ABSTRACT
Local government authorities (LGAs) play a key role in facilitating mitigation of road traffic CO2 emissions and must engage in emissions modelling to quantify the impact of transport interventions. Existing Emissions Model (EM) methodologies range from aggregate to disaggregate approaches, with more detail normally entailing more resources. However, it is not clear which approaches LGAs actually utilise. This article reports results of a survey designed to discover the level of detail considered practical by British LGAs (n = 34). Results show that resource scarcity is important, with particular importance attached to EM reusability and convenient input data sources. Most LGA EMs use traffic variable inputs (predominantly traffic flow and traffic average speed), with this approach being the best-fit for LGA resources. Link-by-link sources of data rated highly for convenience are Road Traffic Models and Urban traffic control systems.
1. Introduction
Ultimately, governments are responsible for providing road infrastructure and for achieving agreed greenhouse gas (GHG) emissions reduction targets. Typically, motorways and major trunk roads (the strategic road network, SRN) are administered by central government agencies (e.g. Highways England and Transport Scotland), whilst responsibility for all other adoptedFootnote1 roads is devolved to local government authorities (LGAs). For comparison, the national total of 417 billion vehicle-kilometres travelled (VKT) in England in 2012 was split between 33% (136.3 billion VKT) carried by SRN roads and 67% (280.7 billion VKT) carried by non-SRN roads (DfT Citation2013). Those BritishFootnote2 LGAs responsible for non-SRN roads are known as local highways authorities (LHAs).Footnote3 Under the complex system of local government in Britain, not all LGAs are LHAs. Only first tier LGAs (County Councils) and single tier LGAs (Unitary Authorities, London Borough Councils and Metropolitan District Councils) are LHAs. Second tier LGAs (District Councils, Borough Councils and those City Councils that are not Unitary Authorities) are not responsible for the roads in their region, with the appropriate first tier LGA being responsible instead. The result of this system is that approximately half of British LGAs (206 out of a total of 407) are also LHAs (DECC Citation2014b; DCLG Citation2015).
Road traffic has damaging environmental impacts. One cause of such impacts is vehicle tailpipe emissions, including both GHGs and pollutants detrimental to air quality (AQ). In their capacity as LHAs, much of the responsibility for facilitating mitigation of these emissions is borne by LGAs. When considering transport interventions, it is necessary to quantify an intervention’s effect on road traffic emissions. However, it is impractical to measure real-world emissions at road network level due to the large number of vehicles and traffic conditions involved (Smit Citation2006; Smit, Ntziachristos, and Boulter Citation2010), and impossible to measure real-world emissions when considering hypothetical scenarios. Emissions Models (EMs) can offer a practical (and less expensive) alternative (Grote et al. Citation2016). LGAs have limited resources with which to engage in the emissions modelling process. Constraints on public funds are increasing due to the global financial crisis of 2008 and subsequent austerity measures, with many governments imposing dramatic budget cuts (Lowndes and McCaughie Citation2013), meaning funds for emissions modelling are scarce.
Existing EMs are based on various methodologies, ranging from less detailed, aggregate approaches (e.g. traffic considered as a whole at a constant average speed) to highly detailed, disaggregate approaches (e.g. individual vehicle operating modes sampled at 1 Hz). An absence of research specifically investigating the practicalities of LGAs engaging in emissions modelling has been noted (Grote et al. Citation2016), and it is not clear which of the available methodologies LGAs can afford to utilise and which they ignore. This research contributes to addressing this gap, with a focus on investigating the emissions modelling process that is best-fit for all (or the substantial majority of) LGAs. The methodology used was a survey of LGA emissions modelling experts. Strategies for mitigating climate change must tackle the problem of road traffic GHG emissions, with LGAs in all countries having an important role, and understanding their requirements is essential if practical options for estimating emissions are to be developed and gain traction.
The study’s scope was limited to carbon dioxide (CO2) because this is the largest constituent of transport’s GHG emissions, constituting 99% of CO2eFootnote4 in the UK (DECC Citation2014a). Globally, transport’s contribution to total CO2 emitted from fuel combustion is 23%, of which road traffic is responsible for approximately three-quarters (IEA Citation2014). Additionally, it is acknowledged that other actors may influence the framework within which LGAs make transport decisions, for example, regional partnerships (between LGAs and other interested parties) and the use by LGAs of external consultants. However, ultimately LGAs are the highways authorities responsible for the non-SRN roads within their areas, and are also responsible for assessing any requirement to expend resources on engaging external consultants. The study’s scope was therefore limited to LGAs’ attitudes to CO2 emissions modelling.
It was not possible within the constraints of this research to provide a full analysis of the transferability of the results from the case study survey of Britain. However, previous research into the governance of transport and climate change by Marsden and Rye (Citation2010) concluded that, although decision-making structures may be different in other countries, issues concerning delivery of strategies to tackle climate change were not solely dependent on the formal institutional structures in a country and so ‘the cases of England and Scotland will have some parallels to other locations’.Footnote5
The rest of this article is divided into sections. In Section 2 the method adopted for the research is described. Results are presented in Section 3, followed by a discussion of those results in Section 4. Section 5 is the final section and details the conclusions of the research. Additionally, abbreviations used in this article are included in an appendix.
2. Method
LGA personnel constitute a combination of councillors who are elected to decide policy on behalf of the electorate, and officers who have the expertise to translate policy into practice (Meek, Ison, and Enoch Citation2010). Prerequisites for meaningful survey responses were that participants had expertise, experience and detailed familiarity with road traffic data and emissions modelling. As elected councillors were unlikely to meet such requirements, a decision was made to survey only officers. After consultation with the Local Government Association,Footnote6 a UK public sector database specialist (Oscar Research Ltd) was employed to provide at least one named, senior highways officer contact for each of the 206 LHAs in Britain, giving a total of 376 potential participants. An online questionnaire survey compiled in the iSurvey format (a University of Southampton research tool for distributing online questionnaires) was selected as the most effective method to reach this number of potential participants in the time available, with a cross-sectional survey design being appropriate for gathering data on current LGA attitudes to CO2 emissions modelling. The data gathered by the questionnaire were intended to be statistically analysed and reported. Therefore, closed rather than open questions were used (i.e. including response choices, which produced either categorical or ordinal variables). However, a free-text ‘Further Details’ box was offered at the end of some questions (Oppenheim Citation1992; Fink Citation2003a, Citation2003b). It is accepted that this survey design was unlikely to capture the full nuanced picture of LGAs’ attitudes. However, the aim of the research was to provide an indication of the general situation for all (or the majority of) LGAs, rather than the nuances which are likely to vary from LGA to LGA. The automated iSurvey reminder email facility, followed by three further reminder emails sent manually, were used to maximise rates of response. Finally, those that had shown any interest (by opening the URL link to the survey) but not completed the questionnaire were contacted by telephone in an attempt to encourage participation.
The relative importance of factors affecting allocation of resources to emissions modelling was established by asking participants to indicate the extent of their agreement with nine different statements, each asserting that a particular factor was important. An ordinal five-point Likert scale was used for responses (Strongly disagree, Disagree, Neither agree nor disagree, Agree, Strongly agree). Scores were assigned from Strongly disagree = 0, through to Strongly agree = 4.
EMs require road traffic data as inputs, and resources must be expended on collection of these data. In general, using convenient sources of such data minimises expenditure. Brief details of the numerous different sources of road traffic data about which participants were questioned are provided in the following paragraphs. Traffic counts (manual or automatic) record numbers of vehicles passing a location, and can include vehicle category data (i.e. car, light goods vehicle, heavy goods vehicle, bus, etc.). Automatic number plate recognition (ANPR) cameras read vehicle licence plates allowing vehicle category to be established, and journey times between cameras to be calculated. Queue length surveys record the details of traffic queues (e.g. length, number of vehicles and delay times). Roadside interview (RSI) surveys involve stopping a sample of vehicles passing a survey site and interviewing the occupants. Vehicle tracking data can be collected from in-vehicle devices using Bluetooth, GPS, mobile telephony or Wi-Fi technologies (e.g. Strat-e-gis CongestionFootnote7 provides historic congestion data based on GPS technology). In the UK, there was a requirement (abolished in 2010) for National indicators (NIs) to be reported annually by LGAs to central government. However, LGAs are still encouraged by central government to collect NIs beneficial for monitoring and evaluation purposes (SCC Citation2011a), and some are measures of road network performance (e.g. average journey time per mile along key routes), with data from Strat-e-gis Congestion often used by LGAs to monitor performance against NIs.
Road traffic models (RTMs) are frequently used as sources of road traffic data, and represent how travel demand is satisfied by the road network (Grote et al. Citation2016). RTMs are typically classified by scale. Macro-RTMs model traffic as an aggregated flow described by relationships between traffic density (vehicles/km), traffic average speed (km/h) and traffic flow (vehicles/h) (Lighthill and Whitham Citation1955; Kotsialos et al. Citation2002), and produce equilibrium solutions with demand assumed to be constant over an entire modelled period. Micro-RTMs simulate the movements of individual vehicles through combining detailed network characteristics with detailed driver behaviour sub-models (Papacostas and Prevedouros Citation2005; Ortúzar and Willumsen Citation2011; Ramos, Vasconcelos Ferreira, and Barceló Citation2011), and can produce driving patterns for each vehicle as outputs. Meso-RTMs are a third classification often distinguished between micro-RTMs and macro-RTMs. Vehicle movements and interactions are modelled, but in less detail than in micro-RTMs. For example, SATURNFootnote8 uses a platoon-dispersion moduleFootnote9 to simulate the movement of vehicles between signal-controlled intersections accounting for interaction with vehicles entering/exiting the road and different drivers’ preferred speeds (Papacostas and Prevedouros Citation2005; Ortúzar and Willumsen Citation2011).
Urban traffic control (UTC) systems coordinate traffic signals to achieve good vehicle progression through urban road networks. Signal control data are generated by inductive loop detectors (ILDs) installed beneath the road surface, which send vehicle presence information (ILD occupied or unoccupied) every 250 ms. Also, enhanced ILDs (as distinct from standard ILDs) can provide vehicle category data based on the inductance change due to vehicle passage measured by the detector being distinct for different categories (Grote et al. Citation2016).
Three questions in the study were concerned with road traffic data sources. The first established the perceived convenience of data sources by asking participants to select one categorical response that best described their opinion of the availability of data from each source. In the second, participants were asked to indicate all time periods when (if ever) data from each source had been routinely collected by (or on behalf) of their organisation. An additional time period response category was added to this question of ‘Planned for future collection’ because road traffic data to which LGAs have access ‘going forward’ are the most convenient for use in future emissions modelling. The third question examined in more detail LGAs’ use of RTMs. This was for three reasons: (1) where LGAs have invested in RTMs, the outputs are likely to be a convenient source of EM input data; (2) the type of RTM used (macro/meso/micro) affects the detail of data available as EM inputs and (3) some RTMs incorporate their own built-in EM. Participants were asked whether or not they had used different RTM software applications. If an RTM had been used, participants were asked to select from categorical responses to indicate when it was last used by (or on behalf) of their organisation.
LGA use of EMs was examined by asking participants whether or not they had used different EM software applications, with EM types classified according to the system set out by Smit, Ntziachristos, and Boulter (Citation2010) and briefly detailed here in order of increasing complexity. Aggregate EMs typically use a single, fixed emission factor (EF) for a given vehicle category travelling on a given road type, with the usual distinction being urban, rural or motorway. Average Speed EMs calculate EFs for each vehicle category as a function of traffic average speed. In Traffic Situation EMs a range of traffic situations are specified, with each traffic situation being correlated with EFs for a range of different vehicle categories. Different traffic situations are characterised by road type (e.g. motorway with 120 km/h limit) and a qualitative description of traffic conditions (e.g. free flowing). Traffic Variable EMs calculate EFs for each vehicle category as a function of variables describing the traffic as an aggregate whole (e.g. traffic average speed, traffic density, average delay rate, etc.). Cycle Variable EMs calculate EFs for individual vehicles as a function of variables derived from a vehicle’s driving pattern (e.g. number of stops/km, vehicle average speed, maximum acceleration, etc.). Modal EMs calculate EFs for individual vehicles as a function of vehicle or engine operating modes. The latest generation of Modal EMs predict EFs for operating modes at temporal resolutions of 1 Hz, and are typically termed Instantaneous EMs. Where an EM had been used, participants were asked to indicate when it had last been used by (or on behalf) of their organisation.
Two questions were developed to investigate LGAs’ willingness to commit resources to the emissions modelling process. To permit the extent of resource commitment to be quantifiably evaluated, the questions were designed so that results could be expressed as an implied monetary value placed on CO2 emissions reductions (£/tonne), which would then allow comparison with official UK central government CO2 valuations. Two questions were used, each with a different transport intervention scenario, to assess whether (or not) CO2 valuations remained consistent.
In the scenarios for both questions it was assumed that there was a true amount of CO2 emissions reduction resulting from an intervention. Participants were asked to compare the use of two different EMs (EM1 and EM2) for predicting this reduction. The less expensive, less complex and less accurate model (EM1) under-estimates the reduction. The more expensive, more complex and more accurate model (EM2) gets closer to the true reduction, and ultimately (in the series of response options offered to participants) replicates the true reduction exactly without ever over-shooting. In essence EM2 is assumed to have (near) optimal complexity. The difference between the predictions leads to EM2 predicting a greater emissions reduction than EM1. For both scenarios, this difference ranged from 100 tonnes up to 1000 tonnes (), with the latter occurring when the EM2 prediction exactly matched the true reduction.
Table 1. Response categories for both survey questions investigating LGAs’ willingness to commit resources to emissions modelling.
Of course, both EM1 and EM2 could over-estimate the true emissions reduction. However, if this was the case, EM1 would appear to predict a greater reduction than EM2. A less expensive EM predicting a greater emissions reduction would be appealing to LGAs (regardless of the true emission reduction which would be unknowable in the real-world), and would have undermined the purpose of the two questions. This is why in this hypothetical experiment EM2 needed to be posited as an optimal model and EM1 as a sub-optimal model in a particular direction (i.e. under-estimation). Additionally, for simplicity, any issues about precision or statistical variability in model predictions were deliberately omitted.
Information presented to the participants in the two scenarios (pre- and post-intervention annual road traffic CO2 emissions, intervention cost, EM cost and population size) was based on real-world data from an abstraction of a Southampton (city in southern England) case study (SCC Citation2011b) and an email to the authors from Theo GenisFootnote10 (personal communication, October 14, 2014). This is why the measurements used in the question are absolute (£s and tonnes of CO2) rather than percentages.
Participants were asked, for a given cost increase, what improvement in accuracy from EM2 would justify its extra cost. For both questions, this sub-question was repeated five times, with the cost of EM2 increasing in £10,000 steps from £60,000 to £100,000. For example, participants were asked ‘If EM1 costs £50,000 and EM2 costs £60,000, moving from left to right, select the button that best indicates the point at which the improvement in accuracy offered by EM2 becomes large enough to justify the extra cost of using EM2’ and offered response categories as shown in . Considering only the extra cost of using EM2, and the associated extra predicted CO2 saving, the choices available to participants in both questions placed valuations on CO2 as shown in .
Table 2. CO2 valuations associated with response categories for both survey questions investigating LGAs’ willingness to commit resources to emissions modelling.
3. Results
Thirty-four surveys were returned with at least one question completed, and rates of response to individual questions ranged from a maximum of 9% (n = 34) to a minimum of 6% (n = 23). It is acknowledged that these sample sizes are relatively small, but other surveys of a similar type exist with sample sizes of a comparable order of magnitude (e.g. Xenias and Whitmarsh (Citation2013) used a sample of 53 experts). Best efforts were made to maximise the number of participants, and low response rates may be symptomatic of pressure on LGAs’ resources leading to a lack of available time/manpower to complete the survey. Whilst low response rates are not necessarily indicative of non-response bias (Lineback and Thompson Citation2010), they do increase the need for a non-response bias analysis to provide confidence in the data quality. Of the 206 LHAs, responses were received from at least 27 (13%) different authorities (7 of the 34 participants elected to remain anonymous). The group of known respondents was compared to the group of non-respondents (which also included the seven anonymous respondents) to identify any statistically significant differences. Variables for comparison were resident population (2011 census data), geographical area (hectares), an indicator of urbanisation (population density in residents/ha), and an indicator of spending (LGA netFootnote11 revenue expenditure per capita in £s for 2013/2014). Non-parametric Mann–Whitney tests (Mann and Whitney Citation1947; Field Citation2009) showed that levels of the four variables in the sample of respondents did not differ significantly from those found in the non-respondents. The distribution of regional response rates was as follows (i.e. the number of LHAs that responded as a percentage of LHAs in each region): Scotland 16%; Wales 14%; SE England 11%; SW England 25%; London 3%; East of England 9%; East Midlands 22%; West Midlands 29%; Yorkshire and the Humber 13%; NE England 17% and NW England 4%.
Results from statistical analysis of response scores from the question asking participants their opinion on the importance of factors affecting allocation of resources are shown in , with a higher mean importance score (range 0–4) indicating a factor is perceived as more important. A score of 2 corresponds to the neutral statement: Neither agree nor disagree. Mean importance scores are all greater than 2, indicating that, on average, every factor is regarded as important to some extent. A non-parametric Friedman test (Citation1937) and post-hoc pairwise Dunn tests (Citation1964) were conducted to determine if the observed differences in responses to the different factors were statistically significant. The four pairwise comparisons having statistically significant differences were: ‘Ability to re-use model in future projects’ and ‘Avoiding staff training’; ‘Ability to re-use model in future projects’ and ‘Quick completion’; ‘Ability to re-use model in future projects’ and ‘Avoiding employing external consultants’; and ‘Easy availability of input data’ and ‘Avoiding employing external consultants’. The Friedman test results indicate that, in general, statistically significant differences only exist between responses to factors at the extremes of the rankings (i.e. between the top two and bottom three factors). Friedman test mean rankings are shown in , with a higher ranking (range 1–9) indicating a more important factor.
Table 3. Mean importance scores and Friedman test mean rankings for factors affecting emissions modelling resource allocation.
Participant perceptions of the availability of road traffic data sources are shown in . Time periods when data were collected from these sources are shown in , and are displayed in descending order of the percentage of participants selecting ‘Planned for future collection’ in response to a particular data source. LGAs’ use of different RTM software applications is shown in . LGAs’ use of different EM software applications is shown in . It should be noted that WebTAG is not a software application. Instead, it is the UK central government’s Department for Transport (DfT) guidance on conducting transport studies, and includes methods for calculating road traffic emissions. This is also the case for the design manual for roads and bridges (DMRB), which is the DfT’s guidance on the design, assessment and operation of trunk roads and motorways (although a software application based on the DMRB is available). shows participant responses when aggregated according to EM type.
Figure 1. Participant perception of the availability of road traffic data sources.
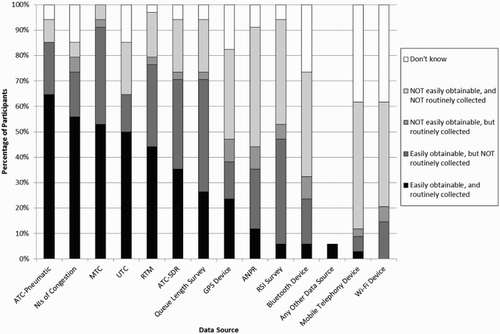
Figure 2. Time periods when data were (or are planned to be) collected by (or on behalf of) LGAs from road traffic data sources.
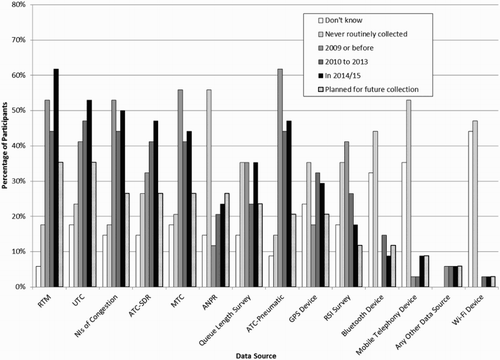
Figure 3. Time periods when RTM software applications were last used by (or on behalf of) LGAs.
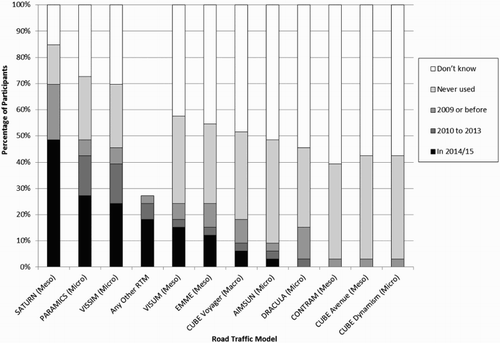
Figure 4. Time periods when EM software applications were last used by (or on behalf of) LGAs.
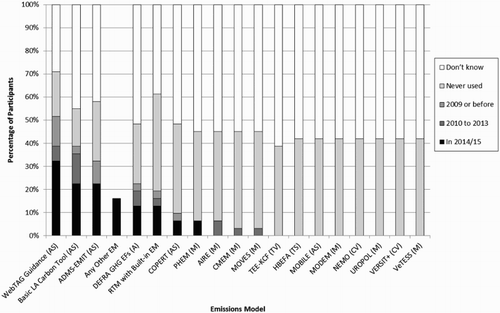
Figure 5. Types of EM software applications used by (or on behalf of) LGAs.
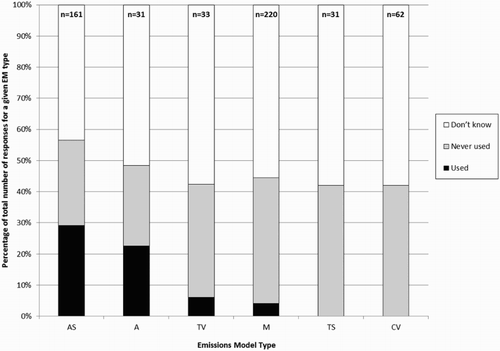
For the two questions quantifying LGAs’ willingness to commit resources to the emissions modelling process, the mean valuation of CO2 emissions from responses to the first question was £11.51/tonne (Standard Deviation, SD £18.56) and from responses to the second question was £14.08/tonne (SD £27.50), with an overall mean valuation of £12.77/tonne (SD £23.35). For comparison, for 2015 the UK central government values CO2 emissions from the untraded sector (i.e. emissions not included in the EU Emissions Trading System, which is the case for petrol and diesel used in road vehicles) at £62.78/tonne (SD £31.39), based on a valuation of £57.40/tonne (SD £28.70) in 2010£s corrected for inflation (DfT Citation2014). Therefore, LGAs’ mean valuations determined here are less than a quarter of central government values but the standard deviations are of a similar order of magnitude. This indicates the limitations of this comparison in that it is not an ideal like-for-like comparison (i.e. the value of EM accuracy is not the same as the value of emissions). However, the comparison serves the purpose of providing a context against which a general sense can be gained of whether the LGA values are plausible, and the fact that they are less than central government values of actual emissions was consistent with expected results.
4. Discussion
An aim of the research was to investigate the emissions modelling process that is the best-fit for (ideally) all LGAs, rather than considering the situation where different EMs are developed to suit different LGAs characterised by urbanisation, population size, location, etc. Hence, in the analysis of survey results LGAs were not disaggregated according to these characteristics. Benefits of a single approach to CO2 emissions modelling are that it allows comparability of results from transport intervention assessments across different LGAs, and that research and development can be focused on one particular methodology; although it is acknowledged that these benefits would have to be weighed against the benefits of using EMs tailored to specific circumstances. A potential procedure for establishing a consensus on LGA requirements would be a regular survey of LGAs across the globe; although it is acknowledged that the interval between such surveys is likely to be measured in decades due to the size of the undertaking, and that there are considerable difficulties (impossibilities?) inherent in securing such international agreements on mitigation of CO2 emissions.
Generally, LGAs indicated concern for all the factors affecting allocation of resources to road traffic emissions modelling (mean importance scores all >2), highlighting an overall opinion that resource scarcity is an important issue. Whilst statistically significant differences only existed between factors at the extremes of the Friedman test mean rankings, inspection of suggests that survey participants considered model reusability to be the most important factor, that is, a preference for EMs that have the flexibility to be used in assessment of future, as yet undetermined, interventions. From the LGA viewpoint, EMs tailored to the one-off assessment of a particular intervention are best avoided. Reusable EMs bring many additional benefits which may explain the importance attached to this factor, such as: staff familiarity; goodness of fit with existing skills; shorter timescales required to use familiar software applications; avoidance of regular staff retraining; reliability of operation; trust in validity of results; comparability of results across different intervention assessments and goodness of fit with road traffic data routinely collected.
Easily available input data also was considered an important factor, being ranked second by participants. Of all the road traffic data sources, the options that appear to be most convenient for LGAs are RTMs and UTC systems. This is for three reasons: (1) they are ranked highly by participants as easily obtainable and routinely collected; (2) they are ranked highest and second-highest, respectively, as planned for future collection which is an important indicator of the data LGAs expect to be available for future emissions modelling and (3) they are available on a link-by-link basis enabling emissions calculations for all (or mostly all) links in a network to be performed. Other data sources considered easily available by LGAs typically do not provide link-by-link data. For example, availability of traffic counts (manual or automatic), surveys and ANPR data are restricted to only a few locations (spatially and also sometimes temporally) within a network, and NIs of congestion are normally only available for certain key routes. Where sufficient penetration is achieved (i.e. number of vehicles from which data can be gathered compared to total number of vehicles), vehicle tracking data from GPS, Bluetooth, mobile telephony and Wi-Fi devices are available on a link-by-link basis. However, these technologies are not considered easily available by LGAs and have not been widely collected, indicating a lack of familiarity and experience in their use. By inspection of a general trend appears of a move away from manual, labour-intensive data collection towards automatic, less labour-intensive sources. Manual traffic counts (MTCs) and RSI surveys are both decreasing (although queue length surveys are fairly constant), whilst RTMs, UTC, automatic traffic count by speed detection radar (ATC-SDR) and ANPR are all increasing (although ATC-pneumatic are decreasing). Explanations for this trend could be the increasing pressure on LGA resource budgets and/or an expectation that the rise in telematics and ‘big’ data will satisfy LGA traffic data requirements, although the technologies underpinning telematics have low ranks as data planned for future collection.
Due to convenience, LGAs are likely to use RTMs and UTC systems as sources for EM input data. Data available from UTC systems are traffic variables, that is, describing traffic as an aggregate whole rather than describing individual vehicles. However, accuracy of the traffic data provided by ILDs is an issue, particularly for single-loop ILDs which are often installed in urban areas (Han et al. Citation2010). For example, where traffic average speed is a required EM input this is typically space-mean-speed,Footnote12 whereas ILDs can only provide estimates of time-mean-speed (which in themselves may be of questionable accuracy). Nonetheless, results of the survey indicate use of this convenient data source (with its associated inherent inaccuracies) to provide EM inputs is a subject worthy of further research. Data output from RTMs depend on RTM type. The RTM most widely used by LGAs is SATURN, which is a meso-RTM that outputs traffic variables rather than individual vehicle driving patterns. PARAMICS and VISSIM are the second and third most used RTMs, respectively, and are both micro-RTMs that can output individual vehicle driving patterns. However, collecting and processing driving patterns for every vehicle in a network is a resource-intensive task. Additionally, micro-RTMs are typically calibrated and validated for aggregate traffic measures (e.g. traffic average speed, traffic flow and average delay) rather than for driving patterns of individual vehicles (Hirschmann et al. Citation2010; Song, Yu, and Zhang Citation2012; Toffolo et al. Citation2013; Song, Yu, and Xu Citation2013). Therefore, driving pattern outputs from micro-RTMs are rarely properly validated, and do not necessarily accurately represent the real-world (Song, Yu, and Zhang Citation2012; Song, Yu, and Xu Citation2013), whereas link-level traffic variable outputs are more likely to be accurate.
A preference for calculating emissions from traffic variables is demonstrated by the common use of Average Speed EMs by LGAs, with the three most used EMs all being of the Average Speed type. Most EMs are currently based on average speed (Boulter et al. Citation2012), and a suggested reason for this prevalence is that readily available data are often restricted to estimates of traffic average speed for each link (Smit, Poelman, and Schrijver Citation2008). However, average speed is not the only traffic variable readily available from sources such as RTMs and UTC systems, and inclusion of the explanatory power of other traffic variables may present an opportunity to improve the accuracy of emissions calculations. To take advantage of this opportunity, the feasibility of developing Traffic Situation EMs or Traffic Variable EMs (both currently not widely used by LGAs) for use by LGAs is worthy of further investigation. Cycle Variable EMs and Modal EMs are also both currently not widely used by LGAs. Likely reasons for this are that these types of EMs are resource-intensive to use, and that they require accurate driving patterns as inputs which cannot be obtained from the data sources regarded as easily available to LGAs, a situation unlikely to change in the near future based on LGA future data collection plans.
A surprising result was that no participant mentioned using the emissions factors toolkit (EFT) EM, which is an Average Speed EM developed by the UK central government’s Department for Environment Food & Rural Affairs (DEFRA) specifically to assist LGAs in carrying out their statutory duty to assess local AQ. Given that approximately 60% of UK LGAs have designated areas where AQ objectives are not being met (Citation2014a) and that road traffic emissions are the reason for such designations in 90% of cases (Bell et al. Citation2013), it seems likely the EFT will have been widely used. A possible reason for omission by participants is its specific design for AQ emissions (although it can also calculate CO2 emissions), whereas the survey was focused on CO2 emissions.
Comparison of results with central government CO2 valuations indicates LGAs are willing to commit far fewer resources to the emissions modelling process than might be expected. The majority of LGAs appear to accept the accuracy provided by EMs currently used (mostly Average Speed EMs) and are reluctant to commit extra resources for increased accuracy, even if that increased accuracy indicates interventions are producing greater emissions reduction outcomes than originally calculated. This highlights that, in order to be tractable, any improvements to CO2 EM accuracy must be at minimal additional cost to LGAs. Both questions produced similarly low mean valuations even though scenarios were worded in different ways.
An important issue with the survey results is the impossibility of being completely certain what was in participants’ minds when answering, and hence uncertainty about whether responses are reflective of organisational-level attitudes or individual-level attitudes. As this article’s title implies, it is organisation-level attitudes that are sought because LGAs engage in the emissions modelling process as a result of organisational decisions, rather than decisions made by a single individual employee. Particularly susceptible to this issue are the two questions designed to quantify LGAs’ willingness to commit resources to emissions modelling. Rather than a simple statement of fact (as in most other questions, e.g. whether particular EMs have been used or not), these questions involved complex, hypothetical scenarios and subjective judgements, but are unlikely to have been discussed at organisational-level prior to response. Hence, comparison with organisational-level central government valuations of CO2 should be treated with caution, although the tendency to under-valuation shown by participants was substantial.
British LGAs do not have a statutory obligation to reduce GHG emissions (DECC Citation2014b). Therefore, non-compliance with any GHG emissions reduction targets LGAs may set voluntarily incurs little penalty, and low CO2 valuations perhaps should not come as a surprise. An interesting contrast is the situation for emissions of oxides of nitrogen (NOx) (of which road traffic is a major source), where the UK is facing potential fines imposed by the European Commission for breach of nitrogen dioxide (NO2) concentration limit values under the EU Air Quality Directive. This financial sanction has been estimated at up to £300 million (Kidney and Price Citation2012), and UK central government is threatening to pass on (full or partial) payment of any fines to LGAs in whose regions these exceedances are occurringFootnote13 (DEFRA Citation2014b). In this situation, LGAs may be willing to commit extra resources to emissions modelling, if that modelling could contribute to a process that demonstrated they were no longer breaching NO2 limits (a pollutant dispersion model and concentration monitoring would be required to complete this process) and fines be avoided. If the threat of financial penalties does lead to NO2 emissions reductions, other pollutant emissions (including CO2) may also be reduced because of potential co-benefits from integrated reduction strategies (DEFRA Citation2009; EEA Citation2009; King et al. Citation2010; Tiwary, Chatterton, and Namdeo Citation2013); although this is not always the case, and a reduction in emissions of one pollutant can sometimes cause increases in another. From a cost-benefit perspective, at a time of scarce resources, there is an increasing need for LGAs to include all possible emissions reduction benefits (i.e. for all pollutants, rather than just NO2) if an argument for implementation of a proposed intervention is to be successful (Tiwary, Chatterton, and Namdeo Citation2013).
Results show that if a transport intervention has high significance to the local political agenda then this was regarded as an important factor in allocation of resources for emissions modelling (third highest factor in ). This finding was reinforced by one participant’s free-text supplementary response which stated that if a ‘political decision has been made that a problem needs to be addressed, as a rule of thumb, we will do whatever it takes (within reason) to make this happen, such as, for example, spend more on a more accurate/detailed modelling package to make sure the case is made and investment is secured’. Threat of fines for breaching NO2 limits has pushed this issue up the local political agenda, and it seems likely more resources will be available for any mitigation actions (including modelling the impact of transport interventions). For example, the DfT recently (November 2015) invited proposals to be submitted for novel solutions to the local transport and AQ problem, with grants available for successful submissions from a total fund of £250,000 (DfT Citation2015). In general, placing increasing responsibility on LGAs to be accountable for achieving their environmental targets should result in the impact of transport interventions on emissions (including CO2) being increasingly pushed up the local political agenda. In turn, this should lead to an increasing willingness on the part of LGAs to commit more resources to the emissions modelling process.
5. Conclusions
A central message of the survey results is that scarcity of resources is an important issue for LGAs when conducting road traffic emissions modelling. Participants placed particular importance on EMs having the flexibility to be reusable for assessment of future transport interventions, and on a requirement for input data to be easily available. Of the road traffic data sources that can provide EM inputs, RTMs and UTC systems represent the most convenient options for LGAs for three reasons: (1) they are ranked highly as easily obtainable and routinely collected; (2) they are ranked highest and second-highest, respectively, as planned for future collection and (3) they can provide traffic variables on a link-by-link basis for all (or mostly all) links in a network. Micro-RTMs can additionally generate individual vehicle driving pattern outputs, but doubts exist concerning their accuracy and their collection and processing is resource-intensive. Therefore, an EM based on traffic variable outputs from RTMs and UTC systems appears to be the most appropriate option for LGAs. An ability to use data from either source is important to overcome situations where UTC data are not available (i.e. links with no ILD installed or hypothetical scenarios). A trend was identified of a move away from road traffic data sources involving manual, labour-intensive data collection processes, and a move towards sources involving automatic, less labour-intensive collection processes. Explanations for this trend could be increasing pressure on LGA resource budgets, and/or an expectation that the rise in telematics and ‘big’ data will satisfy LGA traffic data requirements.
The large majority of LGA emissions modelling is currently achieved using Average Speed EMs, with more detailed EM types being used rarely (if at all). However, other traffic variables (in addition to traffic average speed) are easily available for incorporation and could offer opportunities to improve the accuracy of EMs for LGAs. This is an area for further investigation and development work. Any accuracy improvements must come at minimal cost to LGAs because they have indicated a reluctance to commit extra resources for improved accuracy. However, overcoming this barrier is likely to be possible where a transport intervention is of high significance to the local political agenda. In these circumstances, more resources are likely to be made available for the emissions modelling process.
Acknowledgements
The support of Southampton City Council in completing this work is gratefully acknowledged. The data set used in this study is managed by the University of Southampton. All data sharing must meet the terms of existing participants’ consent and study ethical approvals. The terms of this study’s ethics approval (Research Ethics Committee number 13144) and the terms of the participants’ consent form prevent the sharing of data in this instance.
Disclosure statement
No potential conflict of interest was reported by the authors.
Additional information
Funding
Notes
1. In contrast to private roads, adopted roads (which constitute the vast majority of British roads) are those maintained at public expense.
2. Great Britain comprises England, Scotland and Wales.
3. In this article, LGA is used in preference to LHA because it is a more typical term used to describe local government institutions. However, where LGA is used, this implies a local authority with responsibility for non-SRN roads in their area of administration.
4. CO2-equivalent: amount of CO2 emitted that would cause the same time-integrated radiative forcing, over a given time horizon, as an emitted amount of another GHG.
5. Whilst Wales is not included in this quote, the comparison has merits because England and Scotland together comprise 184 out of the total for Britain of 206 LHAs.
6. The Local Government Association is the national organisation representing LGAs in England and Wales.
7. Produced by Mott MacDonald.
8. Simulation and Assignment of Traffic to Urban Road Networks – developed at the Institute for Transport Studies, University of Leeds and distributed by Atkins Limited.
9. A platoon-dispersion module simulates how a platoon of vehicles released by a green traffic signal disperses as the vehicles progress through the network.
10. Regional Associate, Highways and Transportation, Parsons Brinckerhoff.
11. Net revenue expenditure is gross expenditure less fees and charges for LGA services and specific grants, where specific grants are grants ring-fenced for dedicated purposes.
12. Space-mean-speed is calculated from the arithmetic mean of measured travel times over a measured distance of all vehicles during a given survey period. In contrast, time-mean-speed is the arithmetic mean of measured speeds (i.e. spot speeds) over a short measured distance of all vehicles during a given survey period.
13. At the time of paper revision (June 2016), the UK has just voted to leave the EU and the threat of this fine will recede, which could mean resources for emissions modelling may start to slip down in priority.
References
- Bell, M. C., F. Galatioto, A. Chakravartty, and A. Namdeo. 2013. “A Novel Approach for Investigating the Trends in Nitrogen Dioxide Levels in UK Cities.” Environmental Pollution 183: 184–194. doi:10.1016/j.envpol.2013.03.039.
- Boulter, P. G., I. S. McCrae, J. Price, and P. Emmerson. 2012. A City-Wide Road Traffic Emission Model for Oxford – Scoping Report (PPR473). Wokingham: Transport Research Laboratory.
- Department for Communities and Local Government (DCLG). 2015. Local Government Financial Statistics England – No. 25 2015. London: Department for Communities and Local Government.
- Department of Energy & Climate Change (DECC). 2014a. 2012 UK Greenhouse Gas Emissions, Final Figures. London: Department of Energy & Climate Change.
- Department of Energy & Climate Change (DECC). 2014b. Local Authority Carbon Dioxide Emissions Estimates 2012. London: Department of Energy & Climate Change.
- Department for Environment Food and Rural Affairs (DEFRA). 2009. Local Air Quality Management – Policy Guidance (PG09). London: Department for Environment Food and Rural Affairs.
- Department for Environment Food and Rural Affairs (DEFRA). 2014a. Air Pollution in the UK 2013. London: Department for Environment Food and Rural Affairs.
- Department for Environment Food and Rural Affairs (DEFRA). 2014b. Response Under the Environmental Information Regulations 2004 to a Request for Information About air Pollution Infraction Fines. London: Department for Environment Food and Rural Affairs.
- Department for Transport (DfT). 2013. Action for Roads – A Network for the 21st Century. Norwich: The Stationery Office.
- Department for Transport (DfT). 2014. WebTAG Databook (Spreadsheet November 2014). London: Department for Transport.
- Department for Transport (DfT). 2015. DfT Local Transport Air Quality Challenge Innovation Grant – Grant Specification and Evaluation Criteria. London: Department for Transport.
- Dunn, Olive Jean. 1964. “Multiple Comparisons Using Rank Sums.” Technometrics 6 (3): 241–252. doi: 10.1080/00401706.1964.10490181
- European Environment Agency (EEA). 2009. EMEP/EEA air Pollutant Emission Inventory Guidebook 2009 – Technical Guidance to Prepare National Emission Inventories – Part A – Chapter 1: Guidebook Introduction. Copenhagen: European Environment Agency.
- Field, Andy. 2009. Discovering Statistics Using SPSS. 3rd ed. London: Sage.
- Fink, Arlene. 2003a. The Survey Kit 2 – How to Ask Survey Questions. 2nd ed. Thousand Oaks, CA: Sage.
- Fink, Arlene. 2003b. The Survey Kit 6 – How to Design Survey Studies. 2nd ed. Thousand Oaks, CA: Sage.
- Friedman, M. 1937. “The Use of Ranks to Avoid the Assumption of Normality Implicit in the Analysis of Variance.” Journal of the American Statistical Association 32: 675–701. doi: 10.1080/01621459.1937.10503522
- Grote, Matt, Ian Williams, John Preston, and Simon Kemp. 2016. “Including Congestion Effects in Urban Road Traffic CO2 Emissions Modelling: Do Local Government Authorities Have the Right Options?” Transportation Research Part D: Transport and Environment 43: 95–106. doi: 10.1016/j.trd.2015.12.010.
- Han, Jiang, John W. Polak, Javier Barria, and Rajesh Krishnan. 2010. “On the Estimation of Space-Mean-Speed From Inductive Loop Detector Data.” Transportation Planning and Technology 33 (1): 91–104. doi:10.1080/03081060903429421.
- Hirschmann, Karin, Michael Zallinger, Martin Fellendorf, and Stefan Hausberger. 2010. “A New Method to Calculate Emissions with Simulated Traffic Conditions.” IEEE 13th International Annual Conference on Intelligent Transportation Systems, Madeira Island, Portugal, September 19–22.
- International Energy Agency (IEA). 2014. CO2 Emissions from Fuel Combustion – Highlights. Paris: International Energy Agency.
- Kidney, David, and Howard Price. 2012. Occasional Paper: Part 2 of the Localism Act 2011. London: Chartered Institute of Environmental Health.
- King, D., O. Inderwildi, C. Carey, G. Santos, X. Yan, H. Behrendt, A. Holdway, N. Owen, T. Shirvani, and A. Teytelboym. 2010. Future of Mobility Roadmap – Ways to Reduce Emissions While Keeping Mobile, edited by D. King. Oxford: Smith School of Enterprise and the Environment.
- Kotsialos, Apostolos, Markos Papageorgiou, Christina Diakaki, Yannis Pavlis, and Frans Middelham. 2002. “Traffic Flow Modeling of Large-Scale Motorway Networks Using the Macroscopic Modeling Tool METANET.” IEEE Transactions on Intelligent Transportation Systems 3 (4): 282–292. doi: 10.1109/TITS.2002.806804
- Lighthill, M. J., and G. B. Whitham. 1955. “On Kinematic Waves. II. A Theory of Traffic Flow on Long Crowded Roads.” Proceedings of the Royal Society A: Mathematical, Physical and Engineering Sciences 229 (1178): 317–345. doi: 10.1098/rspa.1955.0089.
- Lineback, Joanna Fane, and Katherine J Thompson. 2010. “Conducting Nonresponse Bias Analysis for Business Surveys.” 2010 Joint Statistical Meetings (JSM), Vancouver, Canada, July 31–August 5.
- Lowndes, Vivien, and Kerry McCaughie. 2013. “Weathering the Perfect Storm? Austerity and Institutional Resilience in Local Government.” Policy & Politics 41 (4): 533–549. doi:10.1332/030557312X655747.
- Mann, H. B., and D. R. Whitney. 1947. “On a Test of Whether one of Two Random Variables is Stochastically Larger than the Other.” The Annals of Mathematical Statistics 18 (1): 50–60. doi: 10.1214/aoms/1177730491
- Marsden, Greg, and Tom Rye. 2010. “The Governance of Transport and Climate Change.” Journal of Transport Geography 18 (6): 669–678. doi:10.1016/j.jtrangeo.2009.09.014.
- Meek, Stuart, Stephen Ison, and Marcus Enoch. 2010. “UK Local Authority Attitudes to Park and Ride.” Journal of Transport Geography 18 (3): 372–381. doi:10.1016/j.jtrangeo.2009.09.005.
- Oppenheim, A. N. 1992. Questionnaire Design, Interviewing and Attitude Measurement. New ed. London: Continuum.
- Ortúzar, Juan de Dios, and Luis G Willumsen. 2011. Modelling Transport. 4th ed. Chichester: John Wiley & Sons.
- Papacostas, C. S., and P. D. Prevedouros. 2005. Transportation Engineering & Planning. SI ed. Jurong: Prentice Hall.
- Ramos, Ana Luísa, José Vasconcelos Ferreira, and Jaume Barceló. 2011. “Intelligent Urban Traffic: A Guide Through Micro Modelling Approaches.” IEEE International Conference on Systems, Man, and Cybernetics (SMC), Anchorage, USA, October 9–12.
- Southampton City Council (SCC). 2011a. Local Transport Plan 3 – Strategy and Implementation Plan for Southampton. Southampton: Southampton City Council.
- Southampton City Council (SCC). 2011b. Southampton Sustainable Travel City – Local Sustainable Transport Fund Bid. Southampton: Southampton City Council.
- Smit, Robin. 2006. “An Examination of Congestion in Road Traffic Emission Models and their Application to Urban Road Networks.” Thesis submitted for Doctor of Philosophy, Griffith University, Brisbane, Australia.
- Smit, Robin, Leonidas Ntziachristos, and Paul Boulter. 2010. “Validation of Road Vehicle and Traffic Emission Models – A Review and Meta-Analysis.” Atmospheric Environment 44 (25): 2943–2953. doi: 10.1016/j.atmosenv.2010.05.022.
- Smit, Robin, Muriel Poelman, and Jeroen Schrijver. 2008. “Improved Road Traffic Emission Inventories by Adding Mean Speed Distributions.” Atmospheric Environment 42 (5): 916–926. doi: 10.1016/j.atmosenv.2007.10.026.
- Song, Guohua, Lei Yu, and Long Xu. 2013. “Comparative Analysis of Car-Following Models for Emissions Estimation.” Transportation Research Record: Journal of the Transportation Research Board 2341: 12–22. doi: 10.3141/2341-02
- Song, Guohua, Lei Yu, and Yanhong Zhang. 2012. “Applicability of Traffic Microsimulation Models in Vehicle Emissions Estimates.” Transportation Research Record: Journal of the Transportation Research Board 2270: 132–141. doi: 10.3141/2270-16
- Tiwary, Abhishek, Tim Chatterton, and Anil Namdeo. 2013. “Co-managing Carbon and air Quality: Pros and Cons of Local Sustainability Initiatives.” Journal of Environmental Planning and Management, 1–18. doi: 10.1080/09640568.2013.802677.
- Toffolo, Silvana, Eugenio Morello, Daniele Di Rocco, Werner Maier, Cristina Valdes, Alvaro Garcia-Castro, Ilias Vouitsis, Leonidas Ntziachristos, Zissis Samaras, and Vincenzo Punzo. 2013. “ ICT-Emissions Deliverable 2.1 – Methodology.” Brussels, Belgium: European Commission.
- Xenias, Dimitrios, and Lorraine Whitmarsh. 2013. “Dimensions and Determinants of Expert and Public Attitudes to Sustainable Transport Policies and Technologies.” Transportation Research Part A: Policy and Practice 48: 75–85. doi: 10.1016/j.tra.2012.10.007.