Abstract
Outlier detection has been broadly used in industrial practices such as public security and fraud detection, etc. Outlier detection from various perspectives against different backgrounds has been proposed. However, most of outlier detection consider categorical or numerical data. There are few researches on outlier detection for set-valued data, and a set-valued information system (SVIS) is a proper way of tackling the problem of missing values in data sets. This paper investigates outlier detection for set-valued data based on rough set theory (RST) and granular computing (GrC). First, the similarity between two information values in an SVIS is introduced and a variable parameter to control the similarity is given. Then, the tolerance relations on the object set are defined, and based on this tolerance relation, θ-lower and θ-upper approximations in an SVIS are put forward. Next, the outlier factor in an SVIS is presented and applied to various data sets. Finally, outlier detection method for set-valued data based on RST and GrC is proposed, and the corresponding algorithms are designed. Through numerical experiments based on UCI, the designed algorithm is compared with six other detection algorithms. The experimental results show the designed algorithm is arguably the best choice under the context of an SVIS. It is worth mentioning that for a comprehensive comparison, we use two criteria: AUC value and F-1 measure, to show the superiority of the designed algorithm.
Acknowledgements
The authors would like to thank the editors and the anonymous reviewers for their valuable comments and suggestions, which have helped immensely in improving the quality of the paper.
Disclosure statement
No potential conflict of interest was reported by the author(s).
Additional information
Funding
Notes on contributors
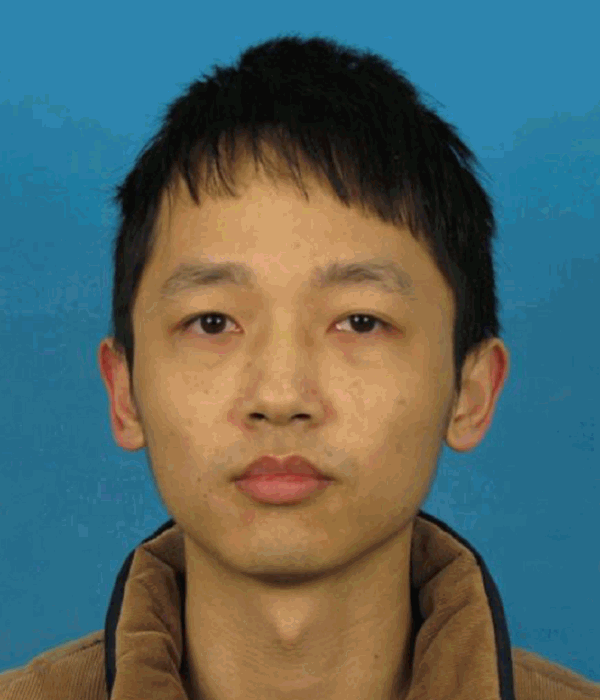
Hai Lin
Hai Lin is a master student at school of Mathematics and Information Science, Guangxi University, Nanning, Guangxi, China. His main research interests include granular computing, rough set theory and information systems.
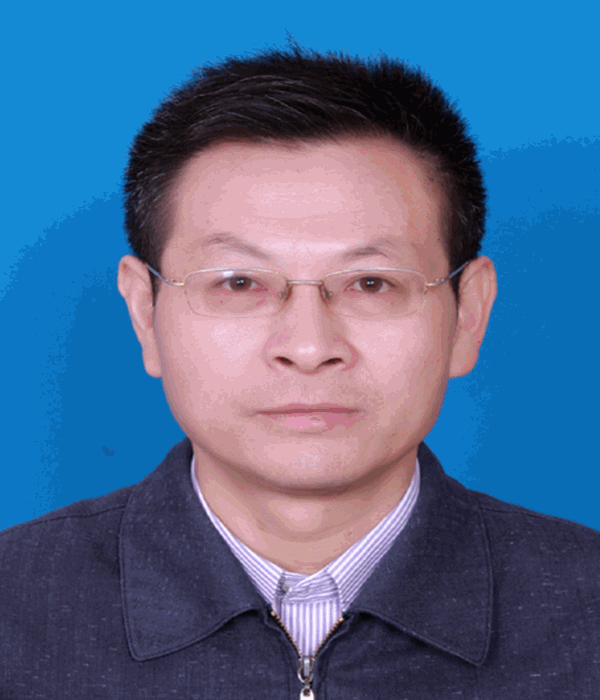
Zhaowen Li
Zhaowen Li received the M.Sc. degree in Mathematics from Guangxi University, Nanning, China, in 1988 and the Ph.D. degree in Mathematics from Hunan University, Changsha, China, in 2008. He is currently a professor in School of Mathematics and Statistics, Yulin Normal University. His research interests include granular computing, rough set theory, data mining, fuzzy set theory and information systems.