plots. The correlation between the increase in the depth of maximal adoption and reduction in the time to maximal adoption for RWE combined with RCTE versus RCTE alone are presented using a scatterplot. The overall correlation among all stakeholder groups and the correlation for each group that indicates the association between the increase in the depth of maximal adoption and the decrease in the time to maximal adoption are presented using Spearman's rank correlation coefficients.
Qualitative analysis
From the perspective of multiple stakeholders, the list of supporting rationales for the expected increase in the depth of maximal adoption, and the decrease in the time to maximal adoption, was extracted from evidence generated using the Jandhyala method. The analysis for heterogeneity among stakeholders of supporting rationales for the effect sizes was achieved by measuring the heterogeneity per item among stakeholders using individual participant-level consensus scores.
Furthermore, Chi-square tests were performed to assess the homogeneity of emergent consensus scores among stakeholders for each item. The degree of differentiation between the supporting rationales for each effect was evaluated using unique, non-unique, and aligned supporting rationales (SRs) per effect. Further analyses were conducted on the classification of each supporting rationale in the activity referred to within the adoption system: input and logic through which the former influenced the latter.
Results
Statistical analysis
Quantifying the expected effects of supplementing RCTE with RWE on the depth of maximal adoption and the time to maximal adoption
Reduction in the time to maximum adoption
When questioned on the expected effect of RWE combined with RCTE at regulatory approval of a new medicine would have on any reduction in time taken to reach maximal adoption of the medicine, all stakeholders agreed that there would be a beneficial reduction in time taken (p < .0001). Furthermore, when asked to quantify the size of the effect, the average decrease in the time to maximal adoption was recorded as 24.94 months per 100 months. However, there was a moderate level of heterogeneity (p = .0127) between the responses given by different stakeholder groups ().
Figure 2. Expected beneficial effect on time to maximal adoption (tmAd) of a new medicine when arriving at its launch with phase III and real-world evidence. Abbreviations. Comm, Commercial; MAcc, Market Access; MAff, Medical Affairs; Raff, Regulatory Affairs; Payo, Payors; Pres, Prescribers.
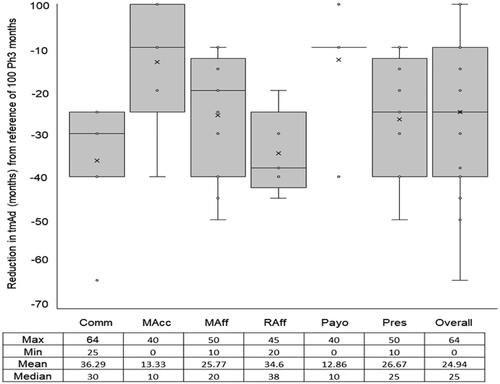
Payors and Market Access stakeholders predicted the shortest average reduction in time to maximal adoption (12.86 and 13.33 months per 100 months, respectively). In contrast, Regulatory Affairs stakeholders indicated the longest average decrease in time to maximal adoption (34.6 months per 100 months). Despite the variability, these results, which are predictions based on the effect of RWE combined with phase III studies, are far less than the average reduction in time to maximal adoption (100 months), which are predicted based on the effect of Phase III studies alone.
Increase in the depth of maximal adoption
All participants (N = 47) agreed that there would be a beneficial increase in the depth of adoption (i.e. an increase in the number of patients receiving the new medicine) when arriving at launch with RWE combined with RCTE (p < .0001). The mean increase in depth of maximal adoption was 30 patients per 100 patients () with a moderate level of homogeneity (p = .1716).
Figure 3. Expected beneficial effect on depth of maximal adoption (dmAd) of a new medicine when arriving at its launch with phase III and real-world evidence. Abbreviations. Comm, Commercial; MAcc, Market Access; MAff, Medical Affairs; Raff, Regulatory Affairs; Payo, Payors; Pres, Prescribers.
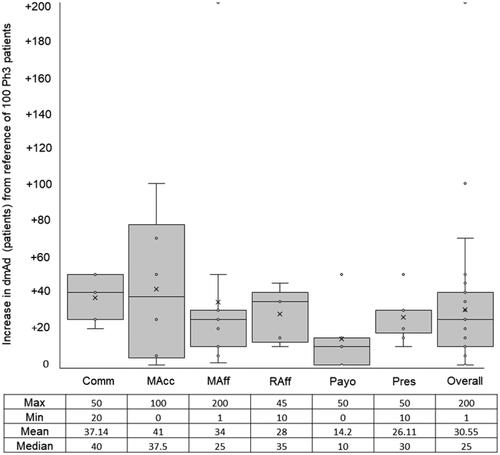
The Comm stakeholder group predicted the highest mean increase in the depth of maximal adoption of 40 patients. In contrast, Payors predicted the lowest mean increase of depth of maximal adoption of 10 patients. Aside from the Payor stakeholder group, there was greater consistency in the responses to this question by the remaining SHGs. These results, which are predictions based on the effect of RWE combined with RCTE, are far higher than the pre-defined reference mean increase of depth of maximal adoption (100 patients), which are predicted based on the effect of Phase III studies alone at launch.
Correlation between reduction in the time to maximum adoption and the depth of maximal adoption
There was a weak positive correlation between the time to maximum adoption and the depth of maximal adoption. For each participant, a higher value of an increase in maximal adoption is associated with a higher reduction in time to maximal adoption and vice-versa (). The points of different colours are interspersed, indicating a lack of heterogeneity in the said association among stakeholder groups ().
Figure 4. Expected beneficial effect on time to maximal adoption (tmAd) and depth of maximal adoption (dmAd) of a new medicine when arriving at its launch with phase III and real-world evidence. Abbreviaitons. Comm, Commercial; MAcc, Market Access; MAff, Medical Affairs; Raff, Regulatory Affairs; Payo, Payors; Pres, Prescribers.
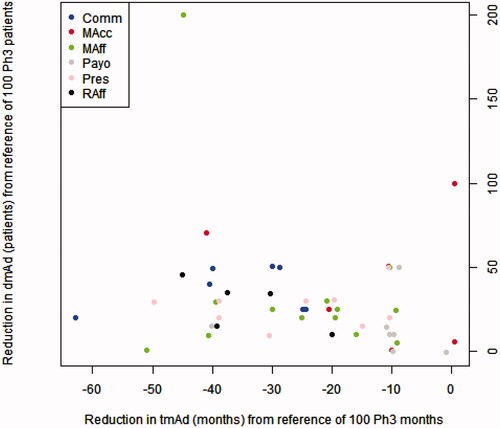
Identifying supporting rationales for any expected changes due to the depth of maximal adoption and time to maximal adoption
The expected reduction in the time to maximal adoption
The stakeholder groups suggested 28 supporting rationales explaining the expected effect size in reducing the time to maximal adoption, with more than four SHGs suggesting 15/28 (53.57%). “Increase in volume of evidence in non-clinical trial outcomes,'” Increase in volume of evidence on the safety, and 'Increase in volume on effectiveness’ was unanimously suggested and agreed on. “Encourages prescribers to prescribe” was the most frequently offered logical rationale supporting this effect size. This supporting rationale resided in the prescriber gatekeeper subsystem.
It is important to note that some supporting rationales (SRs) meeting the consensus threshold for inclusion were identified as influencing both the time to maximal adoption and the depth of maximal adoption. In (showing time to maximal adoption), supporting rationales with an effect on the depth of maximal adoption are identified in the Partner column. Similarly, (showing depth of maximal adoption) supporting rationales with an effect on the time to maximal adoption are identified in the Partner column.
Table 1. Analysis of SRs above the consensus threshold for inclusion (CI > 0.50) in ≥ 4 SHGs and their heterogeneity of consensus scores regarding the time to maximal adoption (tmAd).
Table 2. Analysis of SRs above the consensus threshold for inclusion (CI > 0.50) in ≥ 4SHGs and their heterogeneity of consensus scores regarding the depth of maximal adoption (dmAd).
The expected increase in the depth of maximal adoption
Seven out of the 35 (20%) supporting rationales were suggested by the SHGs to explain the expected increase in the depth of maximal adoption. “Increases the volume of evidence on safety and 'increases volume of evidence on effectiveness’ were both common inputs to those supporting rationales for the time to maximal adoption. “Improves understanding of the burden of disease' and 'improves understanding of unmet patient needs” were the most frequently suggested logic supporting rationales for this effect. The latter resided in the prescriber gatekeeper subsystem. Other supporting rationales suggested by more than four stakeholder groups were “Encourages regulators to grant approval” and “Encourages regulators to approve a broader indication.”
Assessing the extent to which different stakeholder groups proposed different supporting rationales
The expected reduction in the time to maximal adoption
Absolute heterogeneity of a stakeholder group was defined by suggesting at least one supporting rationale unique to that group. A total of 28 discrete (unique and non-unique) supporting rationales were indicated for the expected effect size in reducing time to maximal adoption. In addition, 6/29 were unique to a single stakeholder group. Thus, 3/6 stakeholder groups (1/2 non-pharmaceutical SHG and 2/4 pharmaceutical SHGs) met this threshold: Prescribers 1/6 (16.67%), Commercial 4/6 (66.67%), and Regulatory Affairs 1/6 (16.67%). Commercial SHGs were responsible for suggesting many of the unique supporting rationales. This difference reached statistical significance (p = .049) when compared to Prescribers and Regulatory Affairs stakeholder groups.
The expected increase in the depth of maximal adoption:
A total of 35 discrete (unique and non-unique) supporting rationales were suggested for the expected effect size in increasing the depth of maximal adoption, and 15/35 were unique to a single stakeholder. Thus, 5/6 SHGs (1/2 non-pharmaceutical SHG and 4/4 pharmaceutical SHGs) met the threshold for absolute heterogeneity: Payor 2/15 (13.33%), Commercial SHG 6/15 (40.00%), Market Access SHG 1/15(6.67%), Medical Affairs SHG 1/15 (6.67%) and Regulatory Affairs SHG 5/15 (33.33%). No statistically significant differences were detected between the proportions of unique to total supporting rationales across these stakeholder groups. The prescriber stakeholder group was the only group not to suggest a unique supporting rationale for this effect size ().
Table 3. Number of Unique Supporting Rationales (SRs) suggested by SHGs as a proportion of the total number of SRs suggested regarding time to maximal adoption (tmAd) and depth of maximal adoption (dmAd).
Assessing the extent to which different supporting rationales may apply to changes in the depth of maximal adoption and time to maximal adoption
A total of 28 and 35 discrete supporting rationales were solicited for the reduction in the time to maximal adoption and an increase in the depth of maximal adoption, respectively. 18/28 (62.07%) and 24/35 (68.57%) were unique to their respective effects of time to maximal adoption and depth of maximal adoption. Both effects shared twelve supporting rationales, nine were identical in wording, and three were consistent in theme or concept. The effect size of reducing time to maximal adoption was confirmed to have been driven by the dominant Prescriber subsystem (p = .012). In the effect size of the depth of maximal adoption, no subsystem was dominant in driving this effect size (p = .263) ().
Table 4. Supporting Rationales (SRs) for expected effect sizes by subsystem, commonality and mode of effect: via input or logic.
Discussion
This original research sought to understand the expected effects of RWE in addition to Phase III studies on the three gatekeeper subsystems in the medicine adoption system, as described by pharmaceutical and non-pharmaceutical stakeholder groups. This would justify the implementation of plans to generate RWE in addition to RCTE for submission at the earliest possible opportunity, at regulatory submission. In doing so, identifying the supporting rationale from each of their perspectives for these expected effects enabled a detailed understanding of their nature in terms of the interpreted nature of the input and the logic employed by each subsystem. Finally, the previously generated hypothesis justifying the multiple stakeholder approach to RWE generation could be confirmed by finding further differentiating features of the stakeholder groupsCitation5.
Quantifying the expected effects of supplementing RCTE with RWE on the depth of maximal adoption and time to maximal adoption
The mean expected effect size of coming to launch with RWE in addition to RCTE were profound for both time to maximal adoption and depth of maximal adoption, −24.94 months per 100 months of RCTE alone, and +31 patients per 100 patients with RCTE alone, respectively. There was, however, heterogeneity across the SHGs suggesting a moderate differential in the overall supportive, positive effect. The expected impact of additional RWE at launch was in line with the regulatory authorities' recommendations to submit such evidenceCitation18.
Identifying supporting rationales for any expected changes to the depth of maximal adoption and time to maximal adoption
For both effects, valuable explanatory detail was extracted from their SHG suggested supporting rationales in whether constituted an “input” into or “logic” within the relevant gatekeeper black box subsystem. Inputs generally comprised direct effects on the environment, e.g. the increase in the volume of evidence of one kind or another. Logic explanations included inferences on how evidence within the subsystem was appraised, such as “Assists pricing negotiations” and “Reduces payor uncertainty,” supporting rationales for the time to maximal adoption effect. Each logic item identified here informs a further discrete research question around the characteristics of the RWE, which contribute to a favourable outcome in assisting pricing negotiations or reducing payor uncertainty.
In considering the increase in the depth of maximal adoption, certain high-frequency logic supporting rationales such as “Encourages regulators to approve a broader label” can be seen to increase the population available to adopt the medicine directly. The complementary effect of documented experience in a real-world population alongside evidence from a controlled clinical trial setting may, at regulatory submission, conceivably offer a pathway to a broader label through demonstrating effectiveness in conjunction with efficacy.
Assessing the extent to which different SHGs proposed different supporting rationales
Three stakeholder groups (Prescriber, Commercial, and Regulatory Affairs) demonstrated absolute heterogeneity in their unique explanations for the effect on time to maximal adoption. The Commercial stakeholder group showed relative heterogeneity by suggesting significantly more unique supporting rationales for the observed effect than the other two SHGs. Importantly Prescriber stakeholder group was the only non-pharmaceutical SHG to suggest a unique supporting rationale for the observed effect.
Only the Prescriber stakeholder group did not suggest a unique supporting rationale for the observed effect for the depth to maximal adoption. In contrast, all others stakeholder groups contributed homogenously to the supporting rationales for the observed effect. These results support the findings in earlier work on the need to consider the needs of the multiple stakeholder groups individuallyCitation5. In addition, these data demonstrate heterogeneity in requirements and a differing rationale for the opinions held on contributing factors to the effect sizes.
Assessing the extent to which different supporting rationales may apply to changes in the depth to maximal adoption and time to maximal adoption
The absolute heterogeneity of the supporting rationales in the effect size between the two groups demonstrates the need to consider the two effects as discrete endpoints with different contributing inputs and logic. However, a degree of commonality in supporting rationales was noted, with 12 (nine common, three aligned) represented in both effects. Furthermore, a differential in the dominant subsystem was detected for time to maximal adoption with a significantly larger number of supporting rationales relating to the Prescriber subsystem than for the Regulator and Payor. The inference here is that prescribers require careful consideration in RWE design and planning, as the ultimate gatekeeper. The risk of not doing so would be to disproportionately restrict the time to maximal adoption.
Limitations
Two limitations should be taken into consideration when interpreting the findings from this study. Firstly, all 47 participants in this study were recruited via the extended professional network of the author and had access to online facilities to enable participation in the study. This has highlighted the potential for bias and the generalisability of the findings. Secondly, given the characteristics of study participants, it is essential to note that the views and opinion of other relevant stakeholders less likely to have an online presence might have been missed in the data generated from this study. Finally, it is recognised that some stakeholder groups are more inclined towards the inclusion of RWE in medicine adoption submissions. Hence, this should be considered when interpreting the result of this study.
Conclusion
The participants considered the expected effect of arrival at launch of a new medicine with RWE in addition to RCTE compared to RCTE alone on time to maximal adoption and depth of maximal adoption via their effects on the medicine adoption model presented. RWE combined with RCTE at launch was expected to shorten the time to maximal adoption by 22.17 months per 100 months expected with RCTE alone. Furthermore, it was expected that the depth of maximal adoption under the same circumstances would increase by 31 patients per 100 RCTE patients.
The supporting rationales for these expected effects provided by the stakeholder groups demonstrated a degree of absolute and relative heterogeneity, supporting previous similar findings on the need to adopt a multiple stakeholder approach to RWE generation.
The supporting rationales themselves were amenable to being categorised into Input and Logic in the context of the effect additional RWE would be expected to have on each of the gatekeeper subsystems described. As a dominant gatekeeper subsystem, the prescriber stakeholder group accounted for the expected shortening of time to maximal adoption. No such dominant subsystem was identified for increasing the depth of maximal adoption. The absolute heterogeneity in the supporting rationales noted for each effect also suggested that the effects were largely independent of each other, and strategies aiming to influence them should also reflect a similar degree of independence.
Hence, the detailed description of the supporting rationales for the profound expected effect of RWE in addition to RCTE at launch not only justifies the need to develop RWE in good time for launch but also informs an understanding of the medicine adoption system within which new medicines exist. Thus, further work is warranted to describe the favourable characteristics of RWE capable of stimulating the subsystems’ logic and further optimising adoption.
Transparency
Declaration of funding
This paper received no funding.
Peer reviewer disclosure for declaration of financial/other relationships
Peer reviewers on this manuscript have received an honorarium from CMRO for their review work but have no other relevant financial relationships to disclose.
Ethics approval and consent to participate
All participants provided written informed consent to participate in this research study. It was determined using the NHS Research Ethics Committee (REC) review tool that the study did not require ethical approval to be conducted (certification available upon request).
Competing interests
The author is the Founder and CEO of Medialis Ltd, a medical affairs consultancy and contract research organisation involved in designing and delivering real-world evidence studies. All participants were offered remuneration for their time.
Acknowledgements
RJ would like to thank the biostatisticians and medical writer at Medialis Ltd for their statistical and editorial support and the RWE Multidisciplinary Advisory Panel members for participating in this research.
Data availability statement
The datasets used and analysed during the current study are available from the corresponding author on reasonable request.
References
- Silva H, Koski G, Whalen M, et al. A systems approach to enhance clinical research and medicines development. J Med Dev Sci. 2016;1(1):59–67.
- Smythe W, Kramer N. Investigational medicinal product (IMP) management. a comprehensive and practical guide to clinical trials. London (UK): Elsevier; 2017. p. 103–117.
- Nugent P, Duncan JN, Colagiovanni DB. Chapter 12 – the preparation of a preclinical dossier to support an investigational new drug (IND) application and first-in-human clinical trial. In: Faqi AS, editor. A comprehensive guide to toxicology in preclinical drug development. London (UK): Academic Press; 2013. p. 309–334.
- Van Norman GA. Drugs, devices, and the FDA: part 1: an overview of approval processes for drugs. JACC Basic Transl Sci. 2016;1(3):170–179.
- Jandhyala R. The multiple stakeholder approach to real-world evidence (RWE) generation: observing multidisciplinary expert consensus on quality indicators of rare disease patient registries (RDRs). Curr Med Res Opin. 2021;37(7):1249–1257.
- Bothwell LE, Greene JA, Podolsky SH, et al. Assessing the gold standard-lessons from the history of RCTs. N Engl J Med. 2016;374(22):2175–2181.
- Logviss K, Krievins D, Purvina S. Characteristics of clinical trials in rare vs. common diseases: a register-based Latvian study. PLOS One. 2018;13(4):e0194494.
- Jandhyala R. Neutral theory: a conceptual framework for construct measurement in clinical research (Pre-print). 2020. DOI:10.13140/RG.2.2.33212.16008
- Jandhyala R. Neutral theory: applicability and neutrality of using generic health-related quality of life tools in diseases or conditions where specific tools are available. BMC Med Res Methodol. 2021;21(1):86.
- Sherman RE, Anderson SA, Dal Pan GJ, et al. Real-world evidence – what is it and what can it tell us? N Engl J Med. 2016;375(23):2293–2297.
- Corrigan-Curay J, Sacks L, Woodcock J. Real-world evidence and real-world data for evaluating drug safety and effectiveness. JAMA. 2018;320(9):867–868.
- Girman CJ, Ritchey ME, Zhou W, et al. Considerations in characterizing real-world data relevance and quality for regulatory purposes: a commentary. Pharmacoepidemiol Drug Saf. 2019;28(4):439–442.
- Miksad RA, Abernethy AP. Harnessing the power of real-world evidence (RWE): a checklist to ensure regulatory-grade data quality. Clin Pharmacol Ther. 2018;103(2):202–205.
- Jandhyala R. A novel method for observing proportional group awareness and consensus of items arising from list-generating questioning. Curr Med Res Opin. 2020;36(5):883–893.
- Jandhyala R. Delphi, non-RAND modified Delphi, RAND/UCLA appropriateness method and a novel group awareness and consensus methodology for consensus measurement: a systematic literature review. Curr Med Res Opin. 2020;36(11):1873–1887.
- Khandkar SH. Open coding. University of Calgary. 2009;23.
- Stemler S. An overview of content analysis. Practical Assessment Res Eval. 2000;7(1):17.
- Thompson D, Graff J, Critchlow C, et al. 21st Century Cures Act and the Future of RWE Generation. ISPOR 2018 Symposium. Baltimore (MD): Syneos Health; 2018.