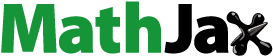
ABSTRACT
This paper elaborates on forecasts in the road traffic and freight flows in the urban–port transport system to quantify the effects of the freight traffic performance considering road capacity expansion in a port city in Poland. The urban–port transport system was broken down into a subsystem of roads that lead directly to the port transshipment areas and a subsystem of urban roads with mixed freight and passenger traffic. The research resulted in an elaborated long-term forecast of freight and traffic flows in the porttransshipmenturban road system. Also, the impact of capacity improvements in the local transport system on port-related freight traffic performance was quantified using an incremental calculus for transport performance for two options: with and without road capacity expansion. As investigated, an intervention will lead to a reduction in time of freight traffic trips, freight transports, and drivers working time as well as to decreased emissions of CO2.
1. Introduction
Interactions at the interface between ports and cities feature in the functioning and development of port cities and are a new and developing area of research.
As stated by Merk and Dang (Citation2013), the interactions between ports and cities where these ports are located have evolved and it is no longer obvious that ports considered as well functioning have a net positive impact on the port/city interface. Conflicts that arose over the environment, air and water pollution, congestion, and other impacts caused by port-related traffic have emerged as new arenas of tension in the interrelationship between ports and cities (Hall Citation2018). Congestion of cargo and passenger transport in port cities is an essential problem and comes not only from port-related cargo and passenger traffic but also from the fast economic growth and development of these cities (Thompson and Taniguchi Citation2008). The accumulation and combination of both port-related and city-related traffic flows result in substantial congestion at port–city interfaces.
While city-related road traffic embraces freight traffic and passenger transport for goods supply and support businesses that operate in the city and its surroundings, cargo traffic is created by seaports that are in urban spaces. As a result, the urban road system must deal with blended passenger–cargo traffic of high intensity and overall road congestion.
For port-related traffic, road congestion affects the rise of travel times and fuel costs, and at the same time, it affects the lowering of the reliability of truck operations, hinders efficient usage of port assets, and deteriorates port competitiveness and its connectivity to markets (Aronietis, Van de Voorde, and Vanelslander Citation2010; Parola et al. Citation2016; Martinez Moya and Valero Citation2017; Notteboom and Yim Yap Citation2012).
For city-related traffic, traffic congestion has an impact on the reduction of population mobility, losing of working time, and increase of environmental costs. In general, traffic congestion has a negative impact on social and economic welfare (OECD Citation2013).
The hinterland traffic of the good part of ports is prevailed by trucks. Moreover, even the ports, where the non-truck hinterland traffic is quite high, can rarely manage to achieve more than half of its traffic by other transport modes than trucks. However, it is truck traffic that causes most of the congestion in port surroundings and thereby generates most external costs of transport. (Merk and Notteboom Citation2015).
The most applied congestion mitigation approaches are various-sector traffic management, providing more transit and freight rail service, operating the existing infrastructures more efficiently, improving road infrastructure by reconstruction of existing roads and/or adding additional lanes (Litman Citation2016, Citation2007; Treintis et al. Citation2011).
Traffic management instruments that can be applied to mitigate the effects of truck usage in hinterland transport including terminal appointment systems, expanding gate hours, truck retirement programmes, and virtual container yard systems have been well analysed and documented (e.g., Giuliano and O′ Brien Citation2007, Citation2008).
Mitigation of congestion within port–city transport systems may result from implementation/availability of dry port in hinterland transportation systems. Dry ports reduce external costs caused by road congestion and accidents (Roso, Woxenius, and Lumsden Citation2009; Korovyakovsky and Panova Citation2011), congestion and waiting time at a seaport and in seaport cities (Roso Citation2009, Citation2013; Roso and Lumsden Citation2010), and limit CO2 emissions at seaport area and in local roads (Roso Citation2007; Lättilä, Hentuu, and Hilmola Citation2013). Dry ports encourage a modal shift, which leads to traffic reduction and congestion decreasing at seaport gates and even in entire area of seaport cities. However, these benefits can only be obtained if dry ports will be merged into transport systems of seaport hinterland, and in cases of poor planning or failure in building well-functioning transport timetables between seaports and dry ports, greenhouse gas emissions and road congestion could increase rather than decrease (Hanaoka and Regmi Citation2011).
There are emerging studies on relationships between seaports and dry ports at the micro-level (Muravev and Rakhmangulov Citation2016; Muravev et al. Citation2019). Simulation of a seaport–dry port system with the use of parameters like intensity of traffic flows, diversity of traffic flows, distance between seaports and dry ports, capacity of transport rail connections, environmental parameters, location of the dry port, and storage capacity of the dry port is analysed with the use of system dynamics models (Muravev et al. Citation2020).
Nevertheless, improving road capacity is one of the most often used and relevant strategies for congestion mitigation (e.g., FHWA Citation2004). Sufficient capacity of infrastructure is a main prerequisite for mitigation of congestion within port–city transport system.
The benefits of improving capacity of road system can be diminished by the induced traffic of passenger cars and cargo transit. Identifying this effect of road capacity extension is a complex problem, and it is concerned with elaborately and rapidly interlinked system components: transport supply system, accessibility, land use, and travel demand. Based on that literature review (see Kitamura Citation2010 for reference review), it is concluded that there are no empirical evidence and indications that increased capacity of the road system creates a significant volume of traffic induced. Also, the benefits to port-related traffic may dominate negative effects of the commuters induced and goods transit traffic. (De Borger, Proost, and Van Dender Citation2008, Citation2005). Because of the difficulty in estimation, the problem defined as the effect of trip-inducing by improved road capacity is not incorporated in the standard procedure of travel demand forecasting (Rothengatter Citation2000; Naess, Nicolaisen, and Strand Citation2012).
Theoretically, predictions of the impact that road capacity has on inter-port competition rests mainly on port duopoly competition (De Borger, Proost, and Van Dender Citation2008) and assumes that rival ports compete either in price (road toll) or in the volume of handled containers.
Zhang (Citation2008) focuses on capacity improvement and corridor congestion. Competition between different ports is considered as the competition between alternate intermodal transport chains, while conditions of hinterland access are represented by both inland roads and corridor facilities. Unlike ports that compete in quantity, an increase of corridor capacity will increase one port’s cargo transshipment, increasing its profit and reducing at the same time its rival port’s transshipment. Alternatively, an increase in the capacity of inland road may or may not increase the same port’s cargo transshipment and profits due to different offsetting effects (Wan, Basso, and Zhang Citation2016).
Yuen, Basso, and Zhang (Citation2008) examine the effects of congestion pricing implemented at a gateway port on the optimal road pricing in hinterland area, road congestion, and social welfare. Further, Wan, Zhang, and Yuen (Citation2013) study the impact of conditions of hinterland access and urban road congestion on the competition of major US container ports. They conclude that adding first/last mile roads and more local roads tends to benefit the port and does harm its rivals (in terms of throughput) by constraining road congestion.
Wan and Zhang (Citation2013) conclude that an increase in road capacity or increasing tolls may increase a port’s profit and reduce its rival port’s profit. Wan, Yuen, and Zhang (Citation2014) explore the influence of hinterland accessibility on the performance of US container terminals. In general, there is a negative correlation between road congestion in the port surroundings and port performance.
A common feature of the reviewed studies is the use of modelling, simulations and, in most cases, aggregate level concept. These studies take a strategic view and examine the interaction between port competition and hinterland accessibility with the use of game theory models and up-to-bottom approach and assume ports compete for a common hinterland, exploring the effects of road congestion and capacity improvement on port competition and efficiency also welfare implications for the port regions. In these studies, empirical findings on road traffic flow developments are constrained. Moreover, the role of investment in the port–city road system and the impact of such investments on road congestion have not been well explored. Consequently, studies into port–city road systems are deficient or restricted to theoretical findings, which limit their practical utility.
This study proposes a method that can fill the existing research gaps and provides potential generalizability as well as practical merits. It focuses on the local captive market (first/last mile road sections in the urban-port road system) and the road cargo traffic of a specific port. The bottom-up approach has been performed in the empirical analysis. It allows to distinguish out the complex transport system, sub-systems being the subjects for further investigation. With the use of primary measures, the issues of forecasting of port road freight traffic are considered explicitly but in terms of interaction with other traffic modes, and this method is rarely found in the existing literature. Importantly, this approach allows to research the implications of capacity expansion on road congestion, a case that seems especially policy relevant for the port cities and local governments. Consequently, the impact of road transport capacity improvement on port-related truck traffic can be specified and evaluated, whereas the empirical studies in this topic are hard to locate in the existing studies.
The major research problems of the manuscript have been defined as follows:
How can we predict the port-related road traffic and freight flows in the complex urban-port road transport system?
How can we estimate the impact of capacity improvements in the local transport system on port-related freight traffic performance?
This paper elaborates forecasts in the road traffic and quantifies the effects of the freight traffic performance considering the reconstruction of the existing local system of roads in the port city of Szczecin, located in the north-western Poland. The length of the roads will remain unchanged, but road surface will be resurfaced, and road axle load will be increased. Some sections of road are planned to be widened, entrance/exit lanes to be reconstructed, the road curvature to be reduced, entrance/exit ramps to be reconstructed, and a few intersections to be reconstructed or built. Lastly, the number of parking places for trucks will be higher after the improvement. The modernised road system is scheduled to be operational from 2024, and the effects of its improved capacity have been set for next 20 years (in 2024–2043).
2. Research concept and data used
Research framework of truck flow prediction and effects of capacity improvement in the system of urban–port roads are presented in .
The urban–port transport system was divided into a subsystem of roads that lead directly to the port loading and unloading areas and a subsystem of urban roads with mixed freight and passenger traffic.
In the former subsystem, a truck traffic forecast was derived from the previously produced forecast of the port throughput. In the case of mixed traffic roads, the traffic flow was measured, and then the future truck traffic was predicted using the elasticity between GDP and truck traffic.
Further, our study advances research on the expected effects of road capacity expansion on port-related traffic performance. Given the interaction between diverse types of road users, traffic congestion relief has been measured directly in the port-related traffic and caused an increase in trucks′ speed. The impact of capacity improvements in the local transport system on port-related freight traffic was quantified using an incremental calculus for truck traffic performance for two options: with and without road capacity expansion.
Primary road traffic flow measurements were made in line with the General Directorate for National Roads and Motorways methodology (Method of General Traffic Measurement Citation2015), and motor vehicles included passenger cars, vans, light vehicles, trucks without and with trailers, and buses.
Annual average daily traffic (AADT) means the average number of vehicles that pass a given section of a road daily. AADT is calculated as follows:
where
AADTi—annual average daily traffic of motor vehicle at the i-th road;
MRi—annual average daily traffic on working days (from Monday till Friday between 6 a.m. and 10 p.m.) at the i-th road;
0,85⋅MRi—annual average daily traffic on Saturdays and days before holidays (between 6 a.m. and 10 p.m.) at the i-th road;
MNi—annual average daily traffic on Sundays and holidays (between 6 a.m. and 10 p.m.) at the i-th road;
RNi—annual average traffic in the night (between 10 p.m. and 6 a.m.) at the i-th road;
N1—the number of working days within a year (in 2016: 252);
N2—the number of Saturdays and days just before holidays (in 2016: 53);
N3—the number of Sundays and holiday days within a year (in 2016: 61); and
N—the total number of days in a year (in 2016: 366).
The average annual traffic (AAT) is a multiplication of AADT by 365 days. Results of primary traffic flow surveys conducted in the road urban–port system in Szczecin are presented in .
Table 1. Results of primary traffic flow surveys conducted in the road urban–port system in Szczecin from October 2016 to November 2016.
The speed of trucks was measured for roads with the highest level of technical wear and tear in the city–port transport system. The truck speed was gauged on three road sections with respective length of 396.5 m, 280.5 m, and 394.5 m. The measurement of speed was based on the representative sample of 34 measurements of truck transit time. The average truck speed and standard error of the estimation were calculated. The estimated average truck speed was 15.58 ± 0.51 km/h (standard error), which corresponds to the speed on roads with a high degree of technical wear and tear. The standard error amounted to 3.3% of the estimated mean value.
In the forecasting works, we used GDP forecasts of the Ministry of Development and Finance recommended for the Polish transport sector (retrieved from the Center of European Union Transport Projects, CUPT). The GDP forecast for 2019–2043 is expressed in the annual average constant prices as growth indicators (calculated year-on-year, previous year = 100) and in corresponding values; however, the forecast did not consider the economic impact of the COVID-19 pandemic. The GDP forecast was applied for forecasting the port throughput as well as for predicting road traffic flow. The long-term prediction of the port throughput and truck traffic forecast have been elaborated for every year and for each cargo group (coal, iron ore + other bulk, grain, general cargo, container general cargo, and oil and oil products) and for each road in the forecasting period to the year of 2043.
The subject of research is a local road system at the approach to port, O-D is limited to one pair: main collecting transit road—port, while the roads within system are streamlined to specific port areas.
As from the data retrieved from the port industries, we estimated (1) the modal split of Szczecin port hinterland (trucks, railway, and waterways) for six cargo groups, i.e., coal, iron ore + other bulk, grain, general cargo, container general cargo, and oil and oil products for the years from 2009 to 2014, (2) historical port throughputs in Polish seaports, and (3) road traffic distribution by the entry gates to the port of Szczecin.
To determine the number of trucks serving the projected volume of port transshipments, we used trucks with the average load weight (tonnes): for containers 18.8, for dry bulk 20.0, for grain and general cargo 18.0, for oil and fuels 34.0, and for liquid gas 25.0.
Data of unit emission factors in tCO2/vehicle-km in relation to the speed of heavy goods vehicles and distinguished for roads—with surface after construction/reconstruction and with degraded surface—were retrieved from ‘Blue Book. Road Infrastructure’ prepared by Jaspers (Blue Book Citation2015).
The statistical relationships between road traffic flow (number of vehicles) and speed with reference to roads capacity in terms of wear and tear and technical parameters were obtained from the ‘Instruction for Assessing the Economic Efficiency of Road and Bridge Projects—Verification of the Research Method According to EU Recommendations and Update of Unit Prices as of 2007. Part II, Tables of Speed’ (Instruction Citation2008).
3. Forecasted demand for transshipment at small- and medium-sized ports
Good part of academic works on port throughput predictions deals with container transshipment only and takes a pure modelling approach. The most common methods are Univariate Autoregressive Moving Average Models (ARMA) and Autoregressive Integrated Moving Average Models (ARIMA) (Jarrett Citation2015; Klein, Citation1996); Seasonal Autoregressive Integrated Moving Average (SARIMA) (Chen and Chen Citation2010); Vector Autoregressive Models (Veenstra and Haralambides Citation2001), and error-correction models (Fung Citation2002; Hui, Seabrooke, and Wong Citation2004). However, methods based on both linear and non-linear trendline extrapolation and econometric modelling are less suitable for long-term predictions of port throughput.
In practice, forecasts are usually based on causal relationships between port transshipments and certain socio-economic variables selected with existing theories, knowledge, experience, and best practice of researcher.
So far, some attempts have been made in scientific work to forecast port transshipments for a very long-term perspective, e.g., till 2100, based on a model that relates port transshipments to GDP growth (Van Dorsser et al., Citation2011). In another paper (De Langen, van Meijeren, and Tavasszy Citation2012), authors proposed a long-term forecasting approach that applied a freight transport model. Expert judgment and commodity-specific research were used in that model. Relationships between port transshipments and GDP and industrial growth have been applied in the port of Antwerp to forecast transshipments of general cargo, as well as bulk cargo, and iron ore in particular (Meersman, Moglia, and Van de Voorde Citation2002). The cargo transshipment depends on imports and exports, which are a function of GDP. Therefore, GDP is a proper factor that can be considered as an explanatory variable in cargo transshipment models. The demand for port services is an outcome of the demand for exports and imports. Another empirical evidence (Wang Citation2009) proved that the coefficient of correlation between GDP and container reloading was remarkably high and amounted 0.97. It testifies to a strong and positive correlation between variables of interest. Thus, GDP is considered as a good example for an explanatory variable in forecasting. The one-dimensional linear regression equation proposed by Wang indicated the relationship between ports and the economy. In another paper (Chou, Chu, and Liang Citation2008), forecasts of the import container transshipments of Taiwan were developed with the usage of a modified regression model.
There are known attempts (Matczak Citation2020) to the use of relevant indexes of containerisation that refer to the macroeconomic descriptors of the country of cargo origin and the country of cargo destination such as foreign trade, population, and gross domestic product.
So far, port cargo turnover forecasts have focused primarily only on major ports and on container turnover mostly. The issue of the throughput prediction in minor seaports has been omitted in the scientific research. In contrast to major seaports, cargo throughput forecasts in smaller seaports cover many types of cargo (besides containers) and are mostly determined by the relationships that occur between major and minor seaports that are in the concerned range.
The port of Szczecin is located about 65 km south from the Baltic Sea. The waterway from Świnoujście, which is situated by the sea, to Szczecin is a water connection between port of Szczecin and the Baltic Sea. Thus, port of Szczecin can handle seagoing vessels and is considered as universal port. The annual cargo transshipment of 9 million tons qualifies it as a small- and medium-sized Baltic seaport. The main disadvantage of the port is the limited navigational access to it due to the depth of the fairway, which will be 12.5 m after modernization. Recently, large-scale investments are being accomplished in the port of Szczecin. It will improve the nautical access to the port. Larger vessels up to 40,000 DWT in comparison to nowadays 20,000 DWT will be able to be served in the port. It will also significantly increase the cargo handling capacity.
Due to infrastructural limitations related to its low nautical and transport accessibility, the port of Szczecin faces obstacles that reduce its throughput capacity and development. Thus, its transport importance has been diminishing. Hitherto, the forecasting process of freight flow was based directly on trendlines extrapolation or referred to internal conditions of Szczecin’s port that was burdened with structural limitations in port growth. Relations with major ports were ignored. Following numerous papers and scientific works on the pace of the growth of multi-port systems (e.g. Hayuth and Fleming Citation1994; Notteboom Citation2005; Feng and Notteboom Citation2011, Citation2013; Slack and Wang Citation2002; Wang, Wang, and Ducret Citation2012; Wilmsmeier and Monios Citation2013), it is generally acknowledged that the development of small- and medium-size ports (SMPs) is impacted by its interactions with major ports that, in turn, are based on the competition and complementarity of meeting the growing demand for services in large seaports. In this case, the port of Szczecin is related with three major Polish seaports such as Świnoujście, Gdańsk, and Gdynia. The method of cargo transshipment forecasting in port of Szczecin is therefore intermediate. It means that first the demand for transshipments in major ports must be forecasted. Then, figures obtained should be changed into statistical chain indices that can be finally used in forecasting process of cargo turnover in small and medium-sized seaports such as the port of Szczecin. This procedure assumes that improved port accessibility (infrastructural investments and facilities improvements) will make the port comparable in terms of the pace of development to large seaports.
To determine transshipment forecasts in major Polish seaports, regression models of transshipments by cargo groups in relation to Polish GDP were used with the following general formula (Bernacki and Lis Citation2017):
where
—the cargo throughput of the j-th group over time t;
GDPt—the observations of the GDP over time t;
—the structural parameters of the regression model for the j-th cargo group; and
—the random component of the model over time t for the j-th cargo group.
Transshipments of all cargo groups in major Polish ports were correlated with GDP in Poland. Moreover, all correlations were positive, strong, and statistically significant at the significance level of 0.05.
Applying the extrapolation method of cargo turnover models, forecasts of all types of cargo transshipment in major Polish seaports for the years 2020–2043 were produced.
The matrix of demand indices for the transshipment of all types of cargo, as established for major seaports in Poland, was used to forecast the demand for port services in Szczecin. Given the knowledge of transshipments in the port of Szczecin in 2019 and the matrix of chain indices based on increases obtained for cargo throughput in major ports, the recursive equation was formulated to produce cargo transshipment forecasts in the port of Szczecin as follows:
where:
—forecasts for j-th cargo group in time t;
—forecasts for j-th cargo group in time t-1;
—the annual chain indices of cargo throughput growth in j-th cargo group; and
—the average level of transit in j-th cargo group determined from k time periods.
Regarding sea relations with the port of Szczecin, no increase in shipping size of general cargo, chemical products, and oil products is expected in the forecasting period and, consequently, in the size of vessels. Thus, for the purpose of projections, it was assumed that the transshipments of general cargo and crude oil and its products will not change. It means that these types of cargo will remain at average throughput volumes recorded from 2007 to 2019. Moreover, the handling capacity of the port of Szczecin has influenced on the necessary reduction in cargo transshipment volumes.
Throughput forecast by cargo groups in port of Szczecin is depicted in .
Table 2. Throughput forecast by cargo groups in port of Szczecin (tonnes).
The produced forecasts of cargo transshipments in Szczecin’s port anticipate a long-term increase from 9.8 to 14.7 million tonnes (+4.9 million tonnes). A moderate increase in transshipment forecasts for the port of Szczecin indicates that first, its universal character will be kept in the future, and secondly, port will serve as a complementary port to the major ports of the region.
4. Forecasted truck traffic volume in the subsystem of roads leading directly to the port’s reloading areas
The research process was conducted as follows:
For roads leading directly to the port reloading areas, the traffic forecast was produced based on the elaborated forecast of transshipment at the port of Szczecin.
The mode structure of hinterland transport (road, rail, or inland waterway) revealed for specific cargo groups was used in forecasting of hinterland traffic. Low cargo turnover as well as difficulties in arranging sustainable rail and inland waterway corridors for shipping larger volumes of cargo also lack dry ports in the port vicinity are factors limiting strategic modal shifts in the future. Therefore, future small and periodical frictions in hinterland mode structure have been disregarded in our analysis.
The projected truck traffic flow on the roads leading directly to the port reloading areas was calculated using the actual traffic distribution by entry gates to the port areas and individual roads.
The organisational and trading conditions of freight in the seaport service are such that cargo deliveries are destined for specific consignees, and each freight transport is organised specifically for a given shipment. This translates into the movement of heavy vehicle traffic to and from the port; trucks arrive at the port with cargo and leave without cargo or arrive at the port without cargo and leave the port with cargo. In case of universal and minor ports, because of lower number of containers transshipments, segmented transport solutions based on feeder services and streamlined cargo distribution to specific consignees, containers pick-ups and drop-offs are rarely coordinated in the port. Therefore, the predicted number of trucks that will appear at the port’s reloading areas should be doubled.
Mean shares of traffic by transport modes calculated in 2009–2014 for the port of Szczecin were used to forecast the cargo transportation by means of port hinterland transport. These forecasts were stated according to the formula:
where
—forecast of traffic by m-th mode of hinterland transport in t-th year;
—forecast of total freight flow in the port in t-th year; and
—the average share of traffic by m-th mode of hinterland transport in 2009–2014.
Forecasted freight flow by means of hinterland transport in port of Szczecin is presented in .
Table 3. Forecasted freight flow by means of hinterland transport in port of Szczecin.
The forecasted truck traffic in relation with Szczecin port was obtained according to the formula:
where
—the traffic flow of the m-th truck type in year t;
—forecasted transshipments in j-th cargo group in year t;
—load capacity of the m-th truck type; and
n—the number of cargo groups in the port transshipment.
The structure of truck traffic at the port entry gates was used to forecast the number of trucks on the roads that lead directly to the reloading areas. Such forecasts were obtained according to the formula:
where
—the truck traffic flow in year t at the i-th entry gate/road leading to reloading areas;
—the truck traffic flow in year t on roads leading to reloading areas; and
—share of truck traffic flow at the i-th entry gate to road leading to reloading areas.
The volume of freight flow on the roads leading directly to the port’s reloading areas was calculated with the formula:
where
—volume of truck freight flow in year t at the i-th entry gate/road leading to reloading areas;
—volume of truck freight flow in year t along road sections leading directly to reloading areas; and
—share of truck traffic flow at the i-th entry gate to the road leading to reloading areas.
Calculations of projected number of trucks and the volume of freight flow on the selected roads leading directly to the port’s reloading areas are exemplified in .
Table 4. Forecasted number of trucks and freight flows (tonnes) on selected roads leading directly to reloading areas of port of Szczecin.
5. Forecasted truck traffic volume in the urban transport subsystem
To predict demand for capacity, the classical transport forecasting four-step models including trip generation, trip distribution, mode choice, and traffic assignment are in use. The fourth step, the traffic assignment, can be accomplished by several models that simulate route and mode choice in a network. The equilibrium principle makes available methods from economics for evaluating the potential benefits that accrue to travellers following a change in travel cost and transit time, while the latter are the key components of all demand models. In Static Traffic Assignment (STA), the models consider the dependence between the traffic on the roads and its generalized travel cost. Moreover, such models compute the flow of a section and the generalized travel cost simultaneously, what makes them consistent (Chiu et al. Citation2011). Adoption of the equilibrium algorithms requires the recalculation of travel times and the iteration of flow assignments. The main behavioural assumptions are that each traveller knows all the information about the characteristics of network alternatives, all travellers decide to choose such routes that minimize their travel cost or time, and finally, all travellers that belong to the same category assign the same values to each network attribute.
Among vast variety of methods for traffic assignment (or methods that are used for calculating the traffic load on the entire network), one may distinguish non-simulating (e.g., all-or-nothing, stochastic multi-flow or equilibrium models) and simulating methods, the latter may be extended on capacity constraints (congestion). The freight transportation traffic assignment models usually opt for all-or-nothing or equilibrium techniques.
European multimodal assignment model (NODUS) for freight transport (de Jong et al. Citation2013) uses the most common assignment techniques such as all-or-nothing, stochastic approach (multi-flow), and equilibrium techniques (Jourquin Citation2005). An all-or-nothing technique is considered as the easiest technique. That technique works by minimizing the total generalized transport costs in each O/D pair trip (least cost path), thus minimizing costs for the entire network. It is best suited for situations where another assignment techniques are considered too complex or just not fit for the approach proposed (Jourquin and Limbourg Citation2006).
In the study (Couto Maia and Fidalgo Do Couto Citation2013), cargo traffic assignment model for multimodal networks considers both rail and road transport modes. Assignment techniques for two kinds of cargos (general and intermodal cargo) are applied with the use of equilibrium with capacity constraints and under assumption of changeable perception of costs by users. The attributes of the network links are used to calculate the generalized transport cost and, if applicable, the traffic capacity of the linkage. The percentage of traffic that uses specific transport mode derives from the stochastic distribution that is estimated using a logit function. The assignment technique used allocates the traffic to the least costly path between the origin and the destination, allowing for the connection of all possible transportation modes.
Dynamic traffic assignment (DTA) is a modelling approach that identifies the relationship between dynamic route choice behaviours (start time and path) and transport network features. The capability of the modelling method for recognizing the spatial and temporal effects of congestion that have an impact on determining route choice, time of departure choice, and mode choice is the major benefit of DTA applications (Sloboden, Lewis, and Alexiadis Citation2012).
DTA models have a wide range of applications mostly in passenger transport. Moreover, they are suitable for such purposes as real-time traffic management, analyses involving incidents, construction zones, intelligent transportation systems, active transportation and demand management, integrated corridor management, and capacity increasing strategies, as well as other operational strategies (Szeto, and Wong Citation2012).
Finally, this class of models have the unique ability to recognizing complex and dynamic interactions between demand and supply in the case of emergency evacuations. With the use of DTA models, research of this complex problem is elaborated (e.g., Ng and Waller Citation2010,; Ng and Waller Citation2012; Ng and Waller Citation2010), and these studies are still advancing.
When analysing relationship between road freight transport demand and economic activity variables like Gross Domestic Product, Gross Final Expenditure, index of industrial production, and commodity sectors are under investigations (Dunkerley, Rohr, and Daly Citation2014).
In the context of cost-benefit analysis conducted by the Authors, the freight road traffic forecasts are associated with the growth of GDP. Predictions are made for truck flow expressed by trucks per day passing by selected road segments in the urban–port road system.
AADT on road sections was the basis for a long-term forecasts of AADTs up to the year 2043. Forecasts of AADT were elaborated in the reference period for any road segment separately as follows (Bernacki and Lis Citation2017):
where:
AADTki,t—annual average daily traffic for k-th category of vehicle in year t;
GDPt,GDPt+1—Gross Domestic Product in year t and t + 1 in constant prices from base period, wherein means a relative increase of GDP in year t + 1 compared to year t (statistical chain rate of changes in time); and
Ek (GDP)—elasticity coefficient for k-th vehicle category.
The annual average truck traffic forecast (AAT) was made as below:
where
AADTi,t—annual average daily truck traffic; traffic flow on the i-th road section in year t of forecast;
Elasticities of freight transport demand in the UK based on relative changes of aggregate commodity sector were in the range from 0.72 to 1.49 (Shen et al. Citation2009), while in another study (Agnolucci and Bonilla Citation2009), elasticity of freight road demand based on GDP was estimated on 0.66. In the research (Meersman and Van de Voorde Citation2013), the elasticity of road haulage with respect to relative changes of GDP (for 11 EU countries in 1970–2010) amounted to between 1.0 and 1.2. As recommended by JaspersFootnote1 (Blue Book Citation2015), the elasticity coefficient of GDP for trucks with trailers was used when predicting truck traffic in the urban transport subsystem. It amounted to 1.00 for Poland.
The long-term prediction of truck traffic was elaborated for every year in the forecasting period of 2020–2043 and for each in the urban transport subsystem. Results for the predictions of truck traffic flow in form of Excel spreadsheet are available on request.
6. Impact of capacity expansion in the urban–port transport system on haulage traffic performance
The analysis was carried out with the use of the differential method by estimating the net difference in transport effects that appear in the two options: with the reconstruction and improvement of the urban–port transport system (hereafter WI) and without the rebuilding and improvement of the urban–port transport system (hereafter W0), i.e., in the incremental calculus (WI-W0). This approach is widely recognized in assessing the impacts of infrastructure projects and of large-scale transport projects (e.g., Dekker, Verhaeghe, and Pols Citation2003; Van Exel et al. Citation2002; De Langhe, Van de Voorde, and Vaneslander Citation2012). The conditions for satisfying the demand for heavy road traffic in the option without intervention and in the option with intervention were quantified and compared and then the differences in traffic and transport performance were accounted.
In this research, a microeconomic approach was applied where the effects are measured directly in the transport system, namely the internal or direct impacts of infrastructural improvements (Lakshmanan and William Citation2002; Mackie, Graham, and Laird Citation2013).
The speed–flow engineering relationships are often used in analysing road traffic flows (e.g., Rothengatter Citation2000; Juhasz, Kosen, and Matrai Citation2016; Leong et al. Citation2020).
As from traffic speed-flow, the average speed of vehicles on urban roads in a good technical condition is 35.4 km/h, while on roads with a high grade of technical wear and tear, the average truck speed estimate is 15.58 km/h. From the comparison of both speeds, the truck speed correction indices depending on the degree of technical road wear and tear were calculated and are shown in .
Table 5. Truck speed correction indices depending on technical road wear and tear.
The truck speed correction indices according to the degree of technical wear and tear were applied on roads and sections of road constituting the urban–port transport system.
The average truck velocity that is determined in the traffic flow tables for the WI and W0 options varies depending on the type of the road and the change in the volume of traffic flow in each year of the analysis. Here, the average truck speeds for any road segment in the port–urban transport system in options WI and W0 are multiplied by the speed correction indices selected depending on the degree of technical road wear and tear in each option.
Incremental accounts (WI-W0) were performed for the following truck traffic performance: in-service work (vehicle-kilometres, v-km), truck trip time (vehicle-hours, v-hs), truck drivers’ working time (working hours, w-hs), road transport time (tonnes-hours, t-hs), and CO2 emissions from trucks (tonnes of emitted CO2, tCO2).
The total in-service work performed by trucks in the urban–port transport system was expressed in vehicle-kilometres (v-km) and calculated according to the formula:
where
—total in-service work in the urban–port transport system performed by trucks in year t of forecast (v-km);
—average annual truck traffic flow on the i-th road section in year t of forecast;
—length of the i-th road in the urban–port transport system: and
n—the number of roads and road sections in the transport system.
The total truck trip time and total truck drivers’ working time (with the assumption that 1 truck = 1 driver (Blue Book Citation2015)) were calculated according to the formulas:
Regarding truck trip time and truck drivers’ working time in WI option:
where
—total truck trip time and total truck drivers’ working time in the urban–port transport system in year t of forecast (v-hs; w-hs);
—truck traffic flow on the i-th road section in year t of forecast;
—length of the i-th road in the urban-port transport system; and
—average speed of trucks established using the traffic flow table for roads in good technical condition.
Regarding truck trip time and truck drivers’ working time in the option W0:
where
—total truck trip time and total truck drivers’ working time in the urban–port transport system in year t of forecast (v-hs; w-hs); and
—truck speed reduction rate due to degree of road wear and tear.
The total time of freight road transport in the urban–port transport system was calculated in option WI and W0, respectively, according to the formulas
where
—total time of freight road transport in the urban–port transport system in year t of forecast in option W0 (t-hs);
—total time of freight road transport in the urban–port transport system in year t of forecast in option WI (t-hs); and
—freight traffic volume on the i-th road section in year t.
The CO2 emissions from heavy goods vehicles (in this case they include vehicles with the gross weight >3,5 tons) were calculated with the use of emission factors: tCO2/v-km for repaired/constructed road surfaces (WI) and for degraded surfaces (W0) on flat terrain.
The two total CO2 emissions in tonnes (tCO2) were calculated according to formulas
where
—total CO2 emission on the i-th road in year t in option WI (tCO2);
—total CO2 emission on the i-th road in year t in option W0 (tCO2);
—unit emission factors of climate change—tCO2/v-km, flat terrain (reconstructed/ constructed road surface) for the i-th road; and
—unit emission factors of climate change—tCO2/v-km, flat terrain (degraded road surface) for the i-th road.
The obtained results are listed in the .
Table 6. Accumulated (in 2024–2043) transport effects resulting from capacity expansion in the road urban-port system.
The investment project involves a reconstruction of the existing urban–port transport system, so the lengths of roads remain unchanged. The projected in-service operation of trucks in the port-city transport system will grow at the same rate for both the W0 and WI options, thus, in the differential variant, the in-service operation of trucks will be 0.
The improvement of the technical condition of roads will increase the speed of heavy goods vehicles and simultaneously reduce vehicle manoeuvres. This results in a reduction in truck drivers’ working time and trucks’ trip time. Savings in the transit time of trucks and the working time of truck drivers are the same in quantitative terms (i.e., 2,777,287 units) because of the assumption that the number of trucks and the number of drivers is the same (1 truck = 1 driver). However, the units of measurement of these features are different. Units are, respectively, expressed as vehicle-kilometres and the working hours of truck drivers. The monetary values of vehicle-kilometres and the working hours of drivers vary, and this is reflected in the reduced vehicle operating and labour costs incurred by road hauliers.
As a result of the increase in truck average velocity and smoother traffic flows, trucks’ transit time will be reduced (during 2024–2043 by −26,665,909 tonne-hours), and CO2 emissions will also be reduced (by a total of −31,599 tonnes).
7. Lessons to be learnt
The relevance of network/zone division and the network partition and hence its size (demand) is a crucial problem of study on road traffic evolution. Moreover, partition of network is a subject of study aims and characteristics of investigated markets. There are several consequences of network partition throughout the entire steps of modelling, as amongst others, it affects traffic assignment methods applied and thereafter credibility of simulated results. Due to the small size or large area and inequality of demand partition, traffic assignments algorithms may produce different results, while some of methods are suitable only for small- and medium-sized networks (Rojo Citation2020; Ameli, Lebacque, and Leclercq Citation2020). When investigating port-related traffic problems, network recognition has some additional severe consequences. If port hinterland means the inland area that surrounds a port from which the goods are either distributed or at which they are gathered for shipping to other ports (Kramberger et al. Citation2015), one may distinguish (1) captive hinterlands which consist of all regions where one port has a substantial competitive advantage because of lower generalized transport costs to these regions and (2) contestable hinterlands which consist of all regions where no single port has a clear cost advantage over other ports in the range (De Langen Citation2007).
In the former, port alone handles most of all types of cargo to/from these regions, while in the latter, traffic may be distributed amongst many ports subject of minimising freight transport cost and transit time. Then, the common approach for modelling freight transport demand are equilibrium traffic assignment models that simulate route and mode choice as an interplay between cost and transit time, and these models are of crucial importance in the analysis. However, if different types of hinterlands systems (captive, contestable markets) and their modal structure (haulage, rail, inland waterways) are not sufficiently recognized, traffic assignment models may generate seemingly inconsistent findings, which could cause confusion for policymakers and practitioners (Wan, Zhang, and Li Citation2018).
Except for freight traffic assignment models for fictional networks, most of the studies focus on specific real networks, with several assumptions made as to market division, time/cost trade-offs, and behavioural patterns of traffic users, also with their perceived attribution for route choice. In this respect, there are at least two issues to be considered. First, most of the studies on traffic modelling are context-wise and case study-oriented, what confirms there does not exist universal method that is suitable for every situation better than for the rest ones (van Lint and Hinsbergen Citation2012). Second, in the study on further advances in freight transport modelling, it is underlined that some traffic simulations can lead to unreliable, uncertain, or even nonsense results if the model does not include a proper description of reality, if it is based on a faulty initial position, if unrealistic and even impractical assumptions have been made, or if uncertainties are strongly neglected. (Meersman, and Van de Voorde Citation2019).
Traditional four-step freight traffic modelling uses data from travel surveys to calibrate demand models and forecast future demand in the network. In the study (Sys and Vanelslander Citation2018), the case of contestable hinterland, multimodal aggregated model considering both passenger and freight traffic was investigated. The future cargo flows by transport mode such as road, rail, and inland waterways, and freight types in respect of forecasted port throughput were tested in scenarios with and without the construction of the motorway. In principle, our study uses similar method and research concept, but it concerns small network, specifically port captive hinterland and road freight traffic. While in both studies traffic assignments in the network are not simulated, the conclusions of both types of research are consistent. The benefits of intervention in the road infrastructure are largely attributable to traffic time gains induced by higher average speed and mitigation of congestion.
With clear market demarcation and network partition also with the use of surveys of traffic flow performance, discrete choice modelling computing the mode splits and route choice can be replaced by the direct traffic assignment using revealed modal structure of port hinterland transport, while the route choice can be determined using historical proportions of freight traffic flows through the port gates.
Traffic surveys in the road network give reliable data concerning the traffic flow, volume, and structure of traffic and provide a sound basis for predictions of traffic demand for each road, especially when the network is local and limited to roads converging in the port. Forecasting demand for road infrastructure capacity can be estimated for each road and derived from regressions or category analysis, with the use of socio-economic growth as independent variables. This can be an alternative to the road traffic assignment models through simulations.
We analysed traffic demand and congestion properties for homogeneous traffic (trucks) and for each road with the use of a volume–speed traffic flow curves derived from engineering relationships. These curves express the average vehicle speed and thereafter travel time on a road as a function of the traffic intensity on the link and its capacity. Thus, the capacity constraints are already included in the accounts through the variation in vehicles velocity.
The travel times on each road are added together to determine aggregated travel time in the network, and by comparing travel time in the scenario with and without investment, aggregated travel time gains (savings) are obtained, and congestion release effect is captured for the whole network. It means the capacity constraints in form of incremental travel time savings is an outcome of road capacity improvement and forecasted development of traffic flow, and these relations are obtained for each analysed road in the network.
The research is aimed at evaluating the potential benefits that accrue to users following a change in the travel conditions due to implementation of transportation project. The local network structure remains unchanged, and O-D is limited to one pair: main collecting road–port, while the roads are streamlined to specific port areas. At the approach to port, the route choice is a truck’s driver decision based on driver-gained experience while the cost/time of travel is already internalized in travel itineraries. In such situations, traffic assignment simulations searching for the equilibrium-based traffic route choice are of minor relevance (Ortúzar and Willumsen Citation2011).
8. Conclusions and further research
The direct effects identified in connection with the intervention in the urban-port road transport system include savings in the following:
the time of trucks′ trips (in vehicle-hours), drivers’ working time (in working hours), and the time of transporting goods (in tonne-hours) by 43.3%, and
the trucks’ CO2 emissions (in tonnes of CO2) by 29.5%.
The final beneficiaries of the capacity improvements in the urban–port transport system will be the following:
haulage operators who will benefit from the time reduction of trucks′ operations and drivers’ working time which, economically, will lead to lower operating costs,
shippers, exporters, and importers—because of faster freight transport, the effectiveness of the international trade handled by the port of Szczecin will increase, and
the local community—thanks to the decreased transport-related environmental externalities through the reduced amounts of CO2 emitted by trucks.
Therefore, the above-described infrastructural project will reduce traffic congestion, translating into an increased speed of heavy vehicles. Consequently, the vehicle travel time and time of transporting goods in the city–port transport system will be reduced, which renders it possible to offer transport services that are cheaper, more reliable, and sustainable.
Limitations of this study result from its assumptions and the prognostic data used. When forecasting port transshipment and truck traffic, a historical relationship between port transshipment and GDP had been used. Nevertheless, in the future, these relationships may change with unknown magnitude and direction. Moreover, the Polish GDP forecast used is also debatable considering the recent global Covid-19 pandemic. Furthermore, projected port cargo volumes and truck traffic forecasts may be affected by future unpredictable structural, social, political, and economic changes and shocks. Therefore, the question of reliability of forecasts elaborated remains valid.
Additionally, the impact of road capacity expansion on congestion is limited to its direct effects on the road transportation system. Also, the relations between capacity expansion and induced traffic in the urban–port transport system and network traffic flow effects are not specified in the study.
We have elaborated the technique to predict the traffic flow of road freight in the local captive port market. It means that the problems such as (1) transport network traffic assignments in the contestable and distant inland markets, the inter-port competition, and port competitiveness, (2) strategic effects of the improved hinterland accessibility on port users′ choice, welfare shifts between local economies, and the performance of competing ports are beyond the study.
Regarding capacity expansion, further research should focus on induced road traffic in the urban–port transport system and on capturing traffic network effects within the road system as well as the indirect (wider) benefits of capacity expansion outside the transportation system (induced economic growth, spatial spillovers, and agglomeration economies). For traffic and port transshipment forecasting, future studies could include probabilistic forecasting solutions and sensitive analysis. Lastly, other directions could continue research on the relationship between road congestion and port throughput and the impacts of capacity expansion on other major players (shipping companies, port authorities, terminal operators) as well as on the interaction between road congestion and port competition.
Acknowledgments
The authors would like to thank three anonymous reviewers and assistant editor for their insightful suggestions and recommendations. However, the usual disclaimers apply.
Disclosure statement
No potential conflict of interest was reported by the author(s).
Additional information
Funding
Notes
1. Joint Assistance to Support Projects in European Regions (Jaspers) is an initiative operating within the structures of the European Investment Bank and aimed at improving the preparation of projects, including transport projects, by applying for EU funding.
References
- Agnolucci, P., and D. Bonilla. 2009. “UK Freight Demand Elasticities and Decoupling.” Journal of Transport Economic and Policy 43 (3): 317–344.
- Ameli, M., J. Lebacque, and L. Leclercq. 2020. “Cross-comparison of Convergence Algorithms to Solve Trip-based Dynamic Traffic Assignment Problems.” Computer-aided Civil and Infrastructure Engineering 35: 219–240. doi:10.1111/mice.12524.
- Aronietis, R., E. Van de Voorde, and T. Vanelslander. 2010. “Port Competitiveness Determinants of Selected European Ports in the Containerized Cargo Market”. Proceedings of IAME 2010 Conference. Lisbon.
- Bernacki, D., and C. Lis. 2017. “Statistical Estimation and Prediction of Annual Average Daily Traffic (AADT) on the First /Last Mile Road Sections in the Port of Szczecin.” Szczecin University Press. Economics Problems of Services 3 (128): 67–80. doi:10.18276/epu.2017.128-05.
- Book, B. 2015. “Road Infrastructure.” Warsaw: Joint Assistance to Support Projects in European Regions (Jaspers)
- Chen, S. H., and J. N. Chen. 2010. “Forecasting Container Throughput at Ports Using Genetic Programming.” Expert Systems with Applications 37: 2054–2058. doi:10.1016/j.eswa.2009.06.054.
- Chiu, Y. C., J. Bottom, M. Mahut, A. Paz, R. Balakrishna, T. Waller, and J. Hicks. 2011. “Dynamic Traffic Assignment A Primer.” Transportation Research E-Circular C153 (6): 14–16.
- Chou, C. C., C. W. Chu, and G. S. Liang. 2008. “A Modified Regression Model for Forecasting the Volumes of Taiwan’s Import Containers.” Mathematical and Computer Modelling 47: 797–807. doi:10.1016/j.mcm.2007.05.005.
- Couto Maia, L., and A. Fidalgo Do Couto. 2013. “An Innovative Freight Traffic Assignment Model for Multimodal Networks.” Computers in Industry 64 (2): 121–127. doi:10.1016/j.compind.2012.10.011.
- De Borger, B., S. Proost, and K. Van Dender. 2005. “Congestion and Tax Competition in a Parallel Network.” European Economic Review 49 (8): 2013–2040. doi:10.1016/j.euroecorev.2004.06.005.
- De Borger, B., S. Proost, and K. Van Dender. 2008. “Private Port Pricing and Public Investment in Port and Hinterland Capacity.” Journal of Transport Economics and Policy 42 (3): 527–561.
- de Jong, G., I. Vierth, L. Tavasszy, and M. Ben-Akiva. 2013. “Recent Developments in National and International Freight Transport Models within Europe.” Transportation 40: 347–371. doi:10.1007/s11116-012-9422-9.
- De Langen, P. W. 2007. “Port Competition and Selection in Contestable Hinterlands: The Case of Austria.” European Journal of Transport and Infrastructure Research 7 (1): 1–14.
- De Langen, P. W., J. van Meijeren, and L. A. Tavasszy. 2012. “Combining Models and Commodity Chain Research for Making Long-term Projections of Port Throughput: An Application to the Hamburg-Le Havre Range.” European Journal of Transport and Infrastructure Research 12 (3): 310–331. doi:10.18757/ejtir.2012.12.3.2968.
- De Langhe, K., Ch. Sys, E. Van de Voorde, and T. Vaneslander. 2012. “Analysis of a Cost-benefit Study: Lessons to Be Learned for Large Investment Projects in Port Areas”. Association for European Transport. European Transport Conference Glasgow, Scotland.
- Dekker, S., R. J. Verhaeghe, and A. A. J. Pols. 2003. “Economic Impacts and Public Financing of Port Capacity Investments: The Case of Rotterdam Port Expansion.” Transportation Research Record: Journal of the Transportation Research Board 1820: 55–61. doi:10.3141/1820-07.
- Dunkerley, F., C. Rohr, and A. Daly. 2014. “Road Traffic Demand Elasticities. A Rapid Evidence Assessment”. Rand Europe. Final report.
- Feng, L., and T. Notteboom. 2011. ““Small and Medium-Sized Ports (Smps) in Multi-Port Gateway Regions: The Role of Yingkou in the Logistics System of the Bohai Sea”.” In Current Issues in Shipping, Ports and Logistics, edited by T. Notteboom, 543–563. Antwerp: University Press Antwerp.
- Feng, L., and T. Notteboom. 2013. “Peripheral Challenge by Small and Medium Sized Ports (Smps) in Multi-Port Gateway Regions: The Case Study of Northeast China.” Polish Maritime Research. Special Issue 20: 55–66. doi:10.2478/pomr-2013-0027.
- FHWA. 2004. Traffic Congestion and Reliability: Linking Solutions to Problems. the United States: Federal Highway Administration (FHWA).
- Fung, M. K. 2002. “Forecasting Hong Kong′s Container Throughput: An Error-correction Model.” Journal of Forecasting 21 (1): 69–80. doi:10.1002/for.818.
- Giuliano, G., and T. O′ Brien. 2007. “Reducing Port-Related Truck Emissions: The Terminal Gate Appointment System at the Ports of Los Angeles and Long Beach.” Transportation Research Part D 12 (7): 460–473. doi:10.1016/j.trd.2007.06.004.
- Giuliano, G., and T. O′ Brien. 2008. “Extended Gate Operations at the Ports of Los Angeles and Long Beach: A Preliminary Assessment.” Maritime Policy & Management 35 (2): 215–235. doi:10.1080/03088830801956854.
- Hall, P. 2018. “Traffic Planning in Port-Cities.” Discussion paper. International Transport Forum. Paris.
- Hanaoka, S., and M. B. Regmi. 2011. “Promoting Intermodal Freight Transport through the Development of Dry Ports in Asia: An Environmental Perspective.” IATSS Research 35: 16–23. doi:10.1016/j.iatssr.2011.06.001.
- Hayuth, Y., and D. F. Fleming. 1994. “Concepts of Strategic Commercial Location: The Case of Container Ports.” Maritime Policy & Management 21: 187–193. doi:10.1080/03088839400000042.
- Hui, E. C. M., W. Seabrooke, and G. K. C. Wong. 2004. “Forecasting Cargo Throughput for the Port of Hong Kong. Error Correction Model Approach.” Journal of Urban Planning and Development 130 (4): 195–203. doi:10.1061/(ASCE)0733-9488(2004)130:4(195).
- “Instruction for Assessing the Economic Efficiency of Road and Bridge Projects – Verification of the Research Method according to EU Recommendations and Update of Unit Prices as of 2007. Part II, Tables of Speed”.2008.Warsaw: Instytut Badawczy Dróg i Mostów. (in Polish) 84–86
- Jarrett, J. E. 2015. “Throughput Port Demand Forecasting.” International Journal of Economics & Management Sciences 4: 1–4. doi:10.4172/2162-6359.1000293.
- Jourquin, B. 2005. “A Multi-flow Multi-modal Assignment Procedure Applied to the European Freight Transportation Networks.” Studies in Regional Science 35: 929–945. doi:10.2457/srs.35.929.
- Jourquin, B., and S. Limbourg. 2006. “Equilibrium Traffic Assignment on Large Virtual Networks: Implementation Issues and Limits for Multi-modal Freight Transport.” European Journal of Transport and Infrastructure Research 6 (3): 205–228.
- Juhasz, M., C. Kosen, and T. Matrai. 2016. “Analysing the Speed-flow Relationship in Urban Road Traffic.” Acta Technica Jaurinensis 9: 128. doi:10.14513/actatechjaur.v9.n2.403.
- Kitamura, R. 2010. “The Effects of Added Transportation Capacity on Travel: A Review of Theoretical and Empirical Results.” Transportation 36 (6): 754–762. doi:10.1007/s11116-009-9245-5.
- Klein, A. 1996. “Forecasting the Antwerp Maritime Traffic Flows Using Transformations and Intervention Models.” Journal of Forecasting 15 (5): 395–412. doi:10.1002/(SICI)1099-131X(199609)15:5<395::AID-FOR628>3.0.CO;2-7.
- Korovyakovsky, E., and Y. Panova. 2011. “Dynamics of Russian Dry Ports.” Research in Transportation Economics 33: 25–34. doi:10.1016/j.retrec.2011.08.008.
- Kramberger, T., B. Rupnik, G. Štrubelj, and K. Prah. 2015. “Port Hinterland Modelling Based on Port Choice.” Promet – Traffic and Transportation 27 (3): 195–203. doi:10.7307/ptt.v27i3.1611.
- Lakshmanan, T. R., and P. William. 2002. Transportation Infrastructure, Freight Services Sector and Economic Growth, 43–44. Washington D.C: The U.S. Department of Transportation, Federal Highway Administration. http://www.ncgia.ucsb.edu/stella/meetings/20020115/Lakshmanan.pdf.
- Lättilä, L., V. Hentuu, and O.-P. Hilmola. 2013. “Hinterland Operations of Seaports Do Matter: Dry Port Usage Effects on Transportation Costs and CO2 Emissions.” Transport Research Part E: Logistics and Transportation Review 55: 23–42. doi:10.1016/j.tre.2013.03.007.
- Leong, L. V., T. A. Azai, W. C. Goh, and M. Belly Mandi. 2020. “The Development and Assessment of Free-flow Speed Models under Heterogeneous Traffic in Facilitating Sustainable Inter Urban Multilane Highways.” Sustainability 12: 1–17. doi:10.3390/su12083445.
- Litman, T. 2007. Congestion Reduction Strategies: Identifying and Evaluating Strategies to Reduce Traffic Congestion. Victoria, BC, Canada: Victoria Transport Policy Institute.
- Litman, T. 2016. Smart Congestion Relief: Comprehensive Analysis of Traffic Congestion Costs and Congestion Reduction Benefits. Victoria, BC, Canada: Victoria Transport Policy Institute.
- Mackie, P., D. Graham, and J. Laird. 2013. “The Direct and Wider Impacts of Transport Projects: A Review.” In A Handbook of Transport Economics, edited by A. D. Palma, R. Lindsey, E. Quinet, and R. Vickerman, 501–527. UK: Edward Elgar Publishing.
- Martinez Moya, J., and M. F. Valero. 2017. “Port Choice in Container Market: A Literature Review.” Transport Reviews 37 (3): 300–321. doi:10.1080/01441647.2016.1231233.
- Matczak, M. 2020. “A Simplified Forecasting Model for the Estimation of Container Traffic in Seaports at National Level-the Case of Poland.” The International Journal on Marine Navigation and Safety of Sea Transportation 14 (1): 153–158. doi:10.1016/j.tra.2018.08.010.
- Meersman, H., F. Moglia, and E. Van de Voorde. 2002. “Forecasting Potential Throughput.” In Port Competitiveness. An Economic and Legal Analysis of the Factors Determining the Competitiveness of Seaports, edited by W, 35–64. Antwerp: Winkelmans, De Boeck Publishing.
- Meersman, H., and E. Van de Voorde. 2013. “The Relationship between Economic Activity and Freight Transport.” In Developments in Transport Modelling. Lessons for the Freight Sector, edited by M. B. Akiva, H. Meersman, and E. Van de, 15–43. Vol. Voorde. UK: Emerald Publishing.
- Meersman, H. , and E. Van de Voorde. 2019. ““Freight Transport Models: Ready to Support Transport Policy of the Future? “.” Transport Policy 83: 97–101. doi:10.1016/j.tranpol.2019.01.014.
- Merk, O., and T. Dang. 2013. “The Effectiveness of Port-City Policies: A Comparative Approach.” OECD Regional Development Working Papers 2013-25. doi:10.1787/5K3TTG8ZN1ZT-EN
- Merk, O., and T. Notteboom. 2015. “Port Hinterland Connectivity.” Discussion Paper no 2015-13, International Transport Forum, OECD Publishing.
- “Method of Carrying Out the General Traffic Measurement in 2015”.2014 (GTM 2015 Guidelines, attachment B), the General Directorate for National Roads and Motorways, Warsaw.
- Muravev, D., H. Hu, A. Rakhmangulov, and P. Mishkurov. 2020. “Multi-agent Optimization of the Intermodal Terminal Main Parameters by Using AnyLogic Simulation Platform: Case Study on the Ningbo-Zhoushan Port.” International Journal of Information Management 57. doi:10.1016/j.ijinfongt.2020.102133.
- Muravev, D., A. Rakhamngulov, H. Hu, and H. Zhou. 2019. “The Introduction to System Dynamics Approach to Operational Efficiency and Sustainability of Dry Port′s Main Parameters.” Sustainability 11 (8): 2413. doi:10.3390/su11082413.
- Muravev, D., and A. Rakhmangulov. 2016. “Environmental Factors′ Consideration at Industrial Transportation Organization in the Seaport-Dry Port System.” Open Engineering 6 (1): 476–484. doi:10.1515/eng-2016-0070.
- Naess, P., M. S. Nicolaisen, and A. Strand. 2012. “Traffic Forecasts Ignoring Induced Demand: A Shaky Fundament for Costs-benefit Analyses.” European Journal of Transport and Infrastructure Research 12 (3). doi:10.18757/ejtir.2012.12.3.2967.
- Ng, M., and S. T. Waller. 2010 . “Reliable Evacuation Planning via Demand Inflation and Supply Deflation.” Transportation Research Part E: Logistics and Transportation Review 46 (6): 1086–1094. doi:10.1016/j.tre.2010.04.001.
- Ng, M., and S. T. Waller. 2012. “A Dynamic Route Choice Model considering Uncertain Capacities.” Computer-Aided Civil and Infrastructure Engineering 27 (4): 231–243. doi:10.1111/j.1467-8667.2011.00724.x.
- Notteboom, T. 2005. “The Peripheral Port Challenge in Container Port Systems.” In International Maritime Transport: Perspectives, edited by H. Leggate, J. Mcconville, and A. Morvillo, 173–188. London: Routledge.
- Notteboom, T., and W. Yim Yap. 2012. “Port Competition and Competitiveness.” In The Blackwell Companion to Maritime Economics, edited by K. Wayne and W. Talley, 549–570, Blackwell Publishing. USA and UK.
- OECD. 2013. “The Competitiveness of Global Port-Cities: Synthesis Report”. OECD Port-Cities Programme. Rotterdam.
- Ortúzar, J. D., and L. G. Willumsen. 2011. Modelling Transport. Hoboken, NJ, USA: Wiley & Sons.
- Parola, F., M. Risitano, M. Ferretti, and E. Panetti. 2016. “The Drivers of Port Competitiveness: A Critical Review.” Transport Reviews 37. doi:10.1080/01441647.2016.1231232.
- Rojo, M. 2020. “Evaluation of Traffic Assignment Models through Simulations.” Sustainability 12 (5536): 5536. doi:10.3390/su12145536.
- Roso, V. 2007. “Evaluation of the Dry Port Concept from an Environmental Perspective: A Note.” Transportation Research Part D: Transport and Environment 12: 523–527. doi:10.1016/j.trd.2007.07.001.
- Roso, V. 2009. “The Emergence and Significance of Dry Ports: The Case of the Port of Geteborg.” World Review of Intermodal Transportation Research 2: 296–310. doi:10.1504/WRITR.2009.026209.
- Roso, V. 2013. “Sustainable Intermodal Transport via Dry Ports: Importance of Directional Development.” World Review of Intermodal Transportation Research 4: 140–156. doi:10.1504/WRITR.2013.058976.
- Roso, V., and K. Lumsden. 2010. “A Review of Dry Ports.” Maritime Economics and Logistics 12: 196–213. doi:10.1057/mel.2010.5.
- Roso, V., J. Woxenius, and K. Lumsden. 2009. “The Dry Port Concept: Connecting Container Seaports with the Hinterland.” Journal of Transport Geography 17: 338–345. doi:10.1016/j.jtrangeo.2008.10.008.
- Rothengatter, W. 2000. “Evaluation of Infrastructure Investments in Germany.” Transport Policy 7 (1): 17–23. doi:10.1016/S0967-070X(00)00012-3.
- Shen, S., T. Fowkes, T. Whiteing, and D. Johnson. “Econometric Modelling and Forecasting of Freight Transport Demand in Great Britain”. In Proceedings of the European Transport Conference, Noordwijkerhout, The Netherlands, 5–7 October 2009.
- Slack, B., and J. J. Wang. 2002. “The Challenge of Peripheral Ports; an Asian Perspective.” Geojournal 56 (2): 159–166. doi:10.1023/A:1022452714114.
- Sloboden, J., J. Lewis, and V. Alexiadis. 2012. “Traffic Analysis Toolbox Volume XIV: Guidebook on the Utilization of Dynamic Traffic Assignment in Modelling”. Washington DC: U.S. Department of Transportation Federal Highway Administration Office of Operations.
- Sys, C., and T. Vanelslander. 2018. “Port Hinterland Relations: Lessons to Be Learned from a Cost-benefit Analysis of a Large Investment Project.” In “Ports and Networks. Strategies, Operations and Perspectives”, edited by H. Geerlings, B. Kuipers, and R. Zuidwijk. Routledge. 146–161.
- Szeto, W. Y., and S. C. Wong. 2012. “Dynamic Traffic Assignment: Model Classifications and Recent Advances in Travel Choice Principles.” Central European Journal of Engineering 2 (1): 1–18. doi:10.2478/s13531-011-0057-y.
- Thompson, R. G., and E. Taniguchi. 2008. “City Logistics and Freight Transport.” In Handbook of Logistics and Supply-Chain Management (Vol.2), edited by A. M. Brewer, K. J. Button, and D. A. Hensher, 393–405. UK: Emerald Publishing Group. doi:10.1108/9780080435930-025.
- Treintis, K., S. Sarangi, D. Teodorovic, and L. Rozzolini. 2011. “Traffic Congestion Mitigation: Combining Engineering and Economic Perspectives.” Transportation Planning and Technology 34 (7): 637–645. doi:10.1080/03081060.2011.602845.
- Van Dorsser, C., M. Wolters, and B. Wee Van. 2011. “A Very Long-term Forecast of the Port Throughput in the Le Havre-Hamburg Range up to 2100.” European Journal of Transport and Infrastructure Research 12 (1): 88–110.
- Van Exel, J., S. Rienstra, M. Gommers, A. Pearman, and D. Tsamboulas. 2002. “EU Involvement in TEN Developments: Network Effects and European Value Added.” Transport Policy 9 (4): 299–311. doi:10.1016/S0967-070X(02)00016-1.
- van Lint, H., and C. Hinsbergen. 2012. “Short-term and Travel Time Prediction Models.” Artificial Intelligence Applications to Critical Transportation Issues 22: 22–41.
- Veenstra, A. W., and E. H. Haralambides. 2001. “Multivariate Autoregressive Models for Forecasting Seaborn Trade Flows.” Transportation Research Part E 37 (4): 395–412. doi:10.1016/S1366-5545(00)00020-X.
- Wan, Y., L. J. Basso, and A. Zhang. 2016. “Strategic Investments in Accessibility under Port Competition and Inter-regional Coordination.” Transportation Research Part B: Methodological 93: 102–125. doi:10.1016/j.trb.2016.07.011.
- Wan, Y., C. L. Yuen, and A. Zhang. 2014. “Effects of Hinterland Accessibility on US Container Port Efficiency.” International Journal of Shipping and Transport Logistics 6 (4): 422–440. doi:10.1504/IJSTL.2014.062908.
- Wan, Y., and A. Zhang. 2013. “Urban Road Congestion and Seaport Competition.” Journal of Transport Economics and Policy 47 (1): 55–70.
- Wan, Y., A. Zhang, and K. X. Li. 2018. “Port Competition with Accessibility and Competition: A Theoretical Framework and Literature Review on Empirical Studies.” Maritime Policy &Management 45 (2): 239–259. doi:10.1080/03088839.2017.1403053.
- Wan, Y., A. Zhang, and C. L. Yuen. 2013. “Urban Road Congestion, Capacity Expansion and Port Competition: Empirical Analysis of US Container Ports.” Maritime Policy & Management 40 (5): 417–438. doi:10.1080/03088839.2013.797615.
- Wang, F. L. 2009. “Study on the Relationship of Huizhou Port and Huizhou Economy.” Journal of Huizhou University 29 (4): 8–10.
- Wang, C. J., J. Wang, and C. Ducret. 2012. “Peripheral Challenge in Container Port System: A Case Study of Pearl River Delta.” Chinese Geographical Science 22: 97–108. doi:10.1007/s11769-012-0517-1.
- Wilmsmeier, G., and J. Monios. 2013. “Counterbalancing Peripherality and Concentration: An Analysis of the UK Container Port System.” Maritime Policy & Management 40 (2): 116–132. doi:10.1080/03088839.2012.756588.
- Yuen, A., L. J. Basso, and A. Zhang. 2008. “Effects of Gateway Congestion Pricing on Optimal Road Pricing and Hinterland.” Journal of Transport Economics and Policy 42 (3): 495–526.
- Zhang, A. 2008. “The Impact of Hinterland Access Conditions on Rivalry between Ports.” OECD/ITF Discussion Paper 2008-8, Paris. doi: 10.1787/235411150338.