Abstract
Objective
Insulin pump use is increasing among people with type 2 diabetes (T2D), albeit at a slower rate compared to people with type 1 diabetes (T1D). Factors associated with insulin pump initiation among people with T2D in the real-world are understudied.
Methods
This retrospective, nested case-control study aimed to identify predictors of insulin pump initiation among people with T2D in the United States (US). Adults with T2D who were new to bolus insulin use were identified from the IBM MarketScan Commercial database (2015–2020). Candidate variables of pump initiation were entered into conditional logistic regression (CLR) and penalized CLR models.
Results
Of the 32,104 eligible adults with T2D, 726 insulin pump initiators were identified and matched to 2,904 non-pump initiators using incidence density sampling. Consistent predictors of insulin pump initiation across the base case, sensitivity, and post hoc analyses included continuous glucose monitor (CGM) use, visiting an endocrinologist, acute metabolic complications, higher count of HbA1c tests, lower age, and fewer diabetes-related medication classes.
Conclusions
Many of these predictors could represent a clinical indication for treatment intensification, greater patient engagement in diabetes management, or proactive management by healthcare providers. Improved understanding of predictors for pump initiation may lead to more targeted efforts to improve access and acceptance of insulin pumps among persons with T2D.
Introduction
Type 2 diabetes mellitus (T2D) is a chronic, progressive disease affecting an estimated 34.2 million people in the United States (US)Citation1. Many people with T2D may require treatment intensification with injectables, including insulin for their T2D management, when oral antihyperglycemic agents (OAHs) and lifestyle interventions fail to provide glycemic controlCitation2,Citation3. Real-world data from the US suggest that approximately 22–54% of people with T2D who initiate basal insulin therapy for glycemic control do not achieve recommended hemoglobin A1c (HbA1c) targets of <7%Citation4–7. Those who require further treatment intensification after basal insulin therapy may be prescribed prandial/mealtime insulin in the form of multiple daily injections (MDI) of basal-bolus insulinCitation2. However, evidence from commercial claims databases in the US suggest that less than a third of MDI-using persons with T2D achieve recommended glycemic targetsCitation6,Citation8. Insulin delivery with pumps, also known as continuous subcutaneous insulin infusion (CSII), is an alternative to MDI for insulin-requiring people with T2DCitation9. Insulin pumps were introduced in the 1970s for people with type 1 diabetes (T1D)Citation10 and can deliver basal and bolus insulin coverage via continuous infusion and boluses to cover mealsCitation11.
A recent study by Perez-Nieves et al.Citation12 reported a trend in the real-world use of insulin pumps by people with T2D, increasing from 3.2% to 4.4% between 2009 and 2018. However, this is significantly lower compared to its use among people with T1D (54.4%)Citation12, in part because not everyone with T2D will require insulinCitation13. Additionally, insulin pump technology can be complex for some people with T2D, especially for those diagnosed at a later stage in lifeCitation14. Other reasons for its low use among people with T2D could be the higher initial cost of the pump (versus MDI), and stringent requirements for reimbursement of pump and pump supplies by health insurance companies and Medicare in the USCitation14–17. People with T2D are generally cared for by primary healthcare providers who, unlike specialists such as endocrinologists, may have limited exposure to newer insulin devices and may be more likely to recommend MDI over insulin pumps for T2D managementCitation18,Citation19. Clinical guidelines support the use of the insulin pump as an individual choice for people with T2D who are on MDICitation20, since in some studies there is evidence of better glycemic control or greater treatment satisfaction for insulin pumps versus MDI among people with T2DCitation21–29. Evidence also suggests reductions in HbA1c and hypoglycemic events with the use of simplified insulin pumps and tubeless patch pumps in people with T2DCitation26,Citation27.
A better understanding of the characteristics of people with T2D who use insulin pumps in real-world clinical practice could help identify those most likely to be receptive to initiate insulin pump use and may help identify potential barriers to pump initiation. The current research aims to identify predictors of insulin pump initiation among people with T2D who are using bolus insulin in a real-world US population.
Methods
Data source
This retrospective, nested case-control study used administrative claims data from the IBM MarketScan Commercial databases between 2015 and 2020. The MarketScan Research databases contain individual-level, de-identified, healthcare claims from employers, health plans, hospitals, and Medicare and Medicaid programs across the US. The present study used claims data from the commercial database with labs. All data were fully de-identified and Health Insurance Portability and Accountability Act (HIPAA)-compliant. Ethics committee approval was not required due to the use of retrospective, de-identified data in this study.
Study population
Adults aged ≥18 years who were new to bolus insulin use, defined as having a first-observed insulin prescription between 1 January 2016 and 31 March 2020 with no bolus insulin prescription filled in the preceding 12 months, were identified from the database. This first-observed bolus insulin prescription was designated as the index date and the preceding 12 months as the baseline. Identified individuals were eligible for inclusion if they: (1) met the claims-based criteria for T2D evaluated 12 months pre-index (assessed using International Classification of Disease, 9th Revision, Clinical Modification [ICD-9-CM] codes of 250.x0, 250.x2 and ICD-10-CM codes of E11.xx; T2D claims ≥ T1D claimsCitation30; to increase the likelihood of identifying people with T2D and with no other type of diabetes, those with secondary diabetes were excluded: ICD-9-CM: 249.xx; ICD-10-CM: E08.xxx, E09.xx, or E13.xx), (2) had non-missing entries for age and sex, (3) had continuous medical and pharmacy enrollment ≥12-months pre-index and in the ≥6-month post-index period, (4) had no evidence of an insulin pump or pump supply claim any time pre-index or in the 6 month post-index period in order to identify incident insulin pump use, and (5) had an additional bolus insulin prescription claim in the 6 months post-index in order to ensure continued use of bolus insulin use ().
Figure 1. Study inclusion–exclusion criteria and attrition. Abbreviations. N, total number of people; n, number in each category; T2D, Type 2 diabetes mellitus. aIn the post hoc analysis, 620 pump initiators were matched (1:4) to 2,480 non-pump initiators. bThe number of pump initiators and matched non-pump initiators in the sensitivity analysis were the same as that in the base case analysis.
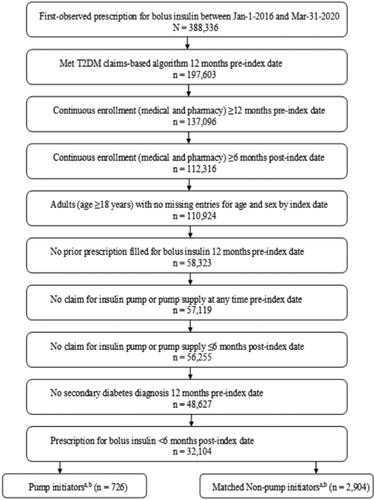
Study design
In this nested case-control study (), pump initiators (cases) and non-pump initiators (controls) were identified from those eligible for inclusion in the cohort. The cohort was followed until the earliest of: (1) the first pump claim, (2) loss of continuous enrollment, or (3) end of study period/end of data. Pump initiators were defined as individuals with a claim for an insulin pump observed at least 6 months post-index. This requirement ensured that the lookback period for insulin pump predictors would not overlap with the baseline period. For pump initiators, the date of the first-observed pump claim was defined as the lookback date. Pump initiators were matched (1:4) to non-pump initiators with replacement using incidence density samplingCitation31,Citation32, matching them for time at-risk (from index date to lookback date) and index date (± 6 months). As such, each non-pump initiator’s lookback date was set such that their time from first bolus insulin prescription to lookback matched the duration of this period for their respective pump initiator. A person who served as a non-pump initiator could subsequently become a pump initiator. Cohort selection and matching was implemented with an established incidence density sampling macroCitation32.
Figure 2. Study design. Note. The cohort was followed until the earliest of the following, i.e. “at-risk” period ends when: (1) a pump claim is observed (becomes a case), (2) loss of continuous enrollment, or (3) end of study period, i.e. end of data. Pump initiators and non-pump initiators were matched for (1) time at-risk and (2) index date (± 6 months). The index period was from 1 January 2016 to 31 March 2020. Including baseline, the study period was from 1 January 2015 to 31 March 2020.
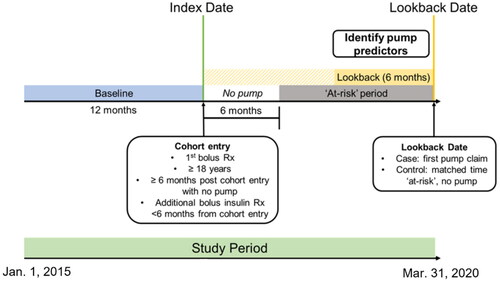
Study variables
Candidate variables associated with pump initiation among people with T2D were identified during the 6 months prior to lookback. Demographic characteristics such as age, region, and insurance type were assessed at index as their values were less likely to change compared to other candidate variables that were assessed 6 months prior to the lookback date (clinical, medication-use, healthcare resource utilization [HCRU]-related variables). Clinical variables included cardiovascular disease (atherosclerosis, other acute ischemic heart diseases, angina pectoris, chronic ischemic heart disease, myocardial infarction and complications of myocardial infarction, ventricular fibrillation and flutter, cardiac arrest, atrial fibrillation and flutter, subsequent myocardial infarction, heart failure, hypertensive heart disease with heart failure, hypertensive heart disease and chronic kidney disease with heart failure, aortic aneurysm and dissection)Citation33, neuropathy, peripheral vascular disease, retinopathy, hyperlipidemia, hypertension, pregnancy, sleep apnea, acute metabolic complications (e.g. ketoacidosis and hypoglycemia with coma), and Charlson Comorbidity Index [CCI]. Medication-related variables included number of diabetes medication classes, presence of bolus insulin prescription, count of 30-day basal and bolus prescription fills, and insulin medication combinations. HCRU-related variables included count of HbA1c tests, acute, non-acute, and any hypoglycemia visits, primary care provider (PCP) visits, endocrinologist visits, diabetes-related emergency room (ER) visits, number of outpatient or diabetes-related outpatient visits, proportion of diabetes-related outpatient visits to total outpatient visits, and continuous glucose monitor/flash glucose monitor (CGM/FGM) use. “Non-acute” hypoglycemia visits were identified using hypoglycemia diagnoses in any position that occurred in the outpatient setting, “acute” were identified using diagnosis codes in the principal or primary position and occurring in the ER, urgent care, or inpatient setting, and “any” included diagnosis codes in any position in the outpatient, inpatient, or ER/urgent care setting. HbA1c values that were not available were coded as missing. Similarly, if region and insurance type were unavailable, they were set to missing/unknown.
Statistical analysis
Differences between baseline and/or clinical characteristics for pump initiators and non-pump initiators were assessed using Chi-squared tests or the Fisher’s exact tests for categorical variables, and ANOVA or the Wilcoxon Rank Sum for continuous variables. Candidate variables () were evaluated using conditional logistic regression (CLR) and penalized conditional logistic regression (PCLR) with pump initiation as the outcome and the candidate variables listed in Supplementary Table S1 entered into the CLR and the PCLR models. For PCLR, the hyperparameter selection was assessed using 10-fold cross-validation. Model fits of the CLR and PCLR models were evaluated using Akaike information criterion (AIC) and the “best” model was determined by the lowest AIC. Predictors identified as significant by the PCLR (had the lowest AIC, Supplementary Table S1) were entered into a CLR model to calculate the odds ratios and associated 95% confidence intervals.
Table 1. Characteristics of pump initiators and non-pump initiators at cohort entry and on selected candidate variables measured 6 months prior to the lookback date.
Sensitivity analysis
A pre-planned sensitivity analysis was conducted that involved running the incidence density sampling without replacement. Also, a post hoc sensitivity analysis was conducted, such that in addition to the original base case matching criteria, pump and non-pump initiators were matched for having a bolus insulin prescription during the lookback period. Only pump and matched non-pump initiators with a bolus insulin prescription during the lookback period were included in the analysis. The data analysis, incidence density sampling, and summary statistics were generated using SAS 9.4 software. Copyright © [2016] SAS Institute Inc., Cary, NC, USA. CLR and PCLR were implemented with R v4.0.3 statistical software using the clogitL1 packageCitation34.
Results
Out of 388,336 bolus insulin users identified from the IBM MarketScan Commercial database, 32,104 adults with T2D were ultimately eligible for inclusion. From this cohort, 726 pump initiators were matched (1:4) to 2,904 non-pump initiators using incidence density sampling in the base case analysis and sensitivity analysis. In the post hoc analysis that required a bolus insulin prescription during the lookback period, 620 pump initiators were matched (1:4) to 2,480 non-pump initiators. In the base case analysis, 161 non-pump initiators served as a control for multiple pump initiators and 35 non-pump initiators eventually initiated a pump (i.e. became a case). In the sensitivity analysis where matching was done without replacement, no non-pump initiators were selected multiple times, but 37 non-pump initiators eventually became pump initiators. Lastly, in the post hoc analysis, 153 non-pump initiators served as a control for more than one pump initiator and 40 non-pump initiators eventually initiated a pump.
Demographic and clinical characteristics
presents the descriptive statistics for the overall cohort at baseline (N = 32,104). The mean age of the overall population was 51.8 years, approximately half of them were female (49.1%), from the South (51.3%), the majority of the population had fee-for-service insurance type (64.5%), and hypertension (73.4%) and hyperlipidemia (68.3%) were the most prevalent comorbidities. presents the descriptive characteristics for 726 pumps initiators and 2,904 non-pump initiators, measured at cohort entry as well as during the lookback period. Among the matched cases and controls, the mean age ranged from 47.9 to 51.3 years, about half were female, the South was the most represented region, and fee-for-service was the most common insurance type (). As expected, due to the nature of the incidence density sampling, there were no differences in the duration of at-risk period between pump and non-pump initiators ().
Table 2. Descriptive characteristics of the overall population at baseline.
Predictive modeling
Based on AIC, PCLR models were considered the best models for the selection of predictors for all scenarios (Supplementary Table S1). There were many significant predictors of insulin pump initiation identified in the base case (). The top five significant predictors (based on the absolute magnitude of the beta coefficients) and their odds ratios (OR) and 95% confidence intervals (CI) were: CGM/FGM use (vs. BGM use; OR = 5.39; 95% CI = 3.75–7.74), no insulin use (vs. using insulin only; OR = 0.21; 95% CI = 0.12–0.38), visiting an endocrinologist (OR = 2.74; 95% CI = 2.21–3.40), acute metabolic complications (OR = 2.50; 95% CI = 1.16–5.36), and Western US region (vs. North Central; OR = .56; 95% CI = .37–.85). Other significant predictors included peripheral vascular disease (OR = .62; 95% CI = .42–.92), count of HbA1c tests (OR = 1.36; 95% CI = 1.21–1.53), number of diabetes-related outpatient visits (OR = 1.08; 95% CI = 1.04–1.11), and hyperlipidemia (OR = 1.30; 95% CI = 1.02–1.64). Age was also a significant predictor (OR = .97; 95% CI = .96–.98) such that every 1-year increase in age was associated with a 3% lower odds of pump initiation. The following medication-use related predictors were also significantly associated with pump initiation: use of insulin plus OAHs (vs. using insulin only; OR = .67; 95% CI = .48–.94), using fewer diabetes-related medication classes (OR = .78; 95% CI = .67–.92), and a higher number of 30-day bolus insulin prescription fills (OR = 1.10, 95% CI = 1.04–1.17) ().
Figure 3. Forest plot of predictors of pump initiation, PCLR model* Odds Ratio (95% CIs) – Base Case analysis. Abbreviations. BGM, blood glucose meter; CCI, Charlson Comorbidity Index; CGM, continuous glucose monitor; CI, confidence interval; DM, diabetes mellitus; ER, emergency room; FGM, flash glucose monitor; GLP-1, glucagon-like peptide-1 receptor agonist; HbA1c, glycated hemoglobin; No., number of; OR, odds ratio; PCLR, Penalized Conditional Logistic Regression; PCP, primary care provider. Bold = significant at p < 0.05. Note. Bold = significant at p<0.05. *Non-pump users selected with replacement; Reference groups: (a) North Central; (b) Capitated insurance; (c) BGM only use; and (d) Insulin only.
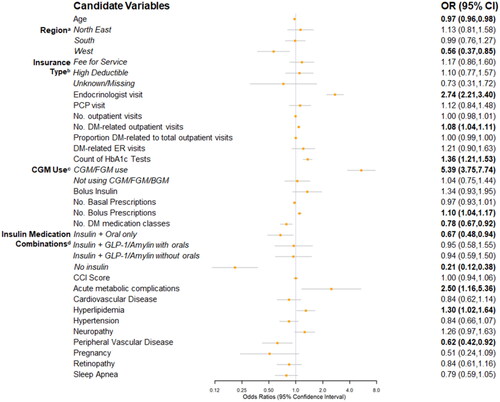
Sensitivity analysis
In the sensitivity analysis, significant predictors of insulin pump initiation were similar to those identified in the base case analysis, sharing four of the top five predictors (Supplementary Figure S1). Specifically, CGM/FGM use (vs. BGM use; OR = 6.14; 95% CI = 4.25–8.88), HbA1c >9% (vs. <7%; OR = 3.98; 95% CI = 1.11–14.35), acute metabolic complications (OR = 2.97; 95% CI = 1.40–6.32), and endocrinologist visits (OR = 2.56; 95% CI = 2.07–3.18) all increased the odds of pump initiation, while no insulin use (vs. using only insulin; OR = .20; 95% CI = .11–.37) decreased the odds of pump initiation. In addition to HbA1c, male gender (OR = .80; 95% CI = .65–.99), non-acute hypoglycemia visits (OR = 2.14; 95% CI = 1.31–3.47), and the presence of a bolus insulin prescription fill (OR = 2.20; 95% CI = 1.49–3.23) were significantly associated with insulin pump initiation in the sensitivity analysis but not in the base case (Supplementary Figure S1). Consistent with the base case analysis, increasing age was associated with lower odds of insulin pump initiation (OR = .96; 95% CI = .95–.97).
Post hoc analysis
When insulin prescription fills were required in the 6 month lookback period, the top five predictors of insulin pump initiation (based on the absolute magnitude of the beta coefficients) were CGM/FGM use (vs. BGM use; OR = 5.80; 95% CI = 3.96–8.50), acute metabolic complications (OR = 4.03; 95% CI = 1.82–8.93), endocrinologist visits (OR = 3.02; 95% CI = 2.38–3.83), any hypoglycemia visits (OR = 1.79; 95% CI = 1.15–2.78), and use of SGLT-2 inhibitors (OR = 1.69; 95% CI = 1.18–2.42; ). In addition to any hypoglycemia visits and prescription fills for SGLT-2 inhibitors, significant predictors of insulin pump initiation identified in the post hoc analysis but not the base case analysis were male gender (OR = 1.08; 95% CI = .85–1.35), inpatient visits (OR = .60; 95% CI = .40–.90), PCP visits (OR = 1.44; 95% CI = 1.07–1.95), and count of 30-day bolus insulin prescription fills (OR = 1.07; 95% CI = 1.01–1.12). Increasing age was associated with lower odds of insulin pump initiation (OR = .95; 95% CI = .94–.96), similar to findings from the base case and sensitivity analyses.
Figure 4. Forest plot of predictors of pump initiation, PCLR model* Odds Ratio (95% CIs) – Post Hoc Analysis. Abbreviations. BGM, blood glucose meter; CCI, Charlson Comorbidity Index; CGM, continuous glucose monitor; CI, confidence interval; DM, diabetes mellitus; ER, emergency room; FGM, flash glucose monitor; GLP-1, glucagon-like peptide-1 receptor agonist; HbA1c, glycated hemoglobin; No., number of; OR, odds ratio; PCLR, Penalized Conditional Logistic Regression; PCP, primary care provider; SGLT-2, sodium glucose transport protein 2. Note. Bold = significant at p<0.05. *Non-pump users selected with replacement. Reference groups: (a) North Central; (b) Capitated insurance; (c) BGM only use; (d) Insulin only. The “no insulin” category was removed since pump users and non-pump users were required to have an insulin prescription during the lookback period.
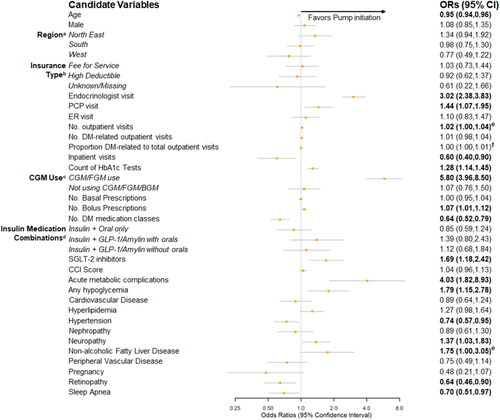
Discussion
This retrospective real-world study examined a US administrative claims database to identify predictors of insulin pump initiation among people with T2D. To the best of our knowledge, the present study is among the first to examine factors associated with pump initiation in people with T2D from a large, population-based datasetCitation35. Across all three analyses (base case, sensitivity, and post hoc), lower age, use of CGM or FGM, visiting an endocrinologist, presence of acute metabolic complications (ketoacidosis, hypoglycemia with coma), higher count of HbA1c tests, and fewer diabetes-related medication classes were significant predictors of insulin pump initiation.
CGM use was the strongest predictor of pump initiation across all analyses. Potential explanations may relate to greater diabetes engagement among persons willing to use CGM, greater comfort with diabetes technology for both CGMCitation36–38 and insulin pump, or treatment intensification because of greater diabetes progression and hypoglycemia risk leading to adoption of both CGM and insulin pumpCitation28. Another potential explanation for this association is that a persons’ insurance coverage may offer similar access to both a CGMCitation39 or insulin pump or a persons’ financial status may allow them to afford to use both technologies. Use of CGM technology provides insights about glycemic variability of the individuals, improves time in range, and reduces hypoglycemic eventsCitation40. This helps Health Care Providers (HCPs) to make informed decisions whether to initiate CGM and insulin pumps in individuals with sub-optimal glucose control.
Like CGM use, several other predictors of insulin pump initiation could be a proxy for intensified management by an HCP secondary to an individual’s clinical status. These include prescription fills for newer medications such as SGLT-2 inhibitors, higher numbers of diabetes-related outpatient visits, visiting an endocrinologist, higher counts of HbA1c tests, and fewer inpatient visits. However, as many people with diabetes who would benefit from treatment intensification do not do soCitation41, these predictors may also reflect increased diabetes engagement and/or particularly proactive diabetes management on the part of the clinician.
Results are consistent with known evidence that pump initiation is less likely among older adults with T2DCitation42. Studies have identified multiple age-related barriers to insulin pump initiation, including cognitive impairment, visual impairment, lack of access to diabetes educators or caregivers to assist with diabetes education and management, as well as reluctance to take up newer technologiesCitation14,Citation43. Research shows that there are a subset of older adults who benefit from pump useCitation44 and designing pumps with simplified features may facilitate uptake in this populationCitation45.
In some instances, insulin pump initiation in persons with T2DCitation44 and T1DCitation42,Citation46 may be triggered by a desire for treatment intensification following acute diabetes complications and hypoglycemia events. Consistent with this, the presence of acute metabolic complications and, in two analyses (post hoc and sensitivity analysis), the presence of hypoglycemia was associated with greater odds of pump initiation. Most hypoglycemic events are not coded in claims data and, thus, the association between hypoglycemia and insulin pump initiation may be stronger than we describeCitation47.
Some limitations of the study include: the lack of access and availability of some candidate predictors, choice of machine learning algorithms as well as access to CGM data. Data on insurance authorization, access to diabetes specialists or educators, social and economic factors affecting pump initiation and clinical characteristics such as weight and duration of T2D were unavailable. Glucose values from self-monitored blood glucose and CGM were unavailable and there was substantial missingness (>90%) of HbA1c values which limited our ability to evaluate the impact of glycemic status on pump initiation. Moreover, many of our observed predictors of pump initiation could covary according to a person’s financial status. The data for this study were drawn from a large insurance claims database which was limited to people in the US with commercial health coverage and, as such, may not be generalizable to other populations, including people on Medicare, Medicaid, or who are uninsured or self-insuredCitation48. In addition, both access to CGM data as well as linking CGM data to claims data is difficult and was unable to be done for this study. In instances where CGM data has been linked to claims, the data are limited in geographic scopeCitation49 or by the type of claims data (e.g. open) that are availableCitation50. Finally to our knowledge, there are no tree-based algorithms to model a conditional binary outcome, this translated to a limited choice in the building and assessment of the prediction models.
Conclusions
At this time, clinical guidelines recommend prescribing insulin pumps on an individual basis for insulin-requiring people with T2DCitation20. Improving our understanding of who may be receptive to pump initiation and of the potential barriers to pump initiation among persons with T2D will allow more targeted efforts to increase access to and adoption of this important diabetes management tool. While we could not measure these directly, potential barriers include the financial impact of pump initiation, limited personal health literacyCitation51, unequal access to an endocrinologist or other diabetes specialistsCitation28, and current complexity of insulin pumps that were not designed around the needs of older adults. Physician training programs to enhance familiarity with insulin pump options, new pumps focused on the needs of persons with type 2 diabetes, and initiatives to improve health equity and health literacy in this space may facilitate opportunities for persons with T2D to gain access to insulin pumps.
Transparency
Author contributions
Emily Hankosky contributed to the conception, design, interpretation, drafting, and critical revision of the manuscript. Michelle Katz was involved in the interpretation and critical revision of the manuscript. Ludi Fan and Chanadda Chinthammit contributed to the design, interpretation, and critical revision of the manuscript. Dongju Liu was involved in the data acquisition, data analysis, and critical revision of the manuscript. Alan Brnabic contributed to the conception, analysis, interpretation, drafting, and critical revision of the manuscript. Elizabeth Eby was involved in the conception, interpretation, and critical revision of the written manuscript. All authors read and approved the final version of the manuscript.
We have presented a part of the study findings as an abstract/poster at the International Society for Pharmacoeconomics and Outcomes Research (ISPOR) Europe Annual conference, 2021.
Supplemental Material
Download MS Word (249.8 KB)Acknowledgements
The authors would like to acknowledge Uma Jyothi Kommoju and Rajiv Ravishankar Ulpe, employees of Eli Lilly Services India Private Limited, for writing and editorial assistance. The authors would also like to acknowledge Amit Kumar Koushik, an employee of Eli Lilly Services India Private Limited, for providing writing support at the time of revision of the manuscript.
Declaration of funding
This study was funded by Eli Lilly and Company. Financial support for the conduct of the research and preparation of the article were provided by Eli Lilly and Company. Employees of Eli Lilly and Company participated in the study design, interpretation of study data, in the writing of the report, and in the decision to submit the article for publication.
Declaration of financial/other relationships
Emily Hankosky, Michelle Katz, Ludi Fan, Alan Brnabic, Dongju Liu, Chanadda Chinthammit, and Elizabeth Eby are full-time employees and stockholders of Eli Lilly and Company. Peer reviewers on this manuscript have no relevant financial or other relationships to disclose.
Data availability statement
The data that support the findings of this study are available from Merative L.P. (formerly IBM Watson Health), upon reasonable request. Restrictions apply to the availability of these data, which were used under license for this study.
References
- Centers for Disease Control and Prevention. National diabetes statistics report website; [cited 2022 Feb 3]. Available from: https://www.cdc.gov/diabetes/data/statistics-report/index.html.
- American Diabetes Association Professional Practice Committee 9. Pharmacologic approaches to glycemic treatment: standards of medical care in diabetes-2019. Diabetes Care. 2019;42(Suppl 1):S90–S102.
- Davies MJ, D’Alessio DA, Fradkin J, et al. Management of hyperglycaemia in type 2 diabetes, 2018. A consensus report by the American diabetes association (ADA) and the european association for the study of diabetes (EASD). Diabetologia. 2018;61(12):2461–2498.
- Peng XV, McCrimmon RJ, Shepherd L, et al. Glycemic control following GLP-1 RA or basal insulin initiation in real-world practice: a retrospective, observational, longitudinal cohort study. Diabetes Ther. 2020;11(11):2629–2645.
- Blonde L, Meneghini L, Peng XV, et al. Probability of achieving glycemic control with basal insulin in patients with type 2 diabetes in Real-World practice in the USA. Diabetes Ther. 2018;9(3):1347–1358.
- Brixner D, Ermakova A, Xiong Y, et al. Clinical and economic outcomes of patients with type 2 diabetes on multiple daily injections of basal-bolus insulin (MDI) therapy: a retrospective cohort study. Clin Ther. 2019;41(2):303–313.e1.
- Raccah D, Chou E, Colagiuri S, et al. A global study of the unmet need for glycemic control and predictor factors among patients with type 2 diabetes mellitus who have achieved optimal fasting plasma glucose control on basal insulin. Diabetes Metab Res Rev. 2017;33(3):e2858.
- Bonafede M, Chandran A, DiMario S, et al. Medication usage, treatment intensification, and medical cost in patients with type 2 diabetes: a retrospective database study. BMJ Open Diabetes Res Care. 2016;4(1):e000189.
- American Diabetes Association. Approaches to glycemic treatment. Diabetes Care. 2015;39(Supplement 1):S52–S59.
- Pickup JC, Keen H, Parsons JA, et al. Continuous subcutaneous insulin infusion: an approach to achieving normoglycaemia. Br Med J. 1978;1(6107):204–207.
- Pickup JC. Insulin-pump therapy for type 1 diabetes mellitus. N Engl J Med. 2012;366(17):1616–1624.
- Perez-Nieves M, Juneja R, Fan L, et al. Trends in U.S. Insulin use and glucose monitoring for people with diabetes: 2009–2018. J Diabetes Sci Technol. 2022;16(6):1428–1435.
- Basu S, Yudkin JS, Kehlenbrink S, et al. Estimation of global insulin use for type 2 diabetes, 2018–30: a microsimulation analysis. Lancet Diabetes Endocrinol. 2019;7(1):25–33.
- Bode BW. Insulin pump use in type 2 diabetes. Diabetes Technol Ther. 2010;12 (Suppl 1)::S17–21.
- National coverage determination (NCD) for infusion pumps. CMS website; [cited 2004 Dec 17]. Available from: http://www.cms.gov/medicare-coverage-database/details/ncd-details.aspx?NCDId=223.
- Ackermann RT, Wallia A, Kang R, et al. Comparative effectiveness and costs of insulin pump therapy for diabetes. Am J Manag Care. 2017;23(6):353–359.
- Centers for Medicare & Medicaid Services: Infusion Pumps: C-Peptide Levels as a Criterion for Use. Medicare National Coverage Determinations; [cited 2005 Feb 4]. Available from: https://www.cms.gov/Regulations-and-Guidance/Guidance/Transmittals/downloads/r27ncd.pdf.
- Lee LJ, Anderson J, Foster SA, et al. Predictors of initiating rapid-acting insulin analog using vial/syringe, prefilled pen, and reusable pen devices in patients with type 2 diabetes. J Diabetes Sci Technol. 2010;4(3):547–557.
- Daly A, Hovorka R. Technology in the management of type 2 diabetes: present status and future prospects. Diabetes Obes Metab. 2021;23(8):1722–1732.
- American Diabetes Association 7. Diabetes technology: standards of medical care in diabetes-2021. Diabetes Care. 2021;44(Suppl 1):S85–s99.
- Berthe E, Lireux B, Coffin C, et al. Effectiveness of intensive insulin therapy by multiple daily injections and continuous subcutaneous infusion: a comparison study in type 2 diabetes with conventional insulin regimen failure. Horm Metab Res. 2007;39(3):224–229.
- Wainstein J, Metzger M, Boaz M, et al. Insulin pump therapy vs. multiple daily injections in obese type 2 diabetic patients. Diabet Med. 2005;22(8):1037–1046.
- Reznik Y, Cohen O, Aronson R, et al. Insulin pump treatment compared with multiple daily injections for treatment of type 2 diabetes (OpT2mise): a randomised open-label controlled trial. Lancet. 2014;384(9950):1265–1272.
- Reznik Y, Morera J, Rod A, et al. Efficacy of continuous subcutaneous insulin infusion in type 2 diabetes mellitus: a survey on a cohort of 102 patients with prolonged follow-up. Diabetes Technol Ther. 2010;12(12):931–936.
- Pickup JC, Reznik Y, Sutton AJ. Glycemic control during continuous subcutaneous insulin infusion versus multiple daily insulin injections in type 2 diabetes: individual patient data meta-analysis and meta-regression of randomized controlled trials. Diabetes Care. 2017;40(5):715–722.
- Mader JK, Lilly LC, Aberer F, et al. Improved glycaemic control and treatment satisfaction with a simple wearable 3-day insulin delivery device among people with type 2 diabetes. Diabet Med. 2018;35(10):1448–1456.
- Layne JE, Parkin CG, Zisser H. Efficacy of a tubeless patch pump in patients with type 2 diabetes previously treated with multiple daily injections. J Diabetes Sci Technol. 2017;11(1):178–179.
- Grunberger G, Sze D, Ermakova A, et al. Treatment intensification with insulin pumps and other technologies in patients with type 2 diabetes: results of a physician survey in the United States. Clin Diabetes. 2020;38(1):47–55.
- Vigersky RA, Huang S, Cordero TL, et al. Improved HbA1c, total daily insulin dose, and treatment satisfaction with insulin pump therapy compared to multiple daily insulin injections in patients with type 2 diabetes irrespective of baseline C-peptide levels. Endocr Pract. 2018;24(5):446–452.
- Schroeder EB, Donahoo WT, Goodrich GK, et al. Validation of an algorithm for identifying type 1 diabetes in adults based on electronic health record data. Pharmacoepidemiol Drug Saf. 2018;27(10):1053–1059.
- Richardson DB. An incidence density sampling program for nested case-control analyses. Occup Environ Med. 2004;61(12):e59.
- Desai RJ, Glynn RJ, Wang S, et al. Performance of disease risk score matching in nested Case-Control studies: a simulation study. Am J Epidemiol. 2016;183(10):949–957.
- Wicke FS, Glushan A, Schubert I, et al. Performance of the adapted diabetes complications severity index translated to ICD-10. Am J Manag Care. 2019;25(2):e45–e49.
- R Core Team. 2021. R: a language and environment for statistical computing. Vienna, Austria: R Foundation for Statistical Computing.
- Hankosky E, Katz M, Fan L, et al. Characteristics of people with Type 2 diabetes initiating an insulin pump: data from a large commercial claims database - Poster presented at Advanced Technologies and Treatments for Diabetes, June 2-5, 2021 (Virtual). Diabetes Technology & Therapeutics; 2021. P190(#76).
- van Bon AC, Kohinor MJ, Hoekstra JB, et al. Patients’ perception and future acceptance of an artificial pancreas. J Diabetes Sci Technol. 2010;4(3):596–602.
- Borges U, Jr., Kubiak T. Continuous glucose monitoring in type 1 diabetes. J Diabetes Sci Technol. 2016;10(3):633–639.
- Tanenbaum ML, Adams RN, Hanes SJ, et al. Optimal use of diabetes devices: clinician perspectives on barriers and adherence to device use. J Diabetes Sci Technol. 2017;11(3):484–492.
- Association AD. Health equity and diabetes technology: a study of access to continuous glucose monitors by payer and race. Executive Summary; Arlington, VA; 2021.
- Battelino T, Alexander CM, Amiel SA, et al. Continuous glucose monitoring and metrics for clinical trials: an international consensus statement. Lancet Diabetes Endocrinol. 2023;11(1):42–57.
- Fu AZ, Sheehan JJ. Treatment intensification for patients with type 2 diabetes and poor glycaemic control. Diabetes Obes Metab. 2016;18(9):892–898.
- Meunier L, Aguadé AS, Videau Y, et al. Age, male gender, and social deprivation are associated with a lower rate of insulin pump therapy initiation in adults with type 1 diabetes: a Population-Based study. Diabetes Technol Ther. 2021;23(1):8–19.
- Stephens EA, Heffner J. Evaluating older patients with diabetes for insulin pump therapy. Diabetes Technol Ther. 2010;12(Suppl 1):S91–S7.
- Kumareswaran K, Thabit H, Leelarathna L, et al. Feasibility of closed-loop insulin delivery in type 2 diabetes: a randomized controlled study. Diabetes Care. 2014;37(5):1198–1203.
- Toschi E, Munshi MN. Benefits and challenges of diabetes technology use in older adults. Endocrinol Metab Clin North Am. 2020;49(1):57–67.
- Burckhardt MA, Smith GJ, Cooper MN, et al. Real-world outcomes of insulin pump compared to injection therapy in a population-based sample of children with type 1 diabetes. Pediatr Diabetes. 2018;19(8):1459–1466.
- Karter AJ, Moffet HH, Liu JY, et al. Surveillance of Hypoglycemia-Limitations of emergency department and hospital utilization data. JAMA Intern Med. 2018;178(7):987–988.
- IBM. MarketScan research databases for life sciences researchers. NY: IBM Watson Health; 2021.
- Mcqueen R, Pnm AG, Juneja R, et al. Longitudinal relationship between time in range and HbA1c in a real‐world clinical practice setting. Adv Technol Treatments Diab; Barcelona: Diabetes Technology & Therapeutics; 2022. p. A-1. A-237.
- Perez-Nieves M, Hankosky ER, Fan L, et al. 75-LB: use of continuous glucose monitoring (CGM) and its association with economic outcomes in the real-world setting. Diabetes. 2022;71(Supplement_1):75-LB.
- Agarwal S, Simmonds I, Myers AK. The use of diabetes technology to address inequity in health outcomes: limitations and opportunities. Curr Diab Rep. 2022;22(7):275–281.