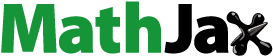
Abstract
We introduce two classes of point processes: a fractional non-homogeneous Poisson process of order k and a fractional non-homogeneous Pólya-Aeppli process of order k. We characterize these processes by deriving their non-local governing equations. We further study the covariance structure of the processes and investigate the long-range dependence property.
1. Introduction
Fractional Poisson processes (FPP) enjoy the property of non-stationarity and long range dependence, which makes them an attractive modeling tool. These processes are widely used in statistics, finance, meteorology, physics and network science, see for instance (Baleanu et al. Citation2012) p. 332, and (Kumar, Leonenko, and Pichler Citation2020).
Fractional Poisson processes were introduced as renewal processes in Mainardi, Gorenflo, and Scalas (Citation2004). The authors generalized the characterization of the Poisson process as the counting process for epochs defined as sum of independent non-negative exponential random variables, and, instead of the exponential, the authors used a Mittag-Leffler distribution. The theory of FPP was further developed by Beghin and Orsingher (Citation2009, Citation2010) and by Meerschaert, Nane, and Vellaisamy (Citation2011).
In particular, Meerschaert, Nane, and Vellaisamy (Citation2011) defined FPP by means of a time-change for the Poisson process N(t), where the time variable t is replaced by the inverse α-stable subordinator Remarkably, they could prove the equality in distribution between
and the counting process defined by (Mainardi, Gorenflo, and Scalas Citation2004).
Leonenko, Scalas, and Trinh (Citation2017) used the same time-change technique to introduce a non-homogeneous fractional Poisson process (NFPP) by replacing the time variable in the FPP with an appropriate function of time.
In a recent paper, Gupta, Kumar, and Leonenko (Citation2020) and Gupta and Kumar (Citation2021) generalize the results available on fractional Poisson processes using the z-transform technique.
Kostadinova and Minkova (Citation2019) introduced a Poisson process of order with insurance modeling in mind. This process models the claim arrival in groups of size k, where the number of arrivals in a group is uniformly distributed over k points.
The Pólya-Aeppli process of order k was studied in Chukova and Minkova (Citation2015) and later by Kostadinova and Lazarova (Citation2019). In this process, the uniform distribution on the integers is replaced by the truncated geometric distribution of parameter ρ.
To deal with dependent inter-arrival times, a generalization of Poisson processes of order k was proposed by Sengar, Maheshwari, and Upadhye (Citation2020). These authors extended the Poisson process of order k by means of time change with a general Lévy subordinator as well as an inverse Lévy subordinator.
Here, we combine the compound Poisson processes of order k and fractional Poisson processes, namely we study a fractional non-homogeneous Poisson process of order k and a fractional non-homogeneous Pólya-Aeppli process of order k (see the definitions below). First, we generalize the results of Kostadinova and Minkova (Citation2019) by considering a non-homogeneous Poisson process of order k. Then, we generalize the results of Sengar, Maheshwari, and Upadhye (Citation2020) by introducing the time non-homogeneity in the fractional Poisson process of order k. Finally, we study a non-homogeneous fractional Pólya-Aeppli process of order k.
This paper is organized as follows. Section 2 collects some known results from the theory of subordinators and provides the definition of the compound distributions of order k. In Section 3, we consider a non-homogeneous fractional Poisson process of order k. We obtain the governing equations and calculate the moments and the covariance function of the process. Section 4 is devoted to a non-homogeneous fractional Pólya-Aeppli process of order k. We derive the non-local governing equations for the marginal distributions of these processes, using non-local operators known as Caputo derivatives. The moments and the covariance structure of the processes are derived, as well.
2. Preliminaries
This section presents known results in the theory of subordinators and provides the definition of the compound distributions of order k.
2.1. Compound distributions of order k
Consider a random variable that can be represented as a random sum where
is a sequence of independent identically distributed random variables (i.i.d. r.v’s), independent of a non-negative integer-valued random variable Y. The probability distribution of N is called compound distribution and the distribution of X1 is called compounding distribution.
A well-known and widely used example is the compound Poisson distribution, where Y has a Poisson distribution. If then the random variable N has a compound discrete distribution of order k.
Compound discrete distributions of order k were studied by Philippou (Citation1983) and Philippou, Georghiou, and Philippou (Citation1983).
As mentioned previously, in this paper we deal with two types of compounding distributions: the discrete uniform distribution and the truncated geometric distribution. They respectively induce the Poisson distribution of order k and the Pólya-Aeppli distribution of order k as will be shown in the following. We say that the random variable X is uniformly distributed over k points if its probability mass function (pmf) is of the form
(1)
(1)
Its probability generating function (pgf) is
The random variable X has a truncated geometric distribution with parameter ρ and with success probability if
(2)
(2)
Consequently, the pgf of X is given by
(3)
(3)
Note, that for the truncated geometric distribution asymptotically coincides with the geometric distribution with parameter
We can now define the Poisson distribution of order k.
Definition 1
(Poisson distribution of order k). The random variable N has Poisson distribution of order k with parameter Λ if where:
(1) are the i.i.d. r.v’s with the uniform distribution; (2) Y has Poisson distribution with parameter
(3) Y and
are independent.
Note that
where
and
(4)
(4)
The pgf of the Poisson distribution of order k is
(5)
(5)
Note that where
are independent copies of Poisson random variable Y with parameter Λ, and “
“stands for equality in distributions. We now introduce the Pólya-Aeppli distribution of order k as a compound Poisson distribution (see (Minkova Citation2010)).
Definition 2
(Pólya-Aeppli distribution of order k). The random variable N has Pólya-Aeppli distribution of order k with parameter if
where: (i)
are the i.i.d. r.v’s with the truncated geometric distribution with parameter
given by (2); (ii) Y has Poisson distribution with parameter
(iii) Y and
are independent.
Note that the probability generating function of N is where
is given by (3).
The probability mass function of Pólya-Aeppli distribution of order k is defined by (see Minkova Citation2010, Theorem 3.1):
(6)
(6)
where
and
2.2. Inverse 
-stable subordinator
Let be a
-stable Lévy subordinator, that is Lévy process with Laplace transform:
Then the inverse α-stable subordinator (see e.g., Meerschaert and Sikorskii Citation2019, 103) is defined as the first passage time of
(7)
(7)
We will use the following properties of the inverse α-stable subordinator:
The density of
is of the form (see (Meerschaert and Sikorskii Citation2019) p.113):
(8)
(8)
where
is the density of
(see e.g. (Kataria and Vellaisamy Citation2018)).
The Laplace transform
(9)
(9)
The moments of the inverse α-stable subordinator are as follows:
(10)
(10)
(see e.g. (Kataria and Vellaisamy Citation2018, 1640).
The covariance function (see (Leonenko et al. Citation2014; Leonenko, Scalas, and Trinh Citation2017)) is
(11)
(11)
3. Poisson processes of order k
The Poisson process of order k was introduced in Kostadinova and Minkova (Citation2019), see also (Sengar, Maheshwari, and Upadhye Citation2020).
Definition 3.
The Poisson process of order k (PPk) is defined as a compound Poisson process with the compounding discrete uniform distribution:
(12)
(12)
where (1) Xi are independent copies of a discrete uniform random variable distributed over k points given by (1); (2)
is the Poisson process with parameter
(3) N1 and
are independent.
The following Kolmogorov forward equations are valid for
(13)
(13)
(14)
(14)
with the initial condition
and
The pgf is of the form:
and the first two moments are given by
(15)
(15)
3.1. Fractional Poisson process of order k
In this sub-section we shall derive governing equations for a fractional Poisson process of order k and we shall investigate its long-range dependence properties. It is worth noting that Sengar, Maheshwari, and Upadhye (Citation2020) studied the Poisson process of order k time-changed by a general Lévy subordinator and its inverse. However, among their examples, they did not explicitly consider the governing equations for the inverse α-stable subordinator (this particular process is studied in Gupta and Kumar (Citation2021)). That is why we specify some formulae of Sengar, Maheshwari, and Upadhye (Citation2020) that will be used in the following sub-sections. In particular, below, we use EquationEquation (10)(10)
(10) to derive the marginal distributions of the fractional Poisson process of order k.
Definition 4.
(Fractional Poisson process of order k). The process is called fractional Poisson process of order k (FPPk) if
(16)
(16)
where (1)
is the inverse α-stable subordinator, given by (7); (2) N is the Poisson process of order k, given by (12); (3)
and N are independent.
The marginal distributions of the FPPk process is given by
where
and
is defined in (4).
Also
where the variance and covariance of the process
are given by (10) and (11).
3.1.1. Correlation structure and long-range dependence
There exist many definitions of the long-range dependence property. Here, we shall use the definition given in Biard and Saussereau (Citation2014).
Definition 5.
The process has a long-range dependence property (LRD) if for fixed s and some c(s) and
where
is the correlation function of the process
We now investigate the asymptotic behavior of the correlation function of the FPPk process defined by (16).
Theorem 3.1.
The process has the LRD property.
Proof.
Using the result of Leonenko et al. (Citation2014) we have that for a fixed s > 0
where
and
and
are given by (15).
3.1.2. Governing equations
In the sequel we will employ the fractional Caputo (or Caputo-Djrbashian) derivative which is defined as follows (see e.g., (Meerschaert and Sikorskii Citation2019, 30)
(17)
(17)
Theorem 3.2.
The governing fractional difference-differential equations for
are given by
(18)
(18)
(19)
(19)
with the initial condition
Note, that by setting we get the governing equations of the Poisson process of order k given in EquationEquation (13)
(13)
(13) .
Proof.
Note that
(20)
(20)
and remember that
(21)
(21)
We first consider the case By taking the fractional Caputo derivative of both sides (21) and using property (20), we get
For n = 0 we have
Remark 1.
Note that Sengar, Maheshwari, and Upadhye (Citation2020) derived governing equations in which the Caputo derivative is replaced by a more general non-local operator. We present the proof of Theorem 3.2 for the sake of completeness.
3.2. Non-homogeneous fractional Poisson process of order k
We now generalize the fractional Poisson process of order k by introducing a deterministic, time dependent intensity or rate function such that for every fixed
the cumulative rate function follows the following equation
Denote Let
be a homogeneous Poisson process (HPP) of unit intensity, and
be a non-homogeneous Poisson process (NPP) with rate function
then
is non-homogeneous Poisson process of order k (NPPk), with rate function
where
are the i.i.d.r.v’s with the uniform distribution on
independent of
The mgf of the process Nn is of the form:
The process Nn has the following distributions of its increments:
(22)
(22)
Incidentally, this model includes Weibull’s rate function: Makeham’s rate function:
and many others.
We define a non-homogeneous fractional Poisson process of order k (FNPPk) as
(23)
(23)
where
is the inverse α-stable subordinator (7), independent of the NPPk process
3.2.1. Marginal distributions
Define the increment process: Its marginal distributions can be written as follows:
(24)
(24)
where
is the density of the inverse α-stable subordinator (8) and
is given by (22). Consequently the marginal distributions of
are given by
For the NFPP of order
Leonenko et al. (Leonenko, Scalas, and Trinh Citation2017) derived the governing equations for the marginal distributions
of the corresponding increment process
of NFPP (of order k = 1), where
is the homogeneous Poisson process of intensity 1. We shall derive the governing equations for the marginal distributions
of FNPPk.
Theorem 3.3.
The marginal distributions satisfy the following fractional differential-difference integral equations
(25)
(25)
with the initial condition:
where
is given by (22).
Proof.
Note that the mgf of is of the form
while the Laplace transform with respect to t of
is given by (9). Taking both the mgf and the Laplace transform in (24) as above, we have
(26)
(26)
Note that for we have
(27)
(27)
Thus, integrating (26) by parts with U as above, and we get
(28)
(28)
We shall use the following property of the Caputo derivative:
where
stands for the Laplace transform of function
Note that
since
a.s. Hence, by (28)
Inverting the Laplace transform yields
where the mgf
Finally, by inverting the mgf we obtain:
since the mgf of
is equal to
3.2.2. Covariance structure
One can show that for NPPk and its covariance function is
Then the mean and covariance function of FNPPk are given by
4. Pólya-Aeppli process of order k
The Pólya-Aeppli process of order k was defined and studied in the context of ruin problems in Chukova and Minkova (Citation2015) and later by Kostadinova and Lazarova (Citation2019). Related pure fractional birth processes were studied in Orsingher and Polito (Citation2010).
Definition 6.
The process is said to be the Pólya-Aeppli process of order k (PAk) if
where (i) the random variables Xi are i.i.d with the truncated geometric distribution of parameter
given by (2); (ii)
is a homogeneous Poisson process (HPP) with intensity
independent of
The following Kolmogorov forward equations are valid for the marginal distributions
(29)
(29)
where
The marginal distributions of the PAk process are given by
(30)
(30)
where qm are given by (6).
More explicit expressions for can be found in Minkova (Citation2010). The expectation ad variance are as follows:
(31)
(31)
Note that PAk process is a compound Poisson process with the pgf
where
is given by (3).
4.1. Non-homogeneous Pólya-Aeppli process of order k
We now consider a non-homogeneous version by introducing a deterministic time dependent intensity function as above, and
Definition 7.
(Non-homogeneous Pólya-Aeppli process of order k). We define a non-homogeneous Pólya-Aeppli process of order k with cumulative rate function and parameter ρ as
(32)
(32)
where (i)
is a non-homogeneous Poisson process (NPP) with cumulative rate function
(ii) Xi are i.i.d. r.v’s following the truncated geometric distribution with parameter
given by (3); (iii)
is independent from
Note, that the random variable has the Pólya-Aeppli distribution of order k with parameters
that is
(33)
(33)
where qm are given by (6).
Then the marginal distributions of the process are
An alternative definition can be given in terms of transition probabilities.
Definition 8.
The counting process is said to be a non-homogeneous Pólya -Aeppli process of order k with the rate function
and parameter
if (1)
(2)
has independent increments; (3) for all
(34)
(34)
It is easy to verify that the previous two definitions are equivalent.
4.1.1. Marginal distributions of the process
The following theorem holds.
Theorem 4.1.
The functions satisfy the differential equation:
(35)
(35)
Proof.
We first consider the case By fixing u and taking a small h we can write
Thus
Letting yields
For we have
Letting yields
which was the statement of the theorem. □
Note that in case the Pólya-Aeppli process
coincides with the non-homogeneous Pólya-Aeppli process defined in Chukova and Minkova (Citation2019), but for fixed k the Pólya-Aeppli process
is new.
4.2. Fractional Pólya-Aeppli process of order k
To the best of our knowledge, fractional versions of PAk processes have not been considered yet. We define a fractional Pólya-Aeppli process of order as a Pólya-Aeppli process of order k time-changed by the process
such that
(36)
(36)
where (i)
is the homogeneous Poisson process with intensity
(ii)
(iii)
is the inverse α-stable subordinator, defined in (7) and independent of
4.2.1. Marginal distributions
We shall now obtain governing equations for the marginal distributions of the fractional PAk process
where
is given by (30).
Theorem 4.2.
The probabilities satisfy the fractional differential-difference equations:
(37)
(37)
(38)
(38)
where
is the fractional Caputo derivative of the function f given by (17).
Proof.
We first consider the case By taking the fractional Caputo derivative of both sides in (29) and using the property (20), we get
For m = 0 we have
4.3. Correlation structure and long-range dependence property
In this sub-section we shall obtain several important characteristics of the fractional Pólya-Aeppli process of order k such as its expectation, variance and covariance. After that, we are able to study the correlation structure of the process. For the fractional Pólya-Aeppli process of order k, we can use the property of the conditional expectation to write (see (Leonenko et al. Citation2014))
The covariance function can be calculated via the formula:
where the covariance of the process
is given by EquationEquation (11)
(11)
(11) .
Theorem 4.3.
The process has the LRD property.
Proof.
Using the results from (Leonenko et al. Citation2014) similarly to the previous section, we get
where
and
are given by (31). Thus the correlation function of FPAk process decays at rate
and satisfies the LRD property. □
4.4. Non-homogeneous fractional PAk process
As we did before, we can now define a non-homogeneous fractional Pólya-Aeppli process of order k as
where all the symbols have the usual meaning defined above. We assume that the inverse subordinator
is independent of the process
In this sub-section, we shall derive governing equations for the probabilities
Theorem 4.4.
The marginal distributions satisfy the following fractional differential-difference integral equations
(39)
(39)
(40)
(40)
with the initial condition
where
is given by (33).
Proof.
Using (9), the mgf of can be written in the form:
while the Laplace transform with respect to t of
is given by (9). Taking both the mgf and the Laplace transform in (24) as above, we have
(41)
(41)
Note that for one can take derivative in u as follows:
(42)
(42)
Thus, integrating (41) by parts with
we get
(43)
(43)
where
since
a.s. Hence, by (43)
Inverting the Laplace transform yields
where the mgf is
Finally, by inverting the mgf
we obtain:
□
5. Final notes
The counting processes of order k that we have discussed in this paper have this general form
(44)
(44)
where
is a sequence of i.i.d. integer random variables assuming values in
and
is a counting process independent from the sequence. One further assumes that
A simple algorithm in R is given in arXiv:2008.09421 [math.PR] when
is the fractional Poisson process of renewal type used above and discussed by Mainardi, Gorenflo, and Scalas (Citation2004) and when X1 is uniformly distributed in
Acknowledgments
The authors thank Mr. Mostafizar Khandakar and Dr. Kuldeep Kumar Kataria for their thorough reading of the manuscript that highlighted some typos and a wrong formula. Enrico Scalas acknowledges partial support from the Dr Perry James (Jim) Browne Research Center at the Department of Mathematics, University of Sussex.
References
- Baleanu, D., K. Diethelm, E. Scalas, and J. J. Trujillo. 2012. Fractional calculus: Models and numerical methods. In Series on Complexity, Nonlinearity and Chaos Book Vol. 5, 2nd ed. Albemarle, NC: WSPC.
- Beghin, L., and E. Orsingher. 2009. Fractional Poisson processes and related planar random motions. Electronic Journal of Probability 14:1790–827. doi: 10.1214/EJP.v14-675.
- Beghin, L., and E. Orsingher. 2010. Poisson-type processes governed by fractional and higher-order recursive differential equations. Electronic Journal of Probability 15:684–709. doi: 10.1214/EJP.v15-762.
- Biard, R., and B. Saussereau. 2014. Fractional Poisson process: Long-range dependence and applications in ruin theory. Journal of Applied Probability 51 (3):727–40. Correction: 2016. J. Appl. Prob. 53: 1271–1272. doi: 10.1017/jpr.2016.80.
- Chukova, S., and L. Minkova. 2015. Pólya-Aeppli of order k risk model. Communications in Statistics - Simulation and Computation 44 (3):551–64. doi: 10.1080/03610918.2013.784987.
- Chukova, S., and L. Minkova. 2019. Non-homogeneous Pólya-Aeppli process. Communications in Statistics - Simulation and Computation 48 (10):2955–67. doi: 10.1080/03610918.2018.1469763.
- Gupta, N., and A. Kumar. 2021. Fractional Poisson processes of order k and beyond. arXiv:2008.06022 [Math.PR].
- Gupta, N., A. Kumar, and N. Leonenko. 2020. Tempered fractional Poisson processes and fractional equations with Z-transform. Stochastic Analysis and Applications 38 (5):939–57. doi: 10.1080/07362994.2020.1748056.
- Kataria, K. K., and P. Vellaisamy. 2018. On densities of the product, quotient and power of independent subordinators. Journal of Mathematical Analysis and Applications. 462 (2):1627–43. doi: 10.1016/j.jmaa.2018.02.059.
- Kostadinova, K., and M. Lazarova. 2019. Risk models of order k. Ann. Acad. Rom. Sci. Ser. Math. Appl 11 (2):259–73.
- Kostadinova, K., and L. Minkova. 2019. On the Poisson process of order k. Pliska Stud. Math. Bulgar 22:117–28.
- Kumar, A., N. Leonenko, and A. Pichler. 2020. Fractional risk process in insurance. Mathematics and Financial Economics 14 (1):43–65. doi: 10.1007/s11579-019-00244-y.
- Leonenko, N., M. M. Meerschaert, R. Schilling, and A. Sikorskii. 2014. Correlation structure of time changed Lévy proceses. Communications in Applied and Industrial Mathematics 6 (1):483–505.
- Leonenko, N., E. Scalas, and M. Trinh. 2017. The fractional non-homogeneous Poisson process. Statistics & Probability Letters 120:147–56. doi: 10.1016/j.spl.2016.09.024.
- Mainardi, F., R. Gorenflo, and E. Scalas. 2004. A fractional generalization of the Poisson processes. Vietnam Journal of Mathematics 32:53–64.
- Meerschaert, M. M., E. Nane, and P. Vellaisamy. 2011. Fractional Poisson process and the inverse stable subordinator. Electronic Journal of Probability 16 (none):1600–20. doi: 10.1214/EJP.v16-920.
- Meerschaert, M. M., and A. Sikorskii. 2019. Stochastic Models For Fractional Calculus. De Gruyter Studies in Mathematics, Vol. 43, 2nd ed. Berlin: Walter de Gruyter.
- Minkova, L. D. 2010. The Pólya-Aeppli distribution of order k. Communications in Statistics - Theory and Methods 39 (3):408–15. doi: 10.1080/03610920903140072.
- Orsingher, E., and F. Polito. 2010. Fractional pure birth processes. Bernoulli 16 (3):858–81. doi: 10.3150/09-BEJ235.
- Philippou, A. N. 1983. The Poisson and compound Poisson distribution of order k and their properties. Zapiski Nauchnyh Seminarov Instituta im. Steklova 130:175–80.
- Philippou, A. N., C. Georghiou, and G. N. Philippou. 1983. A generalized geometric distribution and some of its properties. Statistics & Probability Letters 1 (4):171–5. doi: 10.1016/0167-7152(83)90025-1.
- Sengar, A. S., A. Maheshwari, and N. S. Upadhye. 2020. Time-changed Poisson process of order k. Stochastic Analysis and Applications 38 (1):124–48. doi: 10.1080/07362994.2019.1653198.