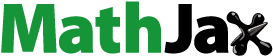
ABSTRACT
The COVID-19 pandemic forced universities to suspend face-to-face instruction, prompting a rapid transition to online education. As many lab courses transitioned online, this provided a rare window of opportunity to learn about the challenges and affordances that the online lab experiences created for students and instructors. We present results from exploratory educational research that investigated student motivation and self-regulated learning in the online lab environment. We consider two student factors: motivation and self-regulation. The instrument is administered to students (n = 121) at the beginning of the semester and statistically analysed for comparisons between different demographic groups. The results indicated students' major was the only distinguishing factor for their motivation and self-regulation. Students' unfamiliarity with online labs or uncertainty about what to expect in the course contributed to the lower levels of self-regulation. The lack of significant differences between various subgroups was not surprising, as we posit many students entered the virtual lab environment with the same level of online lab experience. We conducted interviews among these respondents to explore the factors in greater detail. Using latent Dirichlet allocation, three main topics that emerged: (1) Learning Compatibility, (2) Questions and Inquiry, and (3) Planning and Coordination.
1. Introduction
Online labs (i.e. remote or fully virtual labs) have long been considered a promising option for facilitating alternate lab experiences in STEM fields. However, to ensure students receive a worthwhile educational experience, it is important to ensure that the pedagogical and curricular value of online labs are comparable to their face-to-face counterpart. The COVID-19 pandemic forced universities to suspend face-to-face instruction, prompting instructors to rapidly transition to online instruction. This transition was challenging for many instructors in traditional STEM programmes, especially for engineering instructors who were also required to deliver labs as an integral part of their curriculum. For example, hands-on lab components are integral to the curricular experience for about 70% of Electrical Engineering (EE) and Computer Systems Engineering (CSE) required courses at the [university]. Similar to many other universities, the sudden shift from face-to-face to online instruction created uniquely challenging situations for the instructors of those courses.
Prior to the COVID-19 global pandemic, the college only offered two fully online undergraduate engineering courses (Engineering Statics and Fluid Mechanics). The courses were only offered during summer semesters, and neither of the two courses had lab components. However, our College had designed instruction with online experimentation technology through using both purchased online lab tools and self-developed online laboratory equipment in selected engineering courses.
Nevertheless, the onset of COVID-19 compelled an extensive, wholesale shift to all online instruction. As part of the transition, it was necessary to transform the semester-long, hands-on, experiential learning components of EE and CSE courses to fully online lab experiences. Beginning in April 2020, the college transitioned all instructions online, and by implication, laboratory instruction was also moved to various online formats to facilitate hands-on lab experiences. Although online lab experiences were not widely used before, we were able to leverage our earlier experiences with setting up small-scale online laboratories and our college’s prior intellectual and financial investment in online labs pre-COVID, to facilitate an extensive migration of course laboratory components to online formats.
Inevitably, the pandemic forced all instruction online with little or no time to contemplate the implications of the transitions on the efficacy of learning and pedagogy. The situation did not allow for students to choose whether they wanted their instructions in in-person or online modes. On the one hand, the sudden transition to online instructions presented uncharted challenges for instructors and students alike – especially because learning efficacy, self-directed learning, and students’ beliefs about online learning influence student outcomes (Bernard et al. Citation2004). Conversely, the COVID response also provided a rare window of opportunity to learn about the challenges and affordances that the online lab experiences created for students and instructors at college. As much as the broad pivot to online learning instigated unexpected educational challenges, it also afforded an unprecedented opportunity to investigate the roadblocks, challenges, and successes experienced by students who might not typically have participated in an online learning environment.
In this article, we draw on existing literature and share our experience with transitioning from traditional in-person labs our labs to online modes in order to manage instructional disruptions due to COVID. In addition, we present observations from exploratory educational research that investigated student motivation and self-regulated learning in the online lab environment as it was deployed. With the work presented in this paper, we do not simply discuss our reaction to COVID but also share insights into the transition process from hands-on lab instruction to online experimentation, broadly, and build fundamental understanding about student perceptions. We envisage that such investigation could provide insight for developing positive instructional online laboratory experiences for students and to better understand the challenges and opportunities associated with shifting face-to-face labs to the virtual space.
2. Background
2.1. Online laboratories in higher education
Laboratory and hands-on learning experiences in online STEM education have benefitted from recent technological innovations and developments that support alternative modalities for offering lab experiences. Online labs are currently facilitated through remote labs (where physically existing real equipment is controlled remotely), augmented reality (real existing labs are augmented by Virtual Reality add-ons), and virtual labs (via software-based fully virtual lab that are possible by simulation). These labs are referred to as ‘online labs’, ‘remote labs’, or ‘cross-reality labs’ in the literature (Brinson Citation2015; Faulconer and Gruss Citation2018; May Citation2020). Over the last decades, online laboratories (we use this term to include all non-traditional lab solutions that rely on the internet for lab–user interaction) have gained prominence because they can overcome some of the limitations of classical labs (Corter et al. Citation2007; Potkonjak et al. Citation2016). A number of educational research studies, including individual research efforts and broader literature analysis, have provided evidence to demonstrate the potential benefits of these online labs (Heradio et al. Citation2016; Hernández-de-Menéndez, Vallejo Guevara, and Morales-Menendez Citation2019; Estriegana, Medina-Merodio, and Barchino Citation2019). Some studies show that online laboratories can be efficient tools for engaging STEM students in authentic learning experiences, fostering conceptual understanding, stimulating self-paced learning, offering practical problem-solving experience, and for overcoming the geographic and temporal limitations of scheduling labs in higher education institutions (Kollöffel and de Jong Citation2013; Ma and Nickerson Citation2006).
Researchers are at polar ends of arguments for and against the efficacy of online labs. In a review of hands-on versus simulated and remote laboratories in engineering education, Ma and Nickerson (Citation2006) observed that online laboratories were especially well suited for teaching conceptual knowledge rather than design skills. They also found that students’ belief about the technology and whether they have options between participating in hands-on and online labs affects their lab curricular experiences. However, they also concluded that much of online lab research confound different factors in their studies, which makes it challenging to reach definite conclusions about students’ learning experiences in hands-on versus simulated and remote laboratories. About 10 years later, Brinson (Citation2015) published an extensive review of more than 50 empirical studies that examined different learning outcomes in virtual and remote labs versus traditional hands-on labs. Both reviews concluded that online labs had comparable effects on learning outcomes (e.g. understanding, inquiry skills, practical skills, perception, analytical skills, and social and scientific communication) as traditional hands-on labs under certain conditions (Brinson Citation2015; Ma and Nickerson Citation2006). However, there is yet no consensus in extant research about how effectively online labs facilitate positive learning experiences and outcomes. As such, there are calls for further studies to better delineate how online labs impact learning outcomes and experiential learning experience across instructional contexts (Brinson Citation2015; Brinson Citation2017).
Although there are several calls for more studies to examine the effects and the instructional perspective of online labs, a bibliometric analysis of more than 4000 published papers on virtual and remote labs conducted by Heradio et al. (Citation2016) showed that the themes of most research inquiries of online labs in the literature so far had coalesced around technical considerations for developing, managing, and sharing virtual labs; collaborative learning in virtual labs; and assessing the educational effectiveness of virtual labs. The authors argued that more fundamental educational research is needed to explore how different pedagogical modalities can be embedded within online labs to improve their efficacy for learning in non-traditional labs contexts. Although some evidence suggests that non-traditional labs can be as effective as traditional labs, most of the studies that have evaluated the strengths and weaknesses of online labs conclude that online lab research is quite diverse, and that there is not yet consensus around how to categorise the effectiveness of the various modes of implementing online labs to ensure effective lab-based learning.
2.2. Pre-COVID online lab initiative in the college
Prior to COVID, instructors in our college were exploring using online labs either to scale their lab offering or to provide pre-lab or post-lab engagement to students through an initiative led by the first author. Instructors who took part in the initiative developed and piloted remote lab modules in electrical, mechanical, and bio-engineering courses. For the electric engineering labs, in particular, Virtual Instrument Systems In Reality (VISIR) (Gustavsson et al. Citation2009; Garcia-Zubia et al. Citation2017) was adapted for use in an existing electric circuit course over one (May, Trudgen, and Spain Citation2019).
This prior trial with online labs served as a proof-of-concept for introducing and effectively using a ready-to-use remote lab like VISIR more extensively in existing circuits courses. In addition to demonstrating readiness for implementation, these initial efforts also pointed the team to educational research opportunities and directions that should be explored for a deeper understanding of how online labs platforms like VISIR facilitate learning in electrical engineering courses. Moreover, these initial experiences with VISIR served as a starting point for the development, acquisition, and assessment of further online laboratories (such as the EMONA system) in other scientific fields in the college (Al Weshah, Alamad, and May Citation2021; Pokoo-Aikins, Hunsu, and May Citation2019; Devine and May Citation2021; Li, Morelock, and May Citation2020). In retrospect, these initial experiences with online labs provided critical support to a seamless transition when the rapid shift to online occurred.
Initial feedback from an assessments of this pilot effort was generally positive: many students appreciated the flexibility that online labs afforded, and instructors who took advantage of the effort found online labs to be a useful component of their course instruction (May Citation2020; May, Trudgen, and Spain Citation2019). Although students’ feedback was supportive, they pointed out several areas for improvement to increase the instructional viability of the online labs. In our view of the pilot experiences, we also recognise the need to master how to integrate the lab into the local learning management system, which can add additional burden to instructional preparation for instructors.
Further, because students would have to learn at a distance, online labs need to motivate and stimulate student self-regulated learning to remain on task and schedule with lab work. Much of existing online lab research studies have focused on their technical development, their effects on student learning outcomes, and student satisfaction with online lab experiences. Nevertheless, there is a shortage of research on how online labs promote student motivation and self-regulated learning. Such studies could be critical to understanding how to design an online lab curriculum to facilitate meaningful learning engagement. These aspects of learning support are needed in general and especially in urgent situations, like the one caused by the COVID-19 pandemic in the 2020 and 2021. Consequently, this prior work with online laboratories positioned us to both effectively respond to the challenges induced by the pandemic and build educational research around the online experimentation efforts.
3. Implementing and studying online laboratories in electrical and computer engineering courses
Although a few instructors in our college were involved in exploring online labs, setting them up rapidly in response to the instructional disruptions that COVID caused was still quite challenging. However, we identified this challenging situation to be an ideal set-up for educational research work in the context of transitioning to online experimentation. This resulting research work is part of a project funded by the National Science Foundation to study the implications of instructional migrations due to COVID on learning experiences in engineering contexts. Our broader project integrates three perspectives in studying the online labs: instructor resistance to adoption, user experience and success, and student motivation and self-regulation. These perspectives respond to the necessary, widespread transition to online labs, including the perspectives of instructors and students who might not otherwise have participated in these environments.
This paper specifically responds to the need to explore students’ motivation and self-regulation in online labs. In the following sections, we describe the curriculum context and different types of online labs options implemented to support our EE and CSE courses during the COVID-19 instructional transitions, which build on the aforementioned pilot work. Then, we discuss our empirical investigation of online laboratories in electrical and computer engineering courses through two complementary approaches. First, we used a quantitative survey to explore students’ perceptions toward the online labs at the beginning of the semester. This quantitative approach was already framed by educational theory on student motivation and self-regulation, which operationalise the broader concept of emotional and cognitive student engagement. In a second step, we conducted interviews among these respondents to explore factors of motivation and self-regulation in greater detail. Data collected from human subjects in the form of surveys and interviews were approved by the university Institutional Review Board to ensure compliance.
3.1. Curricular context
The main context for the work presented here is the implementation of two similar, combined lecture and lab courses: ECSE 2170 – Fundamentals of Circuit Analysis, which is targeted toward EE and CSE students, and ENGR 2170 – Applied Circuit Analysis, which provides entry-level circuit analysis content for non-ECE majors. While ENGR 2170 serves as a breadth course for other engineering majors, in the EE and CSE curricula, ECSE 2170 is a critical prerequisite that opens up access to the core courses in the plan of study and, in turn, nearly all technical electives and capstone courses for EE and CSE students. ECSE 2170 serves approximately 100 students annually (about 15 in the summer term). ENGR 2170 serves approximately 250 students annually (about 50 in the summer term).
Though the depth and focus of these courses vary slightly for major and non-major versions, the courses have significant overlap in the learning outcomes and share common lab modules. Both courses provide exposure to analytical skills (i.e. skills required for the design and evaluation of circuits) and hands-on skills (i.e. skills required for building, testing, and debugging circuits). Under normal face-to-face instruction, these courses covered basic circuit analysis concepts in lecture and then reinforced those concepts through a series of lab activities requiring the use of circuit simulation software (e.g. PSPICE, MultiSim) before building the circuits using discrete components on a breadboard and then testing using standard electronic test equipment.
3.2. Online laboratories included in the study
To pivot to online instruction and in our research study, we implemented VISIR and the netCIRCUITlabs from Emona Instruments remote labs for electric circuit courses offered to students across the college. Both remote lab technologies offer online circuit building and testing environments that are suitable for lab activities in electric circuits.
The VISIR virtual workbench was designed to emulate the tactile learning experience that students receive when they build, power, and test a circuit in reality. The VISIR platform replicates the appearance and operational functionality of physical electronics lab bench equipment (i.e. moving components and rotating instrument knobs as you would on at physical lab bench). However, each students’ virtual lab bench can be accessed from any internet connection. Student users access the VISIR workbench (see ) remote lab environment through a web interface that enables them to use virtual versions of familiar benchtop instruments. In the VISIR workbench, students can access a virtual breadboard for building circuits with virtual wires and a set of basic discrete electronic components (e.g. resistors, capacitors, wires) to move onto their breadboard. A virtual power supply or a virtual function generator is used to power the circuits. Once properly wired, students can use additional instruments like a virtual multimetre or a virtual oscilloscope to take measurements and test the performance circuit. The developed virtual circuits are replicated using the VISIR technical back-end, which responds with real measurement data based on physically existing experimentation equipment.
Like the VISIR lab, the Emona TIMS netCIRCUITlabs system also offers online, remote access for multiple students to simultaneously control and measure real electronics circuits. The system is accessible via a web browser and covers a range of experiments such as AC amplifiers, feedback circuits, and differential amplifiers. To implement the remote lab modules presented in this study, student users used a web interface to access the circuit simulation software (see ). The further remote lab equipment comprises a technical back-end control unit and several switchable boards for different experiments.
Figure 2. Structural overview of netCIRCUITlabs remote lab with user interface and technical back-end.
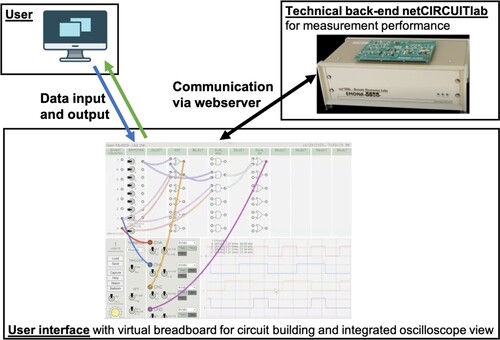
The VISIR and netCIRCUITlabs systems have several similarities but important differences. It is worth emphasising that these virtual lab systems are not simulation environments but remote laboratories using physically existing equipment for experimentation and data measurement. Circuits are physically built on the experiment boards, though they are accessed and controlled through a virtual interface. In both remote lab platforms, web interfaces enable connections to physical components that are connected through a reconfigurable switch matrix. Programmable voltage sources enable the implementation of power supplies and function generators and a build-in data acquisition card makes it possible to change voltage levels at nodes within a circuit. The web interface provides for both remote access to real physical hardware and is programmed to allow a test environment that replicates the appearance and functionality of equipment on a typical electronics test bench. Compared to VISIR, the netCIRCUITlabs system is more sophisticated with regard to the circuits building possibilities and experimentation procedures. Because of the switchable boards, it is also more flexible to be used in different lab courses such as introduction courses early in a curriculum or sensors later on in the study programme. But, based on our experiences, it is also promising to use both remote labs in combination in one course – to start with more basic circuits building using VISIR and switch to more sophisticated experimentation once the students’ abilities are more advanced.
Online labs in our course sessions were optimised as much as possible to create an experience close to those that students would have had in a traditional lab session. The web interface of these online lab platforms, in combination with video conferencing, made it possible to model nearly similar student–instructor and student–student interactions in the virtual labs as would have occurred in a regular face-to-face lab. In our regular labs, the instructor or a lab assistant would demonstrate the appropriate steps to build and test a circuit with the lab apparatus. Remote labs sessions were implemented via Zoom™ to facilitate this demonstration and collaborative engagement between students. By using the screen-sharing feature, it was possible for instructors to model the process in much the same way that had occurred in a face-to-face lab setting. Students who had challenges or questions could also use the shared screen feature to receive assistance from the lab instructor during real-time online lab-sessions.
Although the remote lab options do not fully provide the tactile experience of pushing components into a breadboard or attaching a measurement probe to a component lead as students would receive in a physical lab, the conceptual challenges that students encounter when they convert a circuit diagram to a breadboard layout remain. The systems also emulate how measurements probes are appropriately connected and referenced in circuit analysis. In fact, some instructors have observed that students make as many errors when making circuit connections and taking measurements in remote labs as they did when using physical labs. Moreover, unlike with traditional labs, the remote labs were not limited by geographic and time scheduling constrained. Because the remotes are always available online, students could work individually or in groups via Zoom on lab assignments outside of regular lab schedules. In such cases, technical difficulties or questions could be posted or emailed to their lab facilitators who addressed them asynchronously.
In the following sections, we will describe the education research study components, in which we build the empirical database. We will explain the quantitative study section first, followed by an in-depth description of the qualitative study section.
3.3. Quantitative study
Our first aim in exploring student motivation and self-regulation in online lab contexts is to characterise the perceptions of students taking the courses. In such learning environments, student cognitive and emotional engagement are instrumental (Devine and May Citation2021). We operationalise these as student self-regulation and motivation perspectives. We administered a portion of the Motivated Strategies for Learning Questionnaire (MSLQ) (Li, Morelock, and May Citation2020), aligned with these concepts, and conducted statistical analysis to make comparisons among demographic groups enrolled in the courses. The MSLQ is an instrument the investigators have used previously in educational research settings and are familiar with its applications (Kames et al. Citation2019).
3.1.1. Sampling and participants
Participants in the study were limited to students enrolled in undergraduate engineering courses that include online lab experiences (ECSE 2170 and ENGR 2170) during Summer 2020, the first complete semester with online labs following the outbreak of the COVID-19 pandemic, and Spring 2021, approximately one year from the shift to online instruction. During the academic year, instruction at [University] included hybrid learning, therefore, access to labs remained limited and the engineering courses included in the study continued to use online experimentation.
In total, responses were collected from 121 students between the semesters. Students responding to the research survey included both men and women, many racial identities, and students across majors and years of study in the college (see ). The subjects provided a wide spread of majors, though Mechanical Engineering students were a majority of students enrolled in the course. Additionally, the course did not possess a significant number of prerequisites and students could enrol in the course at nearly any point in their curriculum, resulting in students from freshman to seniors enrolled in the course.
Table 1. Participant demographic data.
3.1.2. Procedures and measurement
Students were invited to complete an electronic survey during the beginning of their enrolment in the courses. This quantitative approach is appropriate for capturing student perspectives broadly. In concentrating on how the transition to online lab instruction would impact student motivation and self-regulation, we selected four subscales of the MSLQ to consider the two student factors. The MSLQ is widely used in educational research to study motivation and self-regulation across learning contexts including engineering. Responses to the MSLQ are measured through a seven-point Likert scale scored from 1 (not at all true of me) to 7 (very true of me). Language in the subscales was slightly modified to relate to online labs. For instance, a question for motivation asked students to rate their agreement that ‘I think I will be able to use what I learn in these online labs in other courses’ and one question about self-regulation rated agreement that ‘When I complete online labs for this class, I set goals for myself in order to direct my activities in each study period’.
3.1.3. Data analysis and preliminary results
Students’ self-reported motivation for approaching the online labs ranged between 2.17 and 7 (x̄ = 5.31, σ = 0.92) and their perceived self-regulation was slightly lower on average, ranging from 2.00 to 6.58 (x̄ = 4.81, σ = 0.81). Both the motivation items and self-regulation items from the MSLQ were reliable (α = .88 and .81, respectively). The first step of the analysis was to compare motivation and self-regulation between semesters and conclude whether data could be integrated. Across semesters of enrolment students reported similar levels of motivation and self-regulation, supporting our simultaneous use of these data to understand how students approach online lab experiences in general.
To understand students’ initial perspectives when enrolling in the course with online labs, we compared motivation and self-regulation scores based on several demographic factors: gender, under-represented race status within engineering (i.e. non-White or Asian), major, and year of study. For demographic characteristics with two groups, a t-test was used; when more responses were possible, analysis of variance (ANOVA) tests were used.
There was no significant difference on motivation or self-regulation responses by gender or minority status (both t-tests). A one-way ANOVA test showed differences for motivation among the engineering majors, F(4, 116) 3.067, p = .019. Post-hoc comparison using a Tukey test showed that EE students had a significantly higher motivation in advance of the course when compared to Agricultural, Environmental, or Biochemical Engineering students (p = .024). CSE students’ motivation also trended higher than that of Agricultural, Environmental, or Biochemical Engineering students, but the difference was not significant (p = .087). Notably, there was no significant difference in self-regulation by major; across majors, students reported the same level of preparedness to manage the online lab materials. Neither was there a difference in motivation or self-regulation by students’ year of study – first-year and sophomore students had marginally higher motivation than upper-class students, but not at a significant level (p > .05).
3.2. Qualitative study
During students’ participation in the course, we extended invitations for in-depth interviews about their perceptions and experiences with online labs. The interview protocol mirrored the conceptual basis of the MSLQ, with questions about motivation and self-regulation, allowing students to elaborate on the factors influencing their engagement in the online labs. The completed interviews were then transcribed and analysed using latent Dirichlet allocation (LDA) (Blei, Ng, and Jordan Citation2003) to extract the topics spoken about by students. LDA is a topic modelling approach used to study text corpus datasets (Chen, Mullis, and Morkos Citation2021). The topics extracted using LDA are used to assist researchers’ focus in student interviews. For example, they describe the frequency of occurrence and sets of words used together and help gather greater insight in the qualitative analysis. By identifying salient topics across student interviews, and seeking to understand their meaning in the context of the interview, we can better understand the range of students’ experiences with the course.
3.2.1. Sampling and participants
Following completion of the quantitative survey, invitations to participate in an interview about the online labs were delivered to students. Interviews were conducted after students had participated in some online labs, either halfway through or at the end of the semester, to ascertain how student perspectives had developed throughout the semester. Two interviews were conducted with volunteers each semester. The interviews comprised four male students (three white and one Asian). Interviews ranged from 30 to 75 min as interviewees were free to elaborate as needed on each question.
3.2.2. Interview protocol
A formal interview protocol was developed using the interview protocol refinement (IPR) process (Yeong et al. Citation2018). This framework was selected to ensure interview questions were created systematically, in-line with the proposed research direction, while considering elements beyond the specific questions (Castillo-Montoya Citation2016; Rubin and Rubin Citation2005). Further, our prior experience (Clark et al. Citation2019; Shah et al. Citation2019) with IPR resulted in the successful collection of qualitative data that was used to reinforce quantitative findings. The IPR is composed of four steps, as shown in . These steps involve aligning interview questions with research questions or relevant themes in the study and formulating an inquiry-based conversation throughout the interview. The IPR supported developing questions to explore student motivation and self-regulation in greater depth.
Figure 3. Interview protocol refinement process (Yeong et al. Citation2018).
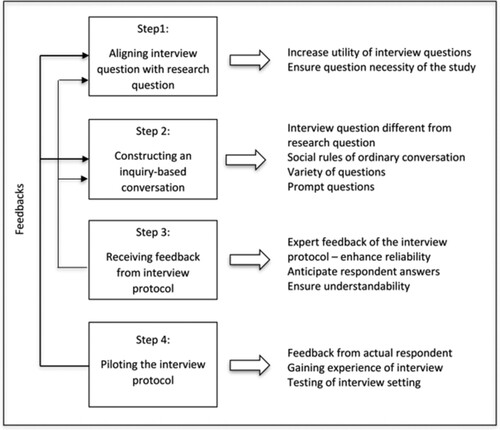
3.2.3. Latent Dirichlet allocation (LDA)
Interviews were completed by a member of the research team and transcribed by an external service. The results were examined by several members of the research team for accuracy before proceeding. A model representing the LDA process is shown in . LDA treats a given corpus compiled of all student interview transcriptions as a collection of words , where each document (
) consists of
words. Assuming each corpus contains a mix of interpretable topics, LDA constitutes a hierarchical model to approximate the topics-word and document-topic distributions (Yeong et al. Citation2018).
Figure 4. Graphical representation of LDA model (Blei, Ng, and Jordan Citation2003).
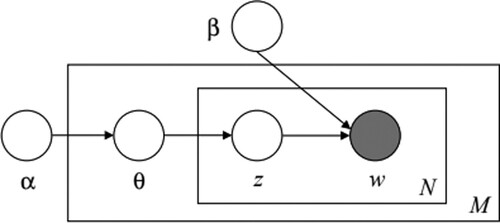
The important function that must be solved is the posterior given by Equation (1)
(1)
(1) where α is the Dirichlet prior for the distribution of topics, β is a topic–word matrix representing the probability of a word for each topic, θ follows a multinomial distribution of topics representing the probability of a topic in a document.
To solve for , we can identify a marginal distribution for the document as shown in Equation (2)
(2)
(2) Model parameters α and β are designed to be estimated (which can be accomplished through various estimation methods). Collapsed Gibbs sampling, a common-use algorithm, was performed to approximate posterior distribution for LDA.
Once approximated, a list of topics (
) is determined alongside the distribution θ. To evaluate the generalisation of each assigned topic, we consider perplexity, a statistical measure of how well the model can predict topics. Equation (3) denotes perplexity, where a lower score represents a better generalisation for a given corpus and model convergence. A tolerance number was set to 0.01, which will stop the calculation once perplexity improves by less than 1%. With a continuously increasing number of topics, the perplexity value will decrease and each associated word will become difficult to interpret. This threshold is used as a strategy to avoid model overfitting. With limited interviews available, an LDA training process was conducted to study the composition of underlying topics, and the testing process was validated by human judgment.
(3)
(3) Once topics were selected, the most frequent terms for each topic were analysed for their saliency (Chuang, Manning, and Heer Citation2012; Chuang et al. Citation2012) and relevance (Sievert and Shirley Citation2014). As shown in Equation (4), saliency measures the likelihood of
that a given word will convey information on topics (Chuang, Manning, and Heer Citation2012; Chuang et al. Citation2012).
(4)
(4)
Relevance measures the placement of a word to a specific topic given a weighted parameter () from 0 ≤
≤ 1, balancing the probability that a word appears in a topic
relative to the likelihood of its appearing over the entire corpus (Sievert and Shirley Citation2014).
(5)
(5)
3.2.4. Data analysis and preliminary results
The LDA was used to determine the topics generated from the corpus of interviews. Modelling began with data preprocessing, to remove noise from the text data (e.g. pluralities, suffixes). After stop-words filtering, the compiled document contained 721 unique words, which were lowercased, tokenised, and lemmatised for LDA analysis. We determined the optimal number of topics to be 11 by plotting the perplexity (Equation (3)) against the number of topics (). While lower perplexities are available at a higher number of topics, the results became saturated and difficult to interpret; instead, we selected a topic number that balances a succinct number of topics and a low perplexity. The words selected for each topic distribution are shown in .
Table 2. Word selections for each topic.
The 11 topics are plotted against the two principal components in , the intertopic distance map. The geometric size of each topic on the plot represents the marginal topic distribution allocation – the relative ‘importance’ of the topic or the probability of the topic in the corpus – with topic numbering in order of marginal topic distribution size (where Topic 1 has the greatest distribution).
Taking this information collectively – the words of each topic, the contextual use of these in the corpus, and the underlying structure of these topics – we labelled the main topics and discuss them. The results indicated there were minimal differences between students when compared quantitatively. However, the qualitative findings provided insight on the challenges students experienced and their approach to completing the lab.
4. Study discussion
Among demographic and enrolment characteristics, students’ major was the only distinguishing factor for their motivation and self-regulation approaching the online labs. Given the course content and expectation that the labs would relate to electronics, it is justifiable that students with certain engineering emphases (EE and CSE) might have greater intrinsic motivation for the experience. Furthermore, this was a prerequisite course in the plan of study, creating utility value for the material. On the other hand, the imposed shift to online instruction and labs may have dampened any motivation differences among students compared to if they had an option for course format.
There were also no significant group differences for self-regulation; while student motivation and self-regulation were moderately correlated (r = .60, p < .001), their unfamiliarity with online labs or uncertainty about what to expect in the course may have contributed to the lower levels of self-regulation overall. The lack of significant differences between various subgroups was not surprising as we posit many students entered the virtual lab environment with the same level of previous experience (none, as this course was the first of its kind) and concerns. Nonetheless, students’ thinking around motivation and self-regulation emerged as important topics in the subsequent qualitative interviews.
When attempting to understand the axis of the principal component graph in , two major themes seemed to underlie student interview responses: execution (horizontal) and scheduling (vertical). The words ‘go’, ‘start’, and ‘complete’ are seen in topic 1, topic 7, and topic 8, respectively, which span the horizontal axis of the intertopic map (see also ). Vertically, topic 3 represents shorter-term self-regulation during the labs as a theme in the interviews; this aligns with quantitative results which show the equivalence of self-regulation among students. Additionally, topic 5 describes long-term planning toward graduation and students’ decisions to take the course. This demonstrates another dimension of planning involved in the labs. A deeper exploration of these topics and verification with additional qualitative approaches and data collection might reinforce the importance of these factors for the student experience in online labs.
Examining important topics of the interviews reveal nuance related to student motivation and self-regulation in their lab experiences. As students described the experience in online labs, their perspectives were often benchmarked against traditional in-person labs. Yet, students noticed important attributes of both lab formats. We labelled the three main topics #1: Learning Compatibility, #2: Questions and Inquiry, and #3: Planning and Coordination and see ways that these correspond to the main aspects of motivation and self-regulation. The most frequent words in each topic are displayed in and we use quotes from the participants to further uncover their meaning.
4.1. Learning compatibility
The first topic related to learning in the lab experience and was a focus for many students during their interviews. Despite being compelled to complete the labs online, students spoke about the benefits and challenges of labs in all formats – in-person and online – and reflected that compatibility was among the most important factors in the lab experience. Student responses highlighted several dimensions for considering this compatibility: with personal preferences or needs and with learning content. Among these benefits of in-person labs were participating in the campus experience, feeling more hands-on, getting greater practice with the equipment, and being immersed in the lab environment. On the other hand, students commented that the online labs allowed them to have less time commuting, go at their own pace, prepare for future digital work environments, or do work from the comfort of home. In light of the pandemic, they commented that working from home also allowed personal health safety. These personal dimensions carried over into student perceptions of learning compatibility. For instance, one student said, ‘I like going in-person because I get to use hands. I get to see how you actually make the connections […] because theory and application in this class are two different things’ (Student D).
Moreover, students felt that certain learning topics might be more compatible with in-person or virtual lab experiences, ‘it depends on what the purpose of the lab is’. One student commented that the experience might change from day to day and be fine:
It depends, because like, my major is electrical engineering, so my major is not hands-on. In some components, like, we connect wires, connect transistors, resistors, and voltages […] I feel like being hands-on would be more helpful. But in some other components, like if we have like a virtual lab one day, it’s fine. (Student A)
4.2. Questions and inquiry
Topic 2 related to questions and inquiry. Students expressed concern regarding how to communicate with their professors or lab mates when they were confused, considering the ease at which this was possible in traditional, in-person formats. As seen by the interview excerpts below, students discussed their approaches for addressing questions or course inquiry, including dialogue between lab partners.
If a friend or someone didn’t understand this question, then I would email the professor, set up a Zoom session with the professor, ask him a question on what to do, how do I take someone forward and answer this question. (Student A)
Sometimes my question that I was asking or my problem would get skewed a little bit in translation to the professor, and then as I’d email back, I'd get half the answer I needed. (Student B)
While this might seem to portray a problem for clear communication in online labs, it is also the case that clear channels for communication are required in in-person settings. Students commented that when the materials were clear, progress was not an issue:
If I understand the material for this week, then I really don’t have a problem with the lab at all, online or in-person, it doesn’t matter. But if I don’t understand it, or if I’m struggling to understand it, then it makes it very difficult for me to complete the lab, especially online. (Student D)
4.3. Planning and coordination
Topic 3 related to planning and coordination, how students executed the lab successfully. This includes ensuring equipment worked so labs can be properly complete or allocating timing for task/activity completion. As seen by the interview excerpts below, students reported varying items for how the timing of the labs and found it was imperative to stay organised.
It’s really easy, it being online, to feel tempted that you can just do something else during the lab time and then come back and do it over the weekend or some other day. I would just advise people to keep to the schedule. Stay on top of it. (Student C)
Always plan ahead. I know in the past, where I’ll have forgotten to look at the schedule for this week, and I think, “Oh I’m online, this should be easy.” But it’s taken me two or three hours more than I’ve set aside for and I’ve had some really late nights trying to finish it. So long as you stay on top of things and as long as you make time for it, then you’ll be fine. (Student D)
4.4. Study Limitations
There are limitations the investigators are aware of that must be addressed in future research efforts, particularly as it relates to data collection. In future data collection, we will collect data before, during, and at the end of the semester to determine the change in motivation and self-regulation throughout the semester. Further, the number of interviews performed must also increase to allow for a larger data set and a more diverse set of interviewees.
For small datasets, LDA is less effective for producing meaningful topics (Tang et al. Citation2014). To overcome this problem, more textual data could improve the model performance. Besides the basic LDA model, future work should explore different LDA model structures. In particular, education practitioners could explore different strategies of finding the optimal number of topics, modelling sentence structure with alternative assumptions, and developing non-overlapping word distributions.
5. Conclusion and future work
Future work includes further testing with additional course cohorts, where data are collected pre- and post-course, to determine if their motivation and learning strategies changed. Further, a formal coding scheme will be developed to realise codes and themes within the interview responses. Similar to the findings of LDA, we anticipate that these codes and themes will relate to the main influences of student motivation and self-regulation and opportunities to shape student thinking about online lab experiences. Beyond student motivation and regulation, research on how students prepared for online courses, given their novelty, is necessary. For instance, an important research direction pertains to how students use prior lab experience to frame their preparation for online lab courses. Further, there are questions pertaining to how students approach an online lab and the effects of this approach. For instance, do they operate at their own pace and how does the experience challenge their conceptual understanding of the content?
The presented work discussed the introduction of online laboratories to EE and CSE courses in the context of a rapid switch from face-to-face to online experimentation from the students’ perspective. However, this perspective only focusses on one party in the classroom. Apart from considering the students’ perspective, it is also important to include the instructor and their perception of online experimentation practice in the account. In parallel to the student perspective-related research activities, our broader project includes the instructor perspective in a separate research thrust. This project section examines how instructors experienced a top–down mandated, time-constrained, and rapid transition to exclusively online-based laboratory modules in engineering courses along a continuum of resistance towards, or embracing of these educational technologies. In a manner similar to the student perspective reported here, the instructor perspective under study also represents a major gap in the literature, since many of the online laboratory studies in the literature are performed by the same instructor which implemented and sometimes developed the respective labs. This observation clearly raises the question of technology adoption and diffusion of innovation (Rogers Citation2010; Froyd et al. Citation2017). With a growing demand for online laboratory solutions in higher education, it will become normal that instructors, instead of developing their own labs, will need to use or adapt labs that are developed by other institutions. Though the general process of diffusion of educational innovations is extensively discussed in the literature, an in-depth consideration in the context of online laboratories is important, yet does not presently exist. A preliminary comparison between the instructor and the student perspective results reveals that both view compatibility to existing instruction practices and communication channels as the area of interest. Both topics seem to have a big impact on how the parties perceive online experimentation as a whole.
Complementary to this research, further investigation into the student user experience (UX) with the online lab experiments will inform researchers of specific roadblocks and challenges students encounter, as well as identify areas of strength in the implementation of online labs. Course redesigns incorporating the UX research information will improve the overall experience and facilitate students’ focus on learning content by removing technical barriers and improving usability.
As we better understand the student, instructors, and UX framing of online lab experiences, we will be able to close the feedback loop of the personal experiences when transitioning lab activities online by making strategic improvements to online lab experiences and the surrounding instructional materials.
Acknowledgement
This material is based upon work supported by the National Science Foundation under grant number 2032802.
Disclosure statement
No potential conflict of interest was reported by the author(s).
Additional information
Funding
Notes on contributors
Dominik May
Dr. Dominik May is an Assistant Professor in the Engineering Education Transformations Institute. He researches online and intercultural engineering education. His primary research focus lies on the development, introduction, practical use, and educational value of online laboratories (remote, virtual, and cross-reality) and online experimentation in engineering instruction. In his work, he focuses on developing broader educational strategies for the design and use of online engineering equipment, putting these into practice and provide the evidence base for further development efforts. Moreover, Dr. May is developing instructional concepts to bring students into international study contexts so that they can experience intercultural collaboration and develop respective competences.
Beshoy Morkos
Beshoy Morkos is an associate professor in the College of Engineering at the University of Georgia. His research group currently explores the areas of system design, manufacturing, and their respective education. His system design research focuses on developing computational representation and reasoning support for managing complex system design through the use of Model Based approaches. The goal of Dr. Morkos' manufacturing research is to fundamentally reframe our understanding and utilization of product and process representations and computational reasoning capabilities to support the development of models which help engineers and project planners intelligently make informed decisions. On the engineering education front, Dr. Morkos' research explores means to improve persistence and diversity in engineering education by leveraging students' design experiences.
Andrew Jackson
Dr. Andrew Jackson is an Assistant Professor of Workforce Education, with expertise in technology, engineering, and design education. His research involves cognitive and non-cognitive aspects of learning. In particular, he is interested in design-based learning experiences, how beginning students navigate the design process, and how students' motivation and confidence plays a role in learning. Dr. Jackson's past work has bridged cutting-edge soft robotics research to develop and evaluate novel design experiences in K-12 education, followed students' self-regulation and trajectories while designing, and produced new instruments for assessing design decision-making.
Nathaniel J. Hunsu
Nathaniel J. Hunsu is an assistant professor of Engineering Education. He is affiliated with the Engineering Education Transformational Institute and the School of Electrical and Computer Engineering in the University of Georgia's College of Engineering. His interest is at the nexus of the research of epistemologies, learning mechanics and assessment of learning in engineering education. His research focuses on learning for conceptual understanding, and the roles of learning strategies, epistemic cognition and student engagements in fostering conceptual understanding. His research also focuses on understanding how students interact with learning tasks and their learning environment. His expertise also includes systematic reviews and meta-analysis, quantitative research designs, measurement inventories development and validation.
Amy Ingalls
Amy Ingalls is a passionate educator who believes in accessibility and equal access to education for all. A part of the original UGA Online Learning team, Amy has extensive experience in developing, designing, and supporting impactful online courses at the undergraduate and graduate levels. Outside of her work at UGA, Amy has experience as a library media specialist and technology instructor in K12 classrooms. As an instructor, a course developer, and a human, Amy believes that online-delivered courses remove barriers to education and the pursuit of education is a part of our mission at UGA.
Fred Beyette
Fred Beyette is the founding chair of the School of Electrical and Computer Engineering in the University of Georgia College of Engineering. Over the past decade, Beyette's research has focused on developing point-of-care devices for medical and health monitoring applications - including devices that guide the diagnosis and treatment of acute neurologic emergencies such and stroke and traumatic brain injury. His work has resulted in 12 patent applications.
References
- Al Weshah, A., R. Alamad, and D. May. 2021. “Work-in-Progress: Using Augmented Reality Mobile App to Improve Student’s Skills in Using Breadboard in an Introduction to Electrical Engineering Course.” In Cross Reality and Data Science in Engineering, edited by M. Auer and D. May, 313–319. Switzerland, Cham: Springer.
- Bernard, R. M., A. Brauer, P. C. Abrami, and M. Surkes. 2004. “The Development of a Questionnaire for Predicting Online Learning Achievement.” Distance Education. 25 (1): 31–47.
- Blei, D. M., A. Y. Ng, and M. I. Jordan. 2003. “Latent Dirichlet Allocation.” Journal of Machine Learning Research 3: 993–1022.
- Brinson, J. R. 2015. “Learning Outcome Achievement in Non-traditional (Virtual and Remote) Versus Traditional (Hands-on) Laboratories: A Review of the Empirical Research.” Computers & Education 87: 218–237.
- Brinson, J. R. 2017. “A Further Characterization of Empirical Research Related to Learning Outcome Achievement in Remote and Virtual Science Labs.” Journal of Science Education and Technology 26 (5): 546–560.
- Castillo-Montoya, M. 2016. “Preparing for Interview Research : The Interview Protocol Refinement Framework.” Qualitative Report (online) 21 (5): 811–831.
- Chen, C., J. Mullis, and B. Morkos. 2021. “A Topic Modeling Approach to Study Design Requirements.” In ASME 2021 International Design Engineering Technical Conferences and Computers and Information in Engineering Conference, August 17.
- Chuang, J., C. D. Manning, and J. Heer. 2012. “Termite: Visualization Techniques for Assessing Textual Topic Models.” In Advanced Visual Interfaces.
- Chuang, J., D. Ramage, C. D. Manning, and J. Heer. 2012. “Interpretation and Trust: Designing Model-Driven Visualizations for Text Analysis.” In ACM Human Factors in Computing Systems (CHI).
- Clark, M., D. Shah, E. Kames, and B. Morkos. 2019. “Developing an Interview Protocol for an Engineering Capstone Design Course.” In Proceedings of the ASME Design Engineering Technical Conference, Vol. 3.
- Corter, J. E., J. V. Nickerson, S. K. Esche, C. Chassapis, S. Im, and J. Ma. 2007. “Constructing Reality: A Study of Remote, Hands-on, and Simulated Laboratories.” ACM Transactions on Computer-Human Interaction 14 (2): 7.
- Devine, R., and D. May. 2021. “Work in Progress: Pilot Study for the Effect of a Simulated Laboratories on the Motivation of Biological Engineering Students.” In edited by M. Auer and D. May, 429–436. Switzerland, Cham: Springer.
- Estriegana, R., J.-A. Medina-Merodio, and R. Barchino. 2019. “Student Acceptance of Virtual Laboratory and Practical Work: An Extension of the Technology Acceptance Model.” Computers & Education 135: 1–14.
- Faulconer, E. K., and A. B. Gruss. 2018. “A Review to Weigh the Pros and Cons of Online, Remote, and Distance Science Laboratory Experiences.” The International Review of Research in Open and Distributed Learning 19 (2): 156–168.
- Froyd, J. E., C. Henderson, R. S. Cole, D. Friedrichsen, R. Khatri, and C. Stanford. 2017. “From Dissemination to Propagation: A New Paradigm for Education Developers.” Change: The Magazine of Higher Learning 49 (4): 35–42.
- Garcia-Zubia, J., J. Cuadros, S. Romero, U. Hernandez-Jayo, P. Orduna, M. Guenaga, L. Gonzalez-Sabate, I. Gustavsson. 2017. “Empirical Analysis of the Use of the VISIR Remote Lab in Teaching Analog Electronics.” IEEE Transactions on Education 60 (2): 149–156.
- Gustavsson, I., K. Nilsson, J. Zackrisson, J. Garcia-Zubia, U. Hernandez, A. Nafalski, Z. Nedic, et al. 2009. “On Objectives of Instructional Laboratories, Individual Assessment, and Use of Collaborative Remote Laboratories.” IEEE Transactions on Learning Technologies 2 (4): 263–274.
- Heradio, R., L. de la Torre, D. Galan, F. J. Cabrerizo, E. Herrera-Viedma, and S. Dormido. 2016. “Virtual and Remote Labs in Education: A Bibliometric Analysis.” Computers & Education 98: 14–38.
- Hernández-de-Menéndez, M., A. Vallejo Guevara, and R. Morales-Menendez. 2019. “Virtual Reality Laboratories: A Review of Experiences.” International Journal on Interactive Design and Manufacturing 13 (3): 947–966.
- Kames, E., D. Shah, M. Clark, and B. Morkos. 2019. “A Mixed Methods Analysis of Motivation Factors in Senior Capstone Design Courses.” American Society of Engineering Education Annual Conference & Exposition: 156–168.
- Kollöffel, B., and T. de Jong. 2013. “Conceptual Understanding of Electrical Circuits in Secondary Vocational Engineering Education: Combining Traditional Instruction with Inquiry Learning in a Virtual Lab.” Journal of Engineering Education 102 (3): 375–393.
- Li, R., J. R. Morelock, and D. May. 2020. “A Comparative Study of an Online Lab Using Labsland and Zoom During COVID-19.” Advances in Engineering Education 8 (4): 1–10.
- Ma, J., and J. Nickerson. 2006. “Hands-on, Simulated, and Remote Laboratories: A Comparative Literature Review.” ACM Computing Surveys 38: 7.
- May, D. 2020. “Cross Reality Spaces in Engineering Education – Online Laboratories for Supporting International Student Collaboration in Merging Realities.” International Journal of Online Engineering 16 (3): 4–26.
- May, D., M. Trudgen, and A. V. Spain. 2019. “Introducing Remote Laboratory Equipment to Circuits – Concepts, Possibilities, and First Experiences.” ASEE Annual Conference & Exposition.
- Pokoo-Aikins, G. A., N. Hunsu, and D. May. 2019. “Development of a Remote Laboratory Diffusion Experiment Module for an Enhanced Laboratory Experience.” In 2019 IEEE Frontiers in Education Conference (FIE), 1–5.
- Potkonjak, V., M. Gardner, V. Callaghan, P. Mattila, C. Gütl, V. M. Petrović, and K. Jovanović. 2016. “Virtual Laboratories for Education in Science, Technology, and Engineering: A Review.” Computers & Education 95: 309–327.
- Rogers, E. M. 2010. Diffusion of Innovations. 4th ed. New York, NY: Simon and Schuster.
- Rubin, H. J., and I. S. Rubin. 2005. Qualitative Interviewing – The Art of Hearing Data, 113–117. 2nd ed. Thousand Oaks, CA: Sage Publications.
- Shah, D., M. Clark, E. Kames, and B. Morkos. 2019. “Development of a Coding Scheme for Qualitative Analysis of Student Motivation in Senior Capstone Design.” In ASME International Design Engineering Technical Conference, Anaheim.
- Sievert, C., and K. Shirley. 2014. “LDAvis: A Method for Visualizing and Interpreting Topics.” In Proceedings of the Workshop on Interactive Language Learning, Visualization, and Interfaces, 63–70.
- Tang, J., Z. Meng, X. Nguyen, Q. Mei, and M. Zhang. 2014. “Understanding the Limiting Factors of Topic Modeling Via Posterior Contraction Analysis.” In Proceedings of the 31st International Conference on International Conference on Machine Learning, Vol. 32, I-190–I-198.
- Yeong, M. L., R. Ismail, M. H. Ismail, and M. I. Hamzah. 2018. “Interview Protocol Refinement: Fine-Tuning Qualitative Research Interview Questions for Multi-Racial Populations in Malaysia.” Qualitative Report (Online) 23 (11): 2700–2713.