ABSTRACT
Background: Whether older adults use effective memory strategies to compensate for their memory decline partly depends on their executive functioning (EF). However, many studies have overlooked the role of cognitive reserve (CR). This study examines the effects of age, EF, and CR on memory strategy use.
Methods: A total of 83 participants (aged 18–85 years) were included. Strategy use was assessed using three measures: (1) self-reported strategy use in daily life, (2) self-reported and observed strategy use in a simulated daily life situation, and (3) self-reported strategy use during a word-pair task.
Results: Results showed that CR was the strongest predictor of strategy use, both in daily life and during memory tasks. Although effects of age and EF were found, most of these effects disappeared when CR was added to the model. Furthermore, a higher CR was related to the use of more complex strategies and to more effective strategies in relation to task performance.
Conclusions: Higher levels of CR seem to enable individuals to use effective strategies. These results highlight the importance of the role of CR in compensating for the aging-related memory decline.
Introduction
Episodic memory is known to decline with aging, although significant individual differences have been found (Nilsson, Citation2003). Several studies have demonstrated that this aging-related decline can in part be explained by deficient strategy use (Shing et al., Citation2010). The production deficiency hypothesis, for example, states that older adults have difficulties with producing memory strategies spontaneously (Kausler, Citation1994). Other studies have confirmed this hypothesis, by showing that older adults more often use ineffective strategies or use fewer strategies during encoding than younger adults (Dunlosky & Hertzog, Citation2001; Glisky, Rubin & Davidson, Citation2001; Kamp & Zimmer, Citation2015; Naveh-Benjamin, Brav & Levy, Citation2007; Seaman, Howard & Howard, Citation2015).
Spontaneous memory strategies can be divided into internal (e.g., rehearsal, visual imagery) and external (e.g., using a calendar, taking notes) strategies (Dixon & Hultsch, Citation1983). Older adults use relatively more external strategies than younger adults (Bouazzaoui et al., Citation2010; Dixon & Hultsch, Citation1983; Loewen, Shaw & Craik, Citation1990), presumably because the use of memory aids requires little cognitive control and can be easily used to compensate for aging-related memory decline (Lovelace & Twohig, Citation1990). Furthermore, there is evidence that the use of internal strategies decreases with age, both in daily life (Bouazzaoui et al., Citation2010; Dixon & Hultsch, Citation1983; Loewen et al., Citation1990) and during laboratory memory encoding tasks (Dunlosky & Hertzog, Citation2001; Glisky et al., Citation2001; Naveh-Benjamin et al., Citation2007). However, other studies did not find age effects on the production of internal strategies during memory tasks (Bailey, Dunlosky, & Hertzog, Citation2009; Dunlosky & Hertzog, Citation1998; Kuhlmann & Touron, Citation2012; Touron, Oransky, Meier & Hines, Citation2010) or in daily life (Ponds & Jolles, Citation1996).
The finding that older adults have particular difficulties with the use of internal memory strategies can in part be explained by the aging-related decline in EF (Bouazzaoui et al., Citation2010; Taconnat et al., Citation2006, Citation2009). The use of memory strategies relies on cognitive control processes that regulate memory functions by selecting, monitoring, and organizing information during encoding in order to improve memory formation (Blumenfeld & Ranganath, Citation2007). Previous studies have shown that EF is associated with internal strategy use (Bouazzaoui et al., Citation2010) and that it positively influences memory performance, specifically in older adults (Bouazzaoui et al., Citation2014; Hinault, Lemaire & Touron, Citation2016).
One crucial factor that has not been taken into account in the previously described studies on strategy use is the potential role of cognitive reserve (CR). CR is assumed to reduce the risk of cognitive decline associated with aging-related brain changes by fostering the use of compensatory cognitive processes (Stern, Citation2002, Citation2009). Adults with higher levels of CR are more likely to use other cognitive resources, such as memory strategies, to compensate for their memory decrements. Previous studies have shown that individuals with a higher CR use additional brain regions associated with better memory task performance (Nyberg et al., Citation2003; Speer & Soldan, Citation2015; Steffener, Reuben, Rakitin & Stern, Citation2011). Only a few studies have directly related CR to strategy use, showing that a higher CR is associated with more spontaneous and efficient strategy use in both younger and older adults (Barulli, Rakitin, Lemaire & Stern, Citation2013; Frankenmolen et al., Citation2017). A limitation of previous studies on strategy use and aging is that some only included participants with high CR levels, as younger participants are university students, and older adults are matched for education (Dunlosky & Hertzog, Citation1998, Dunlosky & Hertzog, Citation2001; Naveh-Benjamin et al., Citation2007). Other studies did not match the younger and older adults with respect to education, resulting in different CR levels between age groups or unknown CR levels, complicating the interpretation of group differences in the light of aging (Bailey et al., Citation2009; Kuhlmann & Touron, Citation2012; Seaman et al., Citation2015; Touron et al., Citation2010). Consequently, differences in CR levels may in part account for these contradicting previous results concerning strategy production deficits in older adults.
It is well-known that CR is associated with EF (Roldan-Tapia, Garcia, Canovas & Leon, Citation2012), suggesting a potential shared variance between EFs and CR regarding the ability to compensate for memory decrements. Bruno, Brown, Kapucu, Marmar, and Pomara (Citation2014), for example, showed that CR had a stronger effect on memory performance, well beyond the contribution of EFs. However, to date, it is unclear what the independent effects of CR and EF are on memory strategy use.
Therefore, the goal of the present study was to examine spontaneous strategy use across the adult lifespan and elucidate the precise role of age, EF, and CR on strategy use. To accomplish this, we selected adults across the entire lifespan with an equal distribution of CR levels. Moreover, previous studies generally examined strategy use either in daily life or during task performance and additionally did not distinguish between self-reported strategies and those that are truly employed during encoding. This latter distinction is crucial, as Saczynski, Rebok, Whitfield, and Plude (Citation2007) have shown that adults are often unaware of the strategies that they have used, suggesting that they may underestimate true strategy use. To overcome these limitations, in our study, we employed a strategy observation task (SOT) in addition to self-report measures of strategy use in daily life and during memory tasks.
Based on previous studies, external strategy use was expected to increase with age (Bouazzaoui et al., Citation2010), whereas no change for internal strategy use was expected (Dunlosky & Hertzog, Citation2001). Additionally, the number of strategies used is expected to relate to better memory task performance (Dunlosky & Hertzog, Citation1998; Hill, Allen & Gregory, Citation1990; Rankin, Karol & Tuten, Citation1984; Richardson, Citation1998; Rogers, Hertzog & Fisk, Citation2000). Furthermore, we expect both EF and CR to be positively related to internal strategy use, both in daily life and during memory encoding tasks (Barulli et al., Citation2013; Bouazzaoui et al., Citation2010), although we expect the effects of CR to be stronger than the effects of EF (Bruno et al., Citation2014; Giogkaraki, Michaelides & Constantinidou, Citation2013). Finally, previous studies have suggested that strategies requiring a deeper level of processing (e.g., imagery and sentence generation) are more effective for task performance than strategies such as rehearsal or associating an item to one’s own environment (Dunlosky & Hertzog, Citation1998; Hill et al., Citation1990). We will therefore examine whether the use of these deeper processed strategies, which require more cognitive control, are positively related to EF and CR or negatively to age.
In summary, the present study was intended to examine (1) the effect of age, EF, and CR on the number of strategies used; (2) the effect of age, EF, and CR on the use of specific strategies; and (3) the relation between strategy use and task performance.
Method
Participants
A total of 83 participants were included in this study. All participants were residents of the Netherlands, lived independently in the community, and were fluent in Dutch. Participants were recruited through advertisement and were screened over the telephone for exclusion criteria: severe psychiatric problems, neurological disorders, substance abuse, and the use of psychoactive medication. Additionally, participants were asked whether they had subjective memory complaints (e.g., whether they experienced that their memory was significantly worse than their peers, or whether they had considered seeking professional help for their memory complaints). Those with subjective memory complaints were not further included in this study. All participants voluntarily participated in this study.
Care was taken to include participants across the total adult lifespan, with an equal distribution of educational level and sex. The age distribution is shown in . The mean age of the participants was 47.9 years (range = 18–85; SD = 18.0), from whom 40 were men and 43 women. Education level was rated according to the International Standard Classification of Education (ISCED-2011; UNESCO Institute for Statistics, Citation2012). This classification system has a range from 1 (primary school) to 8 (doctoral degree). Within our study sample, the median education level of the participants was 3 (range = 1–7). IQ was estimated using the Dutch version (Schmand, Lindeboom, & van Harskamp, Citation1992) of the National Adult Reading Test (Nelson, Citation1982). The mean IQ estimation was 103.5 (SD = 17.1). To examine the equal distribution of these demographical variables across the adult lifespan, Spearman correlations were computed. No significant correlations were found between age and sex (r = −.07, p = .513), education level (r = −.18, p = .100), or IQ estimate (r = .16, p = .150). Sex was also not significantly related to education level (r = −.09, p = .438), or IQ estimate (r = .04, p = .730). These nonsignificant correlations support the equal distribution of these demographical variables. As expected, educational level was strongly related to IQ estimation (r = .58, p < .001).
Both IQ estimation and education level were used as proxy for CR (Barulli et al., Citation2013; Giogkaraki et al., Citation2013; Sakamoto et al., Citation2013). First, scores were transformed into Z-scores. Z-scores reflect the standardized difference of an individual’s performance level compared to the study sample’s mean. These scores are computed by calculating the difference between an individual score and the mean score of the entire study sample, and dividing that difference by the standard deviation of the entire study sample. The resulting Z-score indicates how many standard deviations a single participant’s score deviates from the sample mean. Subsequently, the education and IQ Z-scores were unified into a single CR score by calculating the average of these Z-scores.
Measures
EF
The selected measures of EF were three widely used neuropsychological tests. The Trail Making Test (TMT) is a standardized executive test (Lezak, Howieson, Bigler & Tranel, Citation2012). The TMT-A involves the drawing of a line between numbers from 1 to 25 and is assumed to measure visual control and mental speed. The TMT-B involves drawing a line between numbers and letters. Participants are instructed to alternate numbers and letters in a sequence, that is, 1-A-2-B-3-C, continuing to L-13. The TMT-B measures cognitive flexibility and cognitive control, when compared to TMT-A. The time to complete each part of the TMT is recorded. The TMT B/A ratio is used as an executive measure (Oosterman et al., Citation2010). Furthermore, a verbal fluency test was used, as speeded word generation is also considered to measure EF (Lezak et al., Citation2012). In this study, the DAT Letter Fluency test was used (Schmand, Groenink & Van den Dungen, Citation2008). Participants were instructed to produce as many words starting with a specific letter as possible within a minute, using the letters, D, A, and T. The total number of words was calculated and used as performance score. The third measure was a working memory test: the Digit Span subtest from the Dutch version of the Wechsler Adult Intelligence Scale – Fourth Edition (Wechsler, Citation2008). The Digit Span subtest is a widely used, validated working memory test and consists of a forward, backward, and sequencing part. The total subtest score was used. The raw scores of each subtest were used as an executive measure. For the TMT B/A ratio, a higher score indicates worse performance; therefore, the TMT B/A ratio score was multiplied by −1, to adjust the direction of this score. Higher scores thus represent better performance on all tests.
The three executive measures were not combined into a factor score, as it is known that various executive tests measure different aspects of EF and correlate weakly among each other (Miyake, Friedman, Emerson, Witzki & Howerter, Citation2000). Accordingly, in the present study, only a small significant correlation was found between Digit Span and Letter Fluency (r = .25). However, when controlled for age, this correlation was nonsignificant (r = .16). No significant correlations were found between Digit Span and TMT B/A ratio (r = .20) or between Letter Fluency and TMB B/A ratio (r = .03).
Self-reported strategy use in daily life – Metamemory in Adulthood Questionnaire
The Metamemory in Adulthood questionnaire (MIA; Dixon, Hultsch & Hertzog, Citation1988) describes memory functioning and knowledge about memory processes. The MIA consists of seven subscales: Task, Capacity, Change, Anxiety, Achievement, Locus and Strategy. The Strategy subscale is divided into External Strategies and Internal Strategies. The subscale External Strategies includes memory aids, such as making a shopping list or using a calendar. The subscale Internal Strategies consists of internal mnemonics, such as visual imagery, forming associations, or mental rehearsal. In this study, we used the Strategy subscale of the abridged Dutch version of the MIA (Ponds & Jolles, Citation1996). Both Strategy subscales were found to have a reliable internal consistency, with a Cronbach’s alpha of .77 for External Strategies and .84 for Internal Strategies (Ponds & Jolles, Citation1996). Participants report how often they use a certain strategy by rating items on a 5-point Likert scale (1 = never, 2 = rarely, 3 = sometimes, 4 = often, 5 = always). Each strategy subscale has 8 items, resulting in a maximum score of 40 per subscale. A total score for each subscale was calculated per participant.
Strategy use in a simulated daily life situation – SOT
The SOT was developed in order to observe strategy use during a memory task that mimics daily life situations. Participants were instructed to remember a story that was played as an audio clip, in order to standardize the task as much as possible. Story C of the Dutch version of the Rivermead Behavioural Memory Test (RBMT; Wilson, Cockburn & Baddeley, Citation1985) was used for this purpose. This story consisted of 21 elements. Additionally, participants were asked to spontaneously recall the story exactly 5 min after the end of the audio clip. In the meantime, they were instructed to solve basic math problems. The instructions emphasized that participants should remember as much information as possible and that they were allowed to do or use anything to help them remember the information. A pen, paper, and an alarm clock were available within reaching distance. During the instruction, music was playing in the background. The volume of this music was preset at a level at which it was clearly distracting, but making sure that all instructions could still be understood. When asked, or at the start of the audio clip, the music was turned off. Strategic behavior of the participants was recorded on an observation list. Examples of possible strategies were asking questions about instructions, asking to turn off the music, taking notes or setting an alarm. The total number of observed strategies was calculated for each participant.
The recall of the story ideally took place 5 min after the end of the audio clip. When participants did not recall the story spontaneously 7 min after the end of the audio clip, they received a hint. Story recall was scored according to the scoring procedure of the RBMT. The story consisted of 21 elements. The total number of correctly recalled elements was used as the SOT recall score.
Since most internal strategies cannot be observed, a short self-report questionnaire was added to the task. After finishing the recall of the story, participants were asked which strategies they had used during this task. Subsequently, they were given a list with possible memory strategies that one could use to remember the content of a story. This strategy questionnaire used response options from the story paradigm of Saczynski et al. (Citation2007). Examples of strategies are repetition, visual imagery, or relating story to one’s own life. Participants were allowed to check as many strategies as they had used and were also given the opportunity to add a strategy that was not on the list. In the scoring procedure, the open answers were compared to the multiple choice answers. In case that someone mentioned the use of a strategy in the open answer but did not report this strategy in the multiple choice answer, this strategy was added to the multiple choice answers as “other.” The total number of self-reported strategies was calculated for each participant and added to the total number of observed strategies. For analyses, the total number of strategies on the SOT was used, including both observed and self-reported strategies. To examine the utility of the observation scale, additional analyses were performed with the number of observed strategies only.
Task-specific self-reported strategy use – Verbal Paired Associates
In addition to strategy use in daily life and a strategy task representing daily life situations, we also included a traditional memory task. Word-pair tasks are often used to examine spontaneous internal strategy use in laboratory settings (Dunlosky & Hertzog, Citation1998, Dunlosky & Hertzog, Citation2001; Kuhlmann & Touron, Citation2012; Naveh-Benjamin et al., Citation2007). In this study, the subtest Verbal Paired Associates (VPA I and II) from the Dutch version of the Wechsler Memory Scale – Fourth Edition (WMS-IV; Wechsler, Citation2009) was used. For VPA I, participants were instructed to remember a list of 14 word pairs. Subsequently, the first word of each pair was read out loud and participants were asked to recall the corresponding word. This list was repeated four times, each time in a different order. After VPA I, we administered a short questionnaire to examine self-reported strategy use. First, participants were openly asked which strategies they had used during the encoding of the word pairs. Subsequently, they were given a list with possible memory strategies that one could use to remember the word pairs and were asked to place a check mark next to each strategy that they had used. This strategy questionnaire used response options from the word-pair paradigm of Dunlosky and Hertzog (Citation1998, Citation2001) and from the word-list paradigm of Saczynski et al. (Citation2007). Examples of strategies are visual imagery, rehearsal of word pairs, creating a story or focusing on specific letters. Participants were allowed to check as many strategies as they had used and were also given the opportunity to add a strategy that was not on the list. In the scoring procedure, the open answers were compared to the multiple choice answers. In case that someone mentioned the use of a strategy in the open answer but did not report this strategy in the multiple choice answer, this strategy was added to the multiple choice answers as “other.” The total number of self-reported strategies was calculated for each participant.
Approximately 20 min after the encoding phase, participants performed VPA II, the recall phase of the task. Similar to the encoding phase, the first word of each word pair was read out loud and participants were asked to recall the corresponding word. The VPA I score consisted of the total correct encoded words, with a maximum of 56. The VPA II score consisted of the total number of correct recalled words, with a maximum of 14.
Procedure
This study was approved by the Ethics Committee Faculty of Social Sciences at the Radboud University in Nijmegen. Following recruitment, each participant underwent an initial telephone screening to check for exclusion criteria. When participants were eligible for inclusion in the study, a test session was planned. Participants were tested individually at home in a quiet room. Telephones were turned off and no other people were present during the test session. Participants received information about the study at the beginning of the test session, signed an informed consent form, and were asked for demographical information. As we aimed to examine spontaneous strategy use, participants were told that the goal of the study was to examine memory processes across the adult life span, without explicitly mentioning strategy use. After the session, participants were debriefed. Each test session had a duration of approximately 50 min.
Statistical analyses
For the statistical analyses, IBM SPSS 21.0 was used. Alpha was set at .05 for all analyses. Basic assumptions were checked and outliers with a score of >3 SD were removed. Based on these explorative analyses, the TMT B/A ratio scores of three participants were removed. First, common assumptions (i.e., the correlations between age, CR, and EF measures and their influence on memory performance scores) were checked using Pearson correlation coefficients and linear regression analyses. Second, the effects of age, CR, and EF measures on the total number of used strategies were examined using Pearson correlation coefficients. Explorative analyses showed that sex was positively correlated with MIA external strategies. Therefore, partial correlation analyses were performed on this strategy measure to control for sex. To determine which variables were reliable, unique predictors of strategy use, additional linear regression analyses were performed with age, CR, and EF measures as predictors and the various measures of strategy use as dependent variables. Furthermore, Pearson correlation coefficients were calculated to examine whether the use of specific strategies correlated with age, CR, and EF measures. For these analyses, specific strategies were dummy-coded, with a score of 0 representing the absence of use of a specific strategy, and a score of 1 indicating that the specific strategy was used. When multiple variables correlated significantly with a strategy, additional logistic regression analyses were performed with age, CR, and/or EF measures as predictors and the use of specific memory strategies as dependent variables, to determine which variables were reliable predictors of strategy use. The forward stepwise method was used. Finally, the number of strategies used was related to memory performance using Pearson correlation analyses. To examine which specific strategies were most effective in improving task performance, logistic regression analyses were performed, using the forward stepwise method. Standardized beta weights are reported for all regression analyses.
Results
Common assumptions (i.e., the correlations between age, CR, and EF measures) are shown in . Within the EF measures, only age was negatively related to the Digit Span measure. CR was positively related to all EF measures. Age and CR were unrelated. The effects of age, CR, and EF measures on memory performance are shown in . Age was negatively related to all memory scores. In other words, with increasing age, memory performance declined. Furthermore, CR was a positive predictor for the SOT recall score, but not for the VPA scores. Within the EF measures, only Digit Span was positively related to the SOT recall score. EF measures did not predict the VPA scores.
Table 1. Effects of age, cognitive reserve (CR), and executive function (EF) measures on memory performance.
Figure 2. Plots of correlations (Pearson) between age, cognitive reserve (CR), and the three executive function measures: Digit Span, Letter Fluency, and Trail Making Test B/A ratio (TMT B/A ratio).
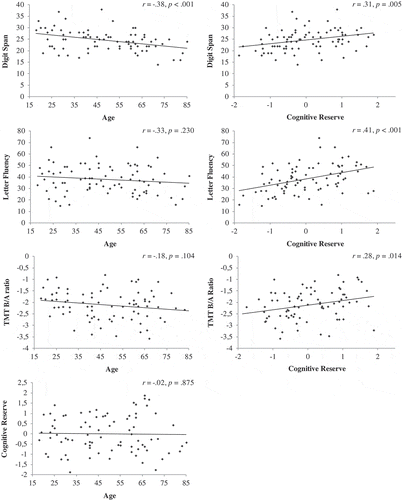
The effects of age, EF, and CR on the number of strategies used
shows the correlations between age, EF, CR and strategy measures. The self-reported strategy use in daily life (MIA) was not significantly correlated with EF measures or age. Moderate positive correlations (cf. Cohen, Citation1992) were present between CR and internal and external strategy use, indicating that participants with a higher CR use more strategies in daily life.
With respect to the SOT, the total number of strategies was negatively correlated with age. No significant correlation was found between the observed strategies and age, indicating that the negative correlation with age was based on the self-report part of the SOT. Both SOT measures were positively correlated with CR and with the Digit Span measure of EF, with a higher CR or higher scores on the Digit Span being associated with the use of more strategies. These correlations were small to moderate. The other EF measures were not significantly correlated with the SOT.
The number of self-reported strategies during encoding of the VPA was positively correlated with CR, in which a higher CR was related to more strategy use. No significant correlations were found with EF measures or with age.
shows the effects of age, CR, and EF measures on the various measures of strategy use. When age, CR, and EF measures were included in the model, only CR proved to be a reliable predictor for strategy use. Overall, a higher CR score predicted the use of more strategies on the MIA, during the SOT and the VPA. In addition, Letter Fluency was a negative predictor for the SOT total score, in which a higher score predicted the use of less strategy. No significant effects were found for age or other EF measures.
Table 2. Pearson correlations between age, executive function (EF) measures, cognitive reserve (CR), and strategy measures.
Table 3. Effects of age, cognitive reserve (CR), and executive function (EF) measures on strategy use.
The effects of age, EF, and CR on the use of specific memory strategies
The prevalence of use of specific memory strategies during the SOT and their correlations with age, CR, and EF measures are shown in . Of the observed strategies, taking notes was negatively correlated with age and positively with CR, Digit Span, and Letter Fluency. Logistic regression analysis showed that the EF measure Digit Span was the strongest predictor of the strategy taking notes (OR = 1.22, p = .005). The strategies setting an alarm and recalling the story fluently were positively correlated with CR and Digit Span. Logistic regression analyses showed that for setting an alarm, CR was the most important predictor (OR = 2.49, p = .002) and for recalling the story fluently, Digit Span was the most important predictor (OR = 1.19, p = .014). Of the self-reported strategies, EF measure Digit Span was negatively correlated with relating the story to one’s own life, indicating that adults with a higher level of working memory are less likely to use this strategy than adults with a lower level of working memory. Also, repetition of story was negatively related to EF measure Letter Fluency. CR and TMT B/A were positively related to picturing the story. Logistic regression analyses showed that for picturing the story, EF measure TMT B/A was the most important predictor (OR = 3.06, p = .014). Furthermore, CR was positively correlated with concentrating on the story and with other. Adults with higher levels of CR used these strategies more often than adults with lower levels of CR.
Table 4. Prevalence of use of observed and self-reported memory strategies during encoding of the strategy observation task (SOT) and their correlations (Pearson) with age, cognitive reserve (CR), and executive function (EF) measures.
The prevalence of use of specific memory strategies during the VPA word-pair task and their correlations with age, CR, and EF measures are shown in . Age correlated negatively with repetition of items, indicating that older adults use this strategy less often than younger adults. EF measure TMT B/A was positively correlated with “picturing items.” CR and Letter Fluency were positively correlated with creating associations between the words. Logistic regression analyses showed that for creating associations between the words, CR was the most important predictor (OR = 2.17, p = .006). CR was also positively correlated with creating a story with the words. Adults with higher levels of CR used these strategies more often than adults with lower levels of CR.
Table 5. Prevalence of use of self-reported memory strategies during encoding of the verbal paired associates (VPA) and their correlations (Pearson) with age, cognitive reserve (CR), and executive function (EF) measures.
The relation between strategy use and task performance
The total number of strategies (r = .36, p < .001) and the number of observed strategies (r = .44, p < .001) were positively correlated to the performance during the SOT recall. Using more strategies was related to a better task performance. Regarding VPA task performance, the number of self-reported strategy use was positively correlated with VPA I (r = .29, p = .009) and VPA II scores (r = .29, p = .008), showing that a larger number of strategies was related to a better task performance, both during encoding and during the recall phase of the task.
Furthermore, we examined which specific strategies were most effective for task performance. For the SOT, the following strategies predicted task performance: taking notes (β = .40, p < .001), concentrating on story (β = .27, p = .002), and recalling the information fluently (β = .25, p = .008) were positively related to task performance, whereas relating the story to one’s own life (β = −.20, p = .028) was negatively related to task performance. For the VPA I, the encoding of the word pairs, creating associations between words (β = .30, p = .006) and visual imagery (β = .27, p = .013), significantly predicted task performance. The strategies creating associations between words (β = .32, p = .003), sentence generation (β = .22, p = .028), and visual imagery (β = .22, p = .036) significantly predicted VPA II recall.
Discussion
This study examined the effect of age, EF, and CR on memory strategy use across the adult lifespan. The present study extended previous findings through some methodological improvements, such as including participants across the adult lifespan with matched educational attainment levels, comparing the effects of EF and CR directly and including multiple strategy measures: strategy use in daily life, observed and self-reported strategy use in a simulated daily life situation, and internal strategy use during a word-pair task. The goals of this study were to examine (1) the effect of age, EF, and CR on the number of strategies used, (2) the effect of age, EF, and CR on the use of specific strategies, and (3) the relation between strategy use and task performance.
First, this study showed that CR was the strongest predictor both for strategy use in daily life and for task-related strategy use. Although this study is the first to show this relationship directly, these findings are consistent with the results of Ponds and Jolles (Citation1996), who reported that a higher education level was related to more strategy use in daily life. In contrast to previous findings (Bouazzaoui et al., Citation2010; Dunlosky & Hertzog, Citation2001; Glisky et al., Citation2001; Naveh-Benjamin et al., Citation2007), the present study only showed a weak correlation and nonsignificant trends between age and strategy measures. Furthermore, the contribution of EF was small and limited to observed strategy use. Taken together, these findings suggest that CR, for which educational attainment and IQ are important proxies (Stern, Citation2009), plays a crucial role in strategy use. Previous studies on strategy use in aging included either participants with high education levels only (Dunlosky & Hertzog, Citation1998, Dunlosky & Hertzog, Citation2001; Naveh-Benjamin et al., Citation2007) or did not match younger and older age groups on education level, resulting in differences in or unknown CR levels between groups (Bailey et al., Citation2009; Bouazzaoui et al., Citation2010; Kuhlmann & Touron, Citation2012; Seaman et al., Citation2015; Touron et al., Citation2010). Therefore, it is possible that previously reported effects of age or EF are a result of variations in education level or solely are applicable to highly educated adults. Regarding EF, the present study confirmed the strong relation between the various EF measures and CR (Roldan-Tapia et al., Citation2012). Although we also found effects of EF on observed strategy use, these effects were mostly eliminated when CR was added to the model. This corroborates and extends previous results of studies including both CR and EF in relation to memory performance (Bruno et al., Citation2014; Giogkaraki et al., Citation2013).
Furthermore, this study examined the relation between age, EF, CR, and the use of specific memory strategies. Additionally, the relationship between strategy use and memory performance was confirmed (Dunlosky & Hertzog, Citation1998; Richardson, Citation1998; Rogers et al., Citation2000; Saczynski et al., Citation2007). With increasing age, participants less often use the strategy “taking notes,” whereas the strategy “repetition” was used more often. CR was positively related to “setting an alarm” or “using a clock,” which are effective strategies for prospective memory tasks (Maylor, Citation1990), and to “taking notes” and “other,” which mostly included focusing on specific elements of the story. Moreover, these strategies were most effective for task performance on the SOT. Furthermore, CR was positively related to visual imagery, creating associations and creating a story between words of each word pair. Despite the fact that we did not measure the complexity of the strategies directly, these findings suggest that CR may be mostly related to more complex strategies that require a deeper level of processing, such as visual imagery, creating associations or creating a story (Dunlosky & Hertzog, Citation1998; Hill et al., Citation1990). In turn, CR may not be related to strategies that do not require semantic processing, such as repetition or focusing on specific sounds or letters. In turn, many of these strategies (e.g., creating associations and visual imagery) were also important predictors for task performance. Therefore, it seems that CR is involved in the selection of effective strategies; however, it remains unclear whether CR also increases the efficacy of specific strategies. Future studies should examine the moderating role of CR on the efficacy of specific strategies on memory performance. In summary, CR seems to be related to the use of more internal and external strategies in daily life, to the application of more complex strategies and to the use of more effective strategies in memory tasks.
In addition to CR, this study also shows a positive relation between specific EF measures and strategy use. Our EF measures consisted of a working memory test, a verbal fluency test, and a flexibility test. Among these measures, working memory was the most sensitive measure for strategy use, suggesting that specifically working memory skills are of the utmost importance for strategy use. Furthermore, working memory was the only EF measure negatively related to age, which is consistent with the knowledge that working memory declines with aging (Van Geldorp et al., Citation2015). Although this finding may indicate that working memory plays an important role in the aging-related decline in strategy use, it may also merely indicate that working memory decline best reflects the effects of aging per se. Future studies should examine whether the aging-related decline in working memory indeed plays a unique role in the previously reported deficient strategy use in older adults (Shing et al., Citation2010).
The present findings clearly show that CR is involved in compensatory processes across the adult lifespan (cf. Stern, Citation2002, Citation2009). Individuals with a higher level of CR are better able to cope with aging-related cognitive decline by using other cognitive resources, such as memory strategies. EEG and imaging studies have confirmed that individuals with a higher CR use additional brain regions for encoding (Nyberg et al., Citation2003; Speer & Soldan, Citation2015; Steffener et al., Citation2011), suggesting the use of other cognitive processes to improve task performance. In addition, higher levels of education have been associated with increased cortical thickness, including the medial-frontal, temporal, and parietal lobes (Cox et al., Citation2016). Moreover, previous studies have shown that a higher CR reduces the risk of developing dementia (Dekhtyar et al., Citation2015; Stern, Citation2012), possibly because individuals who use efficient compensatory mechanisms are better able to compensate for potential disease progression. Since the use of compensatory mechanisms is crucial for memory functioning in daily life, another point of interest is whether interventions can increase strategy use in older adults. The present study has shown that CR plays an important role in the spontaneous production of memory strategies, which suggests that strategy training could be most beneficial for adults with lower levels of CR, who currently use less effective strategies. However, a study of Frankenmolen and colleagues (Citation2017) showed that specifically older adults with higher levels of intelligence benefited substantially from strategy instructions, whereas those with lower levels of intelligence did not. Both findings are consistent with the study of Nyberg et al. (Citation2003), which suggests that CR influences both the production efficiency (e.g., spontaneous strategy use) and the processing efficiency (e.g., efficient use of provides strategies) in older adults. Whether forms of strategy training are particularly effective in older adults with a higher or lower CR should be examined in further research.
Some limitations of this study must be noted. First, it is important to use multiple measures to define CR. The concept CR is often described as a capacity that is built through cognitively demanding and stimulating experiences, such as education (Reed et al., Citation2010). However, other studies suggest that a degree of literacy or verbal IQ might be a better marker for CR than the number of years of education (Alexander et al., Citation1997; Manly, Schupf, Tang & Stern, Citation2005). Therefore, some studies, including the present study, used a combination of education and (estimated) verbal IQ as proxy for CR (Barulli et al., Citation2013; Giogkaraki et al., Citation2013; Sakamoto et al., Citation2013). Although education level and verbal IQ are widely used, additional information on occupational attainment and engagement in cognitively stimulating leisure activities can provide a more accurate estimation of CR (Opdebeeck, Martyr & Clare, Citation2016), since these measures include lifetime experiences (Stern, Citation2009). In future studies, we recommend to use a combination of these measures to define CR. Second, in the present study, we used mostly self-reported measures of strategy use. Therefore, we cannot rule out the possibility that participants with higher levels of CR were more aware of their strategy use, without actually using them more often. Since memory strategies are not easily quantified, most studies rely on self-report measures (Bouazzaoui et al., Citation2010; Dunlosky & Hertzog, Citation1998; Hill et al., Citation1990; Saczynski et al., Citation2007). Only a few tasks include more objective strategy measures, such as the California Verbal Learning Test, in which a word list can be grouped into semantic categories (Delis, Kramer, Kaplan, & Ober, Citation1987), or a visual exploration task, in which exploration patterns can be recorded through mouse movements (Brandstatt & Voss, Citation2014). It would be interesting to also include more objective measures of strategy use in future research. However, these objective measures are often limited to a single strategy and ignore the metacognitive component of strategy use. We made a first attempt in developing a task (SOT) including observation of strategy use and a self-report questionnaire. Both the total strategy scale and the observed scale predicted task performance. Moreover, we confirmed that CR was not only related to self-reported strategy use but also to the observed strategies. Although further research in validating the SOT is needed, it seems to be a valuable measure to examine strategy use in a more objective way.
To summarize, this study is the first to show that CR plays a crucial role in the spontaneous production of memory strategies across the adult lifespan. The present study demonstrated that CR is related to more strategy use in daily life and during memory tasks. Moreover, CR is related to the use of more complex and more effective strategies. Therefore, higher levels of CR seem to enable individuals to use effective strategies to compensate for aging-related memory decline. This study highlights the importance of including measures of CR when examining memory functioning in aging.
Additional information
Funding
Notes on contributors
Nikita L. Frankenmolen
The authors declared that they had no conflicts of interest with respect to their authorship or the publication of this article.
References
- Alexander, G. E., Furey, M. L., Grady, C. L., Pietrini, P., Brady, D. R., Mentis, M. J., & Schapiro, M. B. (1997). Association of premorbid intellectual function with cerebral metabolism in Alzheimer’s disease: Implications for the cognitive reserve hypothesis. American Journal of Psychiatry, 154, 165–172.
- Bailey, H., Dunlosky, J., & Hertzog, C. (2009). Does differential strategy use account for age-related deficits in working-memory performance? Psychology and Aging, 24, 82–92.
- Barulli, D. J., Rakitin, B. C., Lemaire, P., & Stern, Y. (2013). The influence of cognitive reserve on strategy selection in normal aging. Journal of the International Neuropsycholical Society, 19, 841–844.
- Blumenfeld, R. S., & Ranganath, C. (2007). Prefrontal cortex and long-term memory encoding: An integrative review of findings from neuropsychology and neuroimaging. Neuroscientist, 13, 280–291. doi: 10.1177/1073858407299290
- Bouazzaoui, B., Angel, L., Fay, S., Taconnat, L., Charlotte, F., & Isingrini, M. (2014). Does the greater involvement of executive control in memory with age act as a compensatory mechanism? Canadian Journal of Experimental Psychology, 68, 59–66.
- Bouazzaoui, B., Isingrini, M., Fay, S., Angel, L., Vanneste, S., Clarys, D., & Taconnat, L. (2010). Aging and self-reported internal and external memory strategy uses: The role of executive functioning. Acta Psychologica, 135, 59–66.
- Brandstatt, K. L., & Voss, J. L. (2014). Age-related impairments in active learning and strategic visual exploration. Frontiers in Aging Neuroscience, 6, 19.
- Bruno, D., Brown, A. D., Kapucu, A., Marmar, C. R., & Pomara, N. (2014). Cognitive reserve and emotional stimuli in older individuals: Level of education moderates the age-related positivity effect. Experimental Aging Research, 40, 208–223.
- Cohen, J. (1992). A power primer. Psychological Bulletin, 112, 155–159.
- Cox, S. R., Dickie, D. A., Ritchie, S. J., Karama, S., Pattie, A., Royle, N. A., … Deary, I. J. (2016). Associations between education and brain structure at age 73 years, adjusted for age 11 IQ. Neurology, 87, 1820–1826.
- Delis, D. C., Kramer, J. H., Kaplan, E., & Ober, B. A. (1987). California verbal learning test: Adult version. Manual. San Antonio, TX: Psychological Corporation.
- Dekhtyar, S., Wang, H.-X., Scott, K., Goodman, A., Koupil, I., & Herlitz, A. (2015). A life-course study of cognitive reserve in dementia-from childhood to old age. American Journal of Geriatric Psychiatry, 23, 885–896.
- Dixon, R. A., & Hultsch, D. F. (1983). Structure and development of metamemory in adulthood. Journal of Gerontology, 38, 682–688.
- Dixon, R. A., Hultsch, D. F., & Hertzog, C. (1988). The Metamemory in Adulthood (MIA) questionnaire. Psychopharmacology Bulletin, 24, 671–688.
- Dunlosky, J., & Hertzog, C. (1998). Aging and deficits in associative memory: What is the role of strategy production?. Psychology and Aging, 13, 597–607.
- Dunlosky, J., & Hertzog, C. (2001). Measuring strategy production during associative learning: The relative utility of concurrent versus retrospective reports. Memory & Cognition, 29, 247–253.
- Frankenmolen, N. L., Altgassen, M., Kessels, R., de Waal, M., Hindriksen, J. A., Verhoeven, B., … Oosterman, J. (2017). Intelligence moderates the benefits of strategyinstructions on memory performance: An adult-lifespan examination. Aging, Neuropsychology and Cognition, 24, 45–61. doi:10.1080/13825585.2016.1171289
- Giogkaraki, E., Michaelides, M. P., & Constantinidou, F. (2013). The role of cognitive reserve in cognitive aging: Results from the neurocognitive study on aging. Journal of Clinical and Experimental Neuropsychology, 35, 1024–1035.
- Glisky, E. L., Rubin, S. R., & Davidson, P. S. (2001). Source memory in older adults: An encoding or retrieval problem? Journal of Experimental Psychology: Learning, Memory and Cognition, 27, 1131–1146.
- Hill, R. D., Allen, C., & Gregory, K. (1990). Self-generated mnemonics for enhancing free recall performance in older learners. Experimental Aging Research, 16, 141–145.
- Hinault, T., Lemaire, P., & Touron, D. (2016). Aging effects in sequential modulations of poorer-strategy effects during execution of memory strategies. Memory (Hove, England), 1–11. doi:10.1080/09658211.2016.1146300
- Kamp, S. M., & Zimmer, H. D. (2015). Contributions of attention and elaboration to associative encoding in young and older adults. Neuropsychologia, 75, 252–264.
- Kausler, D. H. (1994). Learning and memory in normal aging. New York, NY: Academic Press.
- Kuhlmann, B. G., & Touron, D. R. (2012). Mediator-based encoding strategies in source monitoring in young and older adults. Journal of Experimental Psychology: Learning, Memory and Cognition, 38, 1352–1364.
- Lezak, M. D., Howieson, D., Bigler, E., & Tranel, D. (2012). Neuropsychological assessment (5th ed.). New York, NY: Oxford University Press.
- Loewen, E. R., Shaw, R. J., & Craik, F. I. (1990). Age differences in components of metamemory. Experimental Aging Research, 16, 43–48.
- Lovelace, E. A., & Twohig, P. T. (1990). Healthy older adults’ perceptions of their memory functioning and use of mnemonics. Bulletin of the Psychonomic Society, 28, 115–118.
- Manly, J. J., Schupf, N., Tang, M. X., & Stern, Y. (2005). Cognitive decline and literacy among ethnically diverse elders. Journal of Geriatric Psychiatry and Neurology, 18, 213–217.
- Maylor, E. A. (1990). Age and prospective memory. Quarterly Journal of Experimental Psychology, 42, 471–493.
- Miyake, A., Friedman, P. F., Emerson, M. J., Witzki, A. H., & Howerter, A. (2000). The unity and diversity of executive functions and their contributions to complex ‘frontal lobe’ tasks: A latent variable analysis. Cognitive Psychology, 41, 49–100.
- Naveh-Benjamin, M., Brav, T. K., & Levy, O. (2007). The associative memory deficit of older adults: The role of strategy utilization. Psychology and Aging, 22, 202–208.
- Nelson, H. E. (1982). National Adult Reading Test (NART): Test manual. Windsor, UK: NFER-Nelson.
- Nilsson, L.-G. (2003). Memory function in normal aging. Acta Neurologica Scandinavica, 179, 7–13.
- Nyberg, L., Sandblom, J., Jones, S., Neely, A. S., Petersson, K. M., Ingvar, M., & Backman, L. (2003). Neural correlates of training-related memory improvement in adulthood and aging. Proceedings of the National Academy of Sciences of the United States of America, 100, 13728–13733.
- Oosterman, J. M., Vogels, R. L., van Harten, B., Gouw, A. A., Poggesi, A., Scheltens, P., … Scherder, E. J. (2010). Assessing mental flexibility: Neuroanatomical and neuropsychological correlates of the Trail Making Test in elderly people. The Clinical Neuropsychologist, 24, 203–219.
- Opdebeeck, C., Martyr, A., & Clare, L. (2016). Cognitive reserve and cognitive function in healthy older people: A meta-analysis. Aging, Neuropsychology and Cognition, 23, 40–60.
- Ponds, R. W., & Jolles, J. (1996). The abridged dutch Metamemory in Adulthood (MIA) questionnaire: Structure and effects of age, sex, and education. Psychology and Aging, 11, 324–332.
- Rankin, J. L., Karol, R., & Tuten, C. (1984). Strategy use, recall, and recall organization in young, middle-aged, and elderly adults. Experimentl Aging Research, 10, 193–196.
- Reed, B. R., Mungas, D., Farias, S. T., Harvey, D., Beckett, L., Widaman, K., … DeCarli, C. (2010). Measuring cognitive reserve based on the decomposition of episodic memory variance. Brain : A Journal of Neurology, 133, 2196–2209.
- Richardson, J. T. E. (1998). The availability and effectiveness of reported mediators in associative learning: A historical review and an experimental investigation. Psychonomic Bulletin & Review, 5, 597–614.
- Rogers, W. A., Hertzog, C., & Fisk, A. D. (2000). An individual differences analysis of ability and strategy influences: Age-related differences in associative learning. Journal of Experimental Psychology: Learning, Memory and Cognition, 26, 359–394.
- Roldan-Tapia, L., Garcia, J., Canovas, R., & Leon, I. (2012). Cognitive reserve, age, and their relation to attentional and executive functions. Applied Neuropsychology: Adult, 19, 2–8.
- Saczynski, J. S., Rebok, G. W., Whitfield, K. E., & Plude, D. L. (2007). Spontaneous production and use of mnemonic strategies in older adults. Experimental Aging Research, 33, 273–294.
- Sakamoto, M., Woods, S. P., Kolessar, M., Kriz, D., Anderson, J. R., Olavarria, H., … Huckans, M. (2013). Protective effects of higher cognitive reserve for neuropsychological and daily functioning among individuals infected with hepatitis C. Journal of Neurovirology, 19, 442–451.
- Schmand, B., Groenink, S. C., & Van den Dungen, M. (2008). Letterfluency: Psychometrische eigenschappen en Nederlandse normen [Letter fluency: Psychometric properties and Dutch normative data]. Tijdschrift Voor Gerontologie En Geriatrie, 39, 64–76.
- Schmand, B., Lindeboom, J., & Harskamp, F. (1992). De Nederlandse leestest voor volwassenen. [The Dutch reading test for adults]. Lisse, Nederlands: Swets & Zeitlinger.
- Seaman, K. L., Howard, D. V., & Howard, J. H., Jr. (2015). Adult age differences in subjective and objective measures of strategy use on a sequentially cued prediction task. Aging, Neuropsychology and Cognition, 22, 170–182.
- Shing, Y. L., Werkle-Bergner, M., Brehmer, Y., Muller, V., Li, S. C., & Lindenberger, U. (2010). Episodic memory across the lifespan: The contributions of associative and strategic components. Neuroscience and Biobehavioral Reviews, 34, 1080–1091.
- Speer, M. E., & Soldan, A. (2015). Cognitive reserve modulates ERPs associated with verbal working memory in healthy younger and older adults. Neurobiology of Aging, 36, 1424–1434.
- Steffener, J., Reuben, A., Rakitin, B. C., & Stern, Y. (2011). Supporting performance in the face of age-related neural changes: Testing mechanistic roles of cognitive reserve. Brain Imaging and Behavior, 5, 212–221.
- Stern, Y. (2002). What is cognitive reserve? Theory and research application of the reserve concept. Journal of the International Neuropsychological Society, 8, 448–460.
- Stern, Y. (2009). Cognitive reserve. Neuropsychologia, 47, 2015–2028.
- Stern, Y. (2012). Cognitive reserve in ageing and Alzheimer’s disease. Lancet Neurology, 11, 1006–1012.
- Taconnat, L., Baudouin, A., Fay, S., Clarys, D., Vanneste, S., Tournelle, L., & Isingrini, M. (2006). Aging and implementation of encoding strategies in the generation of rhymes: The role of executive functions. Neuropsychology, 20, 658–665.
- Taconnat, L., Raz, N., Tocze, C., Bouazzaoui, B., Sauzeon, H., Fay, S., & Isingrini, M. (2009). Ageing and organisation strategies in free recall: The role of cognitive flexibility. European Journal of Cognitive Psychology, 21, 347–365.
- Touron, D. R., Oransky, N., Meier, M. E., & Hines, J. C. (2010). Metacognitive monitoring and strategic behaviour in working memory performance. Quarterly Journal of Experimental Psychology (Hove), 63, 1533–1551.
- UNESCO Institute for Statistics. (2012). International Standard Classification Of Education (ISCED 2011). Montreal, Canada: Author.
- Van Geldorp, B., Heringa, S. M., Van den Berg, E., Olde Rikkert, M. G., Biessels, G. J., & Kessels, R. P. (2015). Working memory binding and episodic memory formation in aging, mild cognitive impairment, and Alzheimer’s dementia. Journal of Clinical and Experimental Neuropsychology, 37, 538–548.
- Wechsler, D. (2008). Wechsler Adult Intelligence Scale – Fourth Edition (WAIS-IV). San Antonio, TX: The Psychological Corporation.
- Wechsler, D. (2009). Wechsler Memory Scale-fourth edition (WMS-IV). San Antonio, TX: Pearson.
- Wilson, B. A., Cockburn, J., & Baddeley, A. D. (1985). The rivermead behavioural memory test. Titchfield, UK: Thames Valley Test Company.